Think up a boat sailing on the open sea, representing the supply chain operations, with data science as the sails. They symbolize the flexibility of data science, while the boat represents the stability needed for successful operations. To navigate effectively, the sailboat balances between adjusting the sails and maintaining stability.
Data Science in Supply Chain Requires a Balance
Companies must utilize the flexibility of data-driven insights to adapt to changes, anticipate market trends, and optimize operations. At the same time, they must maintain stability by adhering to reliable processes, ensuring data accuracy, and aligning with strategic goals. As a skilled sailor combines the art of adjusting sails and strengthening resilience to navigate the open sea, companies must harmonize flexibility and stability in data science for supply chain optimization. Book a call, get advice from DATAFOREST, and move in the right direction.
Supply chain operations for business success
Data science in supply chain operations drives cost efficiency, responsiveness, customer satisfaction, risk mitigation, collaboration, and competitiveness. Firms enhance profitability, market share, and overall performance by optimizing supply chain processes by positioning themselves for long-term success in a dynamic and competitive business landscape.
Data science is an interdisciplinary field
Data science in supply chain combines techniques from statistics, mathematics, computer science, and domain expertise to extract knowledge from data. It means collecting, processing, analyzing, and interpreting large volumes of data to uncover patterns that optimize processes.
- Demand Forecasting
- Inventory Optimization
- Route Optimization
- Supplier Relationship Management
- Predictive Maintenance
- Risk Management
- Continuous Improvement
Data science can potentially revolutionize supply chain operations by leveraging the power of data-driven insights and advanced analytics techniques.
Supply Chain Data Science in Turbulent Waters
The current state of supply chain operations can be likened to sailing through turbulent waters with unpredictable winds. At the same time, the sailboat represents the supply chain, and the rough waters represent the challenges and disruptions supply chain operations have faced recently.
Traditional supply chain limitations
While effective in many cases, the traditional supply chain management approach has several limitations that hinder operational efficiency and responsiveness.
- Lack of visibility limits the ability to monitor inventory levels, track shipments, and identify bottlenecks or delays.
- Siloed decision-making hinders coordination and collaboration across the supply chain, leading to suboptimal decision-making, misalignment of goals, and inefficiencies.
- Traditional supply chain management tends to be reactive. This approach may result in firefighting rather than proactive risk management.
- Data is often fragmented across systems, making integrating and analyzing holistically challenging.
- Traditional supply chain management approaches may lack the flexibility and agility needed to respond quickly to demand, supply, or market conditions changes.
- Traditional approaches to inventory management often rely on rule-of-thumb quantity methods. They may result in excess inventory or stockouts, increasing carrying costs, obsolescence, and customer dissatisfaction.
- Lack of collaboration foils information sharing, coordination, and joint decision-making, limiting the potential for end-to-end supply chain optimization.
Organizations address the limitations of the traditional approach and drive innovation in their supply chain operations by embracing these advancements.
Challenges faced by data science in the supply chain
- Demand volatility
- Supply chain complexity
- Risk management
- Cost optimization
- Technology and data integration
- Sustainability and ethical considerations
- Talent management
- Collaboration and communication
- Continuous improvement
- Regulatory compliance
Informed decision-making
A data-driven approach is crucial in supply chain management as it enables optimized operations and improved forecasting, resulting in enhanced efficiency, reduced costs, and increased customer satisfaction in a dynamic and complex supply chain environment. Data science in the supply chain empowers organizations to force data and analytics to drive resilience, collaboration, and continuous improvement, gaining a competitive advantage in the marketplace.
