In recent years, the use of Big Data Analytics has become increasingly popular in various industries, including finance.
Big Data Analytics is the process of extracting relevant information and insights from large datasets to inform and support decision-making. In finance, Big Data Analytics can be used to analyze financial market trends, customer behavior, fraud detection, risk management, and more.
DATAFOREST can assist with your Big Data Analytic challenges in the finance industry by providing innovative, reliable, and cost-effective solutions. We provide Data Scraping, Data Science, Data Integration, Web applications, and DevOps services for the finance industry business processes automation, Data Insights & Forecasting.
In this article, we guide you through everything you need to know about the latest technologies, trends, and best practices to unlock the potential of Big Data Analytics in the finance industry. We know how to handle Big Data; arrange a call and you will know too.
Let us dive in!
Understanding Big Data Analytics and Its Importance in Finance
Big Data Analytics is a powerful set of techniques that provides deeper insights into the vast amount of data generated in the financial industry. It uses advanced analytics techniques such as machine learning, predictive analytics, data mining, and natural language processing to uncover patterns, relationships, and insights that are difficult to detect using traditional methods.
With the explosion of data in the financial industry, the application of Big Data Analytics has become increasingly important in driving informed decisions, reducing risks, and improving operational efficiency. The insights derived from Big Data can help financial institutions identify opportunities, anticipate potential outcomes, and make better decisions in real-time.
Moreover, Big Data Analytics can also help financial institutions to detect and prevent fraud. By analyzing large volumes of data, anomalies, and suspicious activities can be identified, allowing for timely intervention. This not only protects the financial institution but also helps to maintain customer and stakeholder trust
The Origin and Evolution of Big Data Analytics in Finance
The origin of Big Data Analytics in finance can be traced back to the early 2000s when firms started utilizing high-frequency trading algorithms to process vast amounts of data in real time. Over time, the use of Big Data Analytics has evolved to include other areas such as risk management, customer analytics, fraud detection, and compliance. Learn more about the importance of data analytics in business today!
The increasing availability of data, coupled with advancements in technology access and distribution, has contributed to the growth of Big Data Analytics in finance. In recent years, cloud-based solutions, web services and artificial intelligence have become more prevalent in the financial industry, enabling institutions to process larger volumes of data at faster speeds.
Key Benefits of Using Big Data Analytics in Finance
The use of Big Data Analytics offers several benefits to financial institutions, including:
- Improved decision-making: By providing insights into market trends, customer behavior, and risk potential, Big Data enables companies to make better predictions about their future financial performance by analyzing past performance trends and customer preferences. This provides them with a competitive advantage over other companies who don't have access to this kind of information about their customers or competitors' products/services. This may help them decide what new products should be developed next year based on how well they did last year when they were released into the marketplace.
- Increased efficiency: Big Data Analytics can automate processes and reduce manual labor, delivering increased efficiency and improved productivity. With access to real-time insights into their operations (such as production lines), managers can make changes in real time rather than reactively.
- Better risk management: Big Data Analytics can help financial institutions identify and manage risks proactively.
- Enhanced customer experience: Big Data Analytics can help firms better understand customer needs and preferences, resulting in more personalized products and services.

Understanding the Different Types of Data Used in Big Data Analytics for Finance
Big Data Analytics can be applied to a variety of data types, including structured, semi-structured, and unstructured data.
- Structured data refers to well-organized data that can be easily analyzed using traditional methods, such as financial reports and transaction histories.
- Semi-structured data refers to information that is not entirely organized, such as social media posts, blog articles, and customer feedback.
- Unstructured data refers to information that has no specific organization, such as audio and visual content, texts, and images. Big Data Analytics can effectively analyze all of these types of data.
Big data analytics can be used to uncover valuable insights about anything from consumer behavior to financial performance. The key is having access to the right type of data at the right time. Some of the most common types of data used in big data analytics for finance are:
- Financial transactions: This includes payments made by consumers using credit cards, debit cards, or checks. These transactions are stored in databases called transaction processing systems (TPSs).
- Customer demographics: This is information about who is buying what from your company — their gender, age, location, and other demographic characteristics can be used to understand their needs better. This information can also help you target specific segments with advertising campaigns or offers tailored to appeal specifically to them.
- Consumer data: Customer data refers to all the information about your customers that you have collected and stored. This can include their contact details, purchase history, buying preferences, and habits — anything that might help you understand them better.
