Generative AI’s rapid adoption and user-friendly interface signify its potential to democratize technology access. By leveraging expansive neural networks trained on diverse data sets, generative AI surpasses traditional AI models in versatility, enabling various applications from content creation to complex problem-solving. For businesses, the integration of generative AI promises to enhance operational efficiency, foster creativity, and drive competitive advantage, provided CEOs navigate its implementation with strategic foresight and a commitment to continuous learning. As generative AI continues to evolve, it presents a transformative opportunity for organizations to reimagine their operational models, emphasizing the importance of leaders understanding this technology.
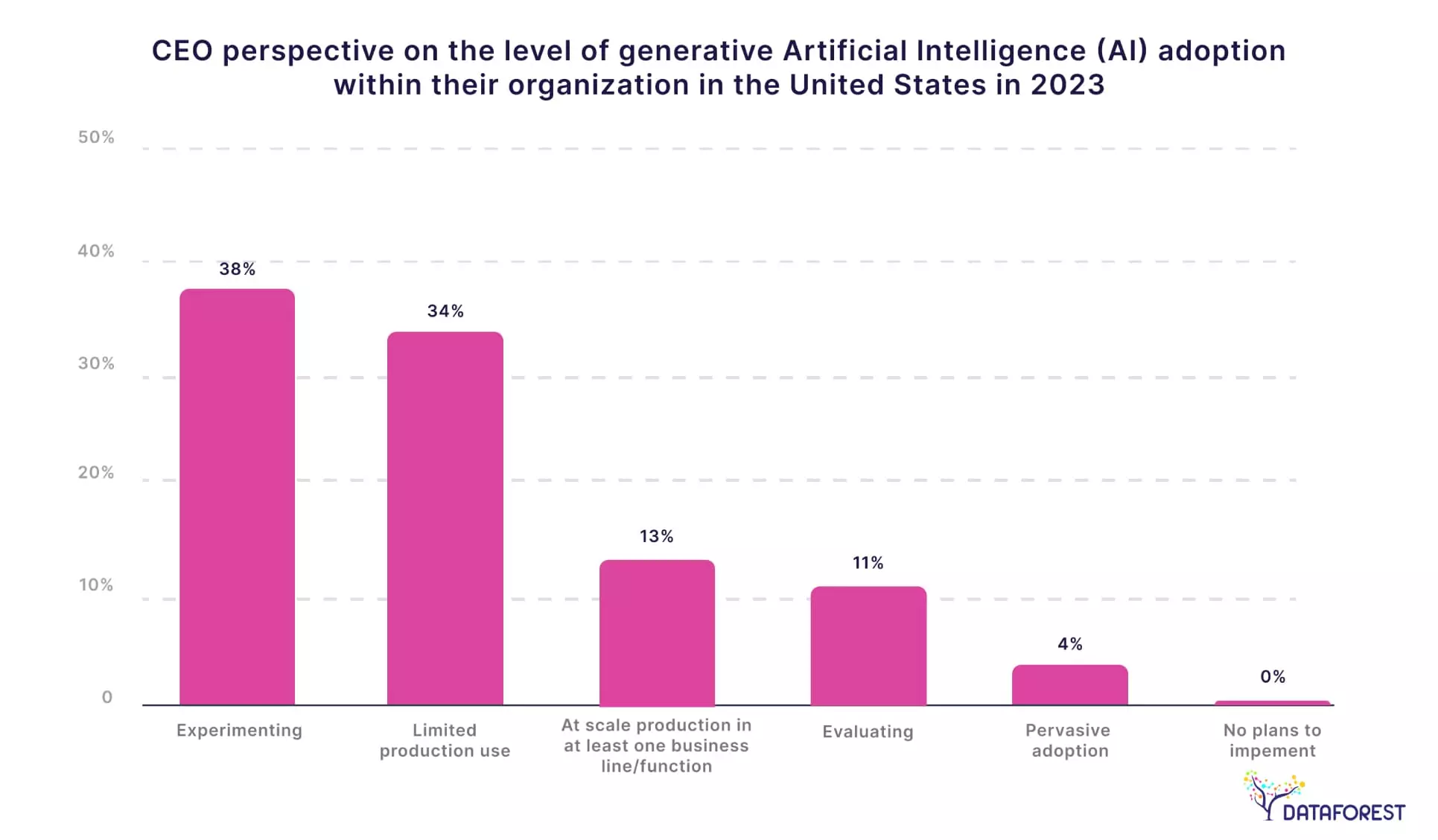
Generative AI is a CEO's Secret Weapon
Generative AI builds upon significant progress in machine learning and artificial intelligence. These ongoing advancements provide a strong foundation for generative AI to develop and improve. Generative AI isn't meant to replace human workers entirely; it augments them. Its usefulness will become increasingly apparent as it integrates seamlessly into existing workflows.
