At the SaaS company, frontline staff embrace AI chatbots and automation tools. The team showed nice results and asked for more new capabilities. While executives hesitate over regulatory concerns and financial constraints, the opportunity for digital transformation slips away. This creates a dangerous gap where competitors can leap ahead, talented employees get frustrated seeing their initiative ignored, and your tech-savvy people start updating their LinkedIn profiles to find companies that walk the innovation talk. McKinney's survey shows that 94% of employees and 99% of C-suite leaders are familiar with generative AI in business tools. However, executives underestimate usage — they believe only 4% of employees use gen AI for 30 %+ of daily work when actual employee-reported usage is three times higher. If you think this is your case, then arrange a call.
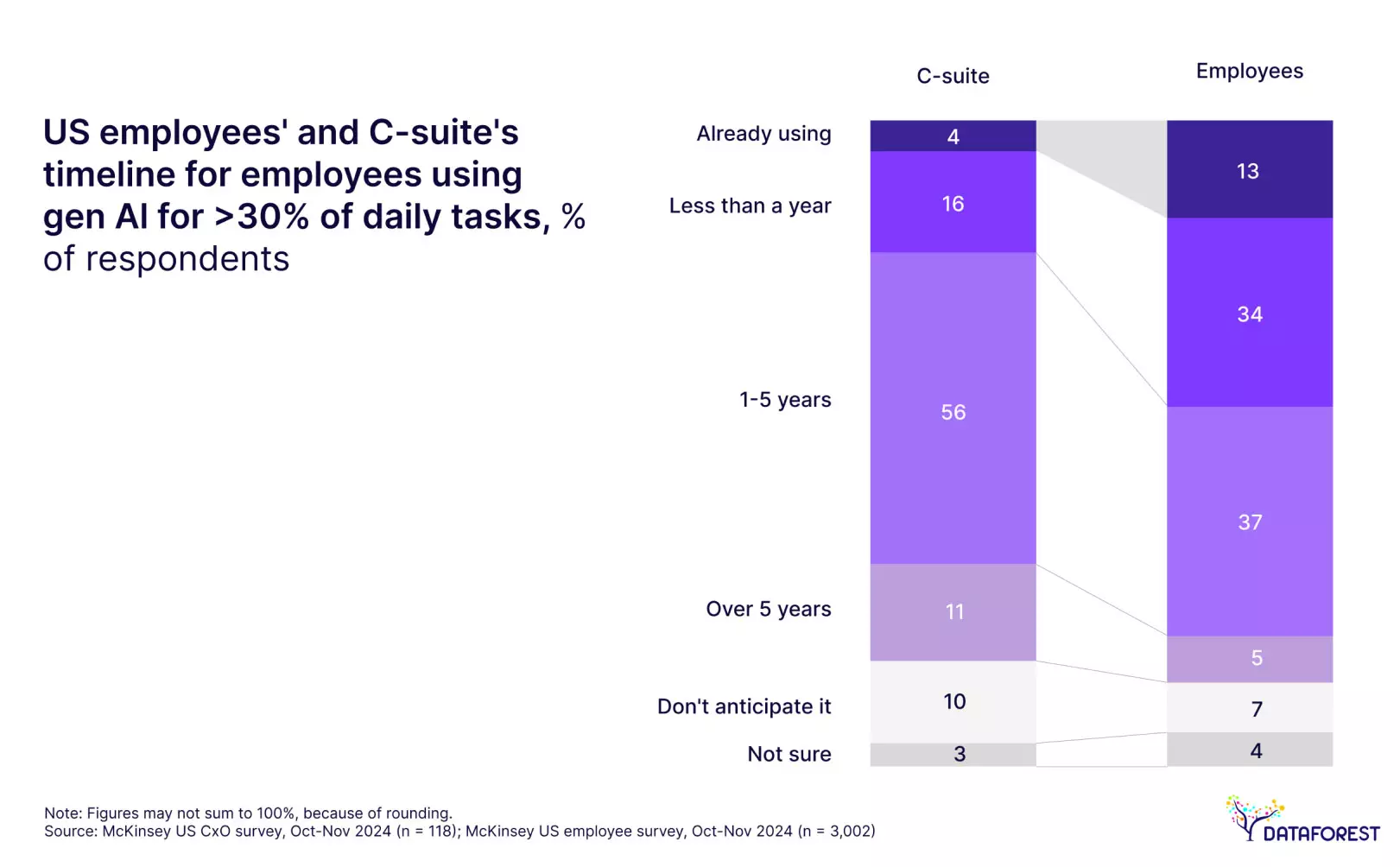
The AI Adoption Paradox — When Employees Lead, but Leaders Lag
Employees often embrace AI applications in business tools to solve immediate daily productivity challenges, while executives worry about governance, security, and ROI without seeing the ground-level benefits. Leaders may fear industry disruption to established business models or lack technical understanding to evaluate AI in business strategic value, creating hesitation where employees see apparent utility. Many CxOs are concerned about compliance risks and data privacy implications that frontline staff might overlook in their enthusiasm for operational efficiency. Executive reluctance stems from organizational inertia and the pressure to maintain legacy systems representing past investments. The speed gap exists because employees experiment with consumer-grade AI in business examples immediately, while leadership must consider enterprise-wide implications requiring more deliberate adoption timelines — a classic change management challenge.
