A global logistics company's general AI predictive system crashed during a major port strike. It left them unable to adapt as shipments piled up and costs skyrocketed. The static models couldn't handle the complexity of real-time rerouting decisions across multiple carriers and regions. So, they have become useless when CxOs need intelligence the most. Operations could be maintained during disruptions only after implementing AI-powered workflow automation with dynamic decision-making capabilities. These agents continuously monitored conditions, negotiated with stakeholders, and executed complex logistics workflows without human bottlenecks. If you think this is your case, then arrange a call.
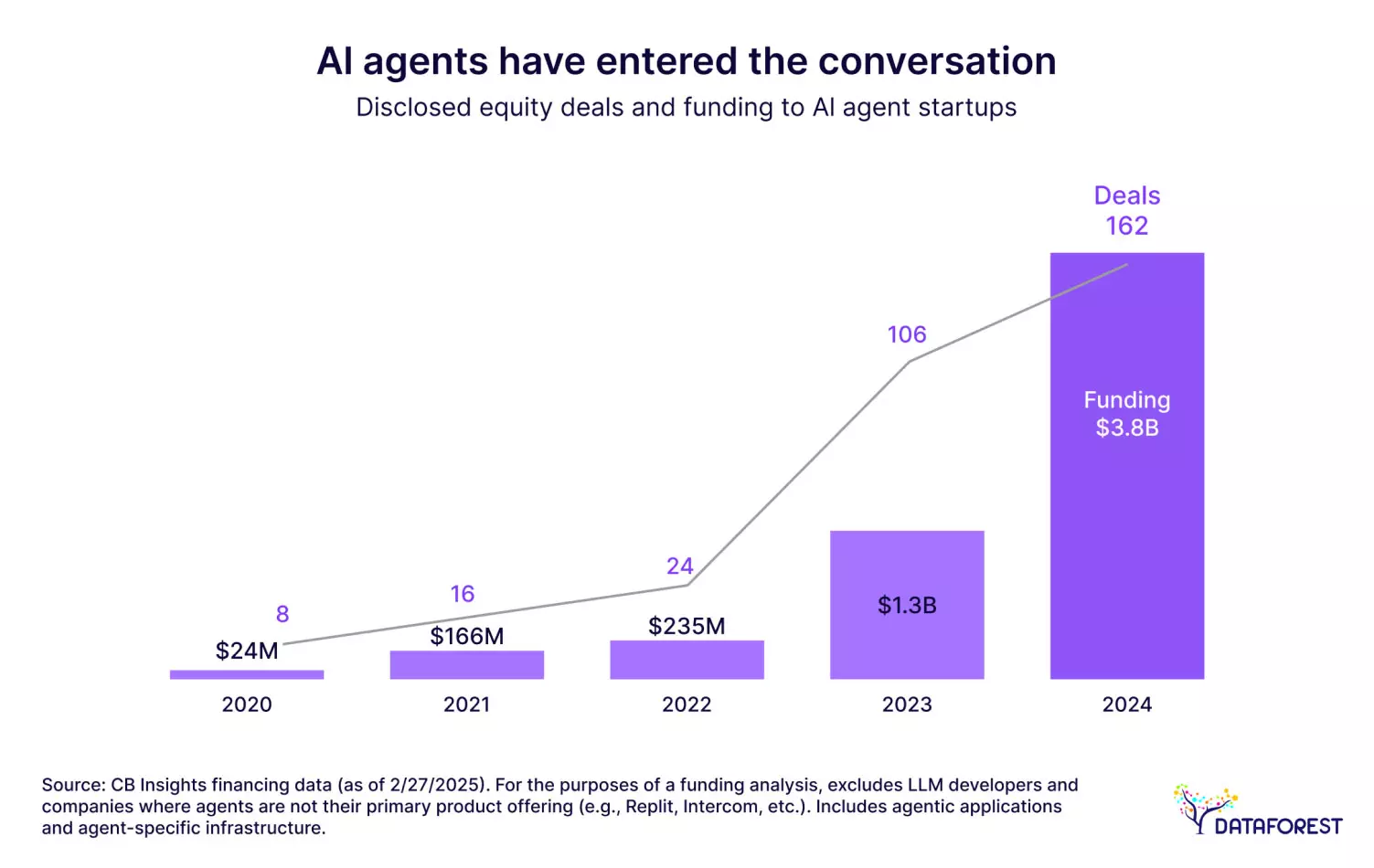
Key Industries Most Benefiting from AI-Driven Business Transformation
Healthcare changes patient care with an AI-powered customer experience that continuously monitors vital signs, adjusts real-time treatment protocols, and coordinates between specialists without human delay. Financial services firms are deploying predictive analytics tools for algorithmic trading, fraud detection, and personalized banking experiences that instantaneously adapt to customer behavior patterns. Manufacturing operations are achieving unprecedented efficiency through AI automation services that manage entire production lines, predict maintenance needs, and reconfigure processes based on supply chain disruptions. Logistics and transportation companies rely on cloud-based AI services to optimize routing across global networks, negotiate with carriers automatically, and respond to weather events or port closures without human intervention. Customer service departments across all sectors implement intelligent virtual agents that handle interactions, learn from customer sentiment, and escalate appropriately while maintaining context across communication channels. Book a call if you want to always be on the cutting edge of technology.
Considering Factors When Choosing an AI Agent Development Company
Don't rush into picking any AI chatbot developers — these systems will become the brains behind the operation, making thousands of decisions when you're not in the room. You need a partner who doesn't talk a big game about machine learning models and deep learning applications but one who's been in the trenches of your industry and can build agents that solve your specific problems rather than forcing you into their cookie-cutter approach.
Technical Expertise and Industry Experience
Dig into whether the company truly understands natural language processing (NLP) because these engines will power your AI agents when making critical decisions on their own. Ask tough questions about their experience in your industry — have they built agents that can navigate your specific regulatory landscape, or will they be learning on your dime? A great partner translates business problems into data processing algorithms focusing on what matters to your company, not what's technically impressive.
