The integration of modern technologies into traditional fields has the potential to revolutionize age-old practices, and one such combination that has garnered considerable attention is data science in agriculture. Data science for agriculture is increasingly becoming a transformative force, offering advanced analytics and predictive modeling techniques that help farmers optimize yields, manage resources, and adapt to changing environmental conditions. Interested in the update? Book a call and we'll tell you what's what.
By fusing data science techniques with agricultural practices, farming methods are being redefined for the 21st century, resulting in heightened productivity, lower costs, and more sustainable operations.
In this guide, DATAFOREST's data scientists will delve into the expanding role of Big Data in agriculture so you can discover its diverse applications in the field and examine some compelling success stories. Please keep reading!
Understanding the Significance of Data Science in Agriculture
With the integration of data science, agriculture gains access to actionable insights derived from comprehensive datasets. The utilization of advanced analytics empowers the sector to make data-driven decisions, improving efficiency and resource allocation.

Key Roles of Data Science in Agriculture
Data Collection: The Foundation of Agricultural Data Science
- Gathering data from diverse sources, including weather stations, soil sensors, and satellites.
- Real-time monitoring of climate conditions, soil moisture levels, and crop health.
Data Analysis: Unleashing the Power of Agriculture Data
- Advanced analytics uncover hidden correlations in datasets.
- Exploration of data through visualization tools, helping identify diseases and forecast market trends.
Predictive Modeling: Shaping the Future of Farm Data Science
- Leveraging machine learning models to predict crop yields, water requirements, and pest infestations.
- Enabling the development of automated irrigation systems and weather prediction algorithms.
Informed Decision-Making: Enhancing Farming Practices with Data Insights
- Empowering agriculture farmers to make informed decisions on crop selection, harvest timing, and resource allocation.
- Mitigating climate change impacts, adapting to weather patterns, and reducing yield losses to pests and diseases.
Paving the Way Towards Sustainable and Smart Farming
The Agriculture Data Act by Science, already in 2018, aims to promote significant data-oriented research in crucial areas such as supply chains, nutrition, and the environment. This initiative highlights the importance of harnessing the power of agricultural data.
Nowadays, researchers at Texas A&M are at the forefront of integrating new AI tools for plant analysis. Their work involves leveraging advanced technologies like sensors, robotics, big data, and controlled environment systems to develop an innovative imaging system specifically designed to study crops.
Furthermore, precision agriculture is making strides by incorporating big data technologies to optimize farming practices. These technologies, including detailed soil mapping and data-driven techniques, are used to optimize farming practices and enhance agricultural productivity.
It's important to acknowledge that these examples represent just a few recent news highlights in the field of data science in agriculture. Ongoing research and developments are continuously shaping this dynamic and transformative industry. By optimizing crop yields, improving resource efficiency, and enhancing decision-making, we can address the challenges of climate change and ensure a sustainable future for farming. By effectively applying data science tools and methodologies, we can unlock the full potential of agriculture and create a resilient and productive agricultural landscape. Select what you need and schedule a call.
Applications of Data Science in Agriculture: A Handbook to Smart, Sustainable Farming Practices
As the world population grows, the need for more innovative, efficient, and sustainable agricultural methods becomes increasingly critical. This section explores some of the most promising applications of data science in agriculture.
Precision Agriculture: The Apex of Data-Driven Farming Techniques
In today's agriculture sector, Precision Agriculture stands as a paragon of data science application in agriculture. By harnessing data from satellites, drones, and IoT sensors, this method allows farmers to receive hyper-targeted agronomic analytics. These analytics inform specific irrigation and fertilizer strategies, impacting soil moisture levels and overall crop health. This shift towards data-driven farming practices optimizes water usage and other resources and amplifies sustainability by minimizing environmental degradation.