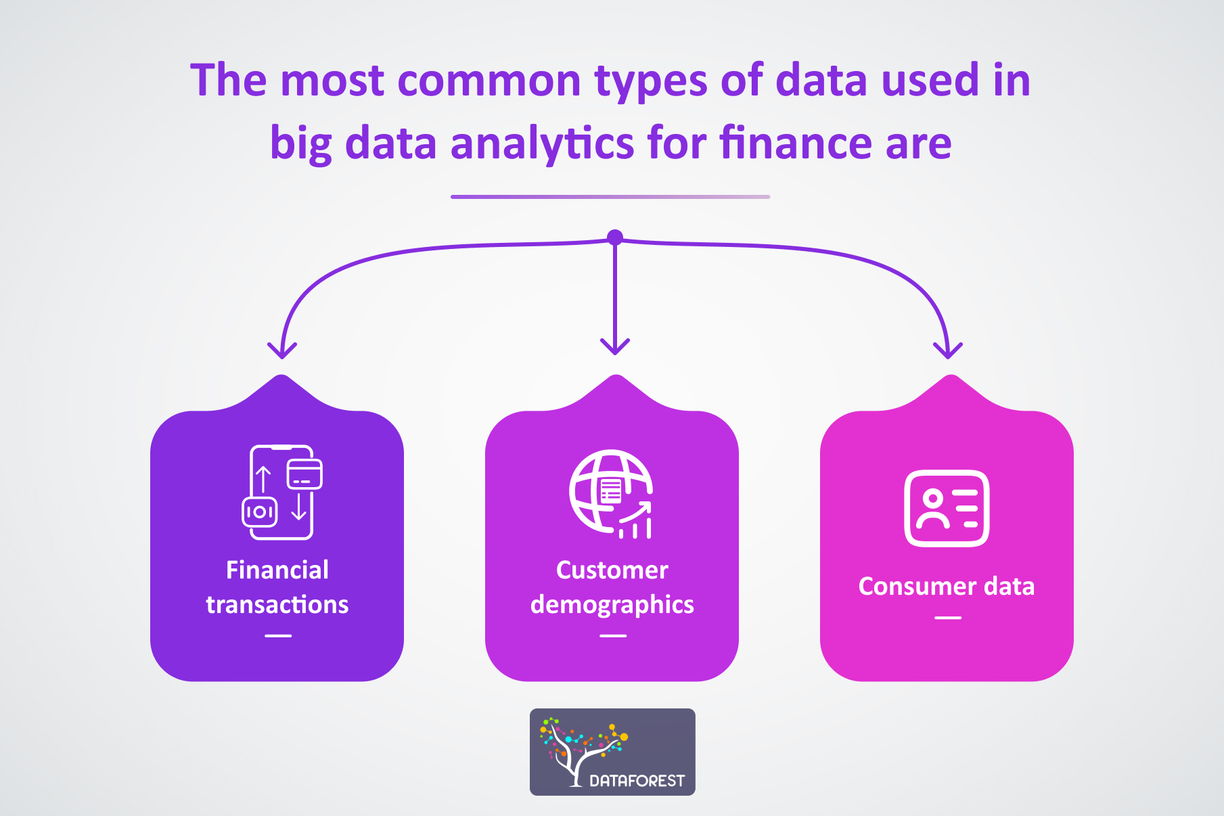
Data Preparation for Big Data Analytics in Finance
- Data preparation is the first step in any data analytics process, and it's essential to get it right. It's also one of the most time-consuming steps, but there are several ways to make it easier.
- Data cleaning and preprocessing: Removing missing values, outliers, and errors from your dataset before you start analyzing it will certainly save you time later on when you're trying to figure out what went wrong with your analysis!
- Data integration: When working with multiple sources of information or datasets that don't match up perfectly (for example because they come from different companies), then this step will help bring all these different sources together into one place in a single consistent and accessible format.
- Data Visualization: The presentation of collected and analyzed data as charts, graphs, summary tables and other visual representations. This step can be an important part of the analysis process because it allows you to get a better idea of how everything fits together, and how different factors interact with one another.
Data visualization tools are an integral part of any big data analytics project. They allow you to create charts, graphs, and other visual representations of your data that help you understand it better. They also make it easier for others to understand what's going on with your business. This can be especially important when communicating with stakeholders outside of IT or finance who may not be as familiar with how these technologies work.