AI Productivity Tools for Industry-Specific Challenges
Different industries face unique workflows, data types, and compliance requirements, so specialized AI applications in business tools are developed to tackle these specific pain points — healthcare needs HIPAA-compliant NLP models. At the same time, manufacturing relies more on computer vision and robotics for quality control. DATAFOREST will do the same; you need to arrange a call.
Tech Companies & SaaS
Document processing AI: Automates extraction and organization of data from contracts, support tickets, and documentation
Code completion tools: Speed developer workflows with context-aware suggestions
Automated testing frameworks: Generate test cases from requirements for better scalability
Retail & Marketplace Operations
Inventory prediction AI: Forecasts stock needs to be based on historical data and market trends
Customer service bots: Handle tier-1 support issues, freeing staff for complex problems
Loyalty system optimization: Personalized marketing engines that increase engagement
Data Engineering
Automated ETL pipeline builders: Create and manage data flows with minimal coding
Anomaly detection systems: Flag unusual patterns in data streams before they cause issues
SQL generation assistants: Convert natural language queries to database commands and fuel AI integration
Startups
AI-powered market research: Analyze competitor products and identify market gaps
Content generation tools: Create product descriptions and marketing copy at scale
Rapid prototyping assistants: Convert sketches to functional mockups with an agile mindset
Enterprise Solutions
Meeting summarization tools: Capture key points and action items automatically
Process mining AI: Identify bottlenecks in existing workflows
Internal knowledge bases: Self-updating documentation with natural language search for future-proofing
Fintech
Fraud detection systems: Real-time transaction monitoring with anomaly flagging
Chargeback analysis tools: Identify patterns in disputed transactions
Document verification AI: Streamline KYC/AML processes through automated checks and improve business growth
Utility Companies
Predictive maintenance platforms: Schedule equipment servicing before failures occur
Field service optimization: Route technicians efficiently based on location and expertise
Customer usage pattern analysis: Identify opportunities for load balancing and conservation for sustainable growth
Traditional AI vs Generative AI in Industry-Specific Tools
Traditional AI in business is excellent at crunching numbers, spotting patterns, and handling repetitive tasks like flagging suspicious transactions or predicting when equipment needs maintenance. Generative AI technology in business is more like having a creative partner who can write that technical documentation you've been putting off, whip up code from your rough idea, or design a whole marketing campaign based on your brand voice. Instead of those robotic "press 1 for support" systems, we're talking about AI that has actual conversations and comes up with solutions on the fly while still keeping everything HIPAA-compliant or SEC-regulated when needed. In the data-driven digital economy, generative AI in business doesn't just tell you “There's an anomaly in the system” anymore — it explains what's wrong in plain English. It drafts a detailed fix-it plan, complete with documentation. While traditional AI is an efficient assistant who organizes your stuff, generative AI is like a whole team of creative experts — a valuable asset for entrepreneurship and innovation.
Three Strategic Steps to Fast-Track Your Company's AI Journey
McKinsey also says leaders predict conservative AI applications in business rates, expecting only 20% of employees to use generative AI for more than 30% of their daily tasks within a year. However, employees are far more optimistic — 47% believe they'll integrate AI into their regular workflow at this level. This striking contrast reveals a significant disconnect between leadership's cautious outlook and workers' readiness to embrace machine learning tools in their day-to-day operations to embrace AI technology in business.
