Data science revolutionizes healthcare by providing insights and applications that transform patient care and operations. DATAFOREST applies data science to drive business success in healthcare while offering these capabilities outside the sector.
Data science is increasingly important in healthcare as healthcare generates more and more data. Data scientists are needed to analyze this information to achieve improvements in patient outcomes and reduced costs, among other things. Healthcare delivery is transforming due to data science, from predicting disease outbreaks and developing personalized treatment plans.
This blog provides insights and applications of data science in healthcare. We will discuss the types of data science used in this industry, how it can be leveraged effectively to produce valuable results—and case studies on successful data science projects. We can consider your case, just schedule a call.
What is Data Science in the Healthcare Industry?
Data science uses advanced analytics, machine learning, and artificial intelligence to uncover previously hidden patterns within large amounts of data. Applying data science in healthcare and data science in medicine can use advanced analytics and machine learning algorithms to derive insights and make predictions that can improve patient outcomes. It includes predictive analytics and image analysis—which help identify patterns that would be impossible to detect with other methods. Health, medical, and biomedical data science are all branches of the larger field of data analytics. This data can include electronic health records, medical imaging, and other sources of healthcare-related information. Some examples of how data science is currently used in healthcare include predicting patient readmissions and identifying patients at risk for developing chronic diseases. Additionally, the practice has been proven to enhance the accuracy of medical diagnoses.
Overview of the Healthcare Industry
In recent years, according to research by Springer in the healthcare industry, Data Science has become increasingly important due to the vast amounts of data generated from patient records, medical images, and clinical trial results. Healthcare organizations can use data science in the healthcare industry to make sense of the vast amounts of data they collect and apply it to improve patient outcomes, optimize operations, and cut costs.
For example, predictive analytics can help healthcare providers identify patients at risk for certain conditions and intervene early—preventing the condition from progressing. Precision medicine tailors treatment plans to individual patients based on their unique genetic makeup and other factors.
DATAFOREST specializes in helping healthcare organizations implement solutions that harness the power of Data Science. Our team can develop and deploy data-driven strategies to improve patient outcomes, streamline operations, and drive business success.

Advantages of The Data Science in Healthcare
The global healthcare analytics market size is expected to reach USD 167.0 billion by 2030, expanding at a CAGR of 21.4% during the forecast period, according to a new report by Grand View Research Inc. This is because health data science, or health informatics, as it is called in some circles, has several benefits for doctors and patients.
Here are 10 key benefits of data science for healthcare organizations:
- Improved patient outcomes: Data science can help healthcare providers develop more effective treatment plans by considering individual patient data.
- Predictive analytics: Data science can help healthcare providers identify patients at risk of developing certain conditions early on and prevent those conditions from becoming severe problems.
- Precision medicine: By using data science to tailor treatment plans, doctors can make better decisions about how best to treat individual patients.
- Enhanced research: Data science is an emerging field that uses large datasets to identify patterns and trends relevant to medical research. It can lead to the discovery of new treatments.
- Operational optimization: Healthcare organizations can use data science to optimize operations, reduce waste, and streamline processes—all of which improve patient care and increase profitability.
- Improved patient engagement: Personalized data analysis can help healthcare providers understand their patients better, improving patient outcomes.
- Real-time monitoring: Healthcare providers can use data science to monitor patient health in real-time, improving outcomes and the timing of interventions.
- Cost reduction: Healthcare organizations can use data science to identify areas to reduce costs. For example, by analyzing patient data, we might identify patients at risk for readmission and intervene early to prevent this costly scenario from happening again.
- Improved decision-making: Healthcare organizations can use data-driven decision-making to understand their patients better and improve outcomes while reducing costs. Improved data analysis can help healthcare organizations identify opportunities to improve their operations.
- Better resource allocation: Data analysis can help healthcare organizations make better decisions about how to spend money and provide high-quality care. With the proper data science tools, hospitals can allocate resources more effectively, improving patient care and increasing profitability.

