Aleksandr Sheremeta, Co-Founder and Managing Partner at DATAFOREST, hosted a session “Future is here: Most important IT Instruments for growing your business” at the Business & Tech Summit 2025.
Aleksandr is a digital transformation expert with 17 years of experience in data science. For the last 9 years, he has worked as a Managing Partner at DATAFOREST, a company that helps companies use AI to automate business and create new tech products.
His previous background includes 12 years in risk management and IT, where he held management positions at UniCredit, Erste, Sberbank, and the National Bank of Ukraine.
In this article, we gathered key insights from his session on artificial intelligence and its impact on modern business processes.
Top 15 Tech Trends
McKinsey highlighted the top trends in tech and conducted an analysis to understand whether global companies are currently investing in these technologies, doing certain pilots, or already implementing these solutions in their companies.

These trends can be grouped into 5 main categories: AI revolution, digital future, communication and computing, advanced engineering, and sustainable world.
AI starts with data. But why are we seeing such rapid changes now? How did we get to this point? Looking back, everything that is happening today is the result of two key factors: big data and the development of computing power. The progress in these areas has opened up opportunities for businesses.
We are now at a stage that can be called the automation of thinking. LLM models have become the first step towards changing how we work, make decisions, and interact with information. It is important to understand that in the next 5-10 years, we will see the development of new models—those that can perform calculations and process information.
For example, today, Chat GPT or similar models cannot perform calculations. They can only use certain functions or methods that will perform calculations for them. In 5-10 years, we will get models for reasoning and calculations.
This year, Deloitte did research among large companies and identified that 80% believe that AI can improve efficiency. Also, 55% analyze and test GenAI solutions, while 52% believe that this is an opportunity for a new revenue stream for their company, but only 37% have implemented and integrated it into their business processes.

The big difference between what we have today in terms of GenAI and traditional artificial intelligence is data analysis. Today's models understand and interpret the data that we provide to them and, based on this data, they make assumptions and then generate texts, pictures, or code. This inability to create something new, although they are called generative, is the difference between what we have today and what we had in the past. GenAI is a new paradigm of the world, and it is changing the customer experience, how we work, our processes, value proposition, and more.
How is Gen AI changing the customer experience?
Increased productivity
Productivity increases through reducing the routine work that people do. We optimize the backlog of tasks that we have to help our employees do it more easily. At the same time, we change the process of performing the work itself when the employees reduce the administrative and operational burdens. Automation with AI takes employees to a higher level and allows them to create. This way, their knowledge and time are focused on understanding what is happening and how to improve this process or create something new.
