Data science in cybersecurity is a vigilant and resourceful guardian standing at the gates of a fortress, equipped with an array of tools and techniques to protect against unseen threats. Imagine the fort as a network or system housing valuable information, and the guardian represents data scientists utilizing their skills and expertise. They keenly watch for unusual activities that may indicate potential threats or anomalies. If you want to always be on the cutting edge of technology, schedule a call.
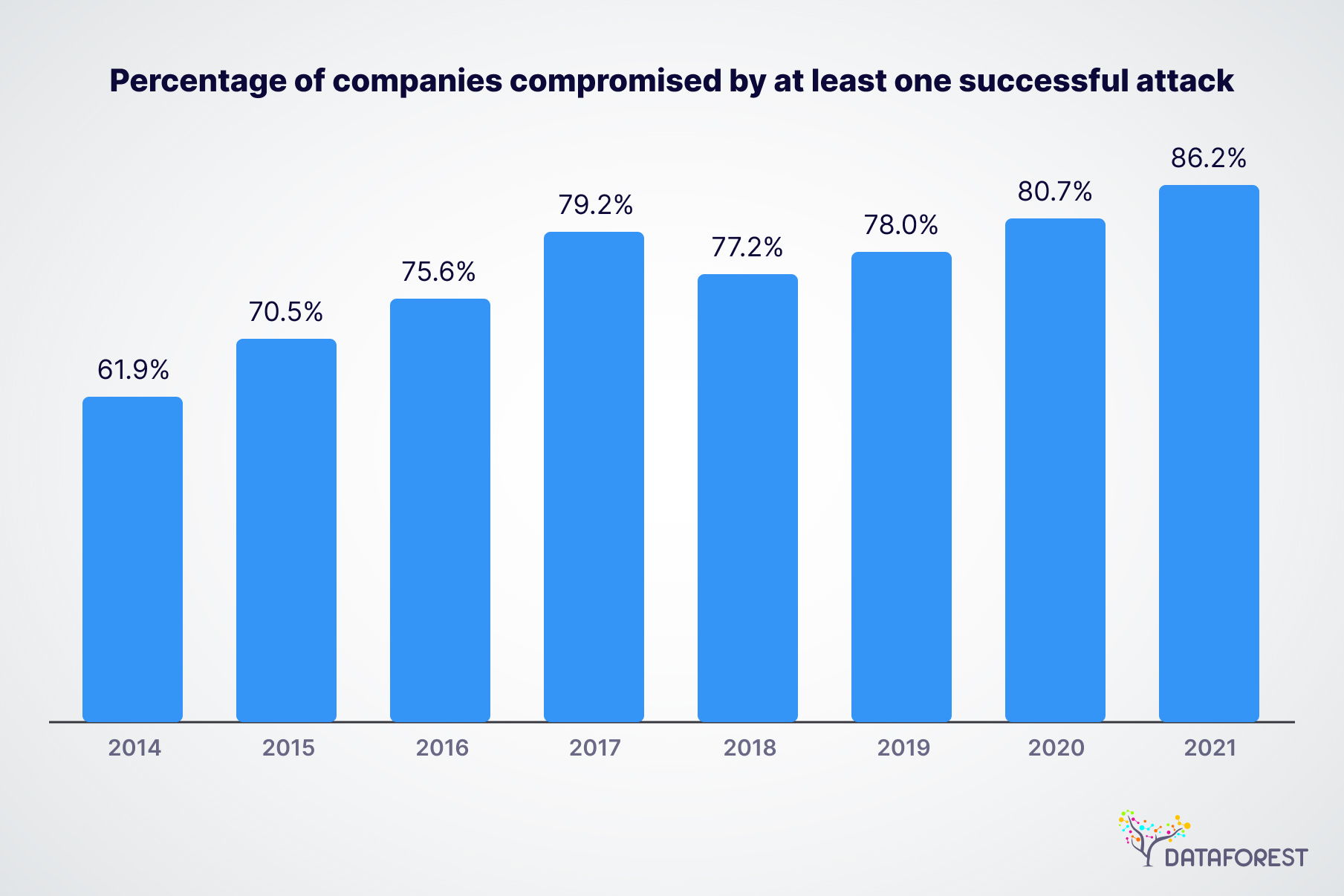
Percentage of companies compromised by at least one successful attack
The Growing Threat of Cyber Attacks for Data Science in Cybersecurity
Cybersecurity is vital to protect sensitive data, preserve privacy, safeguard national security, prevent financial losses, maintain business continuity, defend individuals, and address the evolving threat landscape. A comprehensive and proactive approach to cybersecurity is essential to mitigate cyberattack risks and ensure digital systems and networks' integrity, security, and resilience.
