The main tension in financial risk management can be likened to walking on a tightrope between two tall buildings. The first one represents the pursuit of higher profitability. It symbolizes the desire to take calculated risks and venture into potentially lucrative investments. This building represents the willingness to step outside the comfort zone and embrace risk to achieve financial success. On the other side, the second building represents the need for stability. It symbolizes the importance of protecting assets and maintaining a solid financial foundation. This building represents the caution required to avoid excessive risk-taking and preserve the institution's long-term viability. If you need an individual approach to a solution, arrange a call.
Financial risk management market statistics (2021)
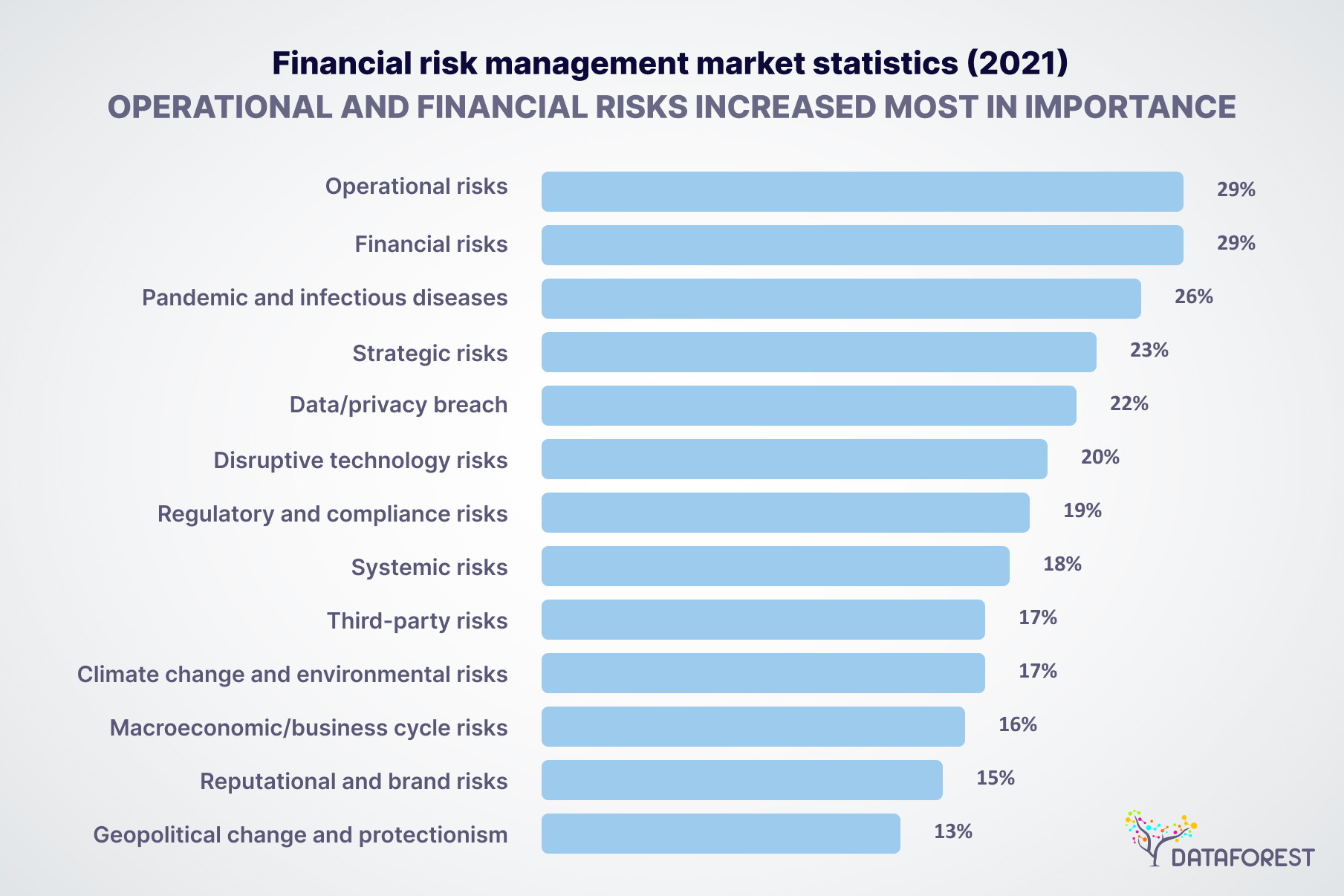
Financial Risk Management Provides Effective Strategies
Financial risk management is important because it helps protect individuals, organizations, and institutions from significant financial losses. It promotes stability and long-term viability by mitigating risks and ensuring resilience in market fluctuations or unexpected events. It also helps comply with regulatory requirements, avoiding penalties and legal consequences. Financial risk management enhances decision-making by providing valuable insights and data-driven information, leading to more effective strategies.