Case Studies and Portfolio Analysis
Demand to see detailed case studies demonstrating how their AI deployment strategies solved specific business problems with measurable outcomes and ROI for companies like yours. Look beyond the glossy marketing materials and ask to speak directly with their previous clients about the development process, how the agents performed in real-world conditions, and what challenges they encountered during implementation. The top AI software engineering company will proudly showcase its battle-tested solutions with transparent metrics on scalable AI solutions, autonomy levels achieved, and the business impact realized over time rather than theoretical capabilities.
Custom AI vs. Off-the-Shelf Solutions
Plug-and-play virtual assistant technology might save you money upfront, but they're like buying a suit off the rack when you need something for an Olympic swimming competition — fundamentally wrong for the job. Custom agents explicitly built for your business can navigate your unique data ecosystem and decision frameworks without trying to force-fit generic solutions into your specialized workflows. The real magic happens when developers know how to blend AI model training services with custom AI product development that actually understand your business — giving systems that can hit the ground running but aren't the exact cookie-cutter solutions your competitors are using.
Scalability and AI software Integration with Existing Systems
Don't get stuck with AI agents that collapse under real-world pressure — make sure your development partner can explain precisely how their solution handles the Monday morning rush, not the Wednesday afternoon demo. A business already has a complex web of systems that took years to build; the last thing you need is an AI agent that acts like a prima donna, refusing to talk to the legacy database or requiring the entire tech stack to bend to its will. Push past the sales pitch and get into the technical weeds about their API flexibility, authentication schemes, and load testing results — because nothing kills ROI faster than an agent that works perfectly in isolation but falls apart when connected to a business environment.
Compliance, Security, AI Ethics and Compliance Considerations
AI-powered customer support requires access to sensitive business data and customer information, so the conversational AI development partner must demonstrate robust security practices, compliance certifications relevant to your industry, and transparent data governance protocols that protect your organization's most valuable assets. Look for partners who build privacy-by-design principles into their development process, including data minimization techniques, encryption standards, and agent behaviors that maintain regulatory compliance even when operating autonomously.