Generative AI thrives on large amounts of data, and the ever-growing volume of data available will fuel its capabilities. Challenges like data privacy and bias are being addressed. As responsible AI development practices are implemented, generative AI can be used ethically and effectively.
Generative AI: Competitive Edge
Faster Time-to-Market: Generative AI automates product development stages—design and prototyping. This allows for quicker iteration and refinement, getting products to market faster.
Enhanced Innovation: AI assists in brainstorming new ideas, generating creative concepts, and creating simulations to test them. This leads to a constant stream of novel products and services.
Operational Efficiency: Generative AI also automates repetitive tasks, optimizes workflows, and identifies process bottlenecks. This frees up human employees for higher-level work and reduces costs.
Data-Driven Decisions: Generative AI analyzes vast amounts of data to uncover hidden patterns and generate valuable insights. Businesses make data-driven decisions that are more likely to be successful.
Personalization at Scale: Generative AI personalizes experiences for individual customers at a massive scale. This can include tailoring marketing messages or customizing product recommendations.
5 Ways Generative AI Revolutionizes Leading Companies
- Content Personalization with Generative AI
The marketing giant Adobe utilizes generative AI to personalize content for its massive user base. Their system analyzes user data and preferences to generate personalized headlines, product descriptions, and blog posts. This allows Adobe to deliver highly relevant content to each user, increasing engagement and conversions.
- Salesforce's Einstein Generative Text
Salesforce is a customer relationship management leader That has implemented generative AI through its "Einstein" platform. Einstein Generative Text helps salespeople by automatically generating personalized email drafts, meeting summaries, and social media posts.
- Generative AI for Financial Reporting
The Associated Press (AP) has partnered with generative AI company Automated Insights to produce quarterly earnings reports. AI analyzes vast financial data sets and generates clear, concise reports in a fraction of the time it would take a human writer. This allows the AP to publish breaking financial news more quickly.
- Generative AI for Oilfield Optimization
Shell, a major oil and gas company, employs generative AI to optimize oilfield operations. The AI analyzes seismic data and production history to predict oil reservoir behavior and recommend optimal drilling locations. This increases efficiency and minimizes environmental impact.
- Generative AI in Video Game Development
Ubisoft, a gaming giant, uses generative AI to streamline video game development. Their AI generates realistic environments, textures, and character dialogue. This allows for faster development cycles and richer in-game experiences.
Generative AI: The Next Wave of Business Innovation
Generative AI tools will become more accessible and user-friendly, allowing smaller companies and startups to leverage its power. AI-powered design platforms will generate prototypes based on user input or AI systems that brainstorm marketing campaigns tailored to specific audiences. This will level the playing field and foster a new era of democratized innovation.
It will enable businesses to personalize experiences for individual customers on an unprecedented scale. AI systems will create custom product recommendations in real time, adjust marketing messages based on customer sentiment, or personalize learning materials for educational institutions. This hyper-personalization will lead to deeper customer engagement.
AI will act as a powerful co-creator, assisting with brainstorming ideas, generating creative text formats like scripts or poems, or composing musical pieces. This will free up human workers to focus on higher-level strategic thinking and creative problem-solving.