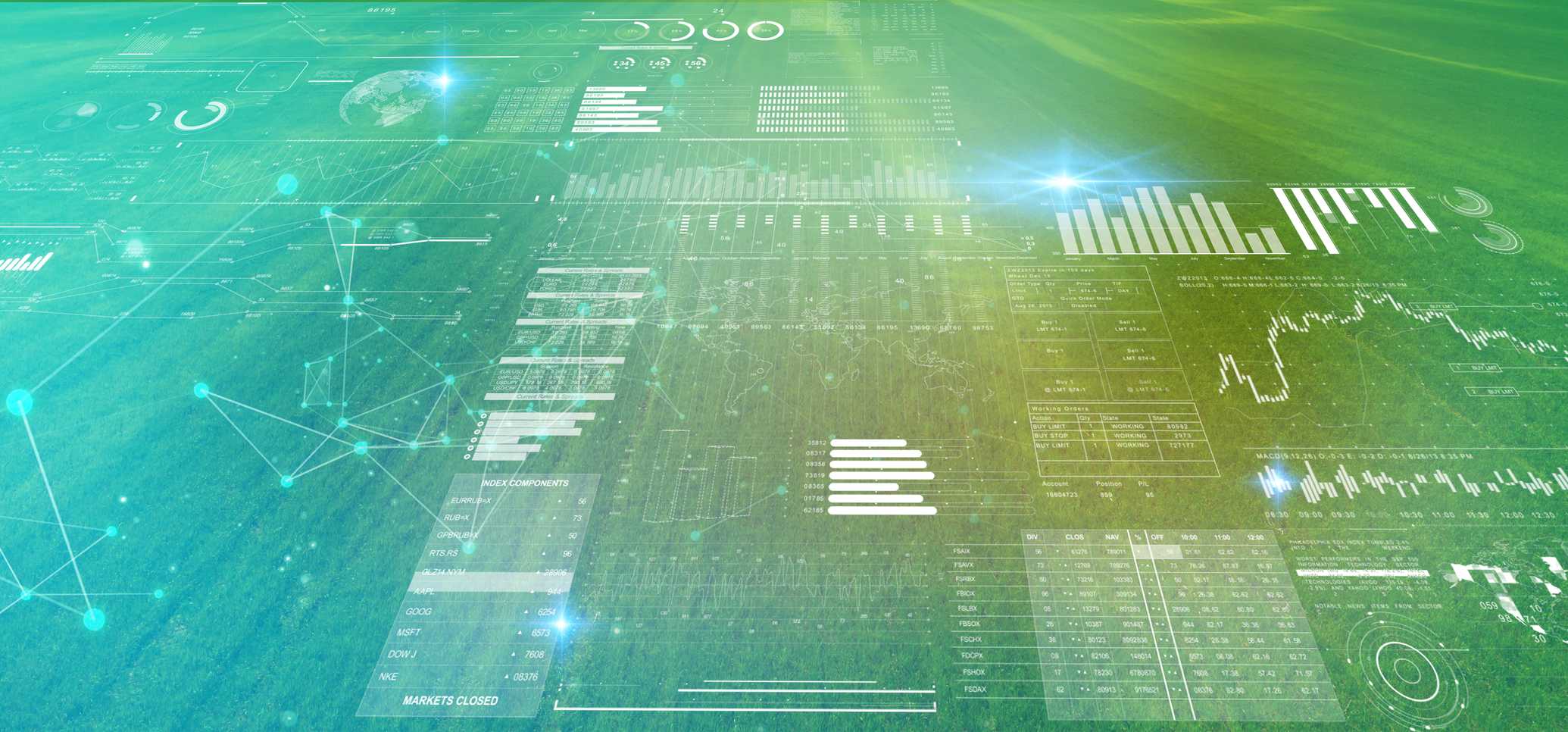
Remote Sensing & IoT: Innovating Crop Monitoring and Yield Prediction
The amalgamation of remote sensing technologies and IoT devices has revolutionized the role of data science in agriculture, particularly in crop monitoring and yield prediction. Real-time data on crop health, moisture levels, and environmental conditions is collected and processed through advanced data science algorithms. These predictive models enable farmers to make informed decisions regarding planting schedules, irrigation weather prediction, and marketing strategies. This proactive approach minimizes the risk of yield loss due to adverse weather patterns and diseases, enhancing overall productivity.
Livestock Management: A Data-Driven Paradigm Shift in Health Monitoring
Data science is equally transformative in livestock management, incorporating sensor data to monitor physiological parameters and animal behavior. Advanced data analytics algorithms enable early disease detection, thereby reducing the spread of diseases among livestock. This approach is a win-win: it mitigates treatment costs and boosts overall animal welfare, thanks to real-time alerts that inform veterinarians and farmers about anomalies in livestock behavior or health.
Climate-Resilient Agriculture: Leveraging Data Science for Weather Forecasting and Risk Mitigation
Weather patterns have always been unpredictable, but with climate change exacerbating these variations, the role of data science in agriculture becomes ever more critical. Sophisticated machine learning algorithms analyze vast datasets of historical weather data and current atmospheric conditions to generate accurate weather predictions. These predictions help farmers to adapt to the changing climate by making timely adjustments to their planting and harvesting schedules, irrigation plans, and crop protection measures.