For managers, this means less micromanagement. Instead of 10 employees doing some manual work, managers can hire only one person who will control the process of automating tasks and improve it.
And it is not only at the employee level that the change is taking place, but also at the management level. That is, we no longer need to administer our employees, we do not need to spend our precious time organizing the process for many employees, because we can optimize. We do not need 10 employees to perform some monotonous work. We can leave only one person who will monitor this process and improve it.
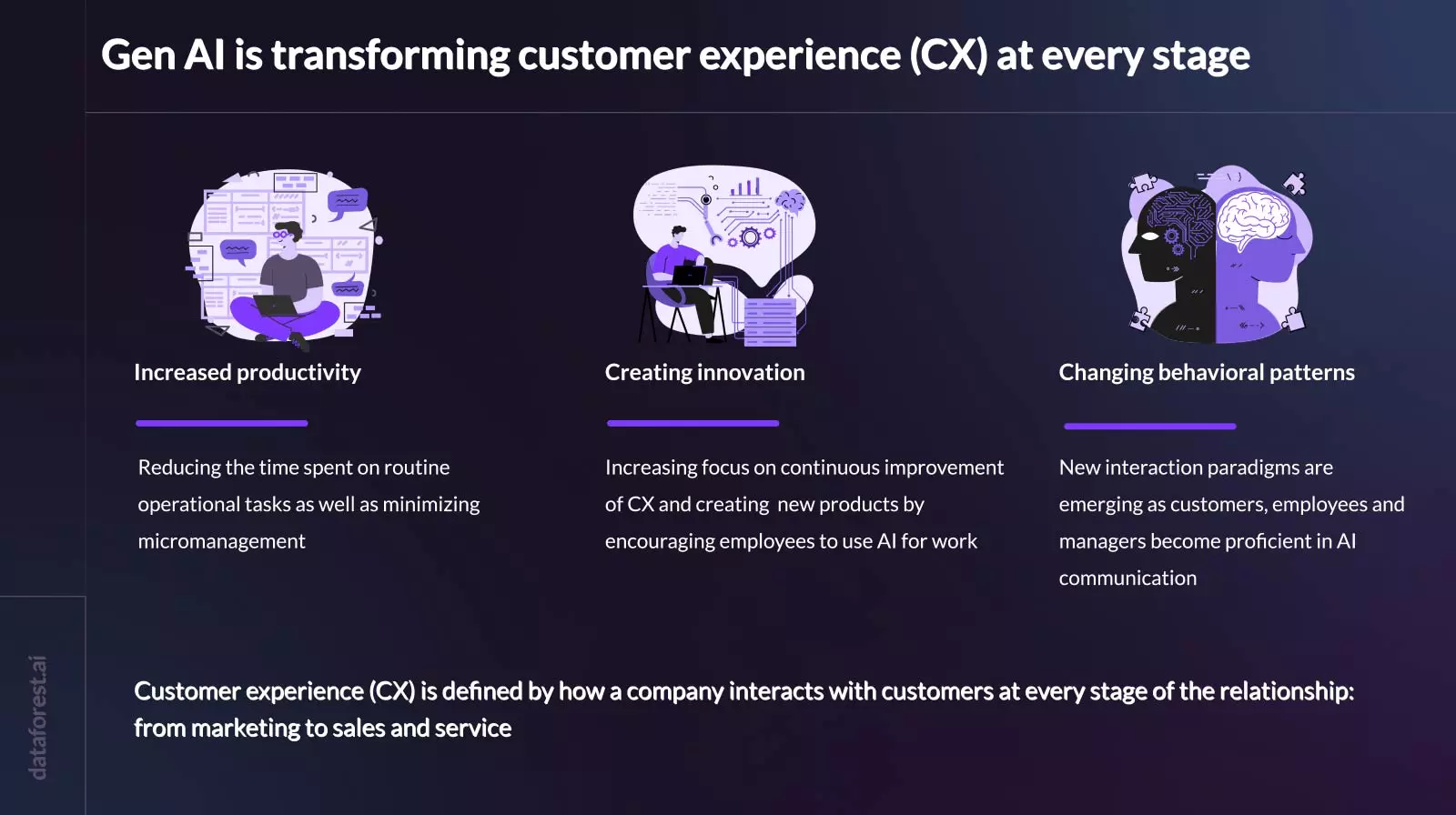
Creating innovation
By automating tasks, we free up time to focus on creating a better customer experience, improving the way we interact with our customers. When moving from increasing productivity to creating innovations, the paradigm of our interaction changes in general.
Changing behavioral patterns
New interaction paradigms emerge as customers, employees, and managers become proficient in AI communication.
Our clients are becoming part of this process, they understand what is being done, who is on the other side, whether it is AI, and what processes are automated. Most clients are okay with it. Of course, at first, there is a certain challenge, but after AI is integrated, we see a positive reaction from the clients. At DATAFOREST, a company that is involved in AI development, we often receive requests for development that were prepared by a person using artificial intelligence. There is a pattern of how AI works, and you can tell that this was prepared using AI. From what we see, these requests are getting better.
How business integrates AI into real processes
Today, we can see the active implementation of artificial intelligence into business processes: from customer interaction analytics to the automation of routine work. And although the idea of creating a “bot that will do everything” sounds great, the reality is much more complicated and requires a gradual process of AI integration. Let’s figure out why.

Many companies are already using AI to:
- analyze communication with customers;
- automatically form and update a knowledge base;
- create AI copilots that help employees in the work process;
- launch AI bots that can directly interact with customers.
It may seem that instead of going through all these steps, you can immediately create a powerful customer bot based on the LLM model and simply transfer all communication to it. However, this approach carries a number of risks.
Models like Chat GPT can confuse facts, inaccurately convey information, or respond differently to the same requests. Then, a completely logical question arises: Are we ready to trust such a tool to communicate with customers?
Often, companies that have gone through the first experiments change their approach: they use AI not directly for customers but as an assistant for employees.
AI needs to be trained. If we want the model to work effectively, it needs to be trained on high-quality content. Here, a new challenge arises: Where can we get this data?