Data science in cybersecurity goals
- Anomaly detection
- Threat intelligence
- Behavioral analysis
- Predictive analytics
- Incident response and forensics
- Threat hunting
- Automation and scalability
Anomaly detection
Anomaly detection, also known as outlier detection, is a technique used by data science in cybersecurity to identify rare, unusual, or abnormal patterns or events within a dataset. It implies distinguishing between normal and atypical behavior that deviates significantly from the norm. Anomaly detection is crucial in identifying security breaches or malicious activities that may go unnoticed by traditional rule-based or signature-based systems.
- Anomaly detection discovers previously unseen or unknown threats that have not been encountered before.
- With data science in cybersecurity, anomaly detection allows for the early detection of security breaches by identifying deviations from expected patterns.
- Anomaly detection techniques effectively detect insider threats, where authorized individuals abuse their privileges or engage in malicious activities.
- Anomaly detection identifies anomalous behaviors or activities that may indicate the presence of a zero-day exploit.
- Anomaly detection can adapt to new attack patterns and variations by learning from historical data and detecting deviations from normal behavior due to data science in cybersecurity.
- Anomaly detection reduces false negatives (undetected security breaches) and false positives (normal behavior classified as anomalies).
- Anomaly detection provides valuable data-driven insights into the security posture of a system or network.
These critical components of data science in cybersecurity enhance the effectiveness of monitoring, incident response, and overall risk management.

What else is there for data science in cybersecurity
- Machine learning algorithms — supervised learning, unsupervised learning, and reinforcement learning — are utilized in cybersecurity. They learn patterns, classify data, and make predictions based on training data.
- Deep learning techniques — convolutional neural networks (CNNs) and recurrent neural networks (RNNs) — have proven effective in tasks like image recognition, natural language processing, and malware detection by data science in cybersecurity.
- Data science techniques facilitate collecting, analyzing, and interpreting vast amounts of data to extract actionable insights for threat intelligence. Data mining, text mining, and natural language processing techniques help identify trends.
- With the increasing volume, velocity, and variety of data generated in cyberspace, big data analytics techniques of data science in cybersecurity enable efficient processing, analysis, and visualization of large-scale datasets.
- Behavioral analytics techniques focus on analyzing and modeling user behavior patterns, network traffic, and system activities to detect anomalies and potential security threats.
- Predictive analytics leverages historical data, machine learning algorithms, and statistical models to forecast future events and anticipate potential cyber threats with data science in cybersecurity.
- Data visualization techniques provide intuitive visual representations of cybersecurity data. They aid in identifying patterns, correlations, and anomalies in large datasets, making it easier for security analysts to interpret complex info.
When combined with domain expertise, these data science and cybersecurity techniques enhance cybersecurity by improving threat detection.
Data Science in Cybersecurity: Discernment of a Guardian
Understanding anomaly detection in cybersecurity is the keen perception of a guardian who can recognize subtle shifts in behavior that deviate from the norm within the fortress they protect. An anomaly detection system is a vigilant guardian, constantly observing the data flowing through the digital defense. Anomaly detection systems monitor the data to identify any unusual activities that may indicate potential security breaches.
Activity deviation
In the context of data science in cybersecurity, an anomaly is any behavior or pattern that deviates significantly from what is considered normal within a given system or network. Anomalies indicate potential security breaches, malicious activities, or system vulnerabilities that require further investigation.
- Anomaly detection in network traffic identifies abnormal communication patterns or unexpected data flows within a network.
- Anomalies in user behavior can indicate unauthorized access attempts, insider threats, or compromised user accounts by data science in cybersecurity.
- Anomalies can be identified by monitoring system processes and detecting deviations from expected behaviors.
- Anomalies can occur within applications or software and may signal potential security issues: unexpected crashes, excessive errors, or irregular resource consumption with data science in cybersecurity.
- Anomalies may be observed in the distribution of packet sizes, transaction amounts, access frequency, or other data characteristics.
