A small custom furniture manufacturer faced skyrocketing costs and lengthy lead times due to the complex nature of translating client ideas into tangible designs. Traditional methods, relying on sketches and physical mockups, were slow and prone to misinterpretations. However, with Multimodal Generative AI, clients can now describe their dream furniture or share inspirational images, and the AI instantly generates realistic 3D models. This streamlined the design process, eliminating costly misunderstandings and reducing production time. The AI's ability to quickly iterate on designs based on client feedback meant that each piece was perfectly tailored. Multimodal Generative AI became the linchpin, improving the process and changing the business model. If you think this is your case, then arrange a call.

Multimodal Generative AI — The AI That Does It All
Multimodal Generative AI is a type of Artificial Intelligence that processes and creates content across multiple data types, or "modalities." It juggles words, pictures, sounds, and videos all at once. This AI isn't just about creating content; it's about understanding it too. It identifies the connection between a picture and a caption or how a song makes you feel.
Happy Customers: Imagine chatbots that get you or virtual shopping assistants showing you products and answering your questions like a friend. That's the personalized experience Multimodal Generative AI creates.
Content Creation: Multimodal Generative AI pumps out high-quality content like nobody's business, from blog posts to product descriptions to eye-catching visuals.
Product Development: Need a new product idea? Multimodal Generative AI helps you brainstorm, prototype, and get real-time customer feedback. It's a supercharged R&D team.
Smarter Decisions, Better Results: By crunching data from all sorts of sources, Multimodal Generative AI uncovers hidden insights about customers, markets, and operations. This means you can make decisions backed by data, not guesswork.
Think this is all hype? Think again. Companies like OpenAI, Meta, and Google already use this tech to create mind-blowing stuff. We're talking Multimodal Generative AI that generates realistic images from text descriptions, creates scratch-made videos, and composes original music.
Complete Multimodal Generative AI Understanding
Multimodal Generative AI leverages powerful algorithms, like transformers, to ingest and understand various data types—think text, images, audio, and video. This allows it to generate creative content in those formats and understand their relationships.
Multimodal Generative AI: The Building Blocks Behind the Magic
Multimodal Generative AI relies on key components to weave together different data types.
- Data Modalities: These are the different forms of informtion the Multimodal Generative AI understands and generates. The most common ones include:
- Text: This can be anything from words and sentences to articles or codes.
- Images: Photos, illustrations, abstract patterns – the Multimodal Generative AI process and generate all of these.
- Audio: Think speech, music, sound effects, raw audio signals.
- Video: This combines images and audio, adding another layer of complexity and potential for creativity.
- Other modalities: Multimodal AI isn't limited to just these. It can also work with data like 3D models, sensor readings, or biological signals.
- Feature Extraction: The Multimodal Generative AI must extract the relevant features from each data type to understand its meaning. An image might identify objects, colors, and textures, while it would look at words, grammar, and sentiment in text.
- Modality Integration: The Multimodal Generative AI combines the extracted features from different modalities, creating a unified data representation. This allows it to understand the relationships, like how a picture relates to a caption or how a song matches a scene in a movie.
- Generative Model: Once the Multimodal Generative AI has a good grasp of the data, it uses this understanding to generate new content. It can create images from text descriptions, write stories based on a series of pictures, or compose music that matches the mood of a video.
The Modality Integration
Integrating different modalities within Multimodal Generative AI is like how our brains combine sensory input to understand the world around us.
Feature Encoding: Each modality is first processed independently to extract meaningful features. For text, this might involve converting words into numerical representations (embeddings) that capture their meaning and context. Images could be broken down into pixel values or higher-level features like shapes and objects. Audio might be represented as waveforms or spectrograms.
Common Representation Space: The Multimodal Generative AI then maps these disparate features into a shared "latent space," a universal language where information from different modalities can be compared and combined. Think of it as translating different languages into a single language.
Attention Mechanisms: The multimodal generative AI employs attention mechanisms to understand how different modalities relate. These mechanisms allow the model to focus on specific parts of the input data most relevant to the task. For example, when generating a caption for an image, the model might pay more attention to the objects in the foreground and their relationship to each other.
Joint Modeling: The Multimodal Generative AI then processes the integrated features from the joint representation space to generate new content. This could involve predicting the next word in a sentence based on an accompanying image, generating music that matches the mood of a video, or creating a realistic image from a textual description.