Soil Health & Nutrient Management: Data-Driven Agriculture's Unsung Hero
Traditional soil analysis methods are getting a significant upgrade thanks to data science. Sensor technology and data analytics now provide an in-depth look into soil composition, nutrient content, and moisture levels. Such granular data allow for the application of precision farming techniques that optimize the balance of fertilizers in the soil, thus reducing waste and negative environmental impacts.
Supply Chain Excellence through Data Science: From Farm to Fork
Data science is essential in refining agricultural supply chain logistics, another pivotal application of data science in agriculture. Data science provides actionable insights for streamlining the entire supply chain by analyzing real-time and historical data on demand trends, transportation efficiency, and storage capacities.
This leads to improved resource allocation, reduced waste, and, ultimately, fresher produce for the consumer.
Data Science in Agriculture: Empowering Sustainable Farming with Innovative Tools and Technologies
Data science in agriculture has grown significantly in recent years, becoming essential to modern farming practices. This transformative shift can be attributed to various data science tools and technologies effectively deployed across agricultural applications. Indeed, the role of data science in agriculture is far-reaching, affecting everything from how we model and predict crop yields to how we manage resources to cope with changing environmental factors like rains, which can often reduce yields.
Data science provides ways to predict, understand, and mitigate factors contributing to yield reduction through its array of tools and technologies. These advancements, from machine learning algorithms to big data platforms, enable a more efficient, sustainable, and productive agricultural ecosystem.
Let's take a closer look at how data science is making waves in agriculture:
Machine Learning Algorithms: Precision in Prediction and Detection
Machine learning algorithms are fundamental in data science, especially in predicting crop yields and detecting diseases such as leaf rust and other foliar diseases. Machine learning models can use exploratory data to predict yields and assess the risks associated with various variables, including rainfall, temperature fluctuations, and soil conditions. Whether detecting early signs of leaf rust or estimating the impact of rainfall on yields, machine learning empowers farmers to take informed, proactive measures.
Geographic Information Systems (GIS): Gaining a Spatial Advantage
Spatial analysis is another area where data science in agriculture proves highly beneficial. Geographic Information Systems (GIS) allow farmers to evaluate land from a different perspective – space. These systems utilize satellite data and other geographical data points to generate graphs and maps that display variations in soil moisture, temperature, and other crucial variables across agricultural lands such as wheat farms. GIS enables precise decision-making for crop rotations, irrigation, and even identifying the most suitable land for specific crop types.
Big Data Platforms: Managing the Data Deluge
Data science in agriculture is incomplete without the power of big data platforms to handle the substantial amounts of data generated by sensors, satellite imagery, and other Internet of Things (IoT) devices. These platforms help store and analyze data to model future yields, identify potential risks, and assess the effectiveness of different farming practices. Commonly used technologies such as Apache Hadoop and Spark process and analyze vast agricultural data arrays, enabling farmers to make data-driven decisions.
IoT Devices: Real-Time Data Collection
The advent of Internet of Things (IoT) technology has revolutionized data collection in agriculture. IoT devices monitor variables such as soil moisture, temperature, and livestock health, providing real-time data essential for immediate decision-making. Whether determining optimal irrigation times or identifying signs of animal illness, these devices simplify farm management for farmers.
Data Visualization Tools: Making Data Understandable
The complexity of agricultural data can be overwhelming. That's where data visualization tools come into play. Tools like Tableau and Power BI transform raw, complicated data into understandable graphs and charts. This lets farmers and other stakeholders quickly grasp patterns and trends, facilitating better-informed decision-making.

The Road Ahead
Data science in agriculture is continually evolving, and its future looks promising with the integration of cutting-edge technologies such as artificial intelligence (AI) and edge computing. As we progress, machine learning models' predictive power is expected to improve, and real-time data processing will become more streamlined.
Data science in agriculture is no longer just a trend; it has become necessary for modern farming. With further improvement and integration of tools and technologies with the sector, data science in agriculture will undoubtedly play an even more critical role in shaping a sustainable and efficient global food production system.
Real-World Examples Overflowing with Applications of Data Science in Agriculture
The unification of data science in agriculture has manifested in significant advancements, spearheading innovations in farming methods and efficient resource allocation. This harmonious merger showcases a plethora of real-world applications where trends in data science in agriculture have truly revolutionized the field.