Establishing a baseline for data science in cybersecurity
Differentiating between normal and abnormal behavior in network traffic, user activity, and system logs requires data science in cybersecurity to establish a baseline of what is considered normal and then identify deviations from that baseline.
Schedule a call to complement reality with a profitable solution.
Preventing security breaches
Timely anomaly detection plays a crucial role in mitigating security breaches.
- Companies initiate immediate response measures to minimize the breach's impact, limit further compromise, and prevent potential data loss or damage by promptly detecting anomalies with data science in cybersecurity.
- Security teams proactively identify vulnerabilities, close security gaps, and implement additional preventive measures to strengthen defenses by detecting deviations from normal behavior or patterns.
- In data science in cybersecurity, timely anomaly detection reduces dwell time by quickly identifying potential breaches, thereby minimizing the time adversaries have to exploit vulnerabilities, steal data, or cause further damage.
- Firms address insider threats swiftly, mitigate risks and prevent potential harm to sensitive data or systems by promptly detecting anomalous behaviors, abnormal data access, or unauthorized system changes.
- Advanced threats — sophisticated malware, targeted attacks, or zero-day exploits — are designed to evade traditional security controls. Timely anomaly detection helps identify these advanced threats by detecting unusual patterns or behaviors.
- Companies promptly initiate data science in cybersecurity incident response procedures, contain the breach, mitigate damage, recover affected systems, and prevent further attack spread by identifying breaches early.
- Ongoing monitoring enables security teams to adapt to threats, detect emerging attack techniques, and update defenses accordingly. Firms enhance their security posture and stay one step ahead of cyber adversaries by continuously analyzing anomalies.
Timely anomaly detection in order of data science in cybersecurity helps organizations maintain a robust security posture and protect their critical assets and sensitive information from malicious actors.
Gathering and Preparation of Vital Info for Data Science in Cybersecurity
The imaginary data science guardian is responsible for collecting information from various sources, ensuring its accuracy, and preparing it for further analysis. They act as a gatekeeper, carefully organizing the data that will be used to fortify the fortress.
Data sources provide valuable insights
- Network traffic logs capture information about the communication between devices within a network in data science in cybersecurity. They include source and destination IP addresses, ports, protocols, packet sizes, timestamps, and other metadata.
- System logs record activities and events within an operating system or software application. They provide information about system events, errors, warnings, configuration changes, resource utilization, and user activities.
- User behavior logs capture information about the actions and activities of users within a network or system. These logs include login attempts, file access events, commands executed, system privileges utilized, and other user-related activities in order of data science in cybersecurity.
- Security event logs — security information and event data management (SIEM) logs — collect information from various security devices, including firewalls, intrusion detection systems (IDS), intrusion prevention systems (IPS), and antivirus software.
- Application logs record events and activities of software applications or services. They include information about user interactions, error messages, access attempts, system integration events, and other application-specific events.
- Threat intelligence in data science and cyber security feeds provides updated info about known threats, vulnerabilities, malware signatures, and indicators of compromise. They are obtained from trusted sources: security vendors, government agencies, or threat intelligence platforms.
- Endpoint logs capture information from individual devices (endpoints) within a network. They include details about processes executed, file changes, system events, network connections, and user activities specific to each endpoint.
Ensuring the quality of subsequent analysis with data science in cyber security
Collecting and preprocessing cybersecurity data presents several challenges that need to be addressed to ensure the effectiveness of subsequent analysis.
- Handling the data volume and velocity is challenging, requiring scalable infrastructure, storage, and processing capabilities in real time.
- Integrating heterogeneous data poses challenges in preprocessing, requiring data mapping, transformation, and normalization techniques to create a unified dataset within data science in cybersecurity.
- Data from different sources may contain errors, inconsistencies, or missing values. They are cleaning the data to address quality issues and handle outliers.
- Ensuring data privacy, adhering to data protection regulations, and implementing anonymization techniques add complexity to data collection and preprocessing.
- In cybersecurity data science, analyzing data in real-time to detect anomalies necessitates advanced data processing techniques, distributed computing, parallel processing, and stream frameworks.
- Integrating data across networks, system logs, and user activities requires effective data fusion, considering temporal relationships, context, and data dependencies.
- Class imbalance poses challenges in training accurate models and anomaly detection algorithms, requiring oversampling, undersampling, or ensemble methods.
- Data collection, preprocessing, and analysis should be performed on time to ensure timely anomaly detection and incident response by cybersecurity data science.
