Today, DATAFOREST discusses the importance of data-driven AI in enhancing your products and how to implement it effectively. Also, we will cover various real-life scenarios and the benefits it brings to product companies. Interested in the update? Book a call and we'll tell you what's what.
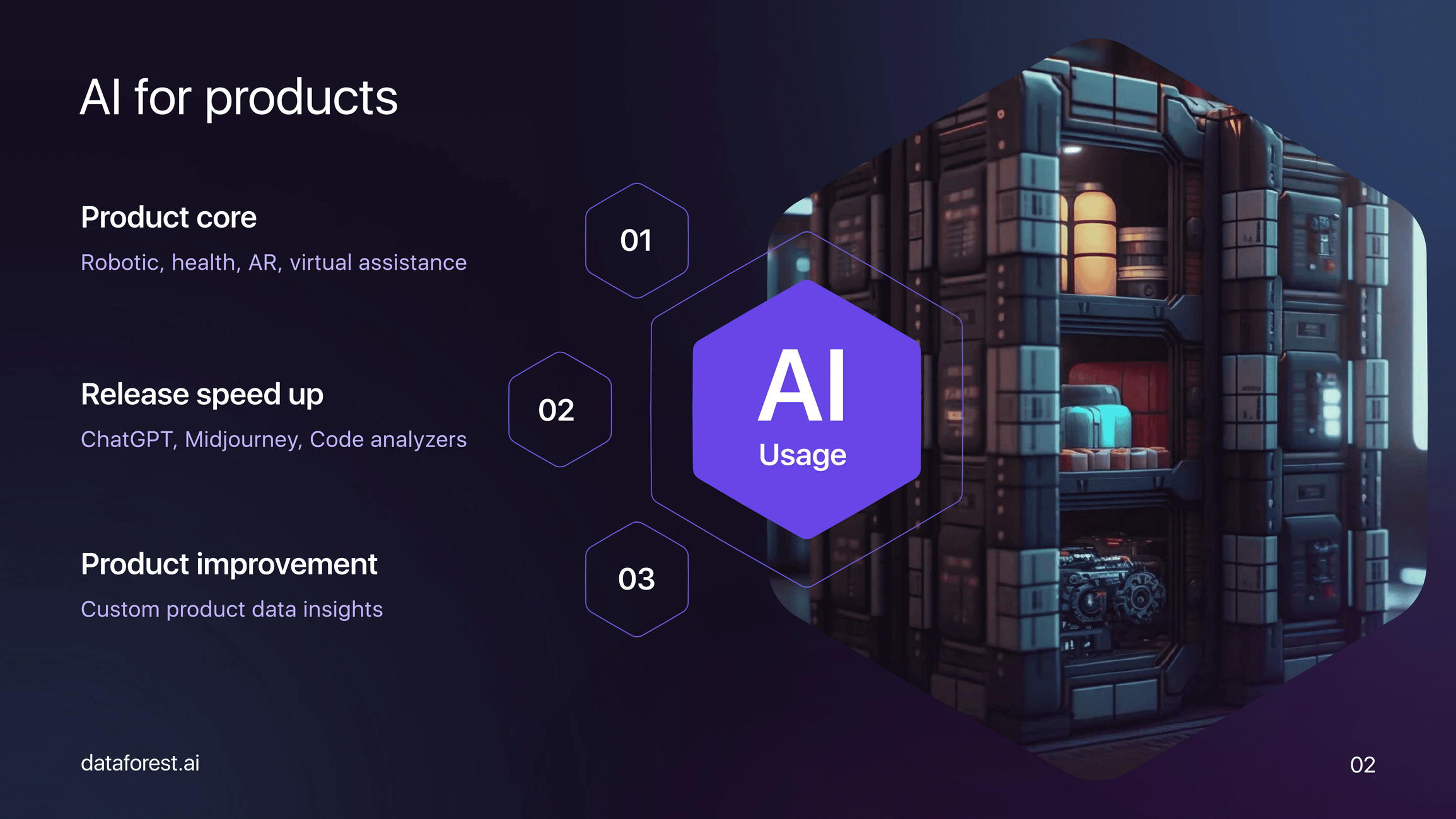
Types of AI for Products
When it comes to AI for products, there are three main categories that we can consider:
AI as the product core refers to virtual assistants like Alexa or healthcare apps that track sleep quality and provide recommendations. In this case, AI itself is the main service or feature of the product.
AI to expedite product development: AI can be used to speed up content generation and test marketing strategies. It can also be utilized for code analyzers, which help identify and eliminate bugs, leading to faster code reviews and reducing time to market.
AI for product improvement: This category focuses on utilizing real data from the product to gain insights and enhance various aspects such as customer satisfaction, security, and overall performance.

