As a well-maintained highway system connects people, Сloud Big Data infrastructure is a high-speed network that delivers data, enabling businesses to transport and access insights. Urban engineers agree that modern cities have significantly grown in breadth, so the further development path leads upward — with multi-level overpasses and light air transport. That is, closer to the clouds. The digital world has been following this path for a long time, and it offers the benefits of Big Data in the cloud and the opportunity to rise above competitors. We know how to handle Big Data; arrange a call, and you will know too.

Popularity of Big data and Cloud computing compared to classical data mining
Big Data Express — Cloud Infrastructure's Route
Building custom roads (traditional on-premises approach) for different cargo types is costly and time-consuming. It's constructing unique roads and ensuring security. Now, consider the cloud infrastructure for Big Data as scalable, efficient, and pre-built. You can transport data without the headache of maintaining your infrastructure. It's the fast lane to cost-effective Big Data management, saving you average computing time and money.
Big Data on the Cloud: Seven Key Benefits
The cloud transforms how businesses handle Big Data, offering benefits that drive innovation and efficiency. Organizations gain a competitive edge, unlock opportunities in a data-driven landscape, and use data big opportunities.
1. Scalability and Flexibility:
Cloud-based Big Data solutions provide unparalleled scalability, allowing businesses to adjust resources quickly based on their evolving needs. This flexibility ensures optimal performance and cost-efficiency as data volumes fluctuate. For example, a rapidly growing e-commerce company can seamlessly scale its cloud infrastructure to accommodate increased website traffic and transaction data during peak seasons.
2. Cost Efficiency:
Cloud-based Big Data significantly reduces capital expenditures by eliminating the need for expensive on-premise infrastructure. Pay-as-you-go models enable businesses to pay only for the resources they use. A healthcare organization can leverage cloud-based Big Data analytics to identify cost-saving opportunities in patient care without investing in costly hardware.
3. Enhanced Data Processing Speed:
Cloud platforms offer powerful computing capabilities, enabling rapid processing of Big Data. This accelerated speed allows businesses to gain real-time insights and respond swiftly to market dynamics. A financial institution can use cloud-based Big Data services to detect real-time fraudulent transactions, minimizing financial losses.
4. Improved Accessibility and Collaboration:
Cloud-based Big Data solutions provide seamless access to data from anywhere, anytime, and on any device. This accessibility fosters collaboration among teams, regardless of geographical location. A global research team can collaborate on a cloud-based Big Data project, sharing and analyzing data in real time, regardless of physical location.
5. Advanced Analytics and Machine Learning Integration:
Cloud platforms often have advanced analytics and machine learning tools, enabling businesses to extract deeper insights from big data cloud solutions. These tools can identify patterns and predict trends. A marketing team can leverage cloud-based machine learning algorithms to personalize customer experiences.
6. Disaster Recovery and Data Redundancy:
Cloud providers offer robust disaster recovery mechanisms and data redundancy, ensuring business continuity during unforeseen events. This safeguards valuable data and minimizes downtime, protecting businesses from potential losses. A government agency can store critical data on the cloud, ensuring its availability and integrity in natural disasters or cyberattacks.
7. Enhanced Security:
Reputable cloud providers invest heavily in security measures, often exceeding the capabilities of individual organizations. This ensures that sensitive Big Data is protected from unauthorized access and cyber threats. A financial services company can leverage cloud-based Big Data analytics to detect and prevent fraudulent activities, safeguarding customer assets.
Scaling Big Data in the Cloud
Scaling Big Data with cloud infrastructure is dynamically expanding the resources needed to manage large, massive datasets cost-effectively and efficiently using cloud-based solutions.
The Scalability of Cloud Infrastructure
We examine a remarkable ability to scale Big Data with cloud infrastructure. Cloud infrastructure expands as needed, which is crucial when dealing with large data volumes. This scalability means you're not locked into a fixed system; it adapts to your requirements.
Flexibility in Action
Elastic scaling is like having a rubber band that stretches as necessary. The cloud data platform service automatically adjusts the resources when data loads vary. Whether there is a surge in data or a lull, your system remains optimized without manual intervention.
Distributing the Load
Horizontal scaling is about dividing the workload across multiple resources. It's teamwork, where each part contributes to data sharing. This approach offers redundancy, fault tolerance, and increased processing power by having multiple units working in parallel.