Data science in cybersecurity prepares data for anomaly detection
Several techniques are used for data cleaning, normalization, and feature engineering to prepare data for anomaly detection.
Data cleaning
- Techniques like imputation (filling in missing values with estimated values based on other data points) or deleting rows/columns with missing data can be employed.
- Outlier detection methods of cybersecurity data science — statistical techniques or machine learning algorithms — identify outliers through removal, imputation, or replacement with estimated values.
Data normalization
- The Min-Max Scaling technique rescales data to a specific range (0 to 1) based on the minimum and maximum values of the feature. It helps in achieving uniform scales.
- Z-score standardization transforms data with a mean of 0 and a standard deviation 1. It allows data science in cybersecurity to compare data in terms of standard deviations from the norm.
- Logarithmic transformation is for normalizing skewed distributions. It compresses large values and expands smaller ones, making the data more suitable for algorithms.
Feature engineering
- Techniques like Principal Component Analysis or Singular Value Decomposition reduce the dimensionality of the dataset by identifying the most informative features.
- Combining multiple related features into a single component within data science in cybersecurity helps capture higher-level information and simplify the analysis process.
- If the data includes temporal information, techniques like lagging, differencing, or rolling window statistics can be applied to capture patterns and trends over time.
Data encoding
- One-hot encoding converts categorical variables into binary vectors, representing each category as a separate feature. It enables the inclusion of categorical data in machine learning models.
- Label encoding by data science in cybersecurity assigns unique numerical labels to each category in a categorical variable. It allows for incorporating categorical data into algorithms that require numerical inputs.
Feature selection
- Statistical tests, such as the chi-square test or analysis of variance (ANOVA), identify features with significant discriminatory power and select them for further research due to data science in cybersecurity.
- Correlation analysis helps identify highly correlated features. Removing redundant or highly correlated features can simplify the dataset and reduce noise in the study.

Data Science in Cybersecurity vs. Traditional Guardians
Traditional approaches to anomaly detection are the experienced guardians relying on their knowledge and predefined rules to identify anomalies, while data science approaches equip the guardians with advanced tools and techniques: machine learning algorithms, statistical analysis, and anomaly detection models.
Traditional statistical methods
Traditional statistical methods have long been used to identify deviations from expected patterns in anomaly detection.
Mean and variance
- The mean, or average, is a fundamental statistical measure that calculates the central tendency of a dataset. Anomalies can be detected by identifying data points significantly differing from the mean. A threshold, defined as a certain number of standard deviations from the norm, determines what is considered anomalous.
- Variance measures the spread or dispersion of data around the mean. Anomalies may exhibit high variance, indicating significant deviations from the expected behavior. Comparing data points to the variance threshold can help identify outliers.
Z-score
Z-Score, a standard score, is a statistical measure that quantifies how many standard deviations a data point is away from the mean. It allows for comparing data points across different datasets or features. Anomalies can be identified by detecting data points with z-scores beyond a certain threshold set at a specific number of standard deviations.
Clustering
Clustering techniques — k-means or density-based clustering — group similar data points together based on their features or proximity. Anomalies are detected as data points that do not belong to any cluster or fall into small clusters. Clustering-based methods rely on the assumption that anomalies are significantly different from normal data points.
Distribution-based methods
Distribution-based methods assume that standard data follows a specific statistical distribution, such as Gaussian (normal) distribution. Anomalies can be detected by measuring how well a data point fits the assumed distribution. Deviations from the expected distribution (extraordinarily high or low probabilities) indicate anomalous behavior.
Time-series analysis
Time-series analysis techniques are applied when data is collected over time. Statistical methods — autoregressive integrated moving average (ARIMA) or exponential smoothing — are used to model the expected behavior of the time series. Anomalies are identified by detecting data points significantly deviating from predicted values.
Limitations hinder the effectiveness
- Reliance on predefined rules and signatures
- Difficulty in capturing complex anomalies
- Limited adaptability
- Struggle to handle large and dynamic data
- Imbalanced datasets
- Lack of contextual understanding
- Limited feature representation
Shift from static methods to adaptive approaches
The transition from traditional to data-driven approaches shifts from rule-based methods to leveraging machine learning and data science techniques for anomaly detection. It entails embracing a data-centric approach, where large volumes of diverse data are collected, analyzed, and used to train adaptive models. This transition enables more accurate and dynamic anomaly detection, contextual understanding, and the ability to adapt to evolving threats in the cybersecurity landscape.