Credit and Market Financial Risk Management
Individuals, corporations, and financial institutions manage market risk through diversification, hedging techniques, setting risk limits, and monitoring market trends and indicators closely.
The Bridge for Financial Risk Management
Credit financial risk management techniques can be compared to building a sturdy bridge across a fast-flowing river. Before constructing, it is crucial to assess the strength and reliability of each bridge pillar. Credit risk management means evaluating the creditworthiness of borrowers. Like a bridge requiring multiple stability pillars, credit risk management software emphasizes diversifying the lending portfolio. Adding support pillars to the bridge enhances its stability. In credit risk management, collateralization implies obtaining collateral from borrowers as security against the loan.
The potential loss in financial risk management
It arises when borrowers fail to fulfill their financial obligations, resulting in non-payment or default. It is a significant risk faced by financial institutions: banks, lending institutions, and credit card companies that provide credit to individuals, businesses, or governments. The impact of credit risk on financial institutions is substantial:
- When borrowers default on their loans or credit obligations, financial institutions can experience direct losses without financial risk management.
- Non-performing loans, where borrowers fail to make scheduled payments, reduce the interest income generated by the institution.
- In financial risk management, significant credit losses deplete the capital reserves of financial institutions, impacting their capital adequacy and solvency ratios.
- Credit risk events, particularly if they gain media attention or affect a large number of customers, harm the reputation of financial institutions.
- In cases where credit risk is widespread and affects multiple financial institutions, it contributes to systemic risk and contagion for financial risk management.
To mitigate the impact of credit risk, financial institutions employ credit financial risk management techniques.
Traditional credit risk
Traditional credit financial risk management techniques require a set of practices and strategies financial institutions use to assess, mitigate, and manage credit risk.
- Credit assessment
- Credit scoring models
- Collateral and security
- Risk-based pricing
- Credit limits and exposure management
- Credit monitoring and early warning systems
- Loan covenants
- Loan loss provisioning
These traditional credit financial risk management techniques provide a framework for financial institutions to assess borrower creditworthiness, monitor credit performance, and implement strategies to mitigate credit risk.

Data science methodologies for financial risk management
Benefits and limitations
Data science offers significant benefits in financial risk management, including improved accuracy, efficiency, and early detection of risks. However, challenges related to data quality, interpretability, regulatory compliance, and biases need to be addressed to fully harness the potential of data science in credit risk management.
Financial Risk Management for Skilled Sailors
Market risk management techniques are the tools and strategies that make investors and businesses navigate the turbulent waters of the financial markets, much like skilled sailors navigating treacherous seas. These techniques serve as the sextant and charts that guide decision-making, steering through the unpredictable waves of potential risks.
Adverse changes
Market financial risk management touches on the potential for financial losses arising from adverse changes in market prices or conditions. It is the risk that the value of an investment or portfolio will decrease due to fluctuations in interest and exchange rates, commodity prices, equity prices, or market volatility. Market risk greatly impacts financial institutions, including banks, foreign investment firms, and insurance companies.
- A decline in market prices or an unfavorable market environment leads to losses on investments, trading positions, or proprietary trading activities.
- If the market risk example leads to significant losses, it depletes the institution's capital, potentially putting it at risk of breaching regulatory capital requirements.
- If market conditions deteriorate rapidly, it becomes challenging for institutions to sell assets or obtain funding.
- Adverse market events damage the reputation and erode the confidence of stakeholders in financial institutions.
- Non-compliance with regulatory requirements leads to penalties, sanctions, or restrictions on the institution's activities, which impact its operations.
To control market risks, financial institutions must implement robust financial risk management frameworks, including effective market risk management practices.