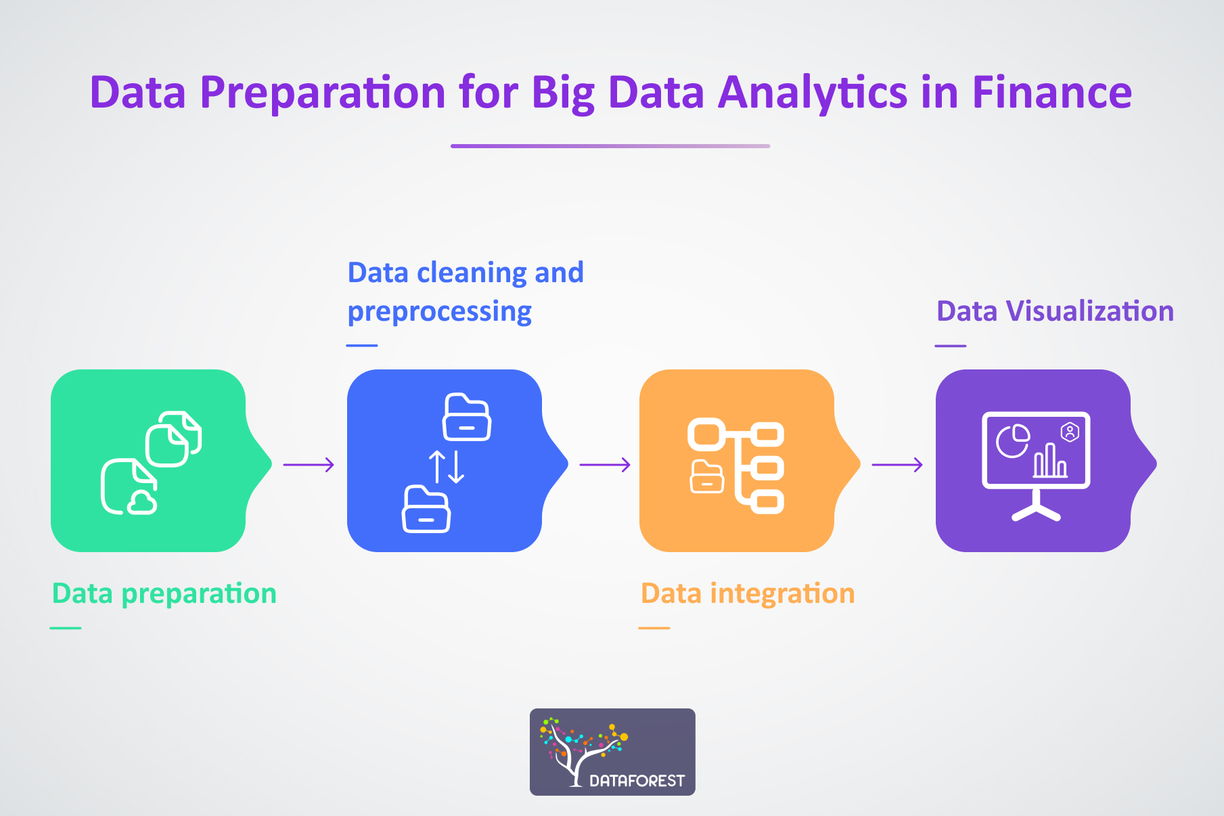
Data Analysis & Interpretation for Big Data Analytics in Finance
Data analysis and interpretation are important steps in the data mining process.
Data analysis helps you identify patterns and relationships in your data, which can lead to new insights and discoveries. It's also used as a tool for visualizing your data so you can better understand it.
Data interpretation is the process of converting raw data into meaningful information. In finance, this can be done by analyzing financial statements, market data, and other sources of information to make informed decisions about investments.
DATAFOREST provides Data Science & Analysis services for business intelligence in the finance industry. We have specialists with expertise in many different domains such as finance, data science, and machine learning who can help you with the exploratory analysis of your data. This process can involve using different techniques like predictive modeling algorithms based on deep learning neural network models which will give you accurate predictions about future outcomes based on historical data sets. It will help you interpret your data sets accurately providing valuable insights that lead to better decision-making. Interested in the update? Arrange a call and we'll tell you what's what.