Machine Learning Anomaly Detection — Guardians Recognizing Intruders
Like guardians recognizing unusual activities, machine learning techniques identify anomalies by detecting patterns, behaviors, or events that deviate significantly from the established baseline. These deviations are flagged as potential threats by data science in cybersecurity.
Using labeled data to train models for anomaly detection
Supervised learning algorithms learn from labeled data to build a model that predicts the class label of new, unseen data instances, allowing them to identify anomalies effectively with data science in cybersecurity.
Decision trees
Decision trees are hierarchical structures that recursively partition the data into subsets based on feature values. Due to data science in cybersecurity, each internal node represents a decision based on a specific feature, and each leaf node corresponds to a class label (normal or anomalous). In anomaly detection, a decision tree is trained using labeled data, where normal instances are assigned the majority class label, and anomalies are given the minority class label.
Support vector machines (SVM)
SVM is a robust supervised learning algorithm of data science in cybersecurity used for classification tasks, including anomaly detection. It aims to find a hyperplane that best separates standard instances from anomalous ones. The training data consists of labeled cases, with typical data points as positive and anomalies as negative samples. SVM finds the hyperplane that maximizes the margin between the two classes while minimizing the classification errors.
Unsupervised learning is a machine-learning approach
This data science in cybersecurity approach detects anomalies without relying on labeled data. Instead, it forces clustering, density estimation, and novelty detection algorithms to identify patterns or data instances that deviate significantly from most data.
Clustering algorithms
Clustering algorithms group data instances based on their similarity in the feature space. Similar data instances are assigned to the same cluster, while dissimilar cases are placed in different clusters. Anomalies are often detected as data points that do not belong to any well-defined set or form their collection. These isolated or sparse data points are considered different from most of the data, making them potential anomalies in data science in cybersecurity.
Density estimation
Density estimation techniques model the distribution of the data points in the feature space. In order of data science in cybersecurity, they estimate the density or likelihood of observing a data instance in a specific region of the feature space. Anomalies are detected as instances located in areas with low density or where the observed density deviates from the based on the model. Data points with low probability under the estimated density function are considered anomalies.
Novelty detection algorithms
Novelty detection algorithms are designed explicitly by data science in cybersecurity to identify novel or unseen data instances in a dataset. During training, the algorithm learns the distribution of the majority class (typical models). During testing, it identifies data instances deviating significantly from the known distribution, considering them potential anomalies.
Semi-supervised learning combines both labeled and unlabeled data
Semi-supervised learning leverages the advantages of supervised learning with the limited availability of labeled data while also benefiting from the ability of unsupervised learning to identify novel anomalies within data science in cybersecurity.
One-class SVM
One-class SVM is an extension of the traditional SVM algorithm for binary classification. It is trained due to data science in cybersecurity on normal instances only, as anomalies are assumed to be few and different from the standard data. The One-Class SVM builds a decision boundary that encapsulates the most expected data points. New data points that fall outside are considered anomalies.
Self-organizing maps (SOM)
SOM is an unsupervised learning technique that uses neural networks to map high-dimensional data to a low-dimensional space while preserving the topology and structure of the data.
SOM can be trained on a combination of labeled average data and unlabeled data. The network learns to create by data science in cybersecurity a topological representation of the standard data, and new data points that do not fit into the learned topology are identified as anomalies.
Transductive support vector machines (TSVM)
TSVM is a semi-supervised SVM extension that considers labeled and unlabeled data during training in data science in cybersecurity. In anomaly detection, TSVM uses labeled standard data and a small set of unlabeled data to create a decision boundary that separates normal data from anomalies.
Generative adversarial networks (GANs)
GANs consist of two neural networks — a generator and a discriminator — that are trained in an adversarial manner. In anomaly detection, GANs generate synthetic standard data by introducing the generator on labeled average data. The discriminator then tries to distinguish between accurate and generated data. Anomalies are identified as data points that the discriminator struggles to classify as real or fake by data science in cybersecurity.
The Guardians Equipped with Advanced Neural Networks
Deep learning in data science in cybersecurity approaches utilizes robust neural networks, resembling the guardians with intricate neural structures, to process and learn from vast amounts of complex data. These networks consist of multiple layers of interconnected neurons, enabling them to capture tough relationships and patterns in the data.

