Imagine you're at the helm of a big fashion retail chain and have big data analytics as your secret weapon. It's like a conversation with your customers without saying a word, tracking every glance they make at those trendy jeans or retro sneakers online and in-store. Your system sends spot-on personalized offers, nailing their style preferences, and they're eating it up. Behind the scenes, it's all about playing chess with your inventory, moving the right pieces, like those suddenly in-vogue vintage tees, to where they're most wanted. If you are interested in this topic, please arrange a call—we will explain everything in detail.
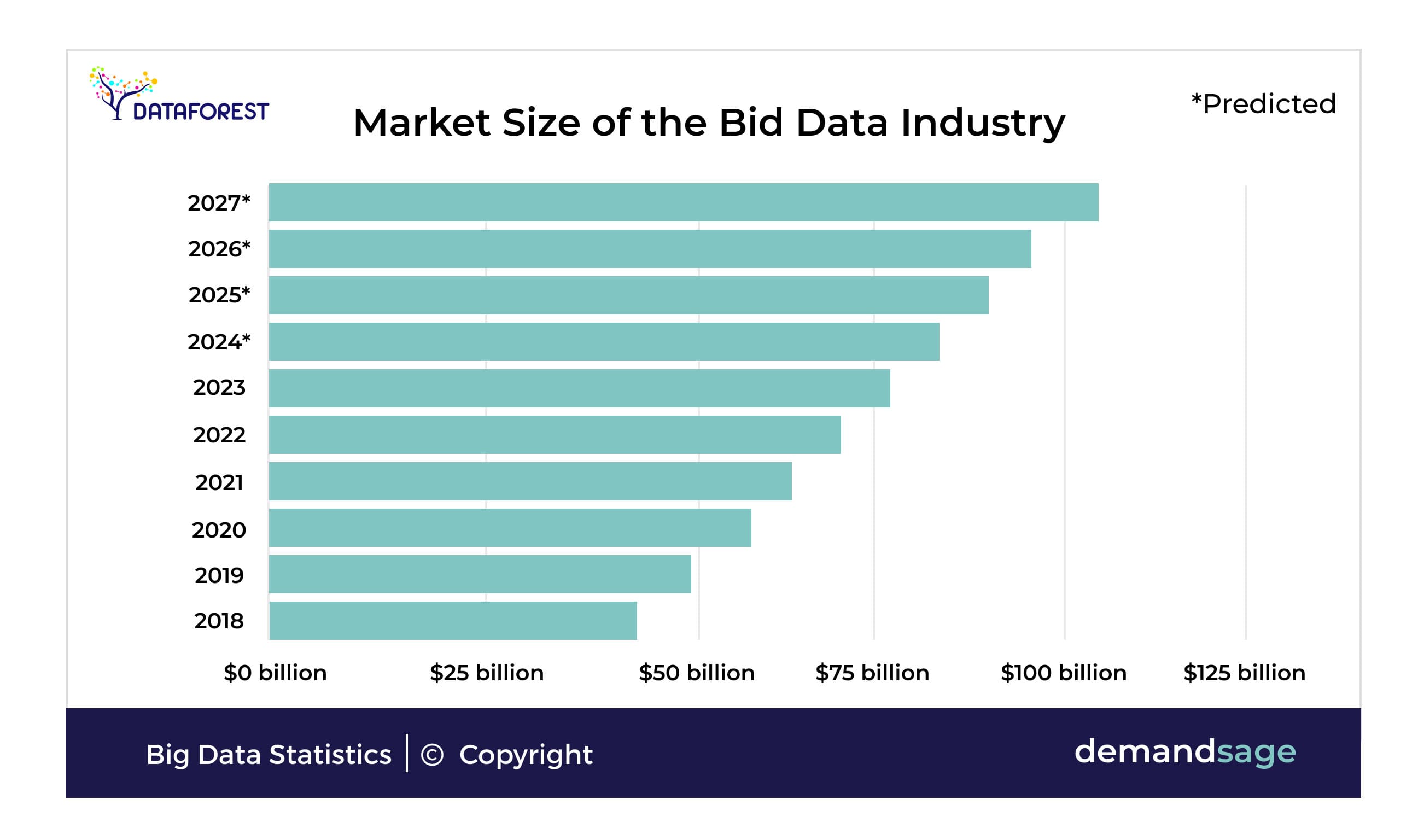
The table displays the big data market size over the past years.