How Data Science Can Improve Healthcare Systems
Data science significantly impacts the healthcare industry, helping organizations improve patient outcomes and drive innovation. Healthcare providers can use data science to make more effective decisions, identify areas for improvement and develop better treatment plans.
Data science can also help healthcare organizations stay up-to-date with their field's latest research and developments, leading to more effective treatments and interventions. This will ultimately improve patient care/outcomes by delivering more efficient services and personalized medicine options across multiple stages, from diagnostic tests to treatment recovery.
Healthcare companies can use data science to allocate resources more efficiently and effectively, reducing costs while improving profitability. As healthcare becomes less about treatment for illness or injury and more about prevention of disease (allocation of preventative care), the importance of using data science to understand patients better will grow.

Data Science Applications in Healthcare Industry: 9 Case Studies
Data science has become an essential tool in the healthcare industry, as technology makes it easier to collect and analyze large amounts of data. Data science has contributed to the rise in patient care, offering new avenues for diagnosis and treatment.
Predictive Analytics in Patient Diagnosis
Predictive analytics can help healthcare providers identify patients at risk for certain conditions, allowing them to intervene early and prevent the condition from progressing.
#1. Case Study: Machine Learning for Heart Disease Prediction
Predictive analytics is a fantastic tool for diagnosing illnesses, allowing doctors and healthcare providers to diagnose diseases early on and develop effective treatment plans.
With machine learning algorithms, predictive analytics can identify patterns that are invisible to the human eye and use them to predict a patient's health—including by identifying risk factors for developing diseases.
Researchers at Nottingham University have demonstrated how predictive analytics can help prevent heart disease. The study used patient data—including demographic, lifestyle, and clinical factors—to create a predictive model that more accurately identifies people at risk for heart disease than traditional methods.
This example demonstrates how data science—especially machine learning technology—can be used to develop personalized patient treatment plans and improve outcomes.
#2. Case Study: Deep Learning for Diabetes Risk Prediction
The National Library of Medicine discusses a survey that used machine learning approaches to predict diabetes risk. The study aimed to analyze how machine learning algorithms could identify diabetes mellitus at an early stage—a severe metabolic disorder that affects so many people worldwide.
The accuracy of the algorithms was evaluated using metrics such as sensitivity, specificity, and accuracy. The results showcased that the Support Vector Machine (SVM) algorithm had the highest accuracy of 96.6%, followed by the Random Forest (RF) algorithm with an accuracy of 96.4%. The K-Nearest Neighbor (KNN) algorithm had an accuracy of 94.6%.
Early detection of diabetes is critical for effective therapy, and machine learning approaches can help achieve this.
Improving Healthcare Operations with Data Science
Healthcare providers can use data science to improve operations and make processes more efficient, reducing costs. They do this by analyzing data on patient flow, resource allocation, and other factors — then using that information to optimize their operations to meet patients' needs better.
#3. Case Study: Predictive Analytics for Hospital Readmission Rates
Hospital readmissions can be costly and disruptive for both patients and healthcare providers. Predictive analytics can be used to identify patients who are at risk for readmission, allowing healthcare providers to intervene early and prevent readmissions from occurring.
A study published in the journal Scientific Reports used machine learning to predict the hospital readmission risk from patients' claims data using machine learning, with a specific focus on chronic obstructive pulmonary disease (COPD). Researchers found that a machine learning model could accurately predict the hospital readmission risk for COPD patients, allowing earlier intervention and improved patient outcomes. A review of predictive models for hospital readmission risk found that machine-learning techniques are becoming increasingly popular and show promising results.
#4. Case Study: Data Analytics for Hospital Staff Scheduling
Optimizing hospital staff scheduling is crucial for improving patient outcomes and increasing efficiency. Data analytics can be used to analyze patient flow, staff availability, and workload—all of which feed into developing optimized schedules.