3 Key Supply Chain Statistics You Should Know
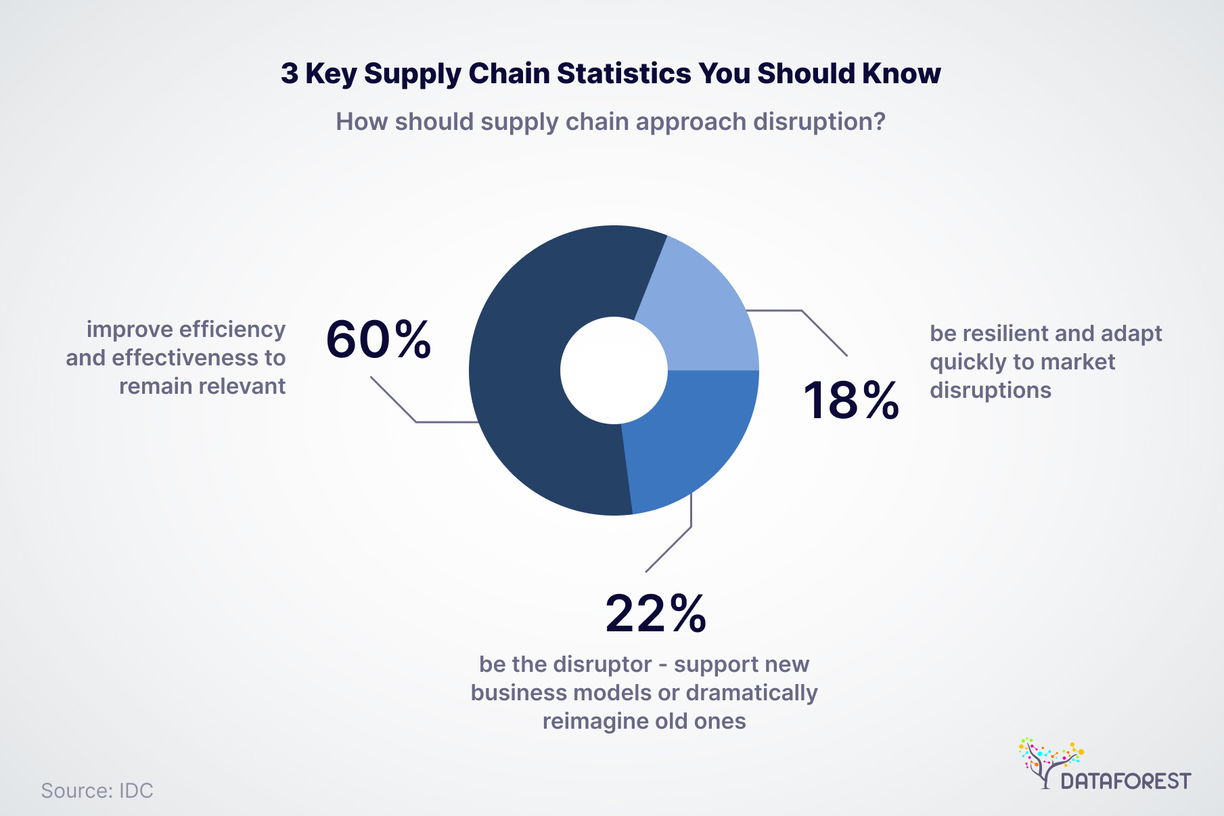

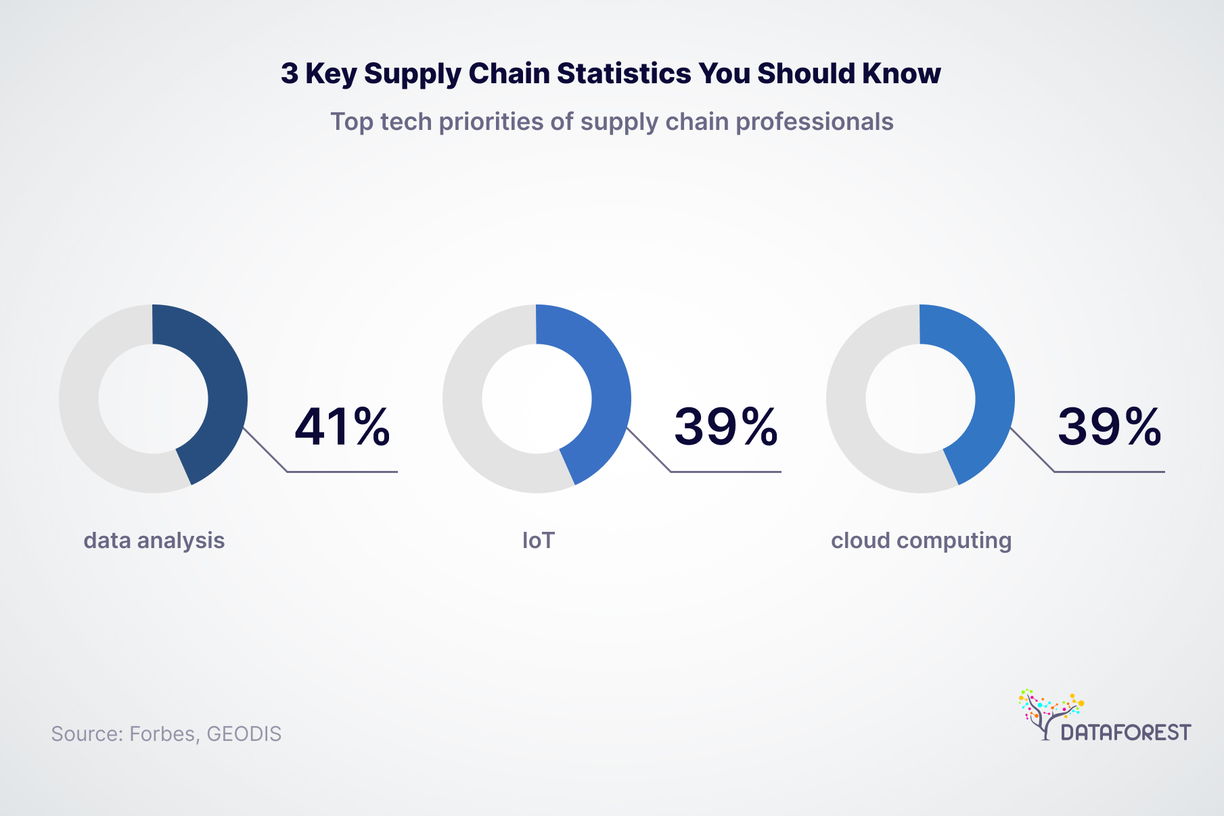
Using Navigation Tools for Data Science in Supply Chain
Leveraging data science in supply chain management uses advanced navigation instruments to chart a course through uncharted waters. The sailboat represents the supply chain, and data science acts as the compass, sextant, and navigational charts, providing valuable insights and guidance.
Advanced analytics from supply chain data science
Interested in the update? Schedule a call and we'll tell you what's what.
Supply chain data science commonly available sources
- Enterprise Resource Planning (ERP) systems
- Point of Sale (POS) systems
- Warehouse Management Systems (WMS)
- Transportation Management Systems (TMS)
- Supplier data
- Internet of Things (IoT) devices
- External data sources
- Customer data
- Financial data
- Government and industry data
Proper data quality with data science in the supply chain
Data quality and governance are of utmost significance in supply chain operations as they ensure accurate, reliable, and consistent data that forms the foundation for informed decision-making, effective planning, and optimized processes. Proper data quality and governance practices enable organizations to mitigate risks, improve operational efficiency, and maintain trust and credibility in their supply chain operations.

Applications of Data Science in Supply Chain
They include accurate demand forecasting to adjust the sail's position based on changing winds, inventory optimization to find the optimal balance for smooth sailing, and route optimization to chart the most efficient course through the open sea. These data-driven applications help to streamline processes, reduce costs, and navigate the complexities of the supply chain more effectively, leading to improved customer satisfaction.
Demand forecasting in supply chain data science
Data science in the supply chain involves analyzing historical sales data, market trends, customer behavior, and external factors to estimate customer orders' expected quantity and timing. Demand forecasting enables one to make informed decisions regarding production planning, inventory management, and resource allocation. Data science techniques — statistical models, time series analysis, machine learning algorithms, and predictive big data analytics — are applied to identify patterns, seasonality, and other factors influencing demand.