Employees Want AI Training, but Many Aren’t Getting It
Employees know AI will change how they work in big ways, and they’re ready for it — but they need their companies to step up. Nearly half of them say formal training would be the best way to help them get comfortable with examples of AI in business and make adoption smoother. They also want hands-on experience, like access to beta versions or pilot programs of AI tools. On top of that, incentives like financial rewards or recognition could go a long way in encouraging people to embrace these new technologies.
The problem? Many employees feel left behind. More than 20% say they’ve received little to no support in skill development. And outside the U.S., workers are asking for even more training opportunities to help them keep up with the pace of change. Companies have some catching up to do to stay ahead in workforce management.
Millennials Are Leading the AI Charge
Millennials—now in their mid-30s to mid-40s—are stepping into leadership roles as managers and team leaders, bringing a ton of AI implementation to business enthusiasm. The survey shows they’re the generation most excited about AI and most confident in their skills. 62% of employees aged 35-44 say they’re highly proficient with AI tools, compared to 50% of younger Gen Z workers (18-24) and just 22% of baby boomers over 65.
Two-thirds of millennial managers say they’re fielding AI-related questions from their teams at least once a week. And just as many are actively recommending AI tools to help solve everyday problem-solving tasks. Leaders act as AI coaches to bridge gaps between generations in the workforce.
By tapping into millennials’ tech-savvy energy, companies turn them into champions of business transformation in generative AI — and unlock the power of strategic thinking in action. Imagine managers who aren’t just overseeing projects but also teaching their teams how to use AI tools effectively. It’s a way to build trust, boost productivity, and push organizations toward AI maturity.
But millennials can’t do it alone. Companies need to give them the support—and the tools—to keep leading the charge. Whether it’s training programs, access to beta AI tools, or recognition for their efforts, empowering millennial managers could be the key to unlocking smoother, faster AI implementation in business across the board.

Employees Lead the Charge, Leaders Must Act
AI adoption feels different. Workers are already comfortable with AI in business examples and eager to see it transform jobs. It’s a clear signal to leaders: act now.
Start By Listening
Employees are experimenting, problem-solving, and imagining how using AI in business could reshape their workflows. Leaders who take the time to hear their ideas — whether a customer service rep using AI to draft emails faster or a marketing team brainstorming AI-driven campaigns — will uncover practical insights to inform their strategies.
Fill The Gaps
While employees are enthusiastic, many still need training to master AI technology in business. It’s like giving someone a new smartphone: they’ll figure out the basics, but a quick workshop on advanced features (like data analysis or automation) could unlock even more value. Training, workshops, and access to AI tools can empower teams to succeed in a cloud-computing world of AI-powered systems.
Empower Managers to Scale What Works
When a team successfully tests an AI pilot — say, using AI to streamline project management — leaders should give their managers the green light to roll it out company-wide. These managers are already bridging gaps, and with resources, they become catalysts for adaptability.
Move Fast but Thoughtfully
The AI landscape is moving at warp speed. Companies that drag their feet risk falling behind competitors already integrating AI into daily operations. But rushing without a plan isn’t the answer either. Leaders must balance urgency with clarity, ensuring teams understand why AI matters and how it aligns with their goals.
AI Investments Aren’t Paying Off Like They Should — Here’s Why
Many companies have poured money into AI technology in business, but the returns don’t meet expectations. They’re not unlocking AI’s full economic potential. In fact, about half of C-suite leaders at AI-enabled companies say their initiatives are still in progress — either developing or expanding — when they should be much further along by now.
Tech & Digital Platforms: AI is the Product
AI in business examples are baked into the product for SaaS platforms, marketplaces, and fintech companies. Whether intelligent search algorithms, automated fraud detection, or AI-driven financial insights, these businesses use AI to scale fast, personalize experiences, and stay ahead of the competition.
Retail & E-Commerce: AI for Smarter Selling
Retailers and online marketplaces use AI implementation in business to make shopping feel personal, from recommendation engines that predict customers' wants to dynamic pricing models that adjust based on demand. AI also plays a significant role in fraud prevention, helping businesses spot chargeback risks and flag suspicious transactions before they happen.
Manufacturing & Utilities: AI for Keeping Things Running
Factories, plants, and services rely on AI for predictive maintenance. They use data to figure out when equipment will fail. This means less downtime, fewer costly repairs, and smoother operations. AI helps manage supply chains, optimize energy use, and automate routine tasks.
Finance: AI for Smarter, Faster Decisions
Banks, payment platforms, and insurance companies use examples of AI in business to analyze risk, detect fraud, and automate compliance. Whether catching a suspicious transaction in real-time or using machine learning to assess creditworthiness, AI helps financial services make faster, data-driven decisions with less human error.