Boosting Performance
Vertical scaling, on the other hand, is enhancing the capabilities of a single resource. You're not spreading the load; instead, you're increasing the power of a single unit. It improves performance and processing efficiency.
Practical Implementation
In the tech world, companies have embraced these scaling techniques to address the demands of Big Data. They've implemented these strategies, often using a mix of horizontal and vertical scaling to optimize their data deployment.
The Big Picture
Scaling Big Data with cloud infrastructure isn't just a tech concept; it's a game-changer. It's about efficiency, cost-effectiveness, and adaptability in processing vast data volumes and the key to staying ahead in a data-driven world.
Transforming Data Processing for Better Results
Optimizing data processing in the cloud means using resources and techniques to make data tasks faster and more cost-effective. Big Data's characteristics, such as volume and velocity, present a unique problem that companies must address.
The Significance of Data Optimization
In this era where data is king, optimizing data processing in the cloud is the key to unlocking the full potential of your information assets, revolutionizing how businesses make decisions and serve their customers.
Fine-Tuning Data
Next, we roll up our sleeves and dive into the toolbox of optimization techniques. We explore methods such as data partitioning, indexing, and compression. These tricks aren't magic; they're about ensuring that data is stored efficiently, sorted for quick retrieval, and trimmed down to save on storage costs.
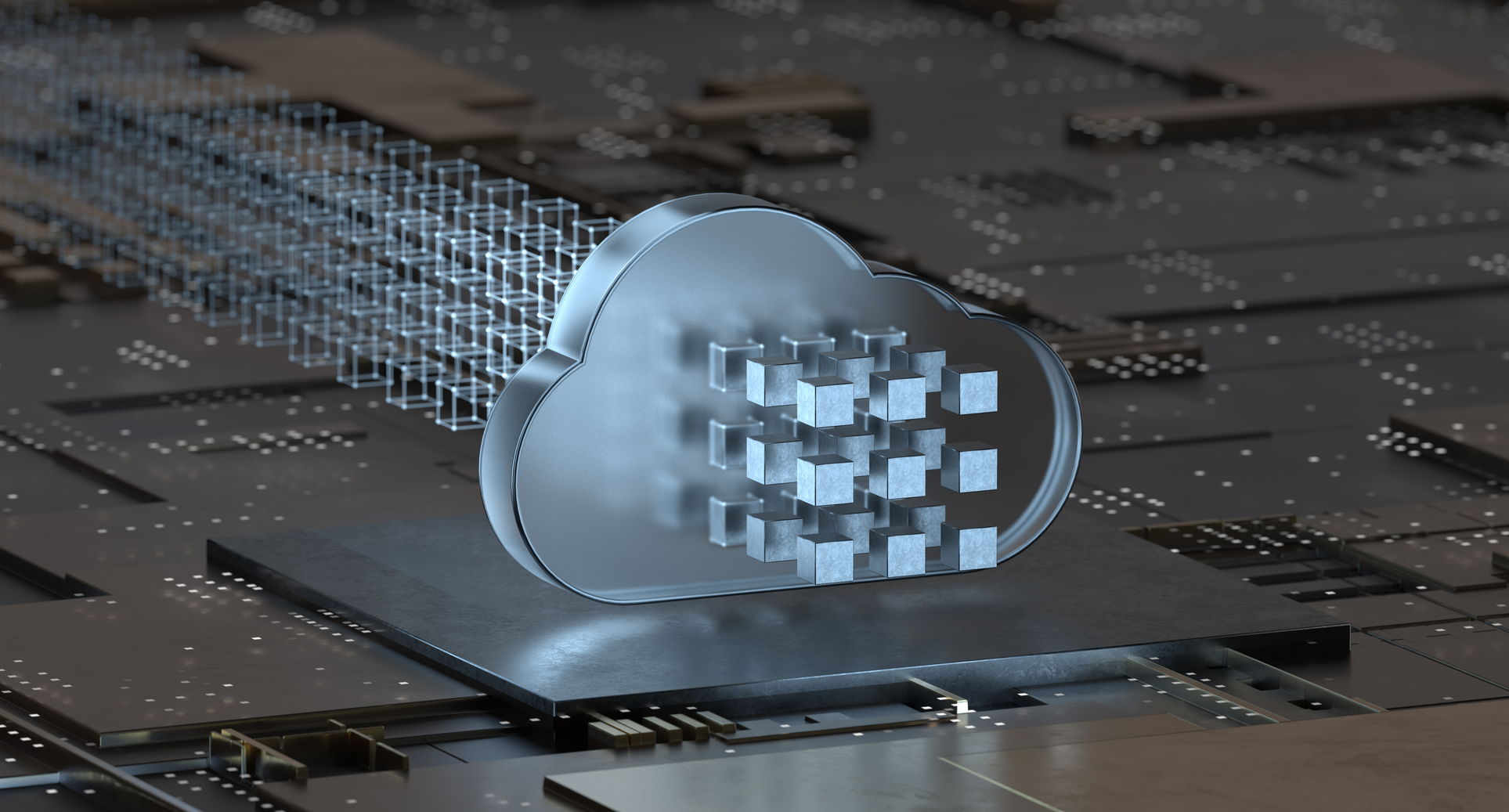
Speeding Up the Race
We then shift gears and introduce the concepts of parallel processing and distributed computing. It's having a whole team working on a puzzle simultaneously, making data processing much faster. We elaborate on how these techniques serve as a transformative force in handling substantial data workloads, improving the speed and efficiency of processing.