Traditional market financial risk management techniques
They encompass various strategies and tools financial institutions employ to identify, measure, monitor, and manage market risks.
- Value at Risk (VaR) is a widely used quantitative measure that estimates the potential loss in value of a portfolio or investment over a specified time horizon with a certain level of confidence.
- Stress testing involves simulating extreme but plausible market scenarios to assess the impact on a portfolio or institution. It goes beyond normal market conditions to evaluate the resilience of investments and the overall financial system.
- Historical data rates the potential losses of a portfolio based on the past behavior of market prices. Financial risk management demands constructing a model that captures the relationship between market factors and asset prices.
- Scenario analysis calls for creating hypothetical market scenarios and assessing their impact on a portfolio or institution. It concerns analyzing the potential outcomes of specific events or changes in market conditions.
- Diversification entails spreading investments across different asset classes, sectors, and geographic regions to reduce exposure to any single investment or market risk. By diversifying their portfolios, dealers achieve a balance sheet between risk and return.
- Hedging presumes taking positions that offset potential losses in a portfolio due to adverse market movements. Institutions use derivatives — futures contracts, options, and swaps — to hedge against specific business risks.
- Active portfolio management implies continuously monitoring and adjusting portfolio positions based on market conditions and risk assessments. Institutions actively manage their portfolios by making strategic investment decisions.
By effectively implementing these techniques, financial institutions enhance their risk management practices, protect capital, and optimize their risk-return profile.
Advanced analytical tools
Data science lifts market risk management techniques by leveraging advanced analytical tools and methods to process large volumes of data, extract insights, and improve decision-making processes.
Improved data analysis
Data science enables financial institutions to analyze vast amounts of historical market data, including price movements, trading volumes, and market indicators. By applying statistical models and machine learning algorithms, data scientists identify patterns and correlations in the data that may not be apparent through traditional analysis methods.
Enhanced risk models
Data science techniques improve the accuracy and effectiveness of risk models used in market financial risk management. Institutions build sophisticated models that capture complex market dynamics and risk factors. These models provide accurate assessments of potential losses, enable better risk quantification, and enhance the ability to stress-test portfolios under different scenarios.
Real-time monitoring and early warning systems
Data science allows for developing real-time monitoring and early warning systems that continuously analyze market data and indicators. Financial institutions can use algorithms to stream data to detect anomalies, deviations from expected patterns, or early signs of market stress.
Predictive analytics
By analyzing historical market data, macroeconomic factors, and other relevant information, predictive models generate forecasts and probability distributions for market variables. These predictions enable risk managers to assess the impact on portfolios, evaluate alternative strategies, and proactively make informed decisions to manage market risks.
Automation and efficiency
Data science automates and streamlines manual processes in market financial risk management, increasing efficiency and reducing errors. Algorithms and artificial intelligence help institutions automate data collection, cleansing, and validation processes. It frees up resources and allows risk managers to focus on higher-value tasks: data interpretation, strategy formulation, and decision-making.
Enhanced stress testing
Data science techniques enhance stress testing capabilities by enabling the simulation of a wide range of scenarios and analyzing the potential impact on portfolios. Incorporating a diverse set of market variables and simulating extreme but plausible scenarios make possible the resilience of their portfolios, identify vulnerabilities, and refine risk mitigation strategies.
Famous data science techniques
- Monte Carlo simulations use random sampling to model the variability of market factors. It means generating multiple scenarios by randomly sampling from probability distributions of key variables: asset prices, interest rates, or exchange rates.
- In market financial risk management, time series analysis techniques — autoregressive integrated moving average (ARIMA) models or exponential smoothing methods — are applied to historical market data to identify patterns, trends, and seasonality.
- Machine learning algorithms, such as random forests, support vector machines, or neural networks, process large volumes of data, learn patterns, and make predictions. They predict price movements, identify risks, or automate decision-making processes.
- Multivariate analysis techniques help analyze the relationships and dependencies between multiple variables simultaneously. Principal component analysis (PCA) identifies common factors or correlations among different asset returns.
- Text mining and sentiment analysis techniques analyze unstructured textual data, such as news articles, social media feeds, or analyst reports, to gauge market sentiment and extract relevant information in financial risk management conception.