Different Business Needs in AI Agent Solutions
Your business isn't like everyone else's, so cookie-cutter AI agents won't cut it when you need systems that understand your specific operational workflows, regulatory landscape, and competitive battleground in ways that off-the-shelf solutions never could.
AI Agent Development for SaaS Platforms and AI-Driven Automation
SaaS platforms need voice recognition software that thrives in the messy reality of cloud environments, juggling thousands of customers' needs simultaneously while keeping everyone's data in their own secure sandbox. Regarding AI-driven automation, you're not looking for glorified if-then statements but sophisticated agents that can wade through the complexity of business processes, make judgment calls on their own, and get smarter over time.
AI Agent Development in Fintech
Fintech companies need AI agents that can operate in real-time transaction environments where milliseconds matter and regulations constantly change, requiring systems that balance lightning-fast fraud detection with regulatory compliance across multiple jurisdictions. These specialized agents must go beyond simple anomaly detection to understand the complex patterns of legitimate financial behavior. They make nuanced risk decisions that protect the business without creating unnecessary friction for genuine customers — while maintaining detailed audit trails that satisfy regulators and your compliance team.
AI Agent Development for Marketplace Optimization
Marketplace platforms need AI agents that balance supply and real-time demand dynamics while optimizing for competing objectives like seller profits, buyer satisfaction, and platform revenue share. These specialized agents master the complexity of multi-sided network effects. They dynamically adjust matching algorithms and pricing strategies based on constantly shifting market conditions without creating winner-take-all scenarios that drive participants away.
AI-Driven Business Intelligence
Enterprise BI demands AI agents that can connect disparate data silos across the organization and transform overwhelming data volumes into actionable insights that drive decision-making at every level. These AI agents need to go beyond simple dashboards to proactively identify business opportunities, predict emerging trends, and deliver personalized intelligence to different stakeholders within their existing workflows. Enterprise AI agents maintain data lineage for governance and explain their reasoning in business terms.
Logistics, CRM, and Workforce Automation
Here, AI agents navigate the messy real-world complexities of physical supply chains, human relationships, and employee workflows while making autonomous decisions that adapt to constantly changing conditions. They seamlessly integrate with legacy systems, learn from historical operational data, and coordinate multiple moving parts — from warehouse robots to customer interactions to staff scheduling. The agents provide transparent decision trails so humans understand why that shipment was rerouted or why a particular client received priority attention.
Factors Affecting AI Development Pricing
Project Complexity: AI development costs skyrocket when business processes involve intricate decision trees and multiple stakeholders or require agents to handle rare edge cases that need extensive training data and customization.
Data Quality: Poor data hygiene will drain a budget faster than anything else, as messy, inconsistent data across legacy systems requires extensive preprocessing before any agent can make reliable decisions.
Customization Level: Off-the-shelf AI foundations with minimal customization might cost 25% of fully bespoke solutions, but the real value often lies in finding the sweet spot between leveraging existing models and building proprietary agent behaviors.
Integration Requirements: Complex environments with numerous legacy systems, third-party APIs, and varied data sources increase development costs due to the custom connectors and middleware required.
Deployment Scope: Phased implementation with targeted pilots demonstrating quick wins on specific high-value problems consistently delivers better ROI than attempting enterprise-wide rollouts that try to solve everything at once.
Avoiding Common Pitfalls When Hiring AI Consulting Services
- Choosing a partner that aligns with your business model. Don't get dazzled by cutting-edge tech demos if the development firm has zero experience in the industry. You'll pay them to learn the business basics while you wait for usable agents who understand workflows.
- The risks of underestimating post-deployment support and maintenance. AI agents aren't "set it and forget it" solutions; they require ongoing monitoring, retraining, and refinement as a business evolves and data drifts. Skimping on support contracts means watching the expensive system slowly become irrelevant.
- Vendor lock-in — how to ensure long-term flexibility. Beware of black-box solutions where you don't own the training data, model weights, or agent configurations. Negotiate clear IP ownership and insist on documented APIs that allow migration away if needed.