What is Big Data Analytics in Retail?
In the retail sector, big data analytics is diving deep into vast oceans of data — from what customers click online to every item they pick up in stores. This isn't just counting beans; it's about understanding patterns, predicting trends, and getting a 360-degree view of what your customers want, even before they know it themselves. It's about harnessing the power of data from multiple sources: social media buzz, website traffic, in-store interactions, and even external factors like weather or economic shifts.
The Modern Retail Industry Landscape
The retail landscape is a speed freeway, constantly evolving and highly competitive. It's no longer just brick-and-mortar versus online stores; it's a complex web of omnichannel experiences where digital and physical realms intertwine. Consumers are more informed and empowered than ever, with expectations for personalized experiences. Retailers are under pressure to keep up and stay ahead, making sense of consumer behaviors, managing inventories smartly, and tailoring marketing strategies that resonate with diverse market segments.
The Retail Industry in the Global Economy
The retail industry is multi-trillion-dollar, touching everything from mammoth supercenters to quaint corner stores. This industry is a vital cog in the wheel of economies, driving employment, influencing consumer prices, and shaping life quality. It's a barometer of economic well-being, where retail spending shifts signal economic trends. Big data analytics has become the linchpin, empowering retailers to make smarter decisions, stay competitive, and meet the demands of consumers worldwide.
Traditional Methods in Retail
- Retailers relied heavily on sales data collected at the cash register. This process was often manual, with sales associates recording sales in ledgers.
- Understanding customer preferences and experiences was done mainly through physical feedback forms and surveys.
- Retailers conducted market research through direct observation. It meant physically watching customer behaviors and noting which products attracted more attention.
- Decision-making regarding stock levels, pricing, and promotions was primarily based on historical sales data.
- Store associates were crucial in gathering information about customer preferences and complaints.
- Decisions about store expansions, new product lines, and marketing strategies were based on broader economic indicators and fundamental financial analysis.
- Relationships with suppliers and decisions about product sourcing were often based on personal experience and intuition.
- Marketing strategies in traditional retail were more generalized. They were based on broad demographics and used standard channels: print ads, TV commercials, and flyers.
Introduction to Big Data Analytics in Retail
Big data in the retail industry has always been about understanding customers and markets, but big data analytics has changed it, bringing a new depth and precision. Today's retail landscape is about harnessing a deluge of data to make smarter decisions, tailor customer experiences, and drive strategic growth.
Big Data Analytics vs. Traditional Data Analysis in Retail
Big data analysis significantly differs from traditional data analysis in several fundamental ways, fundamentally altering how data is collected, processed, and used for decision-making.
Big data analytics represents a paradigm shift in understanding and utilizing data.
Big Data Analytics for Retailers Is a Dynamic Process
Big data in the retail sector involves an intricate process that spans collecting, processing, and analyzing vast amounts of data from many sources.
Collecting Data
It includes traditional sources like sales records and customer databases and more modern sources: social media interactions, website traffic, customer reviews, and IoT devices in stores. The volume of data collected is massive, often reaching petabytes, and it includes a variety of data types — structured data (sales figures), semi-structured data (web logs), and unstructured data — social media posts and video content.
Processing Data
Given the volume and variety, this data requires storage solutions, often utilizing cloud-based platforms for scalability and flexibility. Technologies like Hadoop and NoSQL databases come into play here, managing large datasets' storage and quick retrieval. Data from various sources must be normalized (formatted consistently) and cleaned (removing inaccuracies or duplicates) to ensure reliable analysis.
Analyzing Data
Retailers use advanced AI analytical tools and techniques for processing this data. This includes machine learning algorithms, predictive analytics, and data mining techniques to extract meaningful patterns and insights. One of the critical aspects of big data analytics in the retail market is the ability to process data in real-time. Big data retailers gain deep insights into customer preferences and behaviors by analyzing this info.
Actionable Insights
It requires optimizing supply chains, tailoring marketing campaigns, designing store layouts based on customer traffic patterns, or developing new products based on customer feedback and trends. The process is cyclical. Insights gained from analytics lead to actions, the results of which are again captured as data, feeding back into the system for continuous improvement and refinement.
