A new robo-advisor platform targets young investors. Traditional AI analyzes user data (income, risk tolerance, goals) to build a core portfolio. Generative AI then creates personalized educational content (articles, videos) explaining the rationale behind each investment, using language tailored to the user's financial literacy and interests (e.g., sports analogies for risk management). This combination personalizes investment education, builds trust, and fosters long-term client relationships, all while keeping fees low through automation. Traditional AI monitors portfolio performance and suggests adjustments, while Generative AI keeps users informed with clear, dynamic updates, increasing their engagement and sense of control. So, let's look at the choices of Traditional AI vs Generative AI.
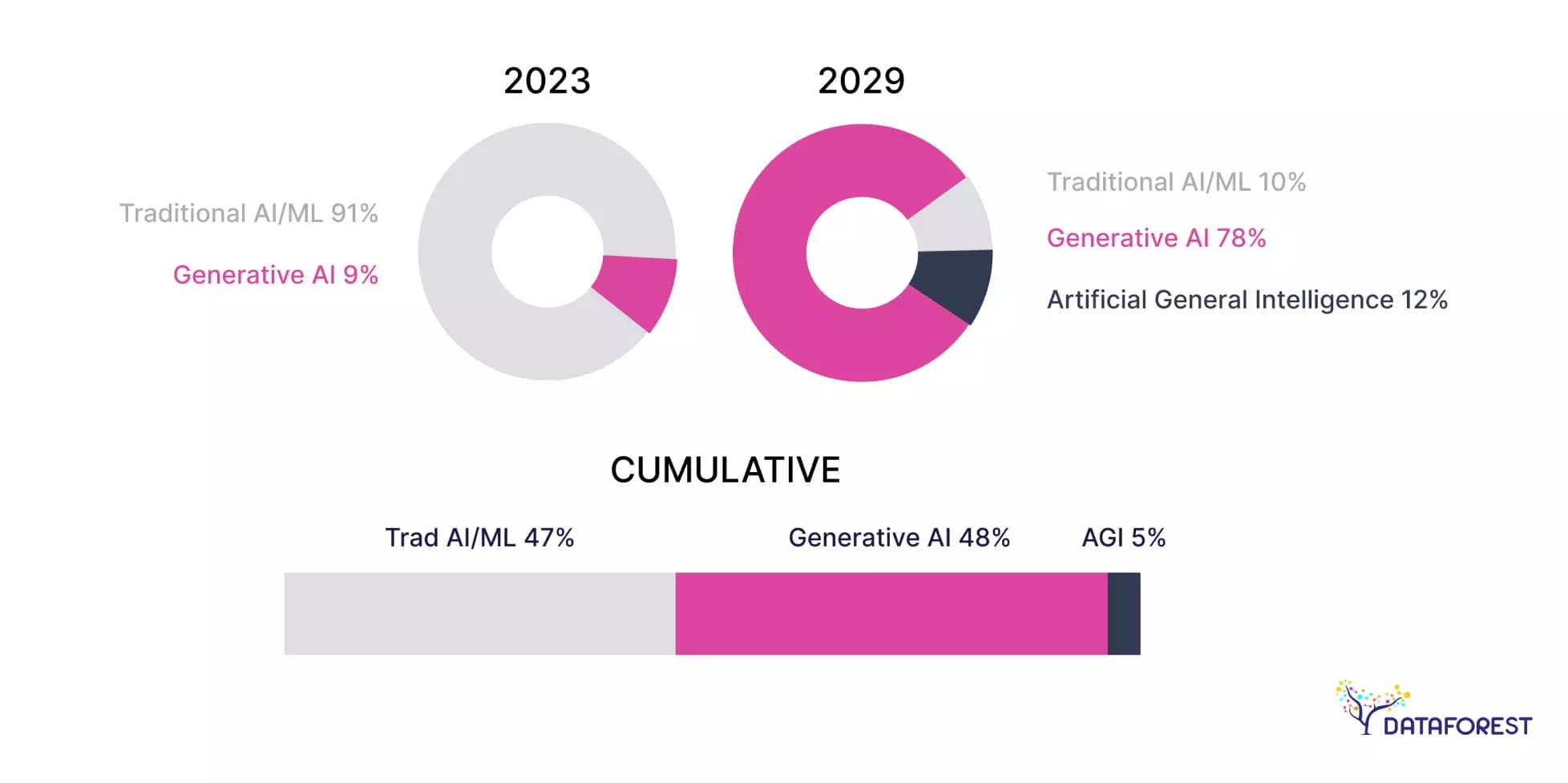
Traditional AI—The Rule Follower
Traditional AI, sometimes called Narrow AI or Weak AI, is artificial intelligence that focuses on performing specific tasks using well-defined rules and algorithms. A chess-playing computer or a spam filter uses traditional AI techniques. Humans program traditional AI systems with explicit rules and instructions on approaching a problem. This makes them transparent and reliable, as their decision-making process is straightforward. While more data-hungry than Generative AI, traditional AI can still be trained on large datasets. This allows them to identify patterns and improve their performance. Traditional AI struggles with tasks requiring creativity or handling situations outside their programmed parameters. They need help to adapt or learn entirely new things. Traditional AI vs Generative AI is a powerful tool for automating tasks, analyzing data, and making predictions within its defined scope.