- Overlooking explainability and transparency requirements. Many companies realize too late they've built powerful AI agents that make great decisions but can't explain their reasoning. It creates regulatory compliance nightmares and internal resistance when stakeholders don't trust the "invisible" decision-making.
- Neglecting to assess the development team's cross-functional expertise. Pure technical brilliance isn't enough — if a development partner lacks domain specialists, UX researchers, and change management experts alongside their ML engineers, you'll end up with technically impressive agents that solve the wrong problems.
Steps to Successfully Implement AI Agents in Business
- Start with an honest assessment of the organization's AI readiness — technical infrastructure and whether data is usable, processes are well-documented, and the leadership is committed to working through the inevitable growing pains.
- Develop a phased implementation roadmap that prioritizes high-value, low-complexity use cases first, giving quick wins to build momentum while establishing the foundation components: unified data pipelines and governance frameworks that more complex agent deployments will require.
- Don't make the classic mistake of treating AI-powered customer experience as pure technology projects. Invest significantly in change management and upskilling programs that help employees collaborate effectively with AI systems. Workflow redesign that optimizes the human-agent partnership rather than simply automating existing processes.
- Measure success beyond technical metrics by establishing clear business KPIs for each agent deployment and creating feedback loops between technical teams and business users. Continuously refine the agents based on real-world performance data and evolving business needs.
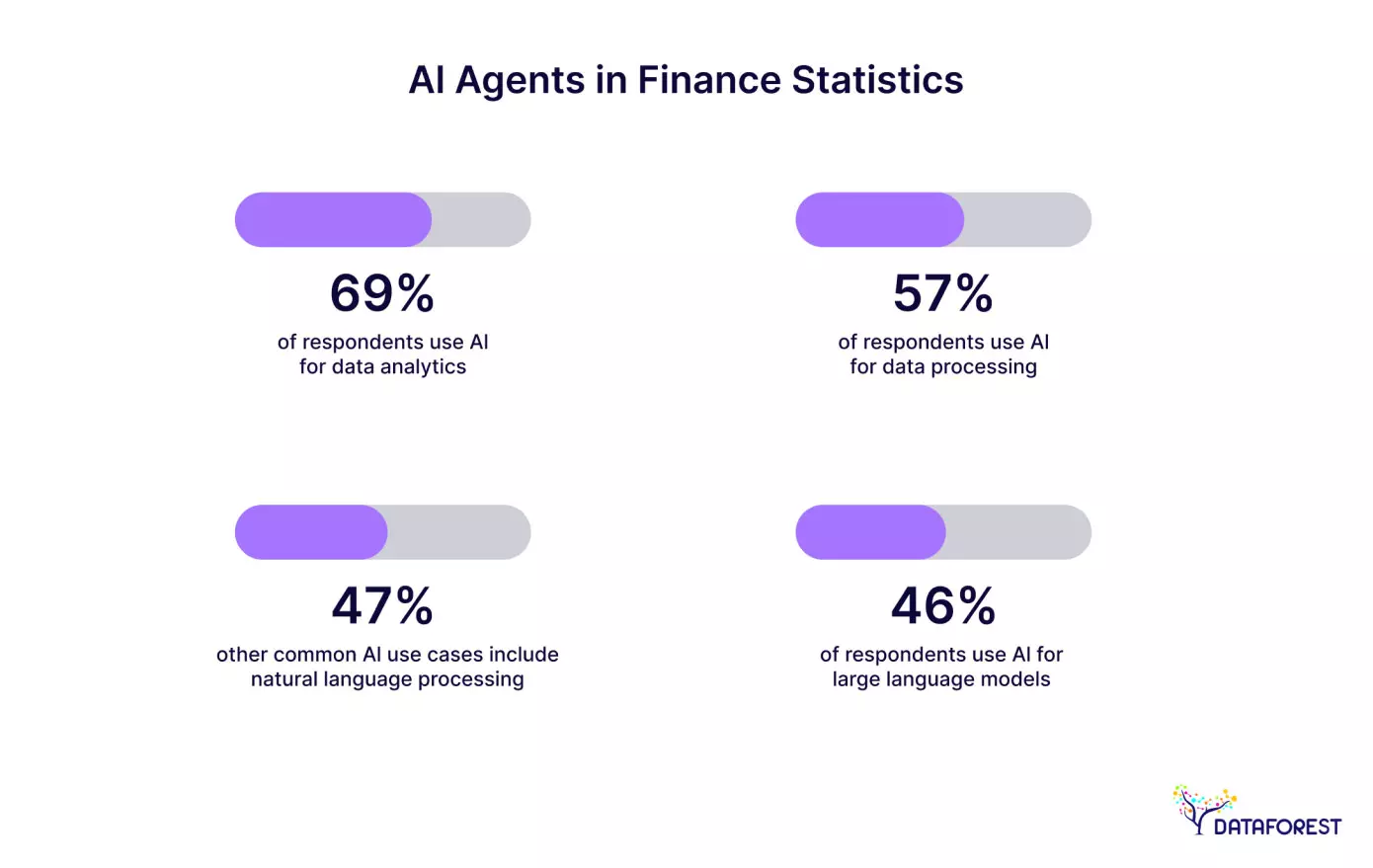
How to Vet an AI Agent Development Company
Portfolio & Case Studies: Look beyond flashy demos to actual production deployments in your industry, with measurable business metrics and proof they've solved problems similar to yours — not just toy examples that won't scale.
AI Technology Stack: Dig into their experience with reinforcement learning, multi-agent architectures, and LLM orchestration frameworks — and whether they build autonomous agents or glorified decision trees with AI branding.
Client References & Reviews: Speak directly with past clients about their experience during development sprains, how the company handled inevitable challenges, and whether the agents performed as promised in real-world conditions.
Compliance & Security Standards: Verify they have formal security protocols, compliance certifications relevant to your industry, and experience building agents that maintain regulatory requirements even when operating autonomously.