Big Data in Retail: The Source of Benefits
The benefit of big data in retail stems from its ability to provide insights into customer behaviors and preferences, enabling personalized marketing and efficient inventory management. Retailers optimize their operations, predict trends, and create tailored shopping experiences by harnessing data from diverse sources.
Big Data Analytics in Retail — Efficient Business Practices
- Enhanced customer personalization
- Improved inventory management
- Effective pricing strategies
- Insightful customer analytics
- Streamlined supply chain operations
- Data-driven decision making
- Targeted marketing and promotions
- Fraud detection and prevention
- Enhanced customer experience
- Competitive advantage
Three Real-World Big Data Use Cases in Retail
- Walmart's Data-Driven Supply Chain
One of the world's largest retailers uses predictive analytics to forecast demand, optimize inventory levels, and manage logistics across its massive network of stores. This approach has enabled Walmart to reduce overstock and stockouts.
- Starbucks' Personalized Marketing
Starbucks provides personalized offers and recommendations by analyzing customer data through its loyalty program and mobile app. This strategy has increased customer engagement, higher sales per visit, and boosted loyalty program sign-ups.
- Amazon's Recommendation Engine
Amazon provides highly personalized product recommendations by analyzing past purchases, browsing history, and customer reviews. This system accounts for a significant portion of sales, showcasing the power of personalized marketing and cross-selling.
Big Data Analytics Solutions
Big data analytics has become a cornerstone in the retail industry, offering many applications that change how retailers manage their operations and interact with customers.
Inventory Management
Retailers use big data analytics to predict which products will be in high demand. They can optimize stock levels by analyzing sales patterns, seasonal trends, and social media trends. It leads to cost savings, improved customer satisfaction, and increased sales.
Customer Segmentation
Big data allows retailers to segment customers more accurately based on their shopping behaviors, preferences, and demographics. Retailers create more effective marketing campaigns and build stronger customer relationships, leading to higher conversion rates.
Pricing Optimization
Retailers adjust prices in real-time by analyzing customer demand, competitor pricing, market conditions, and weather forecasts. It maximizes profits and ensures competitiveness, as prices are always in tune with market dynamics.
Supply Chain Management
By analyzing data from the entire supply chain, retailers identify inefficiencies and optimize predictive maintenance of equipment, route optimization for deliveries, and better supplier management for improved efficiency, reduced costs, and more reliable delivery.
Predictive Analytics for Sales Forecasting
It involves analyzing historical sales data, current market trends, and other external factors like economic indicators. It allows for better strategic planning, more effective marketing, and more efficient inventory management.
Big Data Analytics in Retail Cases
You can see several big data applications in retail:
- Walmart’s analytics hub, known as the Data Café, processes over 2.5 petabytes of data every hour to make real-time decisions on inventory and operations across its stores.
- Target uses big data to predict customer needs, famously identifying expecting mothers based on their shopping habits and targeting them with relevant offers.
- Nike leverages data from its online platforms and apps to personalize marketing and create products that align with customer preferences, enhancing customer experience.
Challenges and Risks of Big Data Analytics in Retail
While big data analytics offers numerous advantages to the retail industry, it also brings challenges and risks that need careful consideration and management. To address these challenges, retailers must adopt a robust data governance framework, ensure compliance with data protection laws, invest in the right technology infrastructure, and focus on building a skilled team with expertise in data analytics.
Ethical and Privacy Considerations
With increasing volumes of personal data being collected, there's a heightened risk of privacy breaches and misuse of information. If not handled correctly, it can lead to legal repercussions under data protection laws like GDPR and CCPA and damage to the retailer's reputation. Customers are increasingly aware of their data rights and are concerned about how their information is used and stored.
Integration and Management of Big Data in Retail Systems
Retailers need to ensure that their infrastructure handles large volumes of varied data and seamlessly integrates it from multiple sources. Poor integration leads to data silos, inefficiencies, and inaccurate analytics, which in turn can result in misguided strategies and decisions. There's also the technological challenge of ensuring data quality and the reliability of analytics outputs.
Staffing and Training Needs for Successful Implementation
Finding and retaining qualified staff can be challenging, especially given the competitive market for these skills. A retail organization might struggle to extract meaningful insights from the data without the right team. Moreover, there's a need for ongoing training and development to keep up with evolving technologies and analytical techniques. We can consider your case, just schedule a call.