Inventory optimization for data science in supply chain
It aims to balance minimizing inventory holding costs and efficiently meeting customer demand. Data science techniques — mathematical modeling, optimization algorithms, and predictive analytics are applied to analyze demand variability, lead times, service level objectives, and cost parameters. Companies optimize reorder points, safety stock levels, and replenishment strategies, resulting in improved inventory management, reduced stockouts, and increased operational efficiency.

Transportation and logistics for data science in supply chain
- Data science techniques are applied to optimize transportation routes and improve logistics efficiency by analyzing data on delivery locations, transportation modes, traffic patterns, and historical performance.
- Data science optimizes load consolidation by analyzing shipment data, order volumes, and capacities. Firms maximize truckload utilization, reduce transportation costs, and minimize the environmental impact associated with space in vehicles with data science in the supply chain.
- Data science enables data-driven carrier selection by analyzing performance metrics, costs, and service levels of carriers. Stakeholders make informed decisions on carrier selection, considering reliability, on-time delivery, and cost-effectiveness.
- Data science techniques optimize costs by analyzing data on freight rates, shipping volumes, and carrier contracts. Organizations negotiate favorable rates, optimize transportation modes, and improve freight cost management.
- Data science is crucial in optimizing last-mile delivery, which refers to the final leg of the supply chain from a distribution center to the end customer. Companies optimize delivery routes and schedule deliveries efficiently.
- Data science enables organizations to monitor and analyze transportation and logistics performance metrics, such as on-time delivery, transit times, and fulfillment rates, by leveraging real-time data and analytics by data science in the supply chain.