Product Improvement AI
Within the realm of product improvement AI, there are three key domains worth exploring:
- Fraud detection involves using AI tools and scenarios to detect and prevent fraudulent activities from internal and external actors. It also helps safeguard against system reverse engineering.
- Forecasting and dynamic pricing: Forecasting is particularly relevant for products dealing with physical goods, aiming to avoid stockouts and ensure customers can always purchase items. Additionally, dynamic pricing allows businesses to adjust prices based on market conditions, optimizing revenue streams.
- User experience optimization and personalization: This field focuses on better understanding customer needs to deliver tailored recommendations and improved user experiences. Businesses can provide targeted offers and interactions at the right time by analyzing user data.
Select what you need and schedule a call.

Implementation Architecture
Regardless of the specific AI tasks we undertake, the implementation architecture remains more or less the same. It typically begins with the data layer, where historical and transactional data is augmented with additional details about customers and external factors like weather or stock market trends.
Next comes the modeling phase, which also relies on data. Hundreds of different features are considered, each potentially providing valuable insights. From there, the actual AI model creation begins. The choice of machine learning model depends on the specific task at hand, whether it's generative AI, clustering, or regression.
Once the final models are selected, system projectization becomes crucial. This involves applying machine learning operations (MLOps) to ensure the models are continuously improved and are successfully deployed to production without significant issues. MLOps helps streamline the release process and ensures the models remain applicable and effective.

Real-Life Use Cases
Now, let's explore some real-life cases and scenarios where AI implementation can have a significant impact on your business:
- High-demand product fraud is particularly relevant for digital and physical goods, such as limited-edition items or tickets. Using AI, we can analyze data to identify and prevent fraudulent activities during high-demand events, ensuring customer satisfaction and avoiding negative feedback.
- Internal trade fraud: Many retail chains struggle with internal trade fraud, which can be costly to detect and prevent manually. By applying machine learning techniques, such as slicing and baseline analysis, we can identify anomalies and potentially fraudulent activities, saving business time and resources.
Suppose Nike releases a limited edition of sneakers that sells out within minutes. As usual, sales spike immediately after the release, resulting in fast shortages and customer dissatisfaction, which can ultimately harm the brand's reputation.
The complexity of tackling this problem lies in the large volume of data generated during these high-demand periods. Simple AI technologies and statistical methods are insufficient due to the sheer amount of data involved. It becomes critical to gather a significant amount of data and patiently wait until the spike subsides. In real-life situations, time is of the essence, leaving us with very limited moments to decide whether to complete a transaction or not. We cannot rely on classical matching techniques as we lack the knowledge to distinguish between legitimate customers and potential fraudsters. Consequently, it becomes important to identify patterns using an anomaly detection subset of AI.
To the right, you will find a diagram illustrating a real-life scenario that effectively eliminates approximately 80% of fraud cases. This solution consists of three layers. The first layer is relatively simple, focusing on differentiating between bots and real humans. Computers find it easier to detect patterns, as humans do not act linearly. Human behavior involves mistakes, like pressing incorrect buttons, excessively long presses, or rapid actions. In contrast, bots typically follow predictable patterns that can be identified and analyzed.
The second layer also addresses non-human solvable cases. For instance, let's examine the scenario where you want to penalize users who purchase excessive items. It is reasonable to assume that no one needs 100 pairs of sneakers monthly or yearly. However, determining what constitutes normal user behavior might be challenging. An average user purchases around 12 to 18 pairs of sneakers per year. Making an incorrect decision based solely on personal experience could lead to losing valued clients who genuinely engage with your business, or you may inadvertently allow a few bots to affect a significant portion of your customer base—especially if you have only limited stock available. AI can effectively address this challenge and find a balance.
Last but not least, an essential aspect of the success of the first two layers is detecting multi-account usage. Treating separate accounts independently will not yield the desired results. This brings us back to the concept of anomaly detection. By gathering personal information, network data, and device details and utilizing neural networks and anomaly detection techniques, we can establish connections among various events, forming a multidimensional array to identify and link related accounts.
In summary, addressing high-demand product fraud requires an approach that combines anomaly detection, pattern recognition for distinguishing between bots and humans, identification of unusual user behavior, and detection of multi-account usage. By leveraging these AI techniques, businesses can effectively mitigate fraud risks and provide a more secure and satisfactory user experience.
In both scenarios, Data Science plays a crucial role. Augmenting it with relevant information, such as shipment details or trade history, helps us detect and prevent fraudulent behavior effectively.
Implementing data-driven AI in these cases requires a multidimensional approach, combining techniques like anomaly detection, behavioral analysis, and multi-account identification.
Data-driven AI can be crucial in improving business processes, enhancing customer experiences, and mitigating fraud risks. By leveraging historical and real-time data, businesses can gain valuable insights and make informed decisions that drive growth and success.