Advanced artificial intelligence algorithms
Deep learning models of data science in cybersecurity are designed to automatically learn and extract complex patterns and representations from data, making them well-suited for handling intricate and dynamic cybersecurity challenges.
Autoencoders are unsupervised learning models that consist of an encoder and a decoder. The encoder compresses the input data into a lower-dimensional representation (encoding), and the decoder reconstructs the data from the encoded picture. During training with data science in cybersecurity, they learn to rebuild normal data instances, capturing their inherent patterns. During testing, if a data instance is reconstructed with significant errors compared to the original input, it is flagged as an anomaly.
Recurrent Neural Networks (RNNs) are a type of deep learning model designed to handle sequential data, where each data point depends on previous ones. RNNs analyze time-series data, network logs, and user behavior sequences. They excel at identifying patterns in dynamic data streams, detecting unusual network traffic patterns, or identifying abnormal user behaviors within data science in cybersecurity.
Flag anomalies in types of data
Autoencoders and GANs are deep learning models that can study complex patterns and representations from data, making them well-suited for detecting anomalies without the need for explicit anomaly labels with data science in cybersecurity.
Deep autoencoders for anomaly detection
- The encoder compresses the input data into a lower-dimensional representation while the decoder reconstructs the data from this encoded representation.
- Autoencoders learn to reconstruct regular data instances during training, capturing their inherent patterns. The model aims to minimize the reconstruction error by data science in cybersecurity.
- In the detection phase, new data instances are passed through the autoencoder, and the reconstruction error is calculated. Instances with significant reconstruction errors compared to the training data are flagged as anomalies.
GANs for anomaly detection
- GANs consist of two neural networks: a generator and a discriminator. The generator generates synthetic data that resembles the actual data distribution, while the discriminator tries to distinguish between accurate and generated data in the conception of data science in cybersecurity.
- During training, the generator produces data that the discriminator cannot easily distinguish from actual data. As a result, the generator becomes skilled at generating data samples that closely resemble the real data distribution.
- In the detection phase, the generator generates synthetic data based on natural distribution. Due to data science in cybersecurity, anomalies are then identified as data instances that deviate significantly from this artificial distribution.
Combining autoencoders and GANs
This hybrid, often called "AnoGAN" or "GANomaly," is achieved through a two-step process. An autoencoder is trained to reconstruct average data, as described earlier. Next, GANs are employed to learn the distribution of normal data in the latent space, enabling the generation of synthetic standard data. During anomaly detection by data science in cybersecurity, new data instances are encoded using the trained autoencoder, and their reconstruction errors are calculated.
Additionally, these encoded representations are compared to the distribution of average data learned by the GAN. Data instances with high reconstruction errors and representations deviating significantly from the GAN-learned distribution are identified as anomalies.
Benefits of deep learning techniques in anomaly detection
They include the ability to automatically learn intricate patterns from complex data, adapt to evolving threats, and provide high accuracy in detecting anomalies without the need for explicit labels. However, data science in cybersecurity challenges include the need for large amounts of labeled training data, potential overfitting on noisy or unrepresentative data, and the difficulty of interpreting the inner workings of deep learning models.
Collaboration of Guardians by Data Science in Cybersecurity
Hybrid approaches and ensemble methods can be illustrated as a collaboration of specialized guardians working together, each bringing their unique skills and expertise to ensure the cybersecurity fortress remains protected from a wide range of threats.
Building a proactive defense
Combining multiple anomaly detection techniques is a strategy used to enhance the accuracy and robustness of anomaly detection systems.
- Different anomaly detection techniques have unique perspectives and capabilities. Some methods may excel at detecting simple anomalies, while others are adept at identifying complex or subtle deviations.
- Each technique captures different aspects of the data and may focus on distinct features or patterns. The system benefits from complementary information, reducing false positives and negatives by integrating their outputs in order of data science in cybersecurity.
- Cybersecurity threats are continually evolving, and new attack vectors emerge regularly. Combining multiple techniques helps build a robust system that adapts to changes in the threat landscape.
- Combining techniques for data science in cybersecurity enables thresholding and weighting mechanisms to calibrate the contribution of each method. The system assigns higher weights to more reliable techniques and lower weights to less reliable ones.
- Combining multiple techniques involves ensemble methods: voting classifiers, stacking, or boosting. These methods aggregate the outputs of individual processes and make collective decisions based on their votes or consensus.