Most companies do have internal knowledge bases: documents, files, videos, and presentations on Google Drive. But how relevant are these materials? When was the last time you updated them? This is what usually remains behind the scenes. It turns out that simply transferring this data to the model is not enough–it either works incorrectly or does not work at all.
We go back to where we started: the basis of any effective implementation of AI is high-quality, structured, and up-to-date data. Without this, the phrase "launching AI for customer service" can only be considered an experiment.
You should pay extra attention to constantly updating the knowledge base, analyzing live communication with customers, and forming an internal system to train AI.
What capabilities are already available for businesses?
Most companies already have AI tools that can be used to collect and analyze knowledge. For example, Zoom automatically generates meeting transcripts and even creates short summaries. These materials are a source of valuable information that can be analyzed both at the management level and at the level of individual employees.
We can determine which communication was effective and which was not. We know the results of the calls: where sales were made, who exactly led the conversation and how. This data can be analyzed to extract real examples of behavior that led to a positive result.
Formation of a knowledge base and its use
If we build a knowledge base with constant analytics, this allows us to create AI assistants for employees. Especially for newcomers, this assistant can speed up the onboarding process and help adapt to corporate communication standards.
One practical way to understand when it’s time to move from a simple copilot solution to a full-fledged automated agent is to give employees the opportunity to rate the usefulness of the answers. A simple metric like “working/not working” allows you to see how ready the system is for launch.
Three approaches to implementing AI in business
Approach 1: AI for employees
This scenario involves using ready-made AI tools. Chat GPT, Midjourney, HeyGen—all of these can be used by employees to help them work more efficiently. This solution does not require complex development.
Approach 2: Quick automation through existing systems
Even a simple IT landscape (Google Sheets, CRM, email, websites) can be automated using LLM and integration platforms like Zapier or Make.com. For example, let’s review a marketing case: a user left their email on the website. We can analyze which page they came from and what interests them, and create a customized email strategy using ChatGPT.
This does not require large investments, but results in a better conversion than classic marketing touches.
We have a detailed article about the top AI tools for business that provides a detailed overview of HeyGen, Zapier, and many others.

Approach 3: Full-fledged AI development
This includes developing complex AI agents. Such agents can integrate into various systems, perform specific actions, and build logical sequences.
For example, an AI agent in a real estate company can:
- Understand the user’s request
- Request additional information (if, for example, the city is missing)
- Analyze the database
- Provide relevant results