The Rule-Based Engine Behind Automation
Compared to Traditional AI vs Generative AI, the first works like a highly skilled specialist following a well-defined playbook.
- Humans define the specific task and create a set of algorithms for the AI to follow. These rules can be like recipes, with clear instructions on how to process information and arrive at a decision.
- Traditional AI systems are trained on large datasets relevant to the task. This data could be anything from financial records for fraud detection to historical chess games for strategic planning.
- The AI analyzes the data with analytics and identifies patterns and relationships between different elements. This allows it to learn how to perform the specific task effectively.
- When presented with new data, the AI applies the learned patterns and programmed rules to make a prediction or take action. For example, a spam filter might analyze an email's content and sender information to decide if it's spam based on patterns learned from past emails.
- In some cases, advanced traditional AI can be further refined as it encounters new data. Programmers might adjust the rules or provide additional training data to improve the AI's accuracy.
Picture a tax preparation AI in the context of Traditional AI vs. Generative AI. Programmers would define the tax code and its calculations as rules. The AI would then be trained on past tax returns (data) to identify patterns and deductions. When presented with a new tax return, it would use the rules and learned patterns to calculate taxes efficiently.
Generative AI: Turning Data into New Realities
Unlike its rule-following counterpart in the Traditional AI vs. Generative AI pair, the latter focuses on creating entirely new data, mimicking the patterns it's been trained on. It devours massive datasets of particular types—text, images, music, code, you name it. This data becomes the raw material for its creative endeavors. Generative AI learns by observing the intricate relationships and patterns within the data. It essentially studies the "language" of the data.
It can be thought of as having two parts: a generator and a discriminator. Like an artist, the generator constantly creates new data, while the discriminator, the harsh critic, analyzes the generated data and compares it to the original dataset. Through a continuous loop, the generator tries to create data that fools the discriminator into thinking it's real. If the discriminator catches the fake, the generator returns to the drawing board. Over time, the generator becomes incredibly skilled at producing new data that closely resembles the training data.
The power of Generative AI lies in:
- Creativity: It produces new and original content, pushing the boundaries of what's possible.
- Adaptability: It can learn new styles and patterns by being trained on different datasets.
- Exploration: It allows for exploring possibilities beyond the existing data.
In the conception of traditional AI vs. Generative AI, Gen AI also faces challenges, such as the potential for bias if trained on biased data and the need for significant computing power for training.
Traditional AI vs Gen AI: Key Differences
Traditional AI is the master of analyzing and applying existing knowledge. Generative AI is the pioneer of creating entirely new possibilities. Here's a breakdown of the key differences between Traditional AI vs Generative AI, focusing on problem-solving approach, data needs, and creative potential:
Traditional AI vs. Generative AI: Where Do They Shine?
This matrix explores the strengths of traditional AI and generative AI in various application areas. Understanding these differences can help identify the most suitable AI approach for a specific task.
A Performance Showdown—Traditional AI vs Generative AI
This matrix compares the performance of generative AI and traditional AI across various aspects. Understanding these strengths and limitations helps decide which AI approach best suits your needs.
Choosing the Right Type of AI
Speaking from DATAFOREST's experience, it involves clearly understanding your needs and the capabilities of Traditional AI vs. Generative AI.
- What problem are you trying to solve, or what process do you want to improve? Be specific. Is it automating customer service interactions, personalizing marketing campaigns, or generating new product ideas?
- Consider the Traditional AI vs Generative AI factors crucial for your project. Do you need highly accurate and explainable results (traditional AI strength), or are creative exploration and adaptability more important (generative AI strength)?
- What type of data do you have available? Traditional AI works best with structured, labeled data, while generative AI thrives on vast amounts of unlabeled data relevant to the task.
- Do you need to understand Traditional AI vs. Generative AI decisions or outputs? Traditional AI offers clear explanations due to its rule-based nature, while generative AI's creative process needs to be more transparent.
- Training complex AI models can be computationally expensive. Assess your budget and available computing power for training and running the AI system.