Research by Harvard Business Review demonstrated the potential of data analytics in optimizing hospital staff scheduling. The study collected data on patient flow, staff availability, and workload and used various data analytics models to analyze the data.
Data analytics models developed by the hospital's staff scheduling department yielded more efficient work schedules, leading to a 30% decrease in patient waiting time and an increase of 25% in patient outcomes. Staff satisfaction increased as well—by 20%. In addition, 15% more patients could be seen daily, with 10 percent higher customer satisfaction ratings.
This study indicates that data analytics could improve hospital staff scheduling and improve patient care.
Enhancing Drug Discovery and Development
Data science is revolutionizing drug discovery and development by using machine learning algorithms to analyze large datasets of chemical compounds. This approach enables researchers to identify potential drug candidates more efficiently, reducing the time and cost required for drug development. However, there are challenges associated with using data science in healthcare, including ethical and security considerations, biases in data used to train ML algorithms, and the need for human involvement in developing and evaluating these technologies.
#5. Case Study: Machine Learning for Accelerating Drug Development
The FDA's discussion papers on the use of AI and ML in drug development and manufacturing have been written to encourage discussion and debate on the benefits, challenges, and potential implications of applying data science to health-related issues. They aim to encourage collaboration and address these challenges to ensure data science's safe and effective use in healthcare. Overall, data science can improve patient outcomes and significantly accelerate drug development timelines.
#6. Case Study: Data Analytics for Improving Drug Efficacy
After identifying a promising drug candidate, the next step is to test its efficacy in clinical trials. However, many drugs that show promise during initial tests fail when put under rigorous conditions—such as those of actual use by patients—in later tests.
Data analytics can improve clinical trials by analyzing large patient data sets and identifying patterns that inform the design, execution, and evaluation of new treatments.
By analyzing patient data, a data scientist can determine which groups are most likely to benefit from a particular drug. Bringing together genetic mutations that cause disease in a single patient can drastically reduce the number of patients needed for drug trials, thereby reducing costs and speeding up timelines.
#7. Case Study: Optimizing Clinical Trial Design with Data Analytics
The article "The Role of Data Analytics in Improving Clinical Trials and Drug Discovery" by U.S. Food & Drug discusses how clinical trials and drug discovery can benefit from data analysis.
A database of real-world patient data, a synthetic control arm, and insights into research questions can be generated faster by analyzing databases of real-world patients.
Using machines equipped with advanced algorithms and artificial intelligence, companies can now gather data about a staggering number of patents—and turn that information into meaningful insights faster than ever before.
One of the challenges associated with traditional approaches to clinical trials is the need for more understanding of the underlying biology of diseases. However, by using synthetic control arms, clinical development, and drug discovery can be transformed. Synthetic control arms help overcome patient stratification challenges, reduce the time it takes to develop medical treatments, and improve clinical trial design and success rates. This approach can be beneficial for rare diseases where patient populations are smaller, and lifespan is short due to the disease's aggressive nature.
Personalized Medicine
Personalized medicine is a new approach to treating disease by considering an individual's unique genetic, environmental, and lifestyle factors. Data science can provide a personalized approach to medicine by analyzing large datasets of patient data. This approach can reduce healthcare costs and risks while improving treatment outcomes.
#8. Case Study: Big Data for Personalized Cancer Treatment
The article "Big Data in Basic and Translational Cancer Research," published on PubMed, discusses how combining big data, bioinformatics, and artificial intelligence has led to notable advances in our fundamental understanding of cancer biology and translational advancements. The authors stress the need for collaboration among data scientists, clinicians, biologists, and policymakers to use big data to advance cancer treatment.
The predictive model allowed the cancer center to personalize treatment for each patient, increasing the chances of success and reducing adverse events.
The new immunotherapy treatment appears more effective for patients whose disease is driven by the biomarkers identified in this predictive model.