The ability of generative AI to analyze vast datasets and identify hidden patterns will change scientific research and discovery. AI systems can design new materials with previously unknown properties, accelerate drug discovery by simulating molecular interactions, or generate hypotheses for scientific experiments.
As generative AI interacts with the natural world through its creations, it will learn continuously. AI systems analyze customer responses to personalized marketing campaigns and use that data to refine future campaigns. This will guide to more practical applications of generative AI over time.
Generative AI in Business
Generative AI automates repetitive tasks like data entry, report generation, and content creation. Generative AI uncovers hidden patterns and generates insights when vast amounts of data need analysis. It also personalizes marketing messages, product recommendations, or learning materials. From design prototyping to content generation, it accelerates product development cycles, bringing products to market quickly.
Generative AI models rely heavily on data. The AI's outputs will also be unreliable if data is scarce, unreliable, or biased. It struggles with tasks requiring empathy and cultural sensitivity. Currently, human customer service representatives are irreplaceable. AI outputs' explainability and ethical considerations become crucial in regulated sectors. If a task can be easily automated with existing tools, the cost and complexity of implementing generative AI might outweigh the benefits. However, the implementation requires investment in technology and data infrastructure.
Consider your business's specific needs and challenges. If the task involves large datasets, repetitive work, or personalization, generative AI could help. However, exploring other solutions for tasks requiring human touch, emotional intelligence, or working with limited data might be wise.
CEOs in Generative AI Opportunities
Here's a roadmap for CEOs to identify opportunities for generative AI in their business model:
- Identify areas in current operations that are inefficient, repetitive, or lack creativity. Generative AI excels at automating repetitive tasks, optimizing workflows, and assisting with creative brainstorming.
- Are there areas where your business has a wealth of data but struggles to extract insights? Generative AI analyzes large datasets and uncovers hidden patterns, leading to valuable business intelligence.
- Consider touchpoints where customer experience can be personalized. Generative AI personalizes marketing messages and product recommendations or tailors content based on customer preferences.
- Stay caught up in the hype. Link generative AI adoption to your overarching business goals, such as increasing market share, improving customer satisfaction, or launching new products faster.
- Research how your competitors are using generative AI. Understanding competitor strategies can highlight potential opportunities for your own business.
- Gather a team from different departments (marketing, operations, product development) to brainstorm potential applications of generative AI. This diverse perspective sparks innovative ideas.
- Only try to overhaul some things at a time. Choose a specific pain point and implement a pilot project using generative AI. This allows us to test its effectiveness and measure the impact before scaling up.
Keep yourself updated on the latest advancements in generative AI. Attend industry conferences and consult with AI experts. Ensure your data practices are ethical and your AI outputs are fair and unbiased.
Integrating Generative AI in 10 Steps
- Start by pinpointing areas in your current workflows that are inefficient. Generative AI excels at automating repetitive tasks, optimizing workflows, and assisting with content creation.
- Generative AI thrives on data. Identify existing datasets relevant to your chosen use case. Consider data collection strategies or explore alternative AI models if data is insufficient.
- Numerous generative AI tools cater to different needs. Explore platforms that align with your use case (e.g., text generation, image creation, code development).
- Consider the tool's scalability to accommodate future growth and user-friendliness for seamless integration with your existing systems.
- Ensure the data you feed into the AI model is clean, accurate, and unbiased. Preprocessing steps like removing errors and formatting inconsistencies are necessary.
- Depending on the chosen tool, you might need to set up APIs (Application Programming Interfaces) to connect the AI model to your existing workflows or develop user interfaces for easy interaction with the AI.
- Integrate the generative AI model into your existing workflows as smoothly as possible. This might involve training employees on using the new technology and developing instructions for incorporating AI outputs into current processes.
- Thoroughly test the generative AI model within your workflow. Evaluate the quality and accuracy of its outputs and identify any potential biases that need correction.
- Continuously monitor the generative AI model's performance. Track key metrics relevant to your use case (e.g., efficiency gains, customer satisfaction) to assess its impact.
- Based on your monitoring and user feedback, refine the model and adjust your workflows as needed. New data and feedback can continuously improve generative AI models.