Each stage involves using a different agent, but they all interact in a logical chain. If you need to add extra information about an object, another agent is activated, which extracts data from structured or unstructured sources, summarizes it through LLM, and returns it to the user.
How do AI agents work?
Imagine a call center employee. He works according to a script, acts within the framework of clearly defined rules, and does not deviate from the script. This is exactly how AI agents work: they perform the assigned role, but do not go beyond the given context. Without clear instructions, without understanding how to process information, and without a defined format of the result, even the best model will not show the expected effect.
This means that if we want the agent to work effectively, we must not simply give him a task, but provide the full context, the amount of information, the logic of analysis, and the desired result. Exactly how we do it with a new employee during the onboarding period.
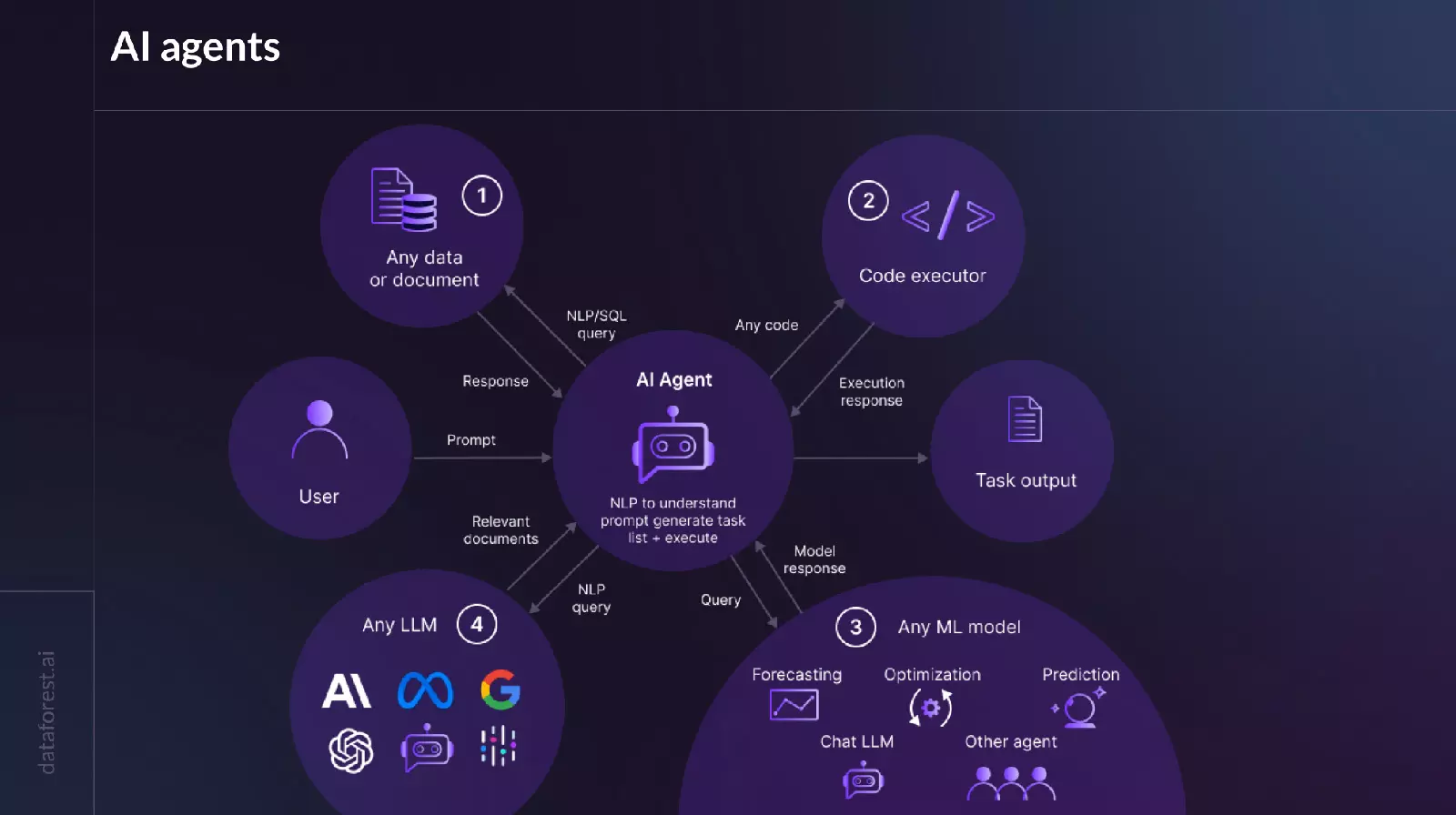
Types of AI agents
We can break down AI agents into certain levels of complexity. There are simple ones that work with databases or separate functions. For example, an agent that searches for relevant articles or performs basic analysis based on a user's query.
There are more complex systems that work with terabytes of data. In such cases, it needs to process large amounts of information, find relevant fragments, assemble them into a logical answer, and do it quickly and automatically.
Computer Vision & Gen AI
Another area that has changed with generative AI is computer vision. Today, systems can analyze human behavior: build routes, measure time spent in rooms, and track staff actions.
While it used to take months or even years to create such a system, now it often takes a month or a month and a half to launch a prototype. This is a huge breakthrough for businesses because computer vision allows you to analyze staff workload, evaluate service speed, identify bottlenecks in the company’s work, and even build new approaches to monetization, such as introducing subscriptions.
How to start implementing AI: a simple step-by-step plan
Implementing artificial intelligence in a company doesn’t have to start with complex technological transformations—you can start with an idea.
1. Collect ideas within your company
Invite managers and key employees for a brainstorming session with a simple task: everyone should offer 5 ideas where AI could be integrated in your company. You will be surprised, but at least 2-3 ideas will be similar, and these are the ones you should start with.
2. Create pilot projects
The selected ideas do not need to be scaled up immediately. Launch several pilots and small projects to test hypotheses. Build PoC or MVP to validate the idea and receive real feedback from users. Consider them as elements of an investment portfolio: not all stocks will take off, but some will generate a high ROI.
3. Analyze performance
After 2-3 months, analyze the results. Which pilot project performed best? Where is the greatest potential for return on investment? Based on this, make decisions about scaling it further.
4. Prepare for scaling
Developing a pilot is easy. Usually, one or two months are enough. But the real challenge is scaling: investing in the product, adapting processes, and integrating with other systems.