Cloud-Powered Insights
Leveraging cloud services for Big Data analytics means utilizing cloud-based tools to process, analyze, and derive valuable insights from large and complex datasets. Big data analytics architecture uses advanced analytics to reveal patterns and correlations.
The Cloud's Treasure for Big Data Analytics
The cloud serves as a goldmine of services tailor-made for big data analytics.
- Data lakes act as vast reservoirs where you can store data in its rawest form, ensuring that it is readily available when needed.
- A data warehouse enables a structured data transformation, cleaning, and analysis environment, serving as a well-organized lab for data scientists and analysts.
- Machine learning platforms offer the tools to deploy ML data models, making predictions and uncovering insights from your data.
- Cloud providers take care of the nitty-gritty technical details, handling infrastructure, scaling, and maintenance, allowing you to focus on your AI analytics.
The beauty of these cloud services lies in their scalability and accessibility from anywhere in the world, promoting real-time collaboration.
Seven Key Advantages of Cloud-Based Analytics
Here are seven main benefits of using cloud-based analytics tools for extracting insights from large amounts of data:
- Cloud-based processing tools offer scalable resources for growing data volumes and fluctuating workloads.
- Pay-as-you-go pricing reduces upfront investments and operational costs.
- The quick implementation allows teams to start analyzing data promptly.
- Cloud platforms provide remote access, enabling collaboration among team members.
- Built-in machine learning and analytics capabilities enhance data analysis.
- Robust security measures protect data and ensure regulatory compliance.
- Cloud platforms offer efficient data storage solutions, simplifying data management.
Cloud-based analytics tools provide advanced analytics capabilities, making them an invaluable resource for data-driven decision-making.
Serverless Computing's Role in Data Analytics
Edge computing technologies are expanding across various domains, from banks to enterprises, enabling real-time data processing. Book a call if you want to always be on the cutting edge of technology.
- Serverless computing eliminates the need to provision servers, ensuring you only pay for the computing resources used during data analytics tasks.
- They automatically scale resources based on workload, allowing for efficient handling of complex data analytics tasks without manual intervention.
- Serverless setups expedite the deployment of analytics tasks, reducing the time it takes to set up and manage infrastructure.
- Serverless platforms handle resource allocation, enabling data analysts to focus on analytics rather than server maintenance.
- Serverless architectures are inherently event-driven, making them suitable for handling real-time data streams as soon as data becomes available.
- With serverless, you know exactly what you'll be charged, providing cost predictability and transparency for analytics projects.
- Serverless platforms automatically adjust resources to match the size and complexity of data analytics tasks, ensuring optimal performance without manual tuning.
- Serverless computing simplifies operational tasks, such as software updates and patch management, freeing time for data analysts.
- The agility and scalability of serverless computing lead to quicker results and insights in complex data analytics tasks.
- Serverless platforms allocate resources precisely to match the needs of a specific task, reducing resource wastage.
To stay at the cutting edge of the industry, businesses need to adopt fully managed serverless data solutions and utilize edge computing cloud technologies.
Mastering Big Data in the Cloud
By following these strategic guidelines, businesses effectively manage Big Data in the cloud, unlocking valuable insights.
- Before embarking on any Big Data initiative, defining clear objectives and identifying specific use cases is crucial. What business problems are you trying to solve? What insights are you hoping to gain? You can ensure that your efforts deliver tangible value by aligning Big Data projects with business goals.