Building vs. Buying Generative AI
The world of Generative AI is abuzz with potential, offering capabilities to reform creative endeavors, streamline processes, and unlock innovation. However, a question arises for CEOs seeking to harness this power: build or buy? Here's a breakdown of the two main approaches: in-house development and strategic partnerships.
Combining in-house development for core functionalities with strategic partnerships for specific needs is an effective strategy. This allows you to leverage external expertise while maintaining control over critical aspects of your AI solution.
Ethics for CEOs in the Generative Age
Generative AI is a powerful tool that makes ethical considerations paramount. Picture an AI generating social media content that perpetuates misinformation. Or, consider the potential for bias in AI-driven hiring algorithms, inadvertently disadvantaging specific demographics. These are just a few examples of how, without proper safeguards, generative AI conducts in unintended consequences, tarnishing your brand image.
Beyond the ethical considerations lie genuine business risks. Regulatory landscapes evolve rapidly to address the potential misuse of AI. Failure to comply with these regulations results in reputational damage. AI models trained on biased data can perpetuate those biases in their outputs. This guides discriminatory practices and legal challenges.
By proactively considering ethical implications and mitigating risks, CEOs ensure their company responsibly leverages the immense potential of generative AI.
Embed Ethics in the AI Development Process: Integrate ethical considerations to ensure fairness in your AI models.
Diversity in Training Data: Use diverse and representative datasets to train your AI, minimizing the risk of bias in its outputs.
Human Oversight: Maintain human oversight throughout the AI development and deployment process, ensuring responsible use of the technology.
Transparency and Explainability: Strive for transparency in how your AI generates outputs, fostering trust with consumers and regulators.
By taking these steps, CEOs ensure their company stays ahead of the curve while safeguarding its reputation and future success.
Addressing Ethical Concerns in Generative AI
The foundation of responsible AI lies in using diverse and representative datasets to train generative models. This ensures that AI doesn't perpetuate existing societal biases. Techniques like data augmentation can help create more inclusive training sets. Scrutinize your AI algorithms for potential biases. Fair machine learning frameworks and testing identify bias in the AI's decision-making processes. Maintain a human-in-the-loop approach, where humans oversee the training and deployment of generative AI.
When training AI models, prioritize data anonymization techniques to protect the privacy of individuals whose data is used. This helps prevent individuals from being identified by the AI's outputs. Be transparent about how generative AI is used and how user data is handled. Give users control over their data and how it's incorporated into the AI's training process. Implement differential privacy techniques to add noise to data while preserving its utility for AI training. This protects individual privacy while allowing the AI to learn effectively.
Generative AI can be misused to create realistic but fabricated content. Developing robust detection methods for deepfakes and fostering media literacy are crucial steps in combating the spread of misinformation. Automation powered by AI steers to job losses in specific sectors. Strategies like reskilling initiatives and upskilling programs adapt workers to the changing landscape. Develop frameworks for holding developers and users accountable for the misuse of generative AI. Strive for explainable AI models with a clear rationale behind the AI's outputs.
Best Practices for Responsible Generative AI
With the Generative AI's great power comes great responsibility.
Data is King
The foundation of responsible AI lies in using representative datasets. This mitigates bias by ensuring that AI doesn't amplify societal inequalities based on race or gender. Techniques like data augmentation can help create more inclusive training sets. Track the origin of your data and establish transparent data governance practices. This ensures responsible data collection, storage, and usage throughout the AI's lifecycle. Integrate privacy considerations from the very beginning of the AI development process. Anonymize data when possible and give users control over their data and how it's used to train the AI.
Algorithmic Scrutiny and Fairness
Regularly assess your AI algorithms for potential biases. Maintain a human-in-the-loop approach. Humans should oversee the training and deployment of generative AI, ensuring fairness and preventing biased outputs. Strive for explainable AI models with clear and understandable rationale behind the AI's outputs. This fosters trust and allows for human intervention if necessary.