- Deep learning employs artificial neural networks with multiple layers to extract complex patterns. In market risk management, deep understanding is used for tasks such as natural language processing (NLP), image recognition for analyzing charts and graphs, or anomaly detection for identifying unusual market behaviors.
Using data science for market risk management
Data science offers market financial risk management several benefits, but it also comes with certain limitations.
Schedule a call to complement reality with a profitable solution.
Versatile Toolbox for Financial Risk Management
Imagine a vast workshop filled with curious artisans eager to build innovative tools. It represents the evolving landscape of financial risk management. At first, the artisans possessed only basic tools, symbolizing traditional risk management techniques. These tools, while reliable, need to be improved in capturing the intricacies of modern financial markets. Then, from the depths of the workshop, a new material emerges — data. It flows in like a river, carrying immense potential and untapped knowledge. The artisans realize that by harnessing the power of this data, they can create special tools to navigate the complex world of financial risk management. One by one, the artisans begin to fashion new instruments.
Effectiveness of data science techniques
Financial institutions forcing data science techniques in credit and market financial risk management have observed positive outcomes in terms of improved risk assessment, enhanced decision-making, increased efficiency, and proactive risk mitigation. However, careful attention must be given to data quality, interpretability, skill requirements, and ongoing model validation to maximize the benefits of these techniques and ensure effectiveness in the real world.
Global survey focused on Model Risk Management (MRM) during January and February 2022
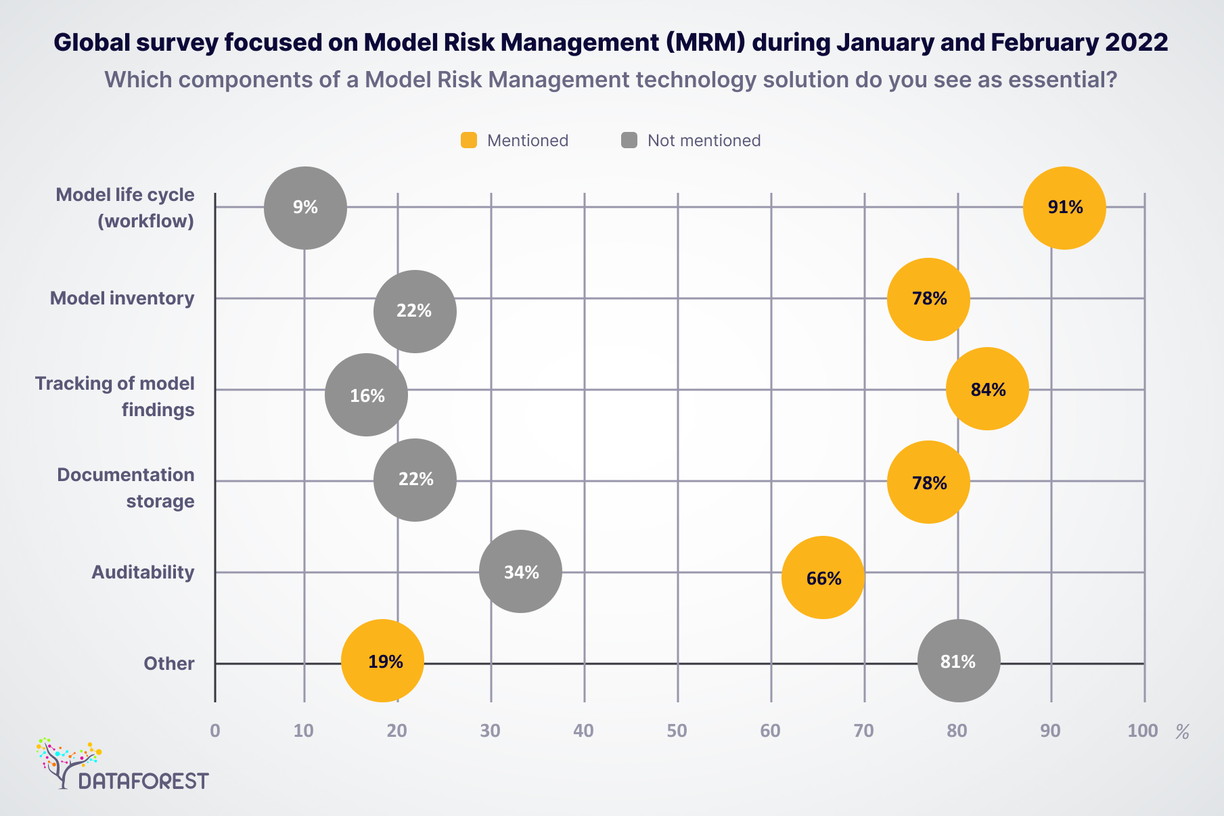
Combining Risk and Return in Financial Risk Management
Combining risk and return in financial risk management with data science as a service from DATAFOREST means leveraging our expertise and resources. Financial institutions can analyze historical data, develop predictive models, and perform risk assessments to optimize their risk-return trade-off by accessing extensive datasets, advanced analytics tools, and custom risk models.