- Selecting the appropriate cloud platform is a critical decision. Evaluate different providers based on their scalability, performance, security, cost, and support for Big Data tools and technologies. Consider your data volume, processing requirements, and budget constraints.
- A well-designed data architecture is the foundation of successful Big Data management in the cloud. Consider factors such as data storage, processing, integration, and access. Choose the right combination of cloud services and tools to create a scalable data architecture.
- Data governance ensures data quality, consistency, and security. Establish clear policies and procedures for data collection, storage, access, and usage. Implement data cataloging and lineage tracking to maintain data integrity and traceability.
- Cloud platforms offer various cloud-native tools and services for Big Data management. These tools, such as data warehouses, data lakes, stream processing engines, and machine learning platforms, simplify and accelerate Big Data processing and analysis.
- Implement robust security measures, such as encryption, access controls, and threat detection, to protect data from unauthorized access and breaches and ensure compliance with relevant data protection regulations.
- Invest in training and development programs to upskill your workforce in cloud technologies, Big Data tools, and data analysis techniques. Foster a data-driven culture within your organization.
- Monitor the performance of your Big Data infrastructure in the cloud regularly. Identify bottlenecks, optimize resource utilization, and fine-tune your data processing workflows to ensure optimal performance and cost efficiency.
- Automation and artificial intelligence (AI) can streamline Big Data management processes, reduce manual effort, and improve efficiency. Leverage AI-powered tools for data cleansing, data integration, anomaly detection, and predictive analytics.
- Stay informed about the latest trends, best practices, and emerging technologies. Continuously evaluate and upgrade your Big Data infrastructure to leverage the latest advancements.
Protecting Big Data in the Cloud
Ensuring security and privacy in Big Data cloud infrastructure means implementing measures to protect sensitive data, maintain data integrity, and prevent unauthorized access or breaches in a cloud-based environment.
The Cornerstones of Big Data Ethics
- Protecting sensitive information
- Maintaining data integrity
- Compliance and legal obligations
- Business Reputation
- Big Data competitive advantage
- Data analytics credibility
- Preventing insider threats
- Data monetization
- Cybersecurity threats
- Data ethics and responsibility

Security Measures by Infrastructure Providers
Data Security and Compliance — Top 10 Best Practices
Ensuring it with relevant regulations is of paramount importance. Here are ten best practices to help companies achieve these goals:
- Classify data based on sensitivity and value.
- Implement encryption for data at rest and in transit.
- Employ strong access controls and authentication methods.
- Stay informed about and comply with relevant data protection regulations.
- Establish data backup and recovery procedures.
- Develop a comprehensive incident response plan.
- Provide security training and raise awareness among employees.
- Assess third-party services for data protection compliance.
- Communicate clear data privacy policies to stakeholders.
- Keep systems and software updated with security patches.
By implementing these best practices, teams foster trust with customers and stakeholders.

Top-3 Cloud Initiatives in Europe
Made in the Digital Age: Big Data and Cloud Computing
With its massive volumes and diverse types of information, Big Data finds a natural ally in cloud computing's scalable infrastructure. This synergy enables businesses to efficiently store, process, and analyze vast datasets without the constraints of traditional on-premise solutions. Cloud's elasticity allows seamless scaling to accommodate fluctuating data demands, ensuring optimal performance and cost-efficiency. Cloud platforms offer a rich ecosystem of Big Data tools and services, empowering organizations to extract valuable insights. The inherent flexibility and accessibility of cloud environments facilitate collaboration among geographically dispersed teams. Moreover, cloud providers invest heavily in security measures, ensuring that sensitive Big Data remains protected from unauthorized access and breaches. This combination enables businesses to gain a competitive edge and optimize operations.
The Complexities of Big Data in the Cloud
A well-defined integration strategy, a focus on interoperability through open standards, and a robust cost management framework are essential for successful Big Data implementation in the cloud.
Integration Complexities
Big Data often originates from diverse sources with unique formats and structures. Integrating this heterogeneous data into a cohesive whole within a cloud environment can be daunting. Data inconsistencies, missing values, and incompatible schemas can hinder seamless integration.
Implementing a robust data integration utilizes data cleansing and transformation tools to standardize data formats, resolve inconsistencies, and enrich data quality. Adopting data integration platforms supporting various data sources and formats can streamline the integration process.
A healthcare organization can use a cloud-based data integration platform to integrate patient data from electronic health records (EHRs), wearables, and medical imaging systems. This enables a comprehensive view of patient health, facilitating personalized care and improved outcomes.