Startups: AI as the Competitive Edge
Startups use AI technology in business to build entirely new products and services. Think beauty match apps, job search platforms, and AI-powered real estate marketplaces. These businesses use AI to create something that didn't exist before. Since startups need to move fast, many invest in AI microservices, plugging in AI-powered features without building everything from scratch.
Enterprises and Physical Businesses
For companies with 100–200 employees — especially those with logistics, sales teams, or field operations — AI in business examples is about automation and optimization. AI-powered mini-CRMs, fleet management, and sales automation help streamline processes, eliminate repetitive tasks, and allow employees to focus on higher-value work.
AI in Customer Loyalty & Engagement
Retailers and businesses with loyalty programs use AI implementation in business to better understand customers and personalize experiences. AI helps predict who's likely to churn, what offers will drive engagement, and how to fine-tune marketing strategies to boost customer retention.
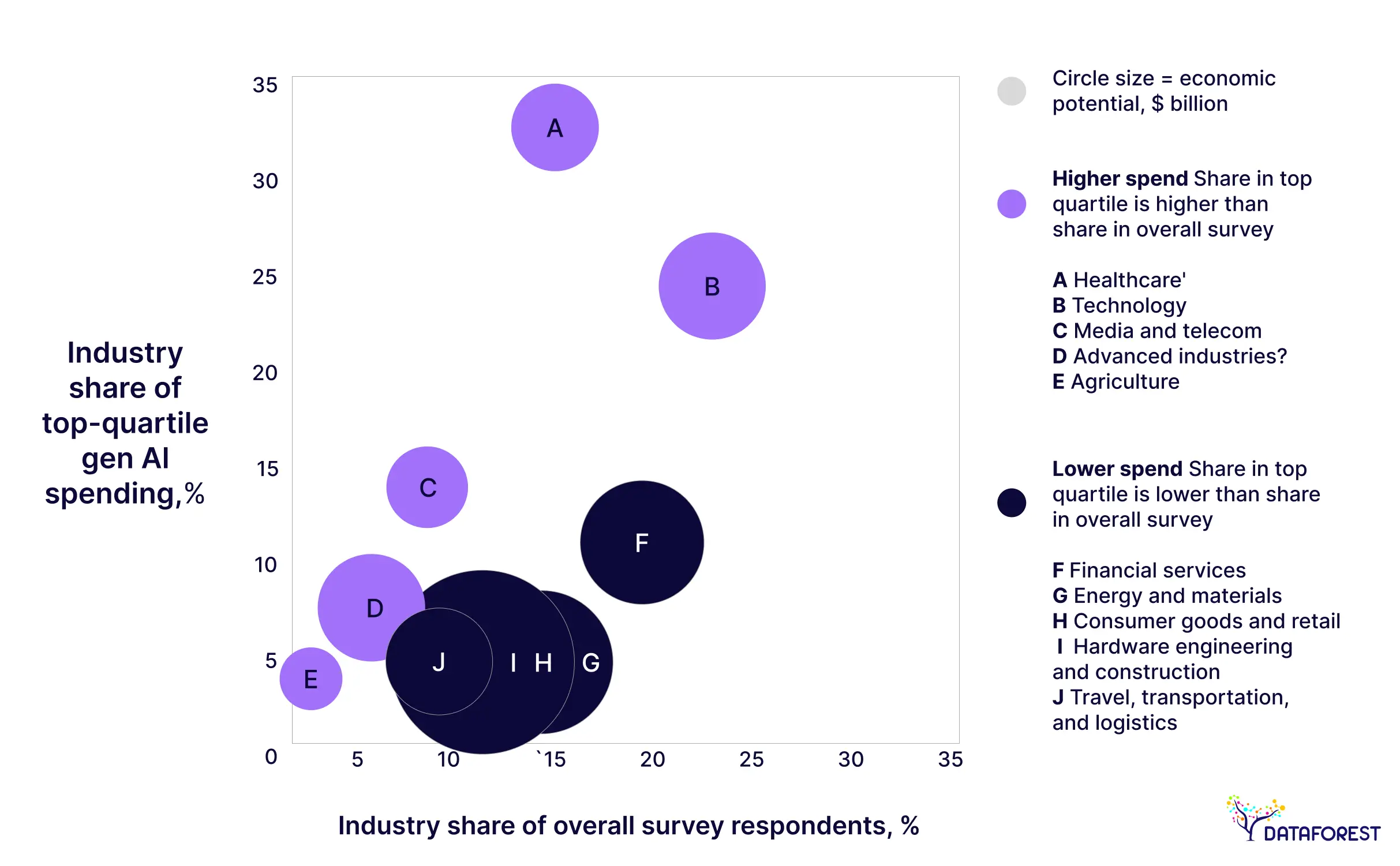
Why Gen AI Hasn’t Delivered Enterprise-Wide ROI — Yet
Generative AI promises faster workflows, more intelligent automation, and game-changing insights. But for many enterprises, the reality hasn't lived up to the hype. AI pilots look promising, yet enterprise-wide ROI remains elusive. The good news? That can change.