- Combining techniques mitigates the risk of overfitting, a common issue in anomaly detection. Due to data science in cybersecurity, if an individual method overfits a specific subset of the data, combining diverse techniques helps balance the overall model.
- Some techniques may be computationally expensive or require extensive data for training. Companies achieve a balance between accuracy and resource efficiency, tailoring the solution to their specific needs by combining methods.
Ensemble methods with data science in cybersecurity
Ensemble methods are machine learning techniques that combine multiple individual models to make collective decisions, and they have proven to be effective in cybersecurity anomaly detection.
Bagging
Bagging, short for Bootstrap Aggregating, means training multiple instances of the same base model on different subsets of the training data, randomly sampled with replacement. These data science in cybersecurity models are taught independently, and their outputs are combined by averaging (in regression) or voting (in classification). Bagging is applied to traditional machine learning algorithms or even deep learning models. It reduces the variance and improves the overall robustness of the anomaly detection system.
Boosting
Boosting is an iterative ensemble method that focuses on correcting the errors made by individual models. It trains a sequence of models, where each subsequent model gives more importance to the misclassified instances from the previous model. It enhances the performance of weak classifiers (decision trees or shallow neural networks) by sequentially adjusting their weights with data science in cybersecurity. It pays more attention to challenging data points, improving the detection of rare and subtle anomalies.
Stacking
Stacking, also known as meta-learning, requires training multiple diverse base models, and then a higher-level model (meta-model) is introduced to learn from the outputs of the base models. The meta-model uses the predictions of the base models as its input features and learns how to combine them effectively. Data science in cybersecurity combines the strengths of different anomaly detection techniques: traditional statistical methods, machine learning algorithms, and deep learning models.
Bringing together the strengths
Hybrid models are data science in cybersecurity systems that bring together the strengths of traditional approaches, machine learning, and deep learning techniques to create a comprehensive and robust defense against cyber threats. These models combine various methods to detect anomalies, analyze data, and identify potential security breaches. Hybrid models can better adapt to evolving threats and provide a more accurate and robust defense for organizations.
Testing Guardians’ Skills by Data Science in Cybersecurity
As guardians undergo rigorous training and develop their skills, evaluating an anomaly detection system requires assessing its performance on various datasets and scenarios. The system's ability to detect anomalies accurately, minimize false positives, and adapt to threats is analyzed, ensuring it possesses the necessary skills for effective anomaly detection.
Assessing the performance of anomaly detection systems
Metrics for evaluating the performance of anomaly detection systems provide quantitative measures of how well the system is identifying anomalies and distinguishing them from normal behavior.
- Precision, also known as positive predictive value, measures the proportion of correctly detected anomalies among all instances classified as anomalies by data science in cybersecurity.
- Recall, also known as sensitivity or actual positive rate, measures the proportion of true anomalies correctly detected by the system.
- The F1 score provides a balance between precision and recall and is helpful for data science in cybersecurity when there is an imbalance between the number of anomalies and standard instances in the data.
- The ROC curve is a graphical representation of the trade-off between the valid positive rate (recall) and the false positive rate as the detection threshold of the anomaly detection system varies.
- The AUC-ROC is the area under the ROC curve and provides a single scalar value that quantifies the overall performance of the anomaly detection system within data science in cybersecurity.
- The AUC-PR is the area under the precision-recall curve, which plots precision against recall at various decision thresholds.
- Specificity measures the proportion of actual typical instances correctly identified as normal by the system. Data science in cybersecurity complements the false positive rate and indicates the system's ability to identify true negatives correctly.
Challenges of the evaluation process
Evaluating anomaly detection models within data science in cybersecurity is challenging due to imbalanced datasets and evolving threats.
Imbalanced datasets
Anomaly detection datasets exhibit class imbalance, where the number of standard instances significantly outweighs the number of anomalies. In such cases, models may achieve high accuracy by merely classifying all samples as expected, resulting in misleadingly good performance metrics. Evaluating precision, recall, and F1 score becomes essential to assess the model's true performance in detecting anomalies by data science in cybersecurity.
Rare anomalies
Some anomalies may be infrequent in real-world scenarios, making them difficult to capture in the training data. As a result, the model may not have enough information to identify such rare anomalies during evaluation effectively. Data science in cybersecurity ensures that the model's generalizability to detect novel and rare anomalies is crucial.