Interoperability Issues
Ensuring interoperability between different cloud platforms and services can be challenging in a multi-cloud or hybrid cloud environment. Data may be stored in various cloud storage systems or processed using different cloud-based analytics tools, leading to potential compatibility issues.
Embracing open standards and APIs (Application Programming Interfaces) is crucial for promoting interoperability. Utilizing cloud-based data exchange platforms that adhere to industry standards can facilitate seamless data transfer and sharing between different cloud environments.
A financial institution can leverage cloud-based APIs to integrate data from its core banking system with a cloud-based risk management platform, enabling real-time risk assessment and mitigation.
Cost Management Concerns
While cloud computing offers scalability and flexibility, uncontrolled costs can quickly escalate. Big Data workloads often require significant computational resources, storage capacity, and data transfer, leading to potential cost overruns if not managed effectively.
Implementing a comprehensive cost management strategy requires monitoring cloud resource usage, identifying cost optimization opportunities, and leveraging cost-saving features offered by cloud providers, such as reserved or spot instances.
A retail company can use cloud cost management tools to track its Big Data analytics expenses, identify peak usage periods, and adjust resource allocation accordingly, ensuring optimal cost efficiency.
Accelerating Benefits of Cloud Infrastructure on the Digital Superhighway
Like a truck driver knows the best routes, DATAFOREST’s experts navigate the infrastructure, avoiding congestion and roadblocks to maximize data processing speed. As the cloud infrastructure, the digital superhighway is scalable and expands to handle increasing data loads, allowing our team to adapt seamlessly. Security measures on this superhighway protect the data cargo, preventing cyber threats, much like a team of highway patrol officers. Our company also manages large knowledge graphs that help businesses extract insights from industry data. Please fill out the form, and we will go on an exciting and rewarding journey together.
FAQ
What are the benefits of a cloud infrastructure?
The benefits of a cloud infrastructure include scalability, cost-efficiency, flexibility, and accessibility. It allows businesses to manage and process data while efficiently adapting to changing needs.
What is the role of cloud infrastructure in handling big data?
Cloud infrastructure is the foundation for storing, processing, and managing large volumes of Big Data, offering scalability, cost-efficiency, and accessibility to unlock valuable insights and applications.
How does cloud infrastructure enable scalability for managing large volumes of data?
Cloud infrastructure enables scalability by allowing businesses to dynamically allocate and expand resources to accommodate fluctuating data loads, ensuring optimal performance and cost-efficiency.
What are the key optimization techniques for processing Big Data in the cloud?
Essential optimization techniques for processing Big Data in the cloud include data partitioning, indexing, compression, parallel processing, and distributed computing to enhance storage, retrieval, and processing efficiency.
How can businesses leverage cloud services for Big Data analytics?
Businesses can leverage cloud services for Big Data analytics by utilizing data lakes, data warehouses, machine learning platforms, and scalable benefits of cloud computing infrastructure to extract valuable insights and drive informed decision-making.
What security measures are in place to protect data in a big-data cloud infrastructure?
Security measures such as encryption, access controls, and compliance certifications are in place to safeguard data in a Big Data cloud infrastructure, ensuring the protection and integrity of sensitive information.
Are there any benefits of hybrid cloud backup infrastructure?
The benefits of hybrid cloud backup infrastructure include enhanced data resilience, flexibility, and cost-efficiency, offering businesses a reliable data protection and disaster recovery solution. It's only one of the benefits of hybrid cloud infrastructure.
What are the vital benefits of moving infrastructure to the cloud?
The vital benefits of moving infrastructure to the cloud include scalability, cost-efficiency, enhanced security, and improved accessibility to data and applications, enabling businesses to adapt, grow, and streamline their operations.
How do the benefits of private cloud infrastructure compare with others?
The benefits of a private cloud infrastructure, such as enhanced security and control, are balanced with higher costs and limited scalability compared to public or hybrid cloud alternatives.
What is one of the advantages of implementing dynamic scaling to the cloud infrastructure?
Dynamic scaling to the infrastructure of the cloud benefits the system's performance and resource allocation.
How are cloud, IoT, and Big Data related?
The Internet of Things (IoT) generates vast amounts of data from interconnected devices and sensors, which is then stored and processed in the cloud's scalable infrastructure. Big data analytics tools within the cloud environment analyze this data, extracting valuable insights that drive informed decision-making and innovation.