AI Pilots Work—Scaling is the Challenge
Most companies start with small AI experiments, automating customer support responses or generating reports. The projects show quick wins, but when it comes to rolling AI out at scale, businesses hit roadblocks:
- Data fragmentation — AI needs clean, connected data, but many enterprises deal with siloed databases and outdated pipelines.
- Integration issues — Legacy systems don’t always play nice with AI models, leading to expensive workarounds.
- Lack of AI expertise — IT teams can manage traditional software, but fine-tuning AI models and maintaining performance is a different game.
The Fix: Instead of standalone pilots, companies need AI in business strategies, starting with stronger data foundations and modular AI solutions that integrate into existing workflows.
The ROI Takes Time to Show Up
Unlike traditional automation, Gen AI’s impact is more nuanced. It enhances creativity, decision-making, and customer experience — but those benefits don’t always translate to immediate cost savings. Many businesses struggle to measure AI’s real value because:
- Productivity boosts don't show up in revenue immediately (e.g., AI speeds up content creation, but does that lead to more sales?).
- AI needs continuous fine-tuning—what works today might need adjusting tomorrow.
- Regulatory concerns slow adoption, especially in finance, healthcare, and other compliance-heavy industries.
The Fix: Companies need clear AI success metrics beyond cost savings — time saved, improved decision accuracy, and increased customer retention.
Employees Need AI Upskilling
Employees are eager to use AI but don’t know how to get the most out of it. Giving workers AI tools without proper training is handing someone a high-end camera without explaining the settings. They’ll consider the basics but won’t unlock their full potential.
The Fix: Businesses should invest in AI training programs, teaching employees how to customize prompts, interpret AI-generated insights, and spot errors before rolling AI out company-wide.
AI Governance is Slowing the Adoption
Companies are worried about the risks. Hallucinations, bias, and cybersecurity concerns make businesses hesitant to go all in. Many AI models lack transparency, making it challenging for enterprises to trust them for critical decisions.
The Fix: A structured AI governance framework can help companies monitor AI outputs, prevent bias, and ensure security, giving leaders the confidence to scale AI across departments.
So, AI can deliver enterprise-wide ROI — with the right approach.
Don’t Just Use AI — Unleash It
AI in business and its providers, like DATAFOREST, take visionary leadership and are willing to push beyond minor improvements and aim for transformational change.
The pieces are already in place. AI is powerful and evolving fast. Employees are more ready than leaders expect. Leaders have more freedom than they realize to accelerate AI adoption across their organizations. The real challenge is thinking bigger.
Companies that genuinely want to lead in AI must shift their focus from small-scale optimizations to bold, system-wide transformations. That's where real differentiation — and long-term competitive advantage — comes from. Please complete the form and lead AI adoption confidently.
FAQ
What key skills do business leaders need in the age of artificial intelligence?
Business leaders must balance technological understanding with strategic vision, fostering innovation and managing risks and change.
What mistakes do entrepreneurs make when trying to implement AI in business?
Entrepreneurs often underestimate the importance of data quality, overlook the need for employee training, and rush AI adoption without clear objectives.
Does AI affect a leader's emotional intelligence?
AI can enhance a leader's emotional intelligence by providing data-driven insights for better decision-making, but it also requires leaders to be more adaptable and empathetic to change.
How does AI affect leadership skills and management style?
AI affects leadership by shifting management styles towards more data-informed decision-making and promoting agility while requiring leaders to guide teams through technological transitions.
How do we prevent employee resistance when implementing AI?
To prevent employee resistance, it's essential to involve them early in the AI adoption process, provide proper training, and communicate the benefits of AI for both the business and their roles.