Refinement and Optimization: The Multimodal Generative AI model is continuously trained and refined using large datasets of multimodal data. This helps it better understand the relationships between different modalities and generate more accurate and creative outputs.
How Does Multimodal Generative AI Integrate Different Modalities
Multimodal Generative AI is a rapidly growing field with several vital frameworks and models pushing the boundaries of what's possible.
Transformer-based Models
Initially developed for natural language processing (NLP), transformers have become the backbone of many multimodal generative AI models. Their ability to capture long-range dependencies and contextual relationships within data makes them ideal for handling multiple modalities.
GPT-4: This model, developed by OpenAI, excels at generating text in response to various prompts, including images and text. It writes code, answers questions, and generates creative content.
DALL-E 3: OpenAI also generates high-quality images from textual descriptions, making it a powerful tool for artists, designers, and marketers.
Flamingo: Developed by DeepMind, Flamingo combines text and images for tasks like visual question answering and image captioning. It performs impressively in understanding complex visual scenes and generating descriptive text.
Kosmos-1: This Microsoft model is designed to understand and respond to various types of input – images, video, and audio. It has potential applications in robotics, autonomous vehicles, and virtual assistants.
Generative Adversarial Networks (GANs)
GANs are another popular framework used for multimodal generation. They consist of two neural networks: a generator that creates new content and a discriminator that distinguishes between real and generated data. This adversarial training process pushes the generator to create increasingly realistic, high-quality outputs.
StyleGAN2: This model, developed by NVIDIA, is known for generating incredibly realistic images of human faces, landscapes, and objects.
GauGAN2: Another NVIDIA creation, GauGAN2 allows users to create photorealistic images by sketching a scene and labeling objects.
VQGAN-CLIP: This model combines GANs with CLIP (Contrastive Language-Image Pre-training), enabling it to generate images based on textual descriptions and make it a creative expression tool.
Multimodal Generative AI Technical Architecture
- Encoder: An encoder extracts features and represents them in numerical format for each modality. This could be a text encoder like BERT or an image encoder like ResNet.
- Fusion Module: This component combines the features from different modalities, often using attention mechanisms to weigh the importance of each modality for the task at hand.
- Decoder: The decoder takes the fused features and generates the desired output, text, images, audio, or video. The type of decoder depends on the desired output format.
Supercharging Your Business with Multimodal Generative AI
Multimodal Generative AI isn't just a fancy term. But what's the hype all about? Let's break down the incredible benefits that make this tech a must-have.
Taking Your Business to the Next Level
Multimodal Generative AI makes super-accurate predictions and decisions using different types of data. It analyzes information from different sources, like text, images, and videos, and finds connections that a single-modality AI might miss. Multimodal Generative AI understands how a tweet about a product launch relates to an image of the product and a video review from a customer. The AI uncovers patterns and insights that traditional methods would find impossible by analyzing multiple modalities. Multimodal Generative AI gives a more holistic view of your data, leading to more informed decisions.
Multimodal Generative AI: Real-World Examples
New Virtual Dressing Room: Some online shops use Multimodal Generative AI to let you "try on" clothes virtually. You upload a pic, and the AI shows you what different outfits would look like on you. It's like a personal stylist who knows your taste and helps you find clothes you'll love. Plus, it means less hassle with returns and more confidence in your purchases.
The Search for New Medicines: Developing new drugs is like finding a needle in a haystack. But Multimodal Generative AI gives scientists a powerful magnet. Analyzing tons of data on molecules, research papers, and clinical trials, it discovers promising new drug candidates faster than traditional methods. This means life-saving treatments could be in our hands sooner rather than later.
Tech Support: Some companies use super-smart Multimodal Generative AI assistants that answer your questions through text, voice, or pictures. Got a wonky Wi-Fi connection? Snap a pic of your router, and the Multimodal Generative AI might tell you how to fix it quickly.
The Challenges of Multimodal Generative AI
Multimodal Generative AI holds immense potential to transform businesses, but it's not without its challenges. These obstacles, ranging from data integration to ethical considerations, can hinder the successful implementation of this powerful technology. However, by understanding these challenges and employing innovative solutions, businesses can unlock the full potential of Multimodal Generative AI and reap its many rewards.
Select what you need and schedule a call.
Beyond Words and Pictures: What's Next for the Multimodal Generative AI?