- While not exclusively transportation-related, data science in the supply chain also contributes to warehouse optimization. Brands optimize warehouse operations, improve picking and packing processes, and enhance efficiency.

Supplier management for data science in the supply chain
It requires data-driven approaches to evaluate supplier performance, support supplier selection, and qualification, enhance relationships, assess risks, optimize contracts and pricing, foster collaboration and innovation, and ensure compliance with sustainability standards. Companies effectively manage supplier relationships, mitigate risks, and drive value throughout the supply chain by harnessing data-driven insights through data science in the supply chain.
Real-world Examples of Data Science in Supply Chain
Supply chain industry publications like Supply Chain Management Review, Logistics Management, or Harvard Business Review often feature case studies and articles highlighting data science's application in supply chain optimization. It is like a maritime map that shows developed and safe routes.
Successful implementations of data science in supply chain
- Walmart, one of the world's largest retailers, uses advanced analytics and machine learning algorithms to analyze customer data, sales patterns, and inventory levels to optimize replenishment strategies.
- Amazon, a global e-commerce giant, employs predictive analytics and machine learning algorithms to forecast customer demand, optimize warehouse operations, and streamline logistics with data science in the supply chain.
- UPS, a leading logistics and transportation company, applies advanced routing algorithms and real-time data analytics to optimize delivery routes, minimize fuel consumption, and improve overall efficiency.
- Procter & Gamble (P&G), a multinational consumer goods company, works with predictive analytics and machine learning to improve demand forecasting, optimize production planning, and reduce inventory levels due to data science in the supply chain.
- Zara, a renowned fashion retailer, operates real-time sales data, customer feedback, and market trends to rapidly adjust their sail (production and inventory) in response to changing fashion trends.
Benefits of data science in supply chain
- Improved forecasting accuracy
- Enhanced operational efficiency
- Better decision-making
- Increased agility and responsiveness
- Cost reduction and financial savings
- Enhanced customer service and satisfaction
Successful implementations of data science in supply chain optimization have highlighted the importance of quality data, robust analytics capabilities, and collaboration across functions. Companies have learned the value of data governance, data integration, and cross-functional alignment to ensure the success of data science in the supply chain.
Big data in supply chain management
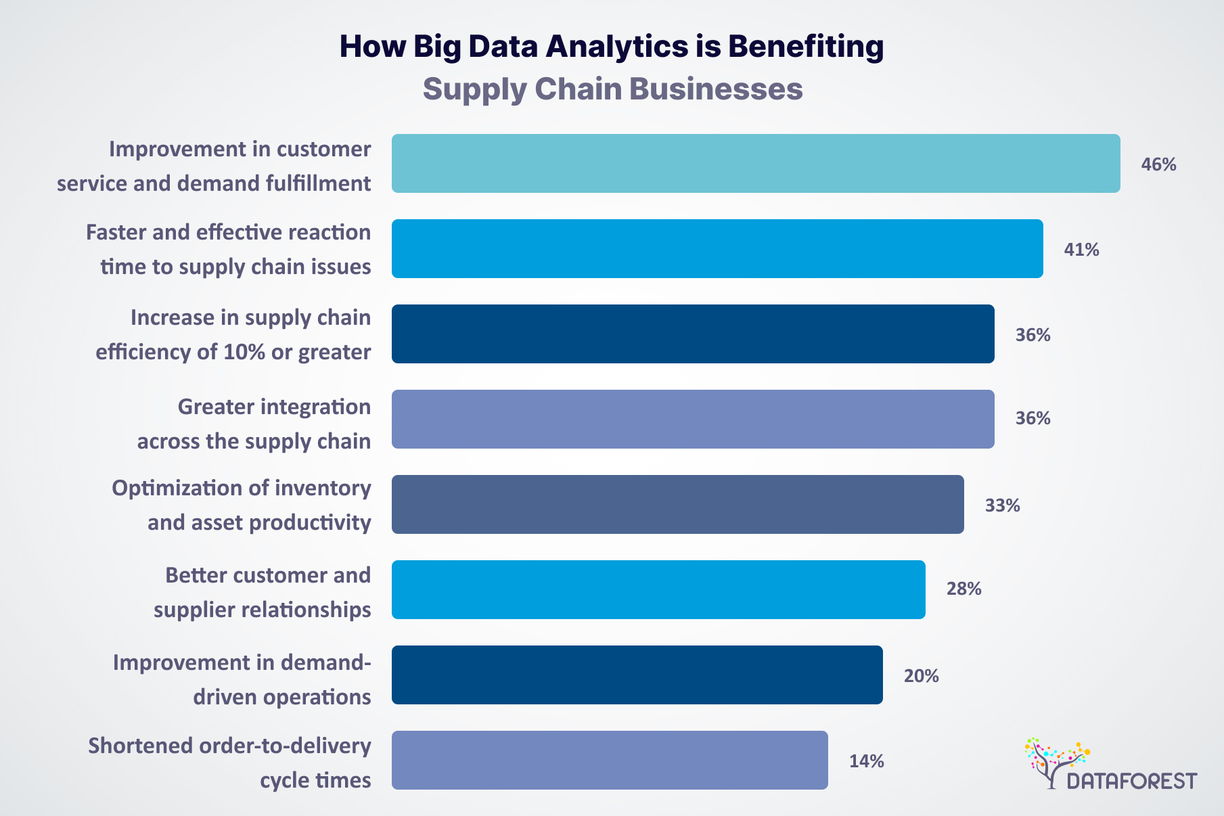
Data Science in Supply Chain: Through Rough Waters
Overcoming challenges and implementing data science in supply chain operations is like navigating a sailboat through rough waters. It requires a combination of skilled sailors (data scientists) who can harness the power of data-driven insights, a sturdy vessel (technology infrastructure) capable of capturing and analyzing large volumes of data, and a well-charted course (strategic planning) that aligns data science initiatives with goals.
Implementation challenges in data science in the supply chain
Fostering a data-driven culture
Fostering a data-driven culture within the company is essential for successfully implementing data science in the supply chain. The key aspects to consider:
- Leadership support
- Education and training
- Collaboration and cross-functional alignment
- Data accessibility and transparency
- Recognition and rewards