Transparency and User Trust
Be transparent about how generative AI is used and how it impacts users. Explain the technology in an accessible language. Give users control over their data and how it's incorporated into the AI's training process. Allow users to opt out of having their data used or request its removal from datasets. Develop robust detection methods for deepfakes and foster media literacy among users. This helps combat the spread of misinformation generated by malicious actors.
Societal Considerations
Recognize the potential for job displacement due to AI automation. Develop strategies to help workers adapt to the changing landscape. Establish frameworks for holding developers and users accountable for the misuse of generative AI. Advocate for responsible AI regulations and best practices within your industry. View AI as a tool to augment human creativity and problem-solving abilities, not replace them. Focus on fostering a collaborative relationship between humans and AI for optimal results.
Considerations for Responsible Generative AI
The legal and regulatory framework surrounding generative AI is constantly evolving, reflecting the dynamic nature of technological advancements. Notably, in March 2024, the European Parliament took a significant step forward by approving the Artificial Intelligence Act. This legislation prioritizes safety and compliance with fundamental rights and aims to foster innovation. Through this exploration, we delve into the implications of this landmark decision, understanding its significance and the challenges it addresses. But some key areas demand close attention.
Who owns the creative content generated by AI? Current copyright laws might not adequately address AI-generated works. Determining ownership and appropriate licensing becomes crucial. Generative AI often relies on vast amounts of data. Regulations like GDPR (General Data Protection Regulation) and CCPA (California Consumer Privacy Act) dictate how user data is collected, stored, and used.
AI outputs can perpetuate biases present in the training data. Regulations might be implemented to ensure fairness and prevent discriminatory practices by AI models. The ability of generative AI to create realistic but fabricated content raises concerns about the spread of misinformation. Laws and detection methods might be needed to address the potential misuse of this technology.
Who is liable if an AI-generated output causes harm? Determining liability for developers, users, and companies deploying generative AI models is also a complex issue requiring legal frameworks.
SEOs must closely monitor the evolving legal and regulatory landscapes surrounding AI. Seek legal counsel to ensure your use of generative AI complies with current regulations. Document the development process of your generative AI model. This transparency helps demonstrate responsible development practices if legal issues arise.
Try for explainable AI models where the rationale behind the AI's outputs is clear and support efforts to develop comprehensive regulations for generative AI. Industry-wide collaboration shapes legal frameworks that promote responsible use. By proactively addressing these legal and regulatory considerations, CEOs can ensure their use of generative AI is innovative and legally sound.
Return on Investment (ROI) in Generative AI
ROI in Generative AI encompasses a broader range of benefits than cost savings. It includes efficiency, productivity, innovation, and customer satisfaction, ultimately leading to revenue growth. Measuring ROI in Generative AI heavily relies on data analytics. Tracking changes in efficiency, output, customer engagement, and market share quantifies the value derived from the technology. The actual return on investment from Generative AI might not be immediately apparent. It's often a long-term play, with benefits accumulating over time as the technology integrates into workflows and drives innovation. Some benefits of Generative AI, like enhanced creativity or improved customer experience, are intangible and difficult to quantify directly. However, their impact on brand reputation and employee morale is undeniable. As the technology matures and new applications emerge, the ways we measure its return on investment will need to adapt to the evolving value proposition.
Measuring Generative AI's Impact on Business Performance
Generative AI promises a future brimming with efficiency. But quantifying its impact on a business performance requires a multifaceted approach beyond traditional financial metrics.
Understanding the Nuances
Generative AI boosts efficiency and unlocks new revenue streams. A holistic approach is critical to capturing the whole picture. The actual return on investment from generative AI might not be immediate. Benefits often accrue over time as the technology integrates into workflows and fuels innovation. Track long-term trends to understand their cumulative impact. Some benefits, like enhanced creativity or improved customer experience, are difficult to quantify directly. Look for proxy metrics or conduct customer surveys to gauge their indirect impact on business success.
Metrics for Success
- Financial Metrics
Thanks to generative AI, cost reductions can be tracked from automation, process optimization, or reduced labor needs. Measure the impact of AI-driven marketing, personalized experiences, or innovative product development on revenue growth.