Concept drift
Anomaly detection models may be trained on historical data, but data distribution can change over time due to evolving threats or system behavior changes. This concept drift can impact the model's performance with data science in cybersecurity, as it may become less effective in detecting new types of anomalies that emerge after the training data is collected.
Adversarial attacks
In cybersecurity, adversaries may attempt to evade detection by intentionally manipulating data to confuse the anomaly detection model. Adversarial attacks can result in false negatives or false positives, compromising the model's reliability of data science in cybersecurity.
Evaluation time lag
In some cases of data science in cybersecurity, the time lag between data collection and anomaly detection evaluation affects the model's performance. For example, a model may detect an anomaly long after it occurred, reducing its efficacy in timely threat response.
Driving progress in anomaly detection research
Benchmark datasets and competitions provide standardized datasets and evaluation criteria, allowing researchers, data scientists, and cybersecurity professionals to objectively compare and benchmark different anomaly detection methods by data science in cybersecurity.
- Benchmark datasets are carefully curated datasets that contain a mix of regular and anomalous instances representing various real-world cybersecurity threats.
- In benchmark datasets and competitions, specific evaluation metrics with data science in cybersecurity are used to assess the performance of anomaly detection algorithms quantitatively.
- Benchmark datasets and competitions enable an objective comparison between different anomaly detection algorithms.
- Benchmark datasets aim to cover a wide range of scenarios to ensure the generalizability of the evaluated algorithms in order of data science in cybersecurity.
- Benchmark datasets and competitions encourage innovation and advancement in anomaly detection.
Benchmark datasets and competitions contribute to developing more effective and reliable anomaly detection solutions within data science in cybersecurity.

FBI Internet Crime Complaint Center’s Internet Crime Report 2021
Solving the Opposition of Accuracy and Efficiency
From its point of view, the data engineering company DATAFOREST observes that to maintain a balance of accuracy and efficiency for data science in cybersecurity, it is necessary to focus on critical assets and high-risk areas for high accuracy while employing feature selection and dimensionality reduction to improve efficiency. Utilize ensemble methods, optimize model hyperparameters, and adopt incremental learning to balance accuracy and efficiency. Implement real-time processing, adaptive thresholds, and continuous monitoring to adapt to evolving threats and maintain an effective and resource-efficient anomaly detection system.
Maintaining this balance may involve more than just cybersecurity issues. Please fill out the form, and we will tell you more about the benefits of data science.
FAQ
What is anomaly detection in the context of cybersecurity?
Anomaly detection in the context of data science in cybersecurity is identifying unusual or suspicious activities, patterns, or behaviors that deviate from expected norms, which may indicate potential security breaches or cyber threats.
Why is anomaly detection important for identifying security breaches?
Anomaly detection is essential for identifying security breaches because data science in cybersecurity can quickly and accurately detect abnormal activities or patterns that may signify cyberattacks or unauthorized access, enabling timely responses to mitigate potential risks and safeguard critical assets.
What are the common data sources used for anomaly detection in cybersecurity?
Typical data sources for anomaly detection in cybersecurity include network traffic logs, system logs, user activity records, authentication data, and application logs.
How do machine learning techniques enhance anomaly detection in cybersecurity?
Machine learning techniques enhance anomaly detection in data science in cybersecurity by enabling automated pattern recognition and adaptive learning from large-scale data, effectively identifying sophisticated and evolving cyber threats that may be challenging for traditional methods.
What metrics are used to evaluate the performance of anomaly detection systems?
Metrics used to evaluate the performance of anomaly detection systems include precision, recall, F1 score, area under the ROC curve (AUC-ROC), and area under the precision-recall curve (AUC-PR).
How do organizations benchmark and assess the effectiveness of anomaly detection algorithms?
Companies benchmark and assess the effectiveness of anomaly detection algorithms by using standardized datasets and evaluation metrics, participating in competitions, and comparing the performance of different algorithms on representative and diverse cybersecurity scenarios due to data science in cybersecurity.
How are data science and computer science related in terms of cybersecurity?
Data science leverages computer science and data analysis techniques to detect and respond to potential threats effectively. Information technology provides data storage and processing infrastructure, while software engineering is instrumental in developing sophisticated anomaly detection algorithms and security solutions. Problem-solving skills are critical in identifying and mitigating cybersecurity risks, making data science a vital tool in safeguarding digital assets and defending against ever-evolving cyber threats.