Future Trends in Big Data for Retailers
As we look ahead, big data analytics in the retail sector is poised for further transformative innovations and trends.
- Advanced AI and machine learning algorithms are becoming more sophisticated, enabling more profound and accurate insights into customer behavior, demand forecasting, and inventory optimization. AI-driven personalization will reach new heights.
- The integration of IoT with big data analytics is a game-changer. Smart shelves, IoT-enabled inventory management, and in-store sensors will provide real-time data streams, enhancing the efficiency of operations and customer engagement.
- Augmented and Virtual Reality (AR and VR) technologies will integrate with big data to offer immersive shopping experiences. Virtual try-ons, interactive 3D product views, and AR-based navigation in physical stores will become more prevalent.
- With the rise of intelligent assistants and visual search technologies, big data analytics will play a crucial role in interpreting and responding to voice and image-based queries, further blurring the lines between online and offline retail experiences.
- Predictive analytics will evolve to offer hyper-personalization in marketing and sales, predicting customer needs and preferences with remarkable accuracy and enhancing the effectiveness of targeted marketing campaigns.
The future of big data analytics in retail is dynamic, with emerging technologies and trends continually reshaping the landscape. The ongoing evolution of these analytics will make big data transforming operations to redefine the shopping experience.
Impact Of Big Data Analytics in Retail
Several more retailers have harnessed the power of big data analytics to drive significant improvements in their operations, sales, and customer experiences. Here are some big data in retail examples.
Home Depot’s Omnichannel Strategy
The home improvement retail giant Home Depot integrated big data analytics into its omnichannel strategy, combining online and offline customer data for a seamless shopping experience. This approach resulted in a 6.7% increase in year-over-year sales and a significant improvement in customer satisfaction scores, as shoppers enjoyed a more personalized and efficient shopping experience across all channels.
Zara's Fast Fashion Powered by Big Data
Zara, a part of the Inditex group, is renowned for its fast fashion model. Big data analytics is pivotal, enabling rapid response to fashion trends. By analyzing sales data, customer feedback, and fashion trends in real-time, Zara reduced its design-to-sale cycle to just two weeks. This agility contributed to a 20% annual increase in sales and helped Zara maintain its position as a leading fashion retailer.
Best Buy’s Customer-Centric Strategy
Best Buy's large chain of electronics stores implemented big data analytics to enhance its customer-centric approach and compete effectively with online retail giants. They optimized product offerings and store layouts by analyzing customer purchasing patterns and feedback. This led to a 5% increase in domestic sales. The analytics also enabled more effective inventory management, reducing carrying costs and improving profitability.
A Retailer's Guide to Adopting Big Data Analytics
Step 1: Define Objectives and Scope
Determine what you want to achieve with big data analytics. Establish the scope of your big data initiative. Before scaling up, decide whether to start with a specific area (like sales or customer feedback).
Step 2: Assess Current Data Infrastructure
Review your current data collection and storage systems. Understand the limitations and potential of your existing infrastructure. Identify gaps in your current data setup, including data types not being captured that could be valuable.
Step 3: Plan Data Collection and Integration
Pinpoint various data sources relevant to your objectives: transaction data, online customer interactions, supply chain data, etc. Develop a strategy for integrating disparate data sources. It may require cloud-based solutions or data warehousing techniques.
Step 4: Select Appropriate Big Data Analytics Tools
Explore big data tools and platforms that align with your objectives and existing systems. Options include Hadoop, Apache Spark, or cloud-based services. Ensure the tools you select are customized to your needs and are scalable as your data requirements grow.
Step 5: Talent Acquisition and Training
Acquire talent with expertise in big data analytics: data scientists, analysts, and IT professionals with relevant experience. Invest in training for your existing staff. Familiarize them with big data concepts and tools to ensure a smooth integration.
Step 6: Implementation and Integration
Start with a pilot program focusing on a specific aspect of your retail operations. It helps in understanding the practical challenges and potential benefits. Gradually expand the implementation to other business areas, integrating insights from the pilot phase.
Step 7: Integral Data Analysis and Insight Generation
Use your analytics tools to continuously analyze the collected data. Look for patterns, trends, and insights that align with your predefined objectives. Translate these insights into actionable strategies. It modifies marketing campaigns and adjusts inventory levels.