FarmLogs – A Milestone in Data Science in Agriculture for Precision Agriculture
FarmLogs, a pioneering platform, serves as an epitome of data science in agriculture, elevating the realm of precision agriculture. By amalgamating data science in agriculture, FarmLogs processes data from a multitude of sources like satellites, weather stations, and soil sensors to equip farmers with actionable, real-time insights. With the judicious application of data science in agriculture through FarmLogs, growers can fine-tune their planting timetables, water-saving irrigation techniques, and efficient fertilization methods. Consequently, data science in agriculture via FarmLogs leads to augmented crop yields, minimized water usage, and reduced fertilizer wastage, achieving the twin goals of heightened profitability and environmental sustainability.
Blue River Technology – An Epitome of Data Science in Agriculture for Weed Detection and Precision Spraying
As a subsidiary of John Deere, Blue River Technology leverages data science in agriculture to tackle the persistent problem of weed management. Their pioneering "See & Spray" technology is imbued with computer vision and machine learning algorithms, both pillars of data science in agriculture. This technology allows for real-time identification of specific plants, thereby enabling precise herbicide spraying that targets solely the weeds. Through this manifestation of data science in agriculture, chemical usage is diminished by up to 90%, thus mitigating environmental damage while curtailing operational costs for farmers.
Climate Corporation – Data Science in Agriculture for Accurate Yield Predictions
A division of Bayer, Climate Corporation offers a powerful testament to the role of data science in agriculture. Utilizing historical weather databases, intricate soil analytics, and machine learning—a core component of data science in agriculture—they furnish farmers with remarkably accurate yield forecasts. These data science in agriculture-driven insights capacitate farmers to make better-informed decisions regarding market strategies and resource allocation. As a result, the in-depth understanding afforded by data science in agriculture helps optimize planting choices and enhances overall farming productivity.

Ceres Imaging – Unleashing Data Science in Agriculture through Aerial Imagery for Crop Health
Ceres Imaging employs aerial photos taken by drones and airplanes, which are then analyzed using data science in agriculture methodologies to identify signs of crop stress, water insufficiency, and nutrient imbalances. By facilitating targeted interventions based on these data science in agriculture-derived insights, growers can foster healthier crops and elevate their yields. This strategic use of data science in agriculture minimizes wasteful resource deployment and maximizes agriculture's overall output.
In wrapping up, these tangible instances of the integration of data science in agriculture cases accentuate its pivotal role in amplifying productivity, fortifying sustainability, and escalating profitability in contemporary farming practices. As the sector keeps evolving, the application of data science in agriculture continues to validate its growing indispensability in modern agriculture.
A Comprehensive Overview of Barriers and Possibilities in Utilizing Data Science in Agriculture
The amalgamation of data science in agriculture is a transformative force with an enormous potential to reshape the agricultural sector. However, the journey towards full-fledged implementation of data science in agriculture encounters several roadblocks. This discussion is an insightful delve into the myriad challenges and opportunities associated with the unfolding narrative of data science in agriculture in modern farming practices.
- Data Privacy and Ownership in Data Science in Agriculture: The collection of agricultural data by technologies aligned with data science in agriculture brings essential questions regarding data ownership and privacy to the fore. Stakeholders, including farmers, are prompted to address these intricate issues while taking advantage of data science in agriculture for the twin goals of profitability and sustainability.
- Data Quality and Integration in the Sphere of Data Science in Agriculture: The varied channels agricultural data pours in—sensors, satellites, or machinery—pose unique challenges to data science in agriculture. Discrepancies and inaccuracies in data can be stumbling blocks for data science in agriculture, demanding meticulous data management methods to ensure data quality and harmonious integration.
- Limited Access to Technology: One glaring issue impacting data science uptake in agriculture is the restricted access to cutting-edge technologies, particularly in rural and developing locales. Bridging this digital gap is vital for the universal acceptance of data science in agriculture and ensuring equitable dissemination of its benefits.
While these challenges in integrating data science in agriculture serve as hurdles, they simultaneously offer avenues for further advancements. By navigating these obstacles effectively, the sector can witness the inception of more productive and equitable data management practices. Such progress could pave the way for more extensive utilization of data science in agriculture, culminating in a more productive and sustainable sector.
To sum up, the penetration of data science in agriculture brings an array of challenges that need to be meticulously navigated. However, by addressing key issues surrounding data privacy, data quality, and technological access, the potential for data science in agriculture to revolutionize the field becomes increasingly attainable. By overcoming these challenges, we can unlock the full capability of data science in agriculture, heralding a new era of innovation and sustainable growth in the agricultural domain.