- Efficiency and Productivity Metrics
Quantify the time saved by automating tasks, reducing errors, and streamlining workflows with generative AI. Track the rise in production or the ability to handle more complex tasks due to AI-powered assistance.
- Customer Satisfaction Metrics
Monitor surveys, reviews, and social media sentiment to gauge customer satisfaction with AI-powered interactions or experiences. Follow website traffic, conversion rates, and customer retention metrics to understand how AI influences customer behavior.
Choosing the Right Tools
Data Analytics Platforms: Leverage data analytics tools to track key metrics, identify trends, and measure the impact of generative AI on various performance indicators.
A/B Testing: Conduct A/B tests to compare the performance of traditional methods with AI-powered approaches. This isolates the specific contribution of generative AI to improvements.
Control Groups: Establish control groups that don't utilize generative AI to compare their performance with those using the technology. This helps isolate the impact of AI on specific metrics.
Cost-benefit analysis Tools: Use them specifically designed for AI projects. These tools factor in development costs, ongoing maintenance, and potential cost savings from efficiency gains to provide a more comprehensive picture of ROI.
Scenario Modeling: Forecast the potential impact of generative AI on future business performance. This explores different adoption strategies and estimates the expected ROI under various conditions.
Additional Recommendations
The metrics you choose will depend on your specific use case and goals for generative AI. Align measurement strategy with the desired outcomes. The quality of your data is paramount. Ensure data collection methods are accurate to generate reliable insights. Don't treat measurement as a one-time exercise. Continuously monitor performance metrics and adopt an approach based on the collected data.
Cultivating Growth for Maximum Impact
Generative AI holds immense potential to change how businesses operate. However, nurturing a fledgling AI initiative into a full-fledged success story requires a strategic approach to scaling.
Start with a well-defined proof of concept (POC) demonstrating generative AI's value proposition for a specific use case. It establishes a strong foundation and secures buy-in from stakeholders. Then, ensure you have access to high-quality data relevant to a chosen use case. Assemble a team with technical expertise in AI development, data science, and machine learning. Alternatively, consider partnering with a reputable AI vendor for specific needs.
Scaling with Strategy
Don't try to do everything at once. Break down your AI implementation into manageable phases, starting with a single use case and gradually expanding as you gain experience and confidence. Develop a standardized infrastructure for deploying and managing your generative AI models. This ensures consistency and scalability and simplifies the integration of new AI applications. Design your AI models with modularity in mind. This allows for easier customization and adaptation to new use cases.
Amplifying Impact
Integrate your generative AI models seamlessly with existing business systems and workflows. This fosters adoption and maximizes AI's impact across the organization. Invest in upskilling your workforce to understand and leverage generative AI's capabilities. This empowers employees to collaborate effectively. Develop a comprehensive change management strategy to address potential resistance or concerns surrounding AI adoption. Effective communication and training ensure user buy-in and a smooth transition.
Continuous Improvement
Track key metrics, identify areas for improvement, and embrace an iterative development approach. Refine AI models based on real-world data and user feedback to enhance their effectiveness over time. Plan for future scalability from the outset. Consider factors like infrastructure needs, data storage requirements, and potential growth in AI applications.
Case Studies Showcasing ROI from Generative AI Investments
Generative AI is evolving from a futuristic concept to a tangible business driver. Here are case studies demonstrating the return on investment (ROI) companies achieve with generative AI.
Personalized Marketing with AI-Generated Content
Adobe needed to create personalized marketing content at scale to effectively engage individual customers. They implemented a generative AI system that creates personalized product recommendations, email content, and social media posts based on individual user data and preferences. They reported a significant increase in click-through rates (CTRs) on marketing campaigns powered by AI-generated content. They also observed improved customer engagement and higher conversion rates.
Drug Discovery Acceleration with Generative Molecules
The traditional drug discovery process is time-consuming and expensive. GenerativeTensor, a biotech company, utilizes generative AI to design novel drug molecules with specific properties. This approach significantly reduces the time and resources required to identify promising drug candidates. GenerativeTensor has partnered with pharmaceutical companies, potentially leading to faster development of life-saving medications.