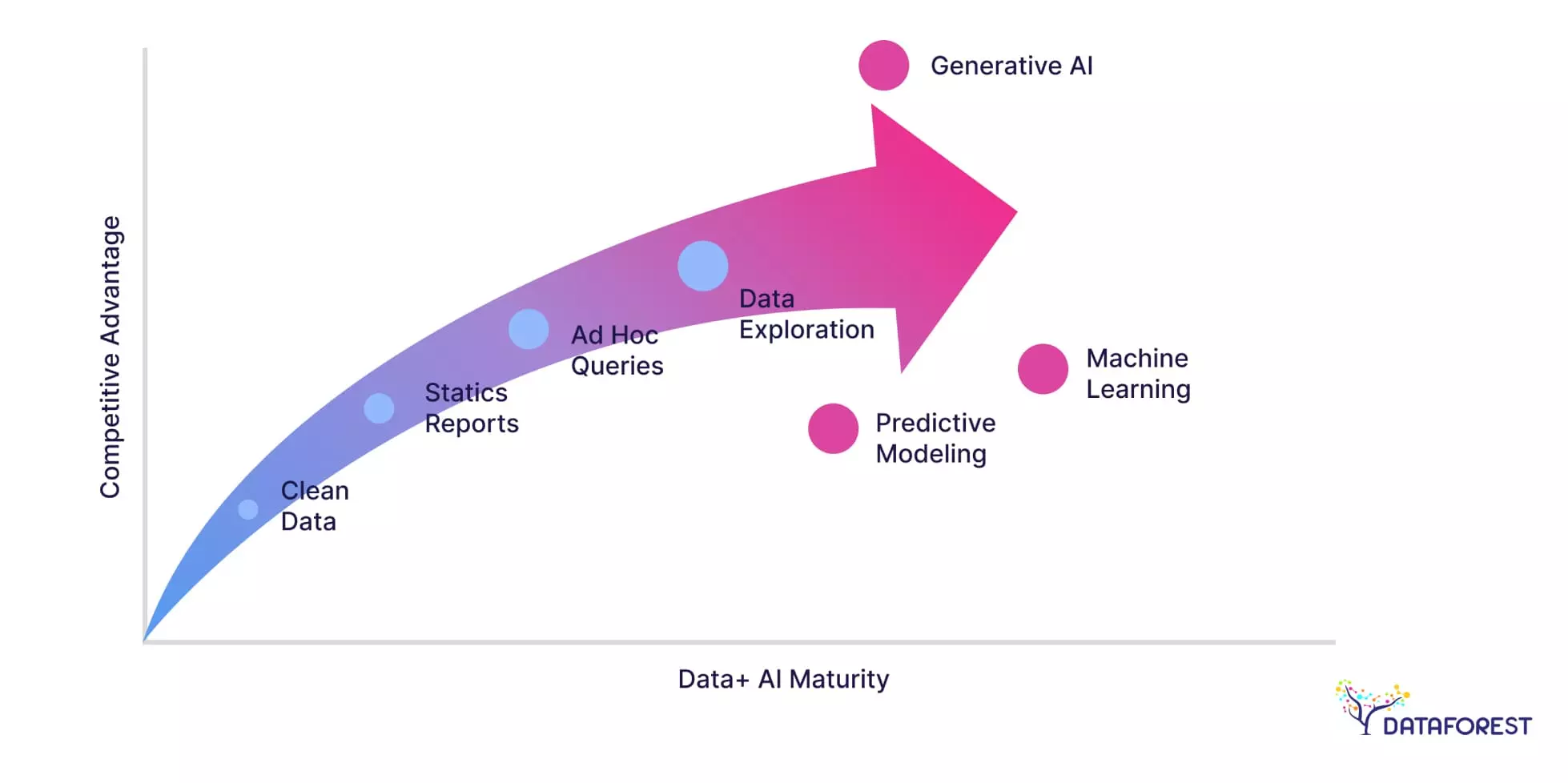
Choose Traditional AI if:
- It would help to have high accuracy and explainability for a well-defined task.
- You have access to clean, labeled data relevant to the task.
- Transparency in decision-making is crucial.
Choose Generative AI if:
- Creativity and exploration of new possibilities are essential.
- You have access to large amounts of unlabeled data.
- Explainability is less critical than achieving a creative outcome.
Please complete the form and book a consultation about Traditional AI vs Generative AI.
FAQ
What is the main difference between traditional AI vs generative AI?
Traditional AI analyzes data and performs specific tasks based on programmed rules. Generative AI, on the other hand, focuses on creating entirely new data, mimicking the patterns it's been trained on, often resulting in creative outputs.
How do I choose Traditional AI vs Generative AI for my business?
To choose the right AI for your business, first identify your needs. Do you require high accuracy and explainability (traditional AI) or creative exploration (generative AI)? Then, assess your data availability—traditional AI prefers structured data, while generative AI thrives on vast amounts of unlabeled data.
Can you provide examples of traditional AI vs generative AI applications in business?
Traditional AI powers spam filters and fraud detection systems, analyzing email content or financial transactions to identify patterns. Generative AI can create personalized marketing content or design new product prototypes, using its understanding of existing data to generate entirely new creative formats.
How can businesses stay informed about developments and advancements in traditional AI vs generative AI technologies?
Businesses can leverage industry publications and attend conferences focused on AI to stay updated on traditional AI vs generative AI advancements. Research institutions and tech companies working on AI can provide valuable insights into the latest developments.
What are some real-world examples of businesses successfully leveraging generative AI for competitive advantage?
Nike utilizes generative AI to design new shoe styles, analyzing vast amounts of data on past designs, trends, and customer preferences to generate unique footwear. Meanwhile, Netflix leverages generative AI to create personalized video previews for its users, analyzing viewing history and preferences to produce trailers more likely to capture their attention and lead to engagement. These brands have chosen between Traditional AI vs. Generative AI.