As digital transformation accelerates, IoT data integration has emerged as a pivotal element in modern business strategy, redefining industries with its transformative power. According to IoT Analytics, global spending on enterprise IoT technologies is projected to reach $721 billion by 2030, reflecting substantial growth in AI and data processing capabilities. This surge underscores the critical importance of IoT data integration in harnessing the potential of interconnected devices to drive efficiency, innovation, and competitive advantage.
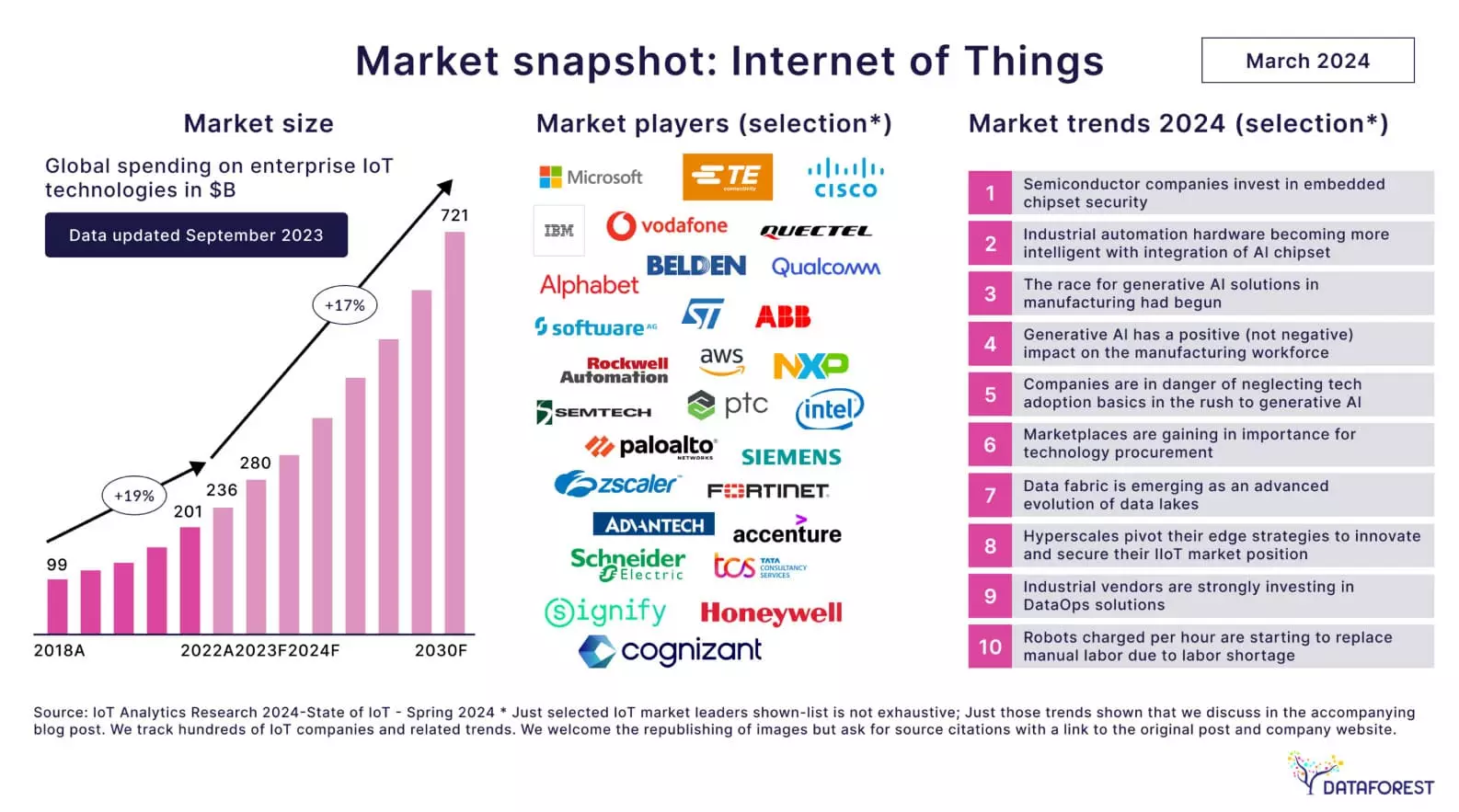
State of IoT Spring 2024: 10 emerging IoT trends driving market growth
The latest market trends for 2024 highlight significant shifts, with semiconductor companies investing heavily in embedded chipset security and industrial automation hardware becoming more intelligent by integrating AI chipsets. The race for generative AI solutions in manufacturing is accelerating, promising to revolutionize production processes. Moreover, the emergence of data fabrics as advanced lake evolutions sets new benchmarks for data management and integration.