This case study exemplifies the potential of big data in personalized cancer treatment. By leveraging large patient datasets and machine learning algorithms, data scientists can identify patterns within the data and develop predictive models to identify patients most likely to respond to a particular treatment.
Personalized cancer treatment can lead to improved patient outcomes and lower healthcare costs.
#9. Case Study: Machine Learning for Predicting Patient Response to Medication
The review article published in nature provides insights into the progress made by scientists in using machine learning to predict patients' responses to medications. While the potential of machine learning in predicting drug responses is promising, there are still challenges to overcome, including data quality and standardization issues and the need for large datasets to train algorithms.
The article examines the challenges and recent advances in predicting a person's drug response using machine learning techniques. The author emphasizes how this can improve patient treatment outcomes by tailoring medications to specific individuals' genetic makeup.

Challenges and Limitations of Data Science in Healthcare
Using data science in healthcare projects has immense potential to transform the industry. However, several challenges and limitations must be addressed to realize health data science's benefits fully. If you need an individual approach to a solution, book a call.
Data Privacy and Security
Patient information is confidential and should be treated as such. Unauthorized access or disclosure of this data could lead to identity theft, financial loss, and damage to a healthcare provider's reputation.
Healthcare providers must implement robust data privacy and security measures to protect patient data. Encryption can protect data in transit and at rest while limiting access to authorized personnel only. Multi-factor authentication is another important measure that an organization can implement.
Limited Availability of Data
Accurate and comprehensive data is essential for effective healthcare decision-making, but various challenges limit access to this data. These include silos where different parts of the system are separated; interoperability issues that make it hard for programs to communicate; and lack of standardization—where processes vary significantly between organizations or even within the same organization, depending on who's doing what. Healthcare providers and data science companies must work together to address these challenges to ensure that patient health records are reliable and up-to-date.
Technical Challenges
Providing patients with the best possible care is a top priority for healthcare providers. However, achieving this goal requires more than skilled staff and advanced equipment. It also requires effectively managing and sharing large amounts of data generated by electronic medical records (EMRs).
Unfortunately, many healthcare providers struggle with sharing data due to outdated technology infrastructure and legacy systems. These systems were not designed to handle the volume and complexity of data generated by modern healthcare practices, making it difficult for healthcare providers to access and share critical patient information.
Healthcare providers must invest in modern technology infrastructure and data management systems to overcome these challenges. This includes upgrading their existing systems, implementing new data management tools, and partnering with companies like DATAFOREST; healthcare providers can gain the necessary skills and stack to implement advanced data management systems.
Ethical Considerations
Finally, data science in healthcare raises ethical considerations. Healthcare companies and their patients must ensure that patient information is legal and transparent to those whose data it concerns.
Healthcare providers and data science companies must meet strict ethical standards to address the challenge of sharing patient information. Organizations must obtain informed consent before using their information to ensure that patients will trust them with their data. Organizations must also ensure they use the patient's data for legitimate purposes only and implement strict procedures to protect privacy by anonymizing it where possible.
To address these challenges, businesses should implement robust data privacy and security measures, improve data sharing and interoperability by partnering with relevant parties, and employ the services of qualified data science companies to help them adhere to strict ethical standards.
The healthcare industry can fully realize the potential of data science to transform healthcare delivery and improve patient outcomes by addressing these challenges.