Step 8: Ongoing Review and Adaptation
Regularly monitor the outcomes of your big data analytics initiatives against your set goals. Be prepared to adapt your strategy based on the insights gained and evolving market conditions. Big data retail analytics is a dynamic process that requires continuous refinement.
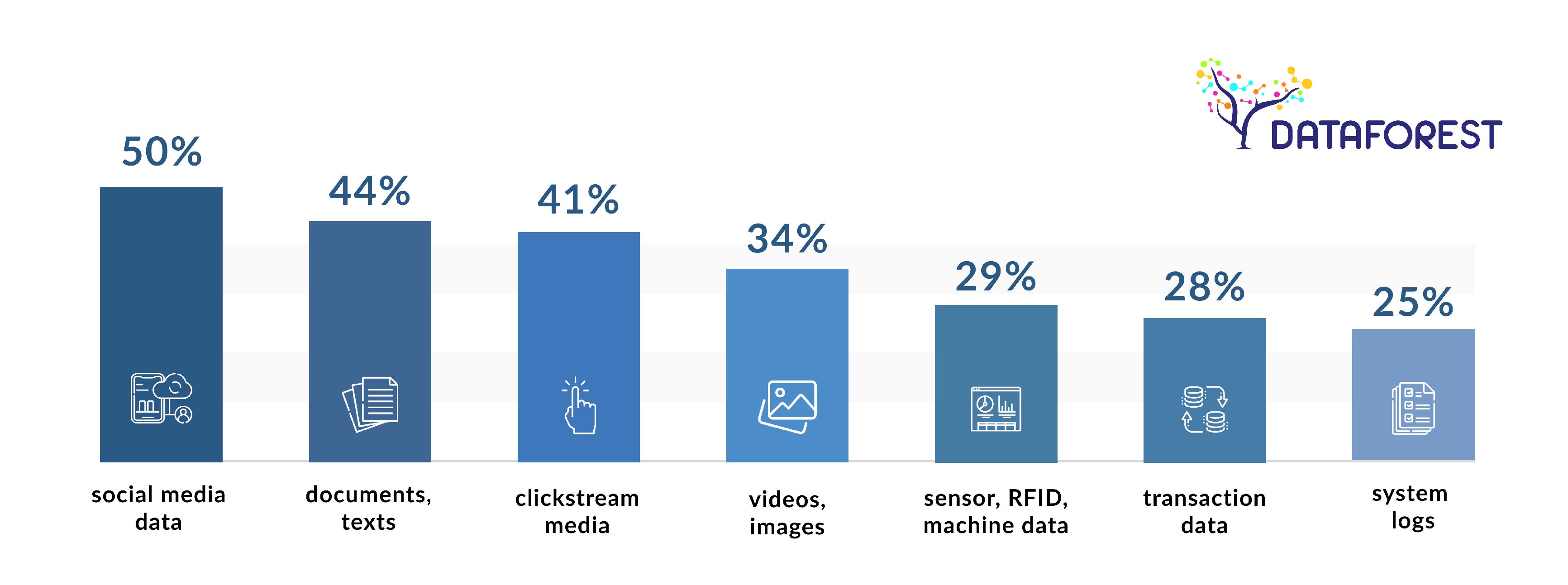
Areas where companies plan to increase their big data analysis investment
The Integral Role of Provider in Big Data Management
A data engineering provider is the mastermind behind managing and making sense of big data. They set up robust systems to collect and store vast amounts of data from varied sources, ensuring stability and efficiency. They organize this complex data, preparing it for analysis. Then, as data scientists, they dive deep into this data, using advanced analytics and machine learning to uncover hidden patterns and insights. They translate these findings into understandable, actionable businesses’ strategies for businesses. Please complete the form, and let's try it in your practice.
FAQ
How is big data used in retail?
Big data in retail is used to analyze customer behavior, preferences, and purchase history to enable personalized marketing technology strategies and optimize inventory management. Additionally, it aids in supply chain optimization by providing insights into demand forecasting and improving operational efficiency for retail companies.
How are big data problems solved in the retail sector?