- Continuous improvement
- Clear communication
This culture shift enhances the ability to optimize supply chain operations, make informed choices, and effectively adapt to dynamic market conditions.
Potential risks in data science in the supply chain
Ethical considerations of data science in the supply chain revolve around the responsible use of data and its impact on stakeholders: safeguarding customer privacy, ensuring data security, and mitigating biases in algorithms and decision-making processes. Risks include the misuse of data, the potential for algorithmic discrimination, and the unintended consequences of relying solely on data-driven decision-making without human judgment and accountability needed for data science in the supply chain.

Data Science in Supply Chain — Solving the Paradox
As a data engineering company, DATAFOREST states from the height of its experience: solving the flexibility-stability paradox of data science in the supply chain means finding the delicate balance between adaptability and stability in decision-making. It requires forcing data-driven insights to drive flexibility while maintaining the stability of core processes. It can be achieved through agile planning, scenario analysis, and supply chain design incorporating modular and scalable configurations. Continuous monitoring and performance measurement systems help detect deviations and enable proactive adjustments.
If you are still trying to handle it yourself, please fill out the form, and we will contact you to discuss improving your supply chain.
FAQ
What is the role of data science in supply chain optimization?
The role of data science in supply chain optimization is to leverage data-driven insights and advanced analytics techniques to improve operational efficiency, reduce costs, enhance decision-making, and drive overall supply chain performance.
How does data science help improve demand forecasting accuracy in supply chain operations?
Data science improves demand forecasting accuracy in supply chain operations by analyzing historical sales data, market trends, customer behavior, and external factors to identify patterns, trends, and potential demand drivers, enabling more accurate predictions and informed decision-making within data science in the supply chain.
How can data science improve transportation and logistics in the supply chain?
Data science improves transportation and logistics in the supply chain by empowering advanced analytics and real-time data analysis to optimize routes, minimize costs, enhance resource allocation, and improve overall efficiency in the movement of goods in order of data science in the supply chain.
How can organizations overcome resistance to change and foster a data-driven culture in supply chain operations?
Organizations can overcome resistance to change and foster a data-driven culture in supply chain operations by effectively communicating the benefits, providing comprehensive training, and actively involving employees in the data-driven decision-making process to build trust and ownership with data science in the supply chain.
How can data science contribute to sustainability and resilience in supply chain operations?
Data science can contribute to sustainability and resilience in supply chain operations by analyzing data on environmental impact, optimizing resource utilization, and enabling proactive risk management to reduce carbon footprint, enhance efficiency, and ensure continuity in the face of disruptions affordable to data science in the supply chain.
In general, what does data science do in the supply chain?
Data science in the supply chain harnesses business intelligence, creates data products through descriptive analytics, and optimizes raw materials utilization for improved operational efficiency and sustainability.