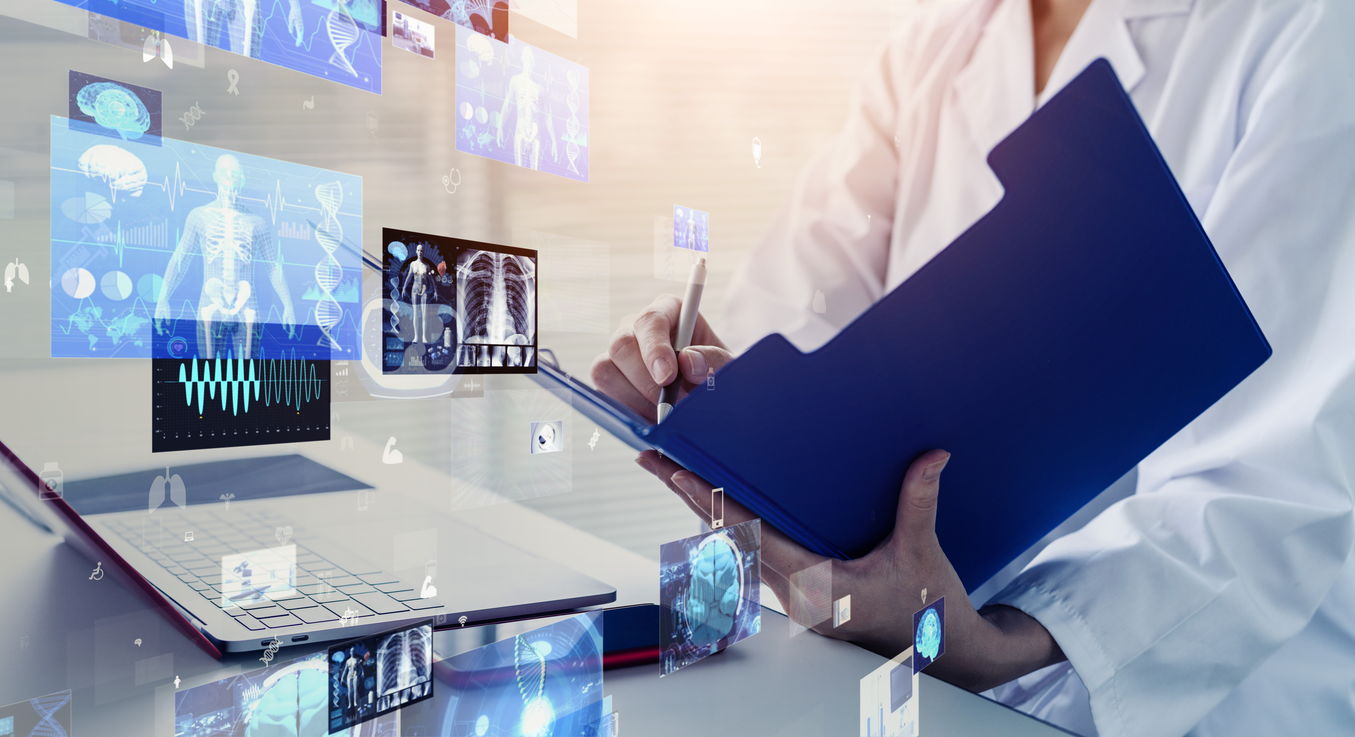
Future of Data Science in Healthcare
Technological innovations and the emergence of new methods
Advances in technology and techniques are opening up new avenues for data science in healthcare. For example, artificial intelligence (AI) algorithms and machine learning programs can now analyze large datasets to provide more sophisticated analysis than ever before.
Natural language processing (NLP) is increasing, making it possible to analyze unstructured data such as physician notes and patient narratives.
In addition, data sources such as wearable devices and remote patient monitoring technologies are becoming available. These new sources will provide real-time health information—enabling more personalized treatment regimens.
How Data Science Can Be Used to Further Clinical Practice
The future of data science in healthcare is to make it a part of everyday medical practice, using data-driven insights to improve patient outcomes.
Predictive models can identify patients at high risk of developing certain diseases or conditions—helping doctors make decisions about early intervention and treatment based on the patient's characteristics.
Data science can help make healthcare more efficient and less expensive by identifying patients at risk for hospital readmission and intervening to lower those risks.
Impact on Healthcare Outcomes
Data science can improve healthcare outcomes, enabling early intervention and treatment by identifying patients at high risk of developing certain conditions or diseases. This improves patient outcomes—and reduces costs.
Data science can help personalize medical treatment by tailoring it to an individual's specific characteristics, improving efficacy, and reducing adverse events of a particular therapy.