Data Governance Principles for Big Data Analytics in Finance
Data governance is the process of managing and governing data assets. It ensures that data is available when needed and that it is accurate, secure, complete, and consistent across all business systems. The following principles should be followed when implementing a data governance program:
- Data Stewardship: The process of ensuring that all data within the domain is managed according to agreed-upon policies and procedures. This includes defining what information needs to be collected; determining how it will be used; controlling access rights; monitoring compliance with regulations such as GDPR etc.; maintaining records of changes made during processing operations so they can be better later if required (e.g. version control). Data stewards are typically assigned to each of the relevant data domains to manage the application of these principles.
- Master Data Management: Ensuring that all master records are consistent across multiple databases without duplication or gaps. This is achieved by centralized control over schema definitions/metadata about each item being stored (i.e. tables), relationships between them (i.e. foreign keys), etc. MDM can be enforced and managed using tools like Informatica Power Center which provides integration capabilities between different systems such as ERP/CRM solutions from SAP®, Oracle®, etc., database management systems like Microsoft SQL Server®, MySQL®, etc., file storage systems like IBM DB2®, Oracle RAC 11gR2 Cluster Edition.
Popular Tools and Technologies Used for Big Data Analytics in Finance
The use of Big Data Analytics in finance requires the use of various tools and technologies to process and analyze data. Some of the popular tools and technologies used include:
- Apache Hadoop: An open-source software framework for distributed storage and processing of big data.
- Apache Spark: A data processing engine that can handle large-scale data processing.
- NoSQL Databases: Databases that can handle unstructured and semi-structured data at scale.
- Machine Learning: A technique that uses algorithms to identify patterns in large datasets.
- Cloud Computing: A method of delivering computing services and infrastructure over the internet.
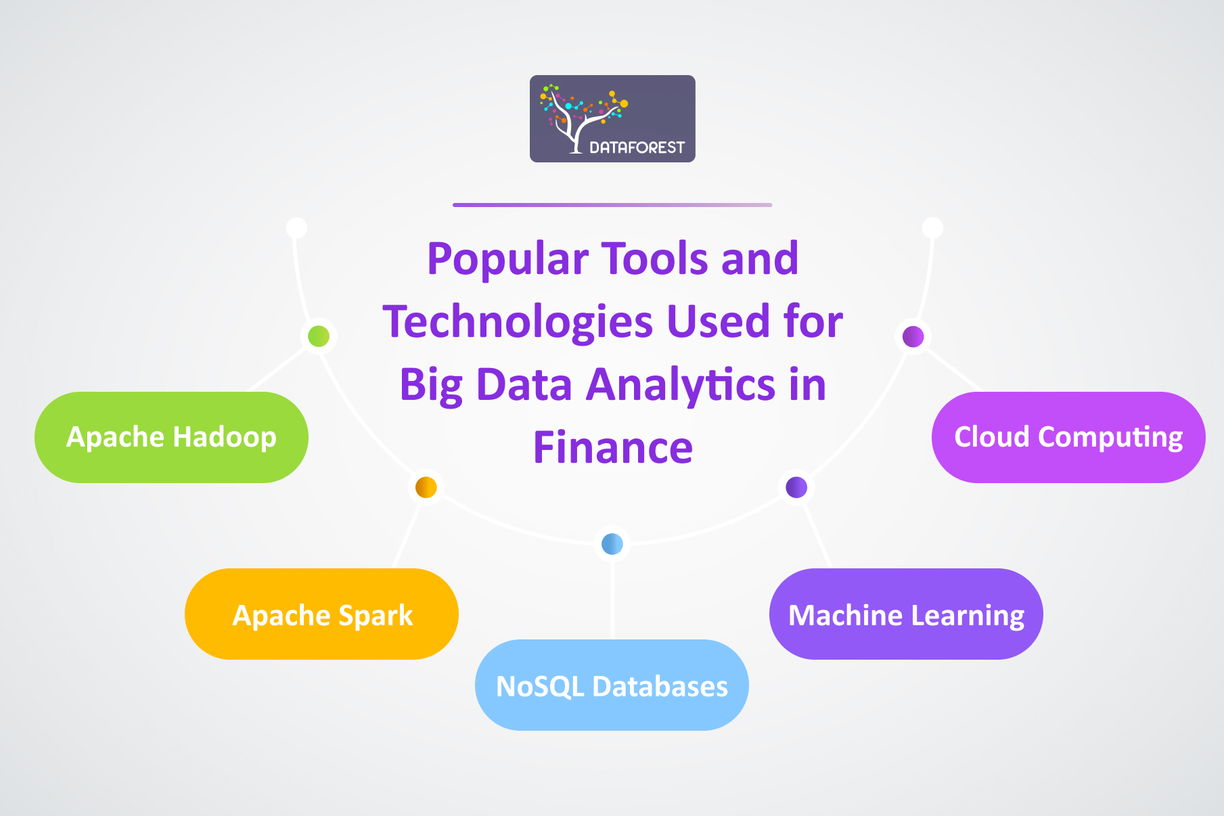
Common Challenges Faced by Financial Institutions While Implementing Big Data Analytics
Despite the many benefits of Big Data Analytics for financial institutions, there are also several common challenges that they face while implementing it. Some of these challenges include:
- Data quality and accuracy: Big Data Analytics is only effective when the data is accurate and of high quality.