5. Change management
Prepare your team for AI integration by explaining that AI does not replace them, but enhances their work, demonstrating a new level of interaction with the product and services, and providing support and training at all stages.
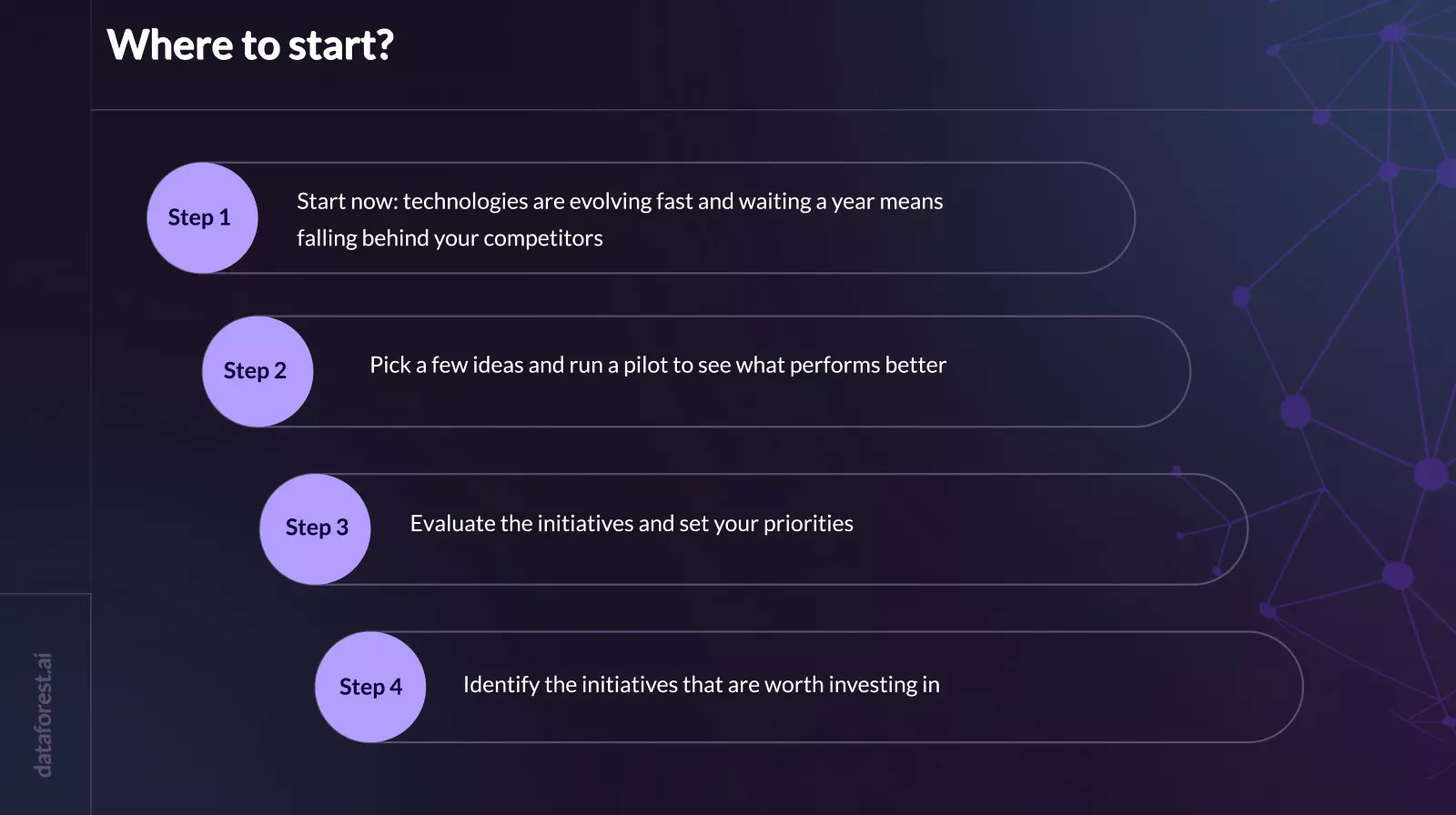
DATAFOREST provides Gen AI consultations to help businesses identify use cases. If you need a tech vendor to guide you from the initial idea to developing and scaling a Gen AI application to maximize revenue growth for your company, book a call with Aleksandr to discuss your future project.