Big data problems in the retail sector are often solved through AI analytics and machine learning algorithms, enabling retailers to extract meaningful insights from vast datasets, optimize inventory management, and personalize customer experiences. Additionally, implementing robust data infrastructure, cloud computing, and data integration solutions helps retailers handle and process large volumes of information efficiently.
What is big data analytics, and how does it apply to my retail business?
Big data analytics involves examining large, diverse data sets to uncover hidden patterns, customer preferences, and market trends. In your retail business, it can help you understand customer behavior, optimize your supply chain, personalize marketing strategies, and ultimately enhance customer experience and boost sales.
What are the key benefits of implementing big data analytics in the retail sector, and how can it improve my bottom line?
Implementing big data analytics in retail offers precise customer targeting, efficient inventory management, and enhanced shopping experiences, leading to increased customer loyalty and sales. By leveraging these insights, retailers streamline operations, reduce costs, and improve their bottom line through more effective marketing strategies and customer-centric decision-making.
Are there any real-world examples of big data in retail that have successfully implemented big data analytics to drive growth and profitability?
Major retailers like Walmart and Amazon have successfully used big data analytics to drive growth and profitability, optimizing everything from supply chain logistics to personalized customer recommendations. Their strategic use of data has enabled them to better understand customer needs, predict market trends, and enhance operational efficiency, setting new standards for the use of big data in the retail industry.
What big data in the retail industry case study can you suggest as significant?
One significant big data implementation in the retail industry involves Amazon, which leverages big data to analyze customer behavior, predict purchasing patterns, and optimize its vast product inventory. Amazon enhances customer recommendations through AI analytics and machine learning, streamlines its supply chain, and continuously improves the shopping experience.
How can I get started with big data analytics in my retail business, mainly if I have limited technical expertise?
To start with big data analytics in your retail business, consider partnering with a data analytics service provider who can tailor solutions to your specific needs and guide you through the process. Additionally, investing in user-friendly analytics software designed for non-technical users helps you gradually build your in-house capabilities and better understand your customers and business. It improves the use of big data in retail.
What are the common challenges or barriers businesses face when adopting big data analytics, and how can I address them?
Common challenges in adopting big data analytics include integrating diverse data sources, ensuring data quality and security, and facing a shortage of skilled data professionals. To address it, businesses invest in robust data integration tools, prioritize data security measures, and seek partnerships or training to build expertise in data analytics.
How does big data analytics impact customer experience and satisfaction in the retail industry?
Big data analytics significantly enhances customer experience in retail by enabling personalized shopping experiences and recommendations tailored to individual preferences and behaviors. This increases customer satisfaction, as shoppers feel understood and valued and are more likely to find products that meet their needs and expectations.
What types of data should I collect, and how can I ensure data privacy and security for my customers?
In your retail business, focus on collecting customer demographics, purchase history, online browsing behaviors, and feedback, which provide valuable insights into customer preferences and trends. To ensure data privacy and security, adhere to data protection regulations like GDPR, implement cybersecurity measures, and be transparent with customers about how their data is used and protected. It's true for all applications of big data in retail.
Can big data analytics help me with inventory management and supply chain optimization, and if so, how?
Big data analytics significantly aids in inventory management and supply chain optimization by analyzing sales patterns, demand forecasting, and supplier performance, allowing for more accurate stock levels and efficient logistics. It leads to reduced costs, minimized stockouts or overstock situations, and a more responsive and streamlined supply chain that aligns closely with customer demand and market trends.
What role does artificial intelligence and machine learning play in the future of big data for retail?
Artificial Intelligence (AI) and Machine Learning (ML) are set to revolutionize big data analytics in the retail industry by enabling more advanced, predictive insights and automating complex decision-making processes. These technologies will allow retailers to anticipate customer needs, optimize operations, and create more personalized shopping experiences, driving innovation and competitive advantage through big data analytics in the retail sector. It also makes handling big data and knowledge management in retail easier.
How can I measure the ROI of my big data analytics initiatives in the retail sector, and what key performance indicators should I be tracking?
To measure the ROI of your big data analytics initiatives in retail, track key performance indicators: increased sales revenue, improved customer retention rates, reduced operational costs, and enhanced marketing campaign effectiveness. Additionally, monitor metrics like inventory turnover, customer satisfaction scores, and supply chain efficiency to gauge the direct impact of your data-driven strategies on business performance and growth.