- Data integration: Big Data Analytics involves processing and analyzing data from multiple sources, and integrating them can be challenging.
- Scalability: Financial institutions must consider how to scale up their Big Data Analytics solutions as their data volumes increase.
- Data security and privacy: Financial institutions must ensure that they comply with regulatory laws around data privacy and security.
Innovative Strategies to Overcome Challenges During Implementation
There are several innovative strategies that financial institutions can use to overcome the challenges they face while implementing Big Data Analytics. These strategies include:
- Data governance: Establishing data governance policies and procedures can help ensure data quality, accuracy, and security.
- Data lakes: Storing data in a central repository can help improve data integration, scalability, and management.
- Data anonymization: Anonymizing data can help financial institutions comply with regulatory laws around data privacy and security.
- Artificial Intelligence: AI can help automate processes and reduce manual labor, resulting in increased efficiency and productivity.
Real-life Examples of How Big Data Analytics is Revolutionizing the Financial Industry
The use of Big Data Analytics has already transformed the financial industry in many ways. Some real-life examples of how it is revolutionizing the industry include:
- Anti-money laundering: Big Data Analytics is being used to identify suspicious activities and detect potential money laundering in real time.
- Fraud detection: Big Data Analytics is being used to detect fraudulent activities, such as credit card fraud, insurance fraud, and identity theft.
- Risk management: Big Data analytics can be combined with machine learning algorithms to build models that predict risks based on historical data as well as current market conditions (e.g., volatility). Models can recommend actions that mitigate these risks, such as increasing capital requirements or reducing exposure limits until more information becomes available about specific trades.
- Predictive analytics: Big Data Analytics is being used to predict market trends and anticipate potential outcomes, allowing financial institutions to make better investment decisions.
- Customer segmentation: Segment customers into different groups based on their behavior patterns so that each group receives personalized services (e.g. advertising, product recommendations or special offer) tailored specifically for them; this helps companies improve customer satisfaction while reducing costs associated with servicing individual customers.
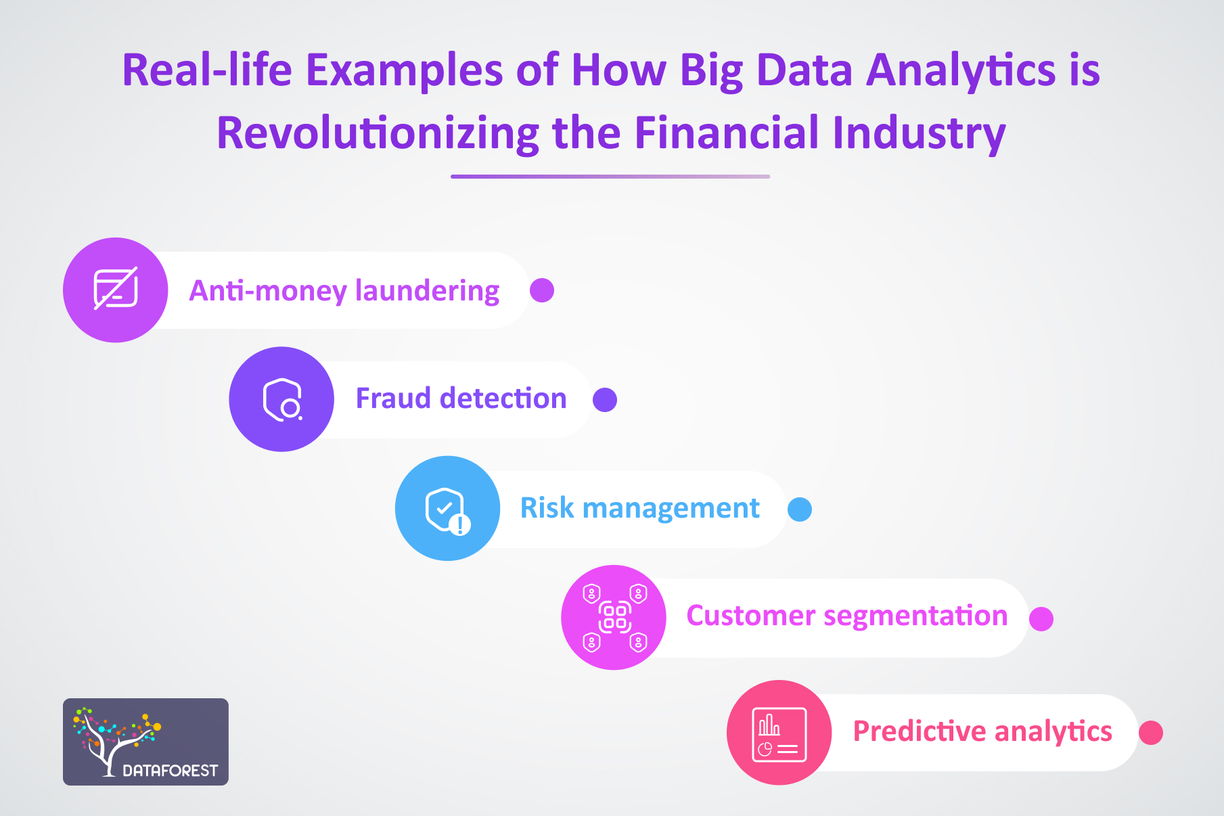
Future Possibilities of Big Data Analytics in Finance
The use of Big Data Analytics in finance is expected to continue growing rapidly. Some of the possibilities for Big Data Analytics in finance include:
- More personalized services: With the help of Big Data Analytics, financial institutions will be able to offer more personalized products and services tailored to individual customer needs.