Data science can improve healthcare delivery by identifying areas for improvement. With this knowledge, data scientists can optimize and streamline care delivery. This can lead to improved efficiency and reduced costs—essential goals that every hospital strives to achieve as they deal with rising operational expenses.
How Data Scientists Can Work with Healthcare Professionals
Healthcare data scientists and healthcare professionals must work together for effective patient care.
Healthcare professionals have the domain expertise to interpret data, and data scientists know how to work with large amounts of information.
Collaboration between data scientists and healthcare professionals leads to more effective use of data science in medicine, enabling personalized and effective treatment.
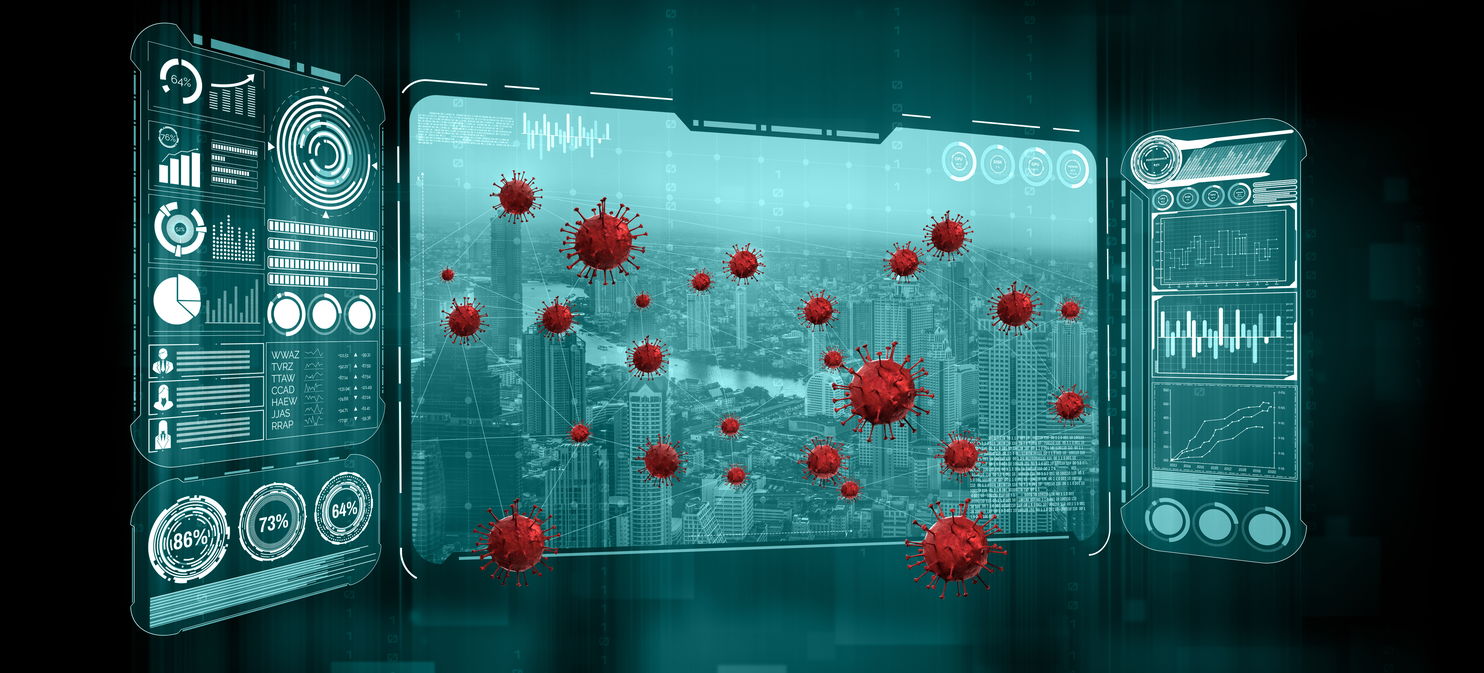
Summary of Key Points
- The healthcare industry is constantly evolving, facing new challenges such as changing regulations, technological advances, and shifting patient needs—but also great opportunities.