AI-Powered Fashion Design and Material Optimization
The fashion industry faces challenges in design innovation and optimizing material usage for sustainability. Material Driven leverages generative AI to create new garment designs and patterns while optimizing fabric usage to minimize waste. This fosters design innovation and reduces material costs and environmental impact. Material Driven has partnered with leading fashion brands, showcasing the potential of generative AI for a more sustainable fashion industry.
Generative AI for Faster News Content Production
News organizations struggle to keep pace with the growing demand for real-time content. The Washington Post uses generative AI to automate the creation of certain types of news content, like sports summaries and financial reports. This frees up journalists to focus on more in-depth and investigative reporting. It allows the newspaper to maintain a high content volume while improving the quality of its core journalism.
AI-Generated Video Ads for Personalized Customer Experiences
Creating personalized video ads for a large customer base can be daunting. X-axis, a marketing technology company, utilizes generative AI to create personalized video ads that target specific audiences with relevant messaging and visuals. AI-generated personalized video ads increased customer engagement and conversion rates for X-axis clients. This demonstrates the power of generative AI in crafting targeted marketing campaigns that resonate with individual customers.

Generative AI as a Service
So, should a CEO tackle Generative AI as a service implementation or outsource it? He can build an in-house team of AI specialists. This gives them more control but requires significant investment in talent and infrastructure. Partnering with a vendor such as DATAFOREST offers a quicker and potentially more cost-effective solution. The vendor handles the technical complexities, but the CEO gives up some control. The best choice depends on your company's resources and expertise. Building your own team might be ideal for maximum control if you have the in-house talent and resources. However, for most businesses, partnering with a Generative AI as a service vendor offers a faster and more cost-effective entry point. Please fill out the form and find out which case is yours.
FAQ
What are the first steps to incorporating generative AI into my business?
First, identify repetitive tasks or areas lacking creative spark. Then, assess your existing data to see if it's relevant and plentiful enough for generative AI. Finally, research available generative AI tools that align with your needs, like content creation or data analysis.
What are the common challenges in implementing generative AI, and how can they be overcome?
A common hurdle is ensuring data quality—biased or insufficient data leads to unreliable AI outputs. This can be addressed by gathering diverse data sets and implementing techniques to mitigate bias. Tasks requiring human empathy or facing strict regulations might not be ideal for generative AI. Focusing on repetitive tasks or personalization can help navigate these limitations.
How can I ensure the ethical use of generative AI in my company?
To ensure ethical generative AI use, prioritize diverse datasets to minimize output bias. Maintain human oversight throughout development and deployment to prevent unintended consequences. Be transparent about how generative AI is used within your company to build user trust.
What are the potential ROI and business outcomes of adopting generative AI?
Generative AI can boost efficiency by automating tasks, leading to cost savings and increased output. It can also personalize customer experiences, potentially raising satisfaction and sales. While some benefits, like improved creativity, are more challenging to quantify, generative AI has the potential to transform businesses by streamlining processes and fostering innovation.
How does generative AI integrate with existing data and technology infrastructure?
Generative AI thrives on data, so existing data sets relevant to your use case are essential. The AI model can be connected to your workflows through APIs (Application Programming Interfaces), allowing it to process data and generate outputs. Integration should be smooth, involving employee training and adjustments to existing processes.
What skills or partnerships are necessary to leverage generative AI effectively?
While deep technical knowledge isn't always required, understanding generative AI's capabilities and limitations is crucial. Partnering with AI experts can bridge this gap and ensure effective model selection and training. Fostering a culture of data literacy within your company empowers employees to prepare data and interpret AI outputs for optimal results.
How can generative AI be used to enhance customer experiences?
Generative AI personalizes the customer journey by tailoring recommendations, content, or product designs based on individual preferences. This fosters deeper engagement and satisfaction. AI chatbots powered by generative AI provide more natural and informative customer service interactions, improving brand perception.