- Improved risk management: Big Data Analytics will help financial institutions identify and manage risks more effectively, resulting in better outcomes for both the institution and its customers.
- Greater transparency: Big Data Analytics can help financial institutions to provide transparency to their customers around their business activities and services.
Embracing Big Data Analytics with DATAFOREST to stay ahead in an increasingly competitive finance market
Big Data Analytics is a powerful tool that can provide financial institutions with deeper insights into vast amounts of data. Despite the challenges that come with implementing Big Data Analytics, financial institutions that successfully address these can use innovative data-driven strategies and reap the benefits.
As we look to the future, the possibilities of Big Data Analytics in finance are endless and the financial institutions that embrace it will stay ahead in the competitive world of finance.
Data Forest is a leading Big Data Analytics enterprise solution provider. We help customers to solve business problems by bringing together different technologies including Machine Learning and Artificial Intelligence techniques, automation tools, Cloud solutions, and Big analytics solutions.
Our expertise lies in offering a complete end-to-end solution for the finance industry which includes data scraping, data science, data integration, web application development, and DevOps services.
DATAFOREST provides advanced Big Data Analytics solutions for financial markets, including:
- predictive analytics
- machine learning
- risk management analytics
- fraud detection analytics
- investment portfolio analytics
- customer segmentation analytics
- regulatory compliance analytics
- data governance
- real-time analytics
- data visualization
- financial performance analytics
- data security
- data integration
- financial forecasting
- financial statement analysis
- financial trend analysis
- financial benchmarking
- financial data mining

Conclusion
Big Data Analytics is an emerging field that has gained in importance in recent years. The techniques used by Big Data Analytics enable adopters to rapidly analyze large amounts of data to gain insights, which can be used to make better decisions.
The benefits of Big Data Analytics can include increased efficiency, productivity, better decision-making, reduced costs, and improved customer service. However, there are also challenges associated with this technology such as privacy concerns and security issues that need to be addressed before it can be implemented effectively.
DATAFOREST is a complete solution for Data Science consulting and outsourcing big data for businesses. With us, you get both the expertise of our team in multiple areas of big data and data science, and complete end-to-end support from Data collection to the finished product.
Contact DATAFOREST by filling out the form and start harnessing the power of Big Data Analytics in the finance industry!