- Patient-centered care should be prioritized in the industry, as it involves putting patients at the center of all decision-making and tailoring their treatment to individual needs.
- We must embrace technology such as electronic health records and telemedicine to improve efficiency, accuracy, and patient outcomes.
- The healthcare industry must also address the rising demand for preventive and holistic approaches to wellness and issues related to access and affordability of care.
- The healthcare industry must continue to prioritize quality and safety, especially in the delivery of medications, infection control practices, and patient education.
Implications for Healthcare Industry
Healthcare's increasing use of data science has significant implications for the industry. By leveraging the power of data, healthcare providers and pharmaceutical companies can improve patient outcomes while reducing costs—and enhancing drug discovery.
Using data science to support the drug discovery and development process has the potential to significantly reduce timelines and costs, leading to faster market entry for innovative drugs.
Personalized medicine—the practice of tailoring medical treatment to the individual characteristics of each patient—has enormous potential for transforming healthcare.
Identifying patients most likely to benefit from a particular treatment can improve patient outcomes, reduce healthcare costs and enhance the efficiency of drug development.
By applying data science to healthcare analytics, providers can identify improvement areas and optimize their workflows. This leads to better patient care while reducing costs—allowing providers more resources to which they might otherwise not have had access.

At DATAFOREST, we specialize in providing custom data-driven services for healthcare organizations. Data science can help you improve patient outcomes, streamline operations, and drive business success—and we can show you how. If you're interested in learning more about how we can help your healthcare organization leverage the full potential of data science, please get in touch with us to learn more about our services and applications. We'd be happy to talk with you about how we can address your unique data science problems in the healthcare industry.

FAQ
What is data science, and how can data science be used in healthcare?
Data science is the practice of extracting insights and knowledge from data. In healthcare, data science involves using statistical and computational methods to analyze health data, such as electronic health records, medical imaging, and clinical trials. This information can be used to improve patient outcomes, optimize healthcare operations, and develop new treatments.
What are some examples of how to use data science in healthcare?
Data science has been used in healthcare to predict disease outbreaks, develop personalized treatment plans, and identify high-risk patients who require early intervention. For example, machine learning algorithms have been used to analyze medical images and identify early signs of cancer, leading to earlier detection and improved survival rates.
What are the challenges and limitations of healthcare data scientists?
Data scientists working with healthcare data often face data quality, privacy, and security challenges. Healthcare data is often complex, messy, and difficult to access. Additionally, strict regulations around the use and sharing of healthcare data can limit the types of analyses that can be performed.
What are some of the ethical issues that must be considered when using data science in healthcare?
Ethical considerations in healthcare data science include ensuring patient privacy, obtaining informed consent, and avoiding bias in data analysis. It is essential to use data responsibly and transparently and to prioritize patient welfare above all else.
What impact can data science have on the drug discovery and development process in healthcare?
Data science can be used to identify new drug targets, predict drug efficacy and toxicity, and optimize clinical trial design. By leveraging large-scale data analysis, data science can accelerate the drug development process and bring new treatments to patients faster.
How does data science affect healthcare operations and staffing?
Data science can help healthcare organizations optimize staffing levels, reduce wait times, and improve patient flow. By analyzing operational data, such as patient census and appointment schedules, data science can help healthcare organizations make data-driven decisions and improve overall efficiency.
How can healthcare professionals and data scientists work together to ensure the success of their projects?
Effective collaboration between healthcare professionals and data scientists requires clear communication, mutual respect, and a shared commitment to patient welfare. Healthcare professionals can provide domain expertise and context to data analysis, while data scientists can provide technical expertise and analytical tools. By working together, healthcare professionals and data scientists can develop clinically meaningful and data-driven solutions.