Post-Launch Support & Maintenance Services: Establish precisely how they'll help you monitor agent performance, retrain models as data drifts, and evolve capabilities as your business needs change - because an AI agent without ongoing maintenance is a slowly degrading asset.
What Vendors Need to Learn from Customers
AI solution providers like DATAFOREST must deeply understand technical requirements and actual business challenges — the problems keeping you up at night that you're hoping AI can solve rather than the features you think you want. They should insist on shadowing key users and subject matter experts to witness firsthand how decisions are currently made, where bottlenecks occur, and what unwritten rules and edge cases exist that never made it into your formal documentation. The most successful AI agent implementations start with developers who ask probing questions about metrics for success, tolerance for different types of errors, and how you'll measure ROI. Please complete the form and put us on the AI development agent providers list.
FAQ
What are the key criteria when choosing an AI agent development company?
Look for partners with proven reinforcement learning and multi-agent systems expertise who have successfully deployed agents in your industry. Equally important are their integration capabilities with your existing tech stack and their approach to ongoing support and maintenance as your agents evolve.
When choosing an AI agent developer, how critical are a company's case studies and portfolio?
Case studies and portfolio examples are absolutely essential as they demonstrate the company's real-world experience solving problems similar to yours. They reveal technical capabilities and the developer's ability to translate business requirements into effective agent architectures that deliver measurable ROI.
How do I determine if my business needs a custom AI agent or if an off-the-shelf solution will suffice?
Custom AI agents become necessary when your business processes are unique, you need tight integration with proprietary systems, or your competitive advantage depends on specialized AI-driven decision-making. Off-the-shelf solutions work well for standardized functions where your requirements align with industry norms, and deep customization doesn't provide significant added value.
What is an AI agent for software development?
An AI agent for software development is an autonomous system that assists with or performs coding tasks such as generating code, debugging, refactoring, and documentation. These specialized agents can understand requirements, suggest implementations, and accelerate development workflows while adapting to a team's specific coding standards and practices.