Unlocking the Next Frontier: Future Developments and Advancements in Data Science in Agriculture — A Professional Business Perspective
The future of data science in agriculture is auspicious, with advanced technologies and methodologies poised to revolutionize the field further. Here, we delve into potential future developments that could elevate the role of data science in agriculture:
- Sophistication in AI and Machine Learning for Agriculture: As AI and machine learning evolve, their enhanced accuracy and predictive algorithms could offer real-time adaptations to fluctuating agricultural conditions, further embedding the importance of data science in agriculture.
- Blockchain for Robust Data Transparency: Blockchain technology could foster trust among various agricultural stakeholders by offering secure and transparent mechanisms for data sharing, a critical frontier for data science in agriculture.
- Edge Computing for Real-Time Decisions: Edge computing processes data locally, enabling immediate decision-making, and is integral for data science in agriculture, particularly in remote locations.
- Advanced Remote Sensing Technologies: Sophisticated remote sensing technologies like hyperspectral imaging offer nuanced data, revolutionizing how data science in agriculture contributes to precision agriculture.
- Collaborative Data Platforms: Future platforms could bring together farmers, researchers, policymakers, and agribusinesses in a standardized data-sharing environment, unlocking holistic solutions in data science in agriculture.
- Predictive Analytics for Pest and Disease Management: Advanced predictive models will likely aid in forecasting pest invasions and disease dissemination, helping farmers take preemptive actions to mitigate losses.
- IoT Integration for Comprehensive Data Science: The Internet of Things (IoT) stands to broaden the scope of data collection within data science in agriculture, offering real-time data on soil moisture, climatic changes, and livestock behavior.
- Data-Driven Sustainable Practices: Data science in agriculture can pave the way for more regenerative agricultural practices by analyzing intricate ecosystem dynamics and soil health.
As these tools and techniques in data science in agriculture mature, they will facilitate a more streamlined, sustainable, and efficient agricultural sector, addressing globally significant challenges related to food security and environmental stewardship. The forthcoming advancements in AI, blockchain, edge computing, remote sensing, and collaborative data platforms can navigate existing challenges and amplify the transformative role of data science in agriculture.
Summing up all of the above
Data-driven agriculture is revolutionizing the way we approach farming, ensuring sustainability and increasing productivity. At DATAFOREST, we are at the forefront of this agricultural transformation, providing customized data processing solutions that empower farmers and policymakers alike.
We understand the importance of data privacy and quality in agriculture, and our expertise allows us to enhance the value of agricultural data as a robust asset. With our data science services, we offer advanced predictive analytics in pest and disease management, providing timely insights for informed decision-making. Our innovative technologies, such as AI, blockchain, and edge computing, enable real-time adaptations to dynamic agricultural conditions.
In addition, we are committed to fostering collaboration in the agricultural industry by developing secure and standardized data-sharing platforms. We can collectively work towards sustainable agricultural practices by bringing together various stakeholders.
Contact us to cultivate a greener, smarter, and more bountiful future in agriculture. With DATAFOREST, you are not just adapting to the future; you are helping to shape it. Let's sow the seeds for a sustainable and prosperous agricultural industry that nourishes the planet for future generations.
FAQ
How Does Precision Agriculture Utilize Data Science for Resource Optimization and Yield Enhancement?
Precision agriculture combines data science with various data sources such as sensors, satellites, and weather stations. Through data science methodologies, this data is analyzed to create detailed field maps that reveal variations in soil quality, moisture levels, and crop health. By leveraging this information, farmers can optimize their irrigation and fertilization practices, reducing resource waste and improving efficiency. This precision in agriculture helps enhance crop yields by ensuring resources are applied precisely where and when needed.
How Do Data Science and Machine Learning Algorithms Contribute to Disease Prediction and Prevention in Crops?
Data science and machine learning algorithms are crucial in predicting and preventing crop diseases in agriculture. These algorithms can predict the likelihood of future disease occurrences by analyzing historical data on weather patterns, soil conditions, and previous disease outbreaks. This early warning system allows farmers to take proactive measures, such as adjusting planting strategies or implementing targeted pest control, to prevent or minimize the impact of diseases. Applying data science in agriculture helps reduce crop losses, minimize pesticide usage, and improve crop health and yield.
What Advantages Does Geographic Information Systems (GIS) Offer in Agriculture?
Geographic Information Systems (GIS) provide numerous benefits in agriculture and are vital to data science. By combining geographical data with satellite imagery, GIS creates detailed maps that reveal important information about soil properties and topography. Farmers can use this information to make informed decisions on planting, irrigation, and resource allocation, considering rainfall and weather fluctuations. GIS also supports optimal land usage, environmental impact assessments, and infrastructure planning, contributing to precision and sustainability in agriculture.
How Do Big Data