Now, let's discuss the second case, which involves addressing internal fraud. There are numerous internal threats, and although solving this issue manually is possible, the high cost makes it prohibitive for modern retail chains. Hence, employing machine learning becomes necessary. As always, we begin by collecting and analyzing data, augmenting it with the required information in the initial stages, and then proceeding with slicing and establishing baseline metrics.
This step depends on the desired outcome. Suppose we consider sales orders or fulfillment; in that case, internal fraud activities typically involve salespeople modifying items ordered, resulting in organized crime, for example, attaching additional jackets to every pair of trousers before reselling them to personal associates. We can slice the data by items, assigning weights to each item and including information about each shipment's weight. With this, DATAFOREST can identify anomalies against human patterns and perhaps not eliminate the fraud problem but at least narrow down the areas that require further investigation.
Similarly, fraud is much more straightforward to detect as we can analyze movements across multiple accounts, monitor the transfer of coins or points to reality cards, to and from accounts, and detect discrepancies between expected and actual transactions to identify bad actors.
Handling more complex fraud cases related to luxury goods stores, which operate primarily with cash, requires a different approach. For instance, managers might register transactions only during discount periods, making it increasingly challenging to track fraudulent activities. Here, we need to adopt a novel approach characterized by a broader range of evaluation metrics that re-evaluates the entire dataset, including information previously deemed irrelevant.
Addressing internal fraud entails analyzing and augmenting data, slicing it to establish baseline metrics, and analyzing anomalies in human patterns to identify areas that require further investigation. Employing machine learning techniques helps us detect discrepancies in transaction movements across multiple accounts and enables us to evolve our evaluation metrics and identify more sophisticated fraud cases.

We have a client who owns a golf club in the United States. They have observed that some long-term members who frequently visit the club exhibit different behaviors compared to those who visit other country clubs within the same chain. These members spend the same amount of time at other clubs but consume fewer services and spend less money.
To address this issue, we implemented two aspects of AI.
Firstly, we focused on customer recognition. As it is impractical to expect customers to present their membership cards every time they visit a restaurant within the club's premises, we leveraged computer vision, Geo-in-app tracking, and device notification technologies. By identifying customers through their smartphones' connection to the club's Wi-Fi, we can track their presence without relying on physical cards.
Secondly, we developed a recommendation system. When customers approach the club's staff members, the staff can view customer profiles on their tablets. These profiles contain valuable information about the customer's preferences, habits, and history across all chains within our client's golf club network. Utilizing this data allows the staff to make personalized recommendations that align with the customer's preferences, enhancing their overall experience.
By combining customer recognition technology with the recommendation system, our client's golf club can provide tailored experiences to each customer based on their past visits and preferences. This approach ensures that customers receive relevant suggestions and recommendations, regardless of which country club within the chain they are visiting. It allows us to increase revenue by 2.5 times for customers who visit the county club for the first time.
If you're looking for more real-life examples of how data science and machine learning can benefit various industries, discover more helpful DATAFOREST cases! From enhancing business operations to improving decision-making processes, these case studies will inspire you and provide specific solutions for implementation. Don't wait any longer; head to the website and explore the endless possibilities of Big Data, ML, and AI!
Implementation of AI

To summarize, AI is a valuable tool that can be implemented in various industries, but it's essential to prioritize quality data to achieve successful outcomes. Whether you're implementing AI in physical goods, hospitality, or any other type of business, be sure to have clear objectives and a creative approach to problem-solving.
Instead of getting stuck on perfecting an AI solution, it's better to prioritize testing, updating, and reproducing it. This iterative cycle allows for continuous improvement and a more effective way to address business challenges. Remember, quality data is critical to achieving results with AI, and it's essential to have a strategic approach to implementation.
With cutting-edge technology and a proven track record, DATAFOREST offers the tools and expertise to gain valuable insights, optimize operations, and make informed business decisions. Take advantage of this opportunity to transform your business. Contact us now!