The collaboration with data science for financial service providers enables institutions to benefit from specialized knowledge, advanced techniques, and comprehensive reporting to make data-driven decisions and enhance financial risk management strategies. That's what you need? Then please fill out the form, and we will share our thoughts with you.
FAQ
What is financial risk management, and why is it important in the financial industry?
Financial risk management identifies, assesses, and mitigates potential risks that could impact financial institutions and their stakeholders. It is crucial in the financial industry to safeguard against adverse events, maintain stability, and protect assets while optimizing returns.
What are the different types of financial risks that companies face, and how can they be managed?
The different types of financial risks that companies face include credit risk, market risk, liquidity risk, operational risk, and reputational risk, which can be managed through various measures such as diversification, risk assessment, hedging, contingency planning, and implementing robust internal controls in financial risk management conception.
What is credit risk, and how can it be managed using techniques like credit scoring and diversification?
Credit risk refers to the potential for loss due to the failure of borrowers to repay their debts, and it can be managed by using credit scoring to assess the creditworthiness of borrowers and implementing diversification strategies to spread the risk across a portfolio of loans or investments with financial risk management. It also depends on the business school.
What is market risk, and how can it be managed using techniques like value-at-risk (VaR) and hedging?
Market risk types refer to the potential for loss arising from changes in market conditions, such as price fluctuations, and it can be managed by using techniques like value-at-risk (VaR) to quantify potential losses and implementing hedging strategies to mitigate the impact of adverse market movements according to financial risk management.
How do companies use stress testing to manage financial risks?
In financial risk management, companies use stress testing as a risk management technique to assess the resilience of their portfolios and identify potential vulnerabilities by subjecting them to extreme scenarios and analyzing the impact on financial metrics and stability.
What is operational risk, and how can it be managed using techniques like risk assessment and contingency planning?
Operational risks refer to the potential for loss resulting from internal processes, people, or systems, and they can be managed by using techniques like comprehensive risk assessment to identify potential vulnerabilities, implementing robust contingency plans, and strengthening internal controls to mitigate operational failures in financial risk management.
What is liquidity risk, and how can it be managed using techniques like asset/liability management and contingency funding plans?
Liquidity risk is the potential for a company to encounter difficulty in meeting its short-term financial obligations, and financial risk management implements techniques like asset/liability management to optimize cash flows and develop contingency funding plans to ensure access to sufficient liquidity during stress periods. Certified risk managers can help institutions.
What are some best practices for effective financial risk management?
Some best practices for effective financial risk management include conducting thorough risk assessments, diversifying portfolios, implementing robust internal controls, regularly monitoring and evaluating risks, and staying updated with regulatory requirements and industry trends. They provide zero risk tolerance.
What are some common challenges companies face when managing financial risks, and how can they be overcome?
Common challenges that companies face with financial risk management include data quality issues, evolving regulatory landscape, and the complexity of risk models, which are overcome by investing in data governance, staying updated with regulatory changes, and collaborating with risk management experts to develop robust and adaptable risk management plan and frameworks. It often happens with enterprise risk management tools in order of financial services.
How can companies use technology and data analytics to improve their financial risk management processes?
Companies can leverage technology and data analytics to enhance their financial risk management processes by automating data collection and financial analysis, implementing advanced risk models and predictive analytics, and gaining real-time insights for proactive risk mitigation and decision-making.
Are there some aspects related to managing financial risks?
During the month-end close, the financial crisis severely impacted the company's expenses, necessitating a reevaluation of the monetary policy and the management of mortgages and transfers to mitigate potential risks.