Insights and Trends
- Semiconductor Investments: Leading semiconductor companies focus on embedded chipset security to ensure robust IoT ecosystems. This investment is crucial for safeguarding data integrity and preventing breaches in interconnected environments (Gartner).
- AI Integration in Automation: Integrating AI chipsets into industrial automation hardware is making systems more innovative and efficient, driving a new wave of innovation in manufacturing.
- Generative AI in Manufacturing: The application of generative AI in manufacturing is poised to enhance productivity and reduce costs, marking a significant shift towards more advanced and adaptive production methods.
- Data Fabric Evolution: The data fabric concept is gaining traction as an advanced evolution of data lakes, offering seamless data integration and real-time analytics capabilities.
- Hyper-scalers' Strategies: Hyper-scalers are pivoting their edge strategies to secure their IIoT market positions, focusing on innovation and security to cater to the growing demands of interconnected systems.
Defining IoT Data and Its Unique Characteristics
IoT data includes information from interconnected devices across various networks. It is predominantly real-time, requiring immediate processing to derive value, and is characterized by high volume, velocity, and variety. This data ranges from simple temperature readings to complex video feeds, necessitating distinct management strategies. Understanding these characteristics is crucial for effectively harnessing IoT's power in business contexts.
Discussing the Challenges of IoT Data
Integrating and utilizing IoT data presents significant challenges. The data's sheer volume and real-time nature demand robust infrastructure and advanced analytics capabilities. Security and privacy issues are also prominent due to the sensitive nature of the data collected and potential vulnerabilities in numerous connected devices.
Additionally, integrating this heterogeneous data into cohesive systems and providing actionable insights requires sophisticated data management and analysis techniques. A 2023 report by Palo Alto Networks highlighted that 98% of IoT device traffic is unencrypted, posing significant security risks.
IoT data integration plays a critical role in modern technological ecosystems. It encompasses the processes and strategies required to collect, process, and analyze data from various IoT devices, transforming raw information into actionable insights. By leveraging IoT data integration, businesses can enhance operational efficiency, propel innovation, and gain a competitive edge.
This guide by DATAFOREST delves into the intricacies of integrating IoT data, providing insights and practical solutions for optimizing your IoT ecosystem. Backed by authoritative sources and comprehensive research, this article will elevate your understanding and implementation of IoT data integration.
Solutions for IoT Data Integration
Building Robust IoT Architectures
Developing a robust IoT architecture is foundational for successful data integration. It involves creating a scalable and secure framework capable of handling high volumes of data.
Utilizing edge computing significantly enhances data processing efficiency by completing computations closer to the data source, reducing latency and bandwidth usage. According to a 2023 Gartner report, 75% of enterprises will leverage edge computing by 2025 to optimize data integration.
Implementing Advanced Data Analytics
Advanced data analytics tools are essential for extracting meaningful insights from IoT data. These tools enable organizations to process real-time data, facilitating proactive decision-making. The IoT Analytics report projects that the global IoT analytics market will grow at a CAGR of 20% through 2025.
Ensuring Data Security and Privacy
Ensuring IoT data security and privacy is not just important; it's paramount. Implementing robust encryption protocols, regular security audits, and adopting a zero-trust model can significantly mitigate risks. Thales's 2023 IoT Security Report emphasizes that 50% of IoT devices will incorporate built-in security measures by 2025, underlining the growing importance of data security in the IoT landscape.
Creating Interoperable Systems
Creating interoperable systems is critical for seamless IoT data integration. Open standards and APIs facilitate communication between diverse IoT devices and systems, ensuring smooth data flow. The 2022 Open Connectivity Foundation report provides a framework that enhances device interoperability, promoting a more unified IoT ecosystem.
IoT Data Sources and Types Exploration
IoT Data Origins and Variations
In the expansive IoT landscape, the variety and complexity of data sources and types shape any IoT system's capabilities. Each source contributes a unique data stream rich in potential applications, from industrial sensors and smart home devices to sophisticated IoT platforms. These diverse data types, whether telemetry, location, or sensor data, offer specific insights and require distinct handling to maximize utility. Understanding these distinctions is essential for businesses aiming to harness and integrate IoT data effectively, enhancing operational intelligence and driving innovation.
Exploring the Diverse Sources of IoT Data
IoT data is derived from extensive sources, each adding value to the ecosystem. Familiar sources include sensors embedded in industrial machinery, wearable health monitors, smart home devices, and automotive sensors. IoT platforms play a crucial role in aggregating this data, providing a unified view that aids in comprehensive analysis and application. This exploration underscores the varied origins of IoT data and its implications for businesses looking to integrate such data into their operations.
IoT data can be categorized based on its nature and utility. Key types include telemetry data, which involves the automatic collection and transmission of data from remote or inaccessible sources; location data, crucial for tracking and managing logistics; and sensor data, which monitors environmental and operational conditions. Understanding these types and their specific applications is essential for developing effective IoT data integration strategies that align with business goals.
The Strategic Imperative of IoT Data Integration
Opening Business Insights and Operational Excellence
Integrating IoT data is more than a technological necessity; it's a strategic imperative that can unlock profound business insights and operational excellence. As IoT devices proliferate across industries, they generate a deluge of data that, when integrated properly, can provide a holistic view of operations, customer interactions, and market conditions. This integrated data is vital for deriving actionable insights that drive more intelligent decision-making, enhance automation, and enable predictive analytics, transforming reactive business models into proactive market leaders.
Why Integrating IoT Data is Paramount for Actionable Insights
The integration of IoT data is foundational to unlocking its full potential within any organization. It transforms raw data into actionable insights, encouraging companies to respond swiftly to trends and operational changes. Effective integration processes consolidate disparate data streams from various IoT devices and sensors, creating a coherent, comprehensive dataset. This harmonization is crucial for developing accurate and actionable insights, allowing businesses to optimize operations, enhance customer experiences, and innovate services.
How Integrated Data Drives Decision-Making, Automation, and Predictive Analytics
Integrated IoT data is a powerful driver for advanced business operations such as decision-making, automation, and predictive analytics. Businesses can make informed judgments with tremendous confidence and speed by providing a unified view of information. Automation becomes more reliable based on comprehensive and accurately integrated data, improving efficiency and effectiveness. Furthermore, predictive analytics rely on this integrated data to forecast future trends and behaviors, allowing organizations to adjust their strategies and operations proactively.
Crafting Robust IoT Data Integration Architectures
Building Efficient and Scalable Systems
Navigating the complexities of IoT data requires a robust and well-designed architecture that can handle the scale and diversity of data effectively. The architecture for IoT data integration is a critical framework that ensures seamless flow and processing of data from myriad IoT devices to the analytical engines where decisions are made. It includes various components such as data collection, preprocessing, storage, and analytics, each tailored to meet the specific challenges posed by IoT data.
Components of a Typical IoT Data Integration Architecture
A typical IoT data integration architecture consists of several fundamental elements that work together to handle the complexities of IoT data effectively. These components include data collection nodes, data preprocessing units, data storage systems, and analytics engines. Each element is fundamental in ensuring that data from IoT devices is accurately captured, processed, and ready for analysis. This structure supports the scalability required by the vast amounts of data generated and ensures the flexibility needed to adapt to evolving technological landscapes.
Data Collection, Preprocessing, Storage, and Analytics Layers
- Data Collection: This layer gathers data from various IoT devices and sensors. It ensures the timely and reliable acquisition of data, which is critical for downstream processing and analysis.
- Data Preprocessing: Once data is collected, it undergoes preprocessing to convert it into a standardized format suitable for integration. This layer may involve normalization, cleaning, and transformation processes, essential for ensuring data quality and usability.
- Data Storage: The processed data is then stored in databases or data lakes designed to handle the scale and complexity of IoT data. Effective data storage solutions are critical for managing IoT data's volume, variety, and velocity, providing robust, scalable, and secure storage options.
- Data Analytics: The final layer involves analyzing the integrated data to extract valuable insights. Cutting-edge analytics, including machine learning and artificial intelligence, are applied to uncover patterns, predict trends, and inform strategic decisions.
Essential Tools and Technologies for IoT Data Integration
Key Tools and Technologies in IoT Data Integration
IoT data integration is powered by many tools and technologies that optimize the collection, processing, and analysis of IoT data.
Apache Kafka stands out for its capability to handle real-time data streams through a robust, scalable, and fault-tolerant publish-subscribe messaging system.
MQTT (Message Queuing Telemetry Transport) is another indispensable technology renowned for its lightweight and efficient communication protocol, which is ideal for devices with limited bandwidth and processing power.
Moreover, specialized IoT platforms such as AWS IoT Core, Microsoft Azure IoT, and Google Cloud IoT offer comprehensive solutions covering the entire spectrum of IoT data integration tasks, from data collection to advanced analytics. These platforms often incorporate enhanced security features and seamless integration capabilities.
Ensuring Data Security and Privacy in IoT Data Integration
Importance of Data Security and Privacy in IoT Data Integration
In an era when data breaches can be financially devastating and severely damage a company's reputation, assuring the security and privacy of IoT data is crucial. Since IoT devices often collect sensitive data, any vulnerability can lead to significant risks. Thus, robust security measures are essential for protecting this data, maintaining customer trust, and complying with stringent regulatory requirements.
Encryption, Access Control, and Regulatory Compliance
Several critical security practices safeguard IoT data. Encryption is extensively used to protect data both at rest and in transit, rendering it inaccessible to unauthorized users. Entrance control mechanisms ensure only authorized personnel can interact with IoT data and systems. Similarly, compliance with data protection regulations such as GDPR in Europe or CCPA in California is imperative for any organization handling personal data through IoT devices. These measures collectively form a comprehensive security strategy that manages IoT environments' unique challenges.
Integrating IoT data into business operations has transformative potential, enabling more informed decision-making, enhanced efficiency, and new service innovations. However, it necessitates a sophisticated architecture to handle the vast and varied data effectively, supported by advanced tools and technologies. Furthermore, given the sensitive nature of IoT data, prioritizing security and privacy is not just a technical requirement but a business imperative. As IoT evolves, businesses must remain agile, adopting new technologies and strategies to leverage IoT data safely and effectively, ensuring they stay competitive in the increasingly connected digital landscape.
This extensive exploration provides a roadmap for business owners to comprehend and implement IoT data integration, highlighting its importance, challenges, and critical tools and technologies.
In conclusion, mastering IoT data integration is essential for businesses aiming to enhance operational efficiency, security, and innovation. Implementing a robust IoT architecture, advanced analytics, and stringent security measures can unlock the full potential of IoT. Effective integration strategies transform raw data into actionable insights, driving intelligent decision-making and predictive analytics.
For more information on successfully implementing IoT data integration, schedule a consultation with a DATAFOREST expert or use the form to contact us.
FAQ
What is the role of IoT data integration in optimizing supply chain management and logistics operations?
IoT data integration is pivotal in optimizing supply chain management and logistics operations. By leveraging IoT data integration, businesses can:
- Achieve real-time visibility and control over supply chain activities.
- Precisely track inventory, ensuring accurate monitoring and timely replenishment.
- Facilitate efficient route planning, optimizing delivery routes, reducing fuel consumption, and enhancing delivery times.
- Enable proactive management, allowing early detection and resolution of potential issues, minimizing disruptions, and improving overall operational efficiency.
IoT data integration is essential for modern supply chain operations, driving cost reductions and performance improvements.
How can businesses maintain data quality and accuracy when integrating data from diverse IoT sources?
Maintaining data quality and accuracy in IoT data integration requires a comprehensive strategy. Businesses should focus on:
- Implementing robust data cleaning and validation processes ensures data from various IoT sources is accurate, complete, and error-free.
- Standardizing data formats and protocols to facilitate seamless data integration IoT from diverse devices and systems.
- Continuously updating device firmware to keep up with the latest security patches and performance enhancements, ensuring data reliability.
- Utilizing advanced analytics tools to audit and correct data, providing actionable insights while maintaining high data quality standards.
By concentrating on these strategies, businesses can ensure the integrity and accuracy of their IoT data integration efforts.
What are the primary considerations for scaling IoT data integration as an organization's IoT infrastructure grows?
To effectively scale IoT data integration as an organization's IoT infrastructure grows, several key considerations must be addressed:
- Ensuring infrastructure can handle the increased volume of data generated by the growing number of IoT devices.
- Investing in scalable storage solutions and high-performance data processing systems capable of managing large datasets in real time.
- Maintaining flexibility in system architecture to allow the seamless addition of new devices and IoT integration data types.
- Bolstering security measures to protect the expanding network from potential threats, including robust encryption protocols and regular security audits.
- Continuously optimizing the integration process to efficiently manage the influx of new devices and data types, ensuring the system remains agile and responsive.
By addressing these considerations, businesses can effectively scale their IoT data integration efforts, maintaining robust performance and security standards.