More Than Meets the Eye (and Ear): Currently, most AI models focus on text and images, but that's just scratching the surface. Soon, they'll be able to understand and create more data types, like 3D models, spatial maps, and even stuff like your heartbeat or brainwaves. Imagine Multimodal Generative AI creates virtual worlds you feel or personalized health advice based on your body's signals.
Your AI Bestie: The next generation of Multimodal AI will be your assistant, understanding your preferences, habits, and mood. It'll recommend stuff you like, create content tailored to your taste, and anticipate your needs before you know you have them.
AI with a Conscience: As AI gets more powerful, it's essential to ensure it's used for good. Multimodal Generative AI's future is about transparency, fairness, and making sure it's not biased. We want AI that helps us, not hurts us.
AI for Everyone: Soon, creating and using Multimodal Generative AI will no longer be reserved for tech whizzes. Tools and platforms will become more accessible and spark creativity and problem-solving. It'll be a superpower in your pocket.
AI Meets the Metaverse: Get ready for a wild ride as Multimodal Generative AI teams up with other cutting-edge tech, such as virtual and augmented reality. We're talking AI-powered virtual worlds that feel authentic, immersive training simulations, and maybe a decentralized internet where creators have more control.
Supercharged Creativity: Multimodal Generative AI isn't here to replace us; it's here to be more creative. Imagine artists, writers, and musicians teaming up with AI to generate mind-blowing ideas and create stuff that's never been seen before.
The possibilities of Multimodal Generative AI are endless, and we're just getting started. It's an exciting time to be alive, and with AI by our side, the future looks brighter than ever.
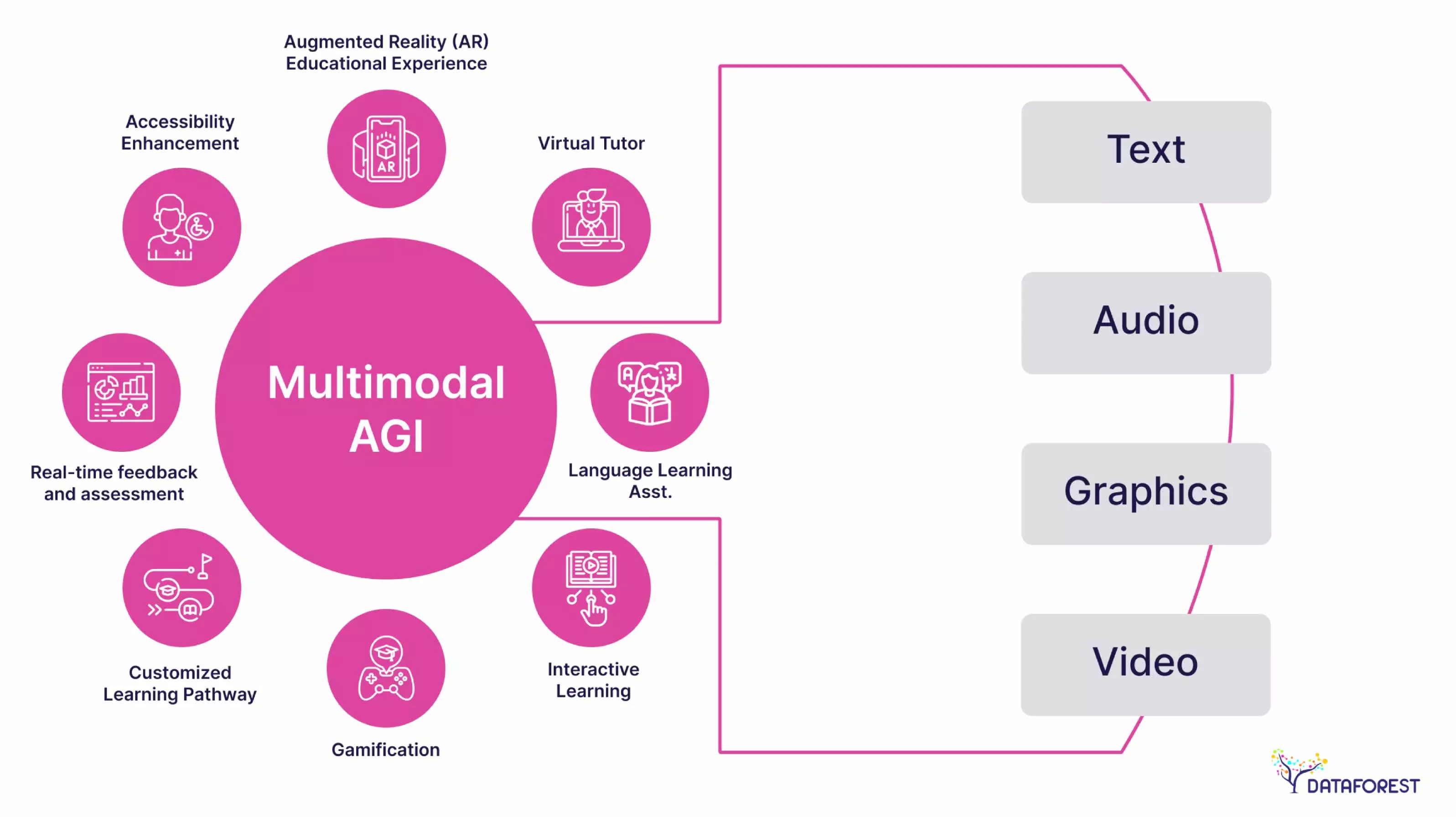
Concerns for Businesses When Adopting Multimodal Generative AI
Businesses often approach tech providers like DATAFOREST with a mix of excitement about implementing multimodal generative AI. They're thrilled about the potential for personalized customer experiences, automated content creation, and faster product development. However, they also worry about the complexity of integrating different data types, the high computational costs, and the potential for bias or inaccuracies in AI-generated outputs. They also need reassurance about data security and privacy and guidance on utilizing this powerful technology to achieve business goals. It's a balancing act of harnessing innovation while mitigating risks, and finding the right tech partner is crucial for success. Please fill out the form – together, it will be easier for us to maintain this balance.
FAQ
What is Multimodal Generative AI?
Multimodal Generative AI is a type of artificial intelligence that can understand and create content using multiple types of data, such as text, images, audio, and video. This means it can not only write a poem but also generate an accompanying image or a piece of music that complements the poem's mood. This ability to work with diverse forms of data makes it a powerful tool for creativity, communication, and problem-solving in various fields.
How does data fusion work in Multimodal Generative AI?
Multimodal Generative AI uses data fusion to combine information from different modalities, such as text, images, and audio, into a unified representation. This involves extracting meaningful features from each modality and mapping them into a shared space where their relationships can be understood. This unified representation allows the AI model to generate new content combining or reflecting elements from multiple modalities, such as creating an image from a textual description.
What are the main applications of Multimodal Generative AI?
Multimodal Generative AI changes numerous industries with its diverse applications. It can analyze medical images and patient records in healthcare to assist with diagnoses and personalized treatment plans. In marketing, it can generate tailored advertisements and product descriptions that resonate with individual consumers. In the creative arts, this technology can compose music, generate artwork, and craft immersive virtual reality experiences.
What are the challenges in implementing Multimodal Generative AI?
Implementing Multimodal Generative AI faces several challenges, primarily due to the complexity of integrating diverse data types. Ensuring the quality and consistency of generated content across modalities is difficult. Gathering and annotating large datasets that capture the intricate relationships between different modalities is a resource-intensive task. Ethical concerns like potential biases in the training data and the misuse of generated content also pose challenges for responsible implementation.
What ethical considerations are associated with Multimodal Generative AI?
The ethical implications of Multimodal Generative AI are complex and multifaceted. One primary concern is the potential for misuse, as AI-generated content can be used to spread misinformation, create deepfakes, or manipulate public opinion. Biases in training data can lead to discriminatory or unfair outputs, perpetuating societal inequalities. There's also the question of intellectual property rights, as AI-generated content raises questions about ownership and attribution.
Compare Multimodal AI vs Generative AI.
Generative AI creates new content. On the other hand, multimodal AI can process and understand multiple types of data, such as text and images combined. Consider Generative AI as an artist who can create a beautiful painting, while Multimodal AI is a critic who can understand and explain why the painting is beautiful, referencing its visual elements and the artist's written description.
What are the most famous examples of Generative AI?
OpenAI's DALL-E 3 is renowned for generating images from text descriptions and turning creative prompts into visual masterpieces. ChatGPT, also from OpenAI, engages in conversations and generates human-like text for tasks like writing emails, poems, or code. Google's Bard, a competitor to ChatGPT, is also gaining traction for its ability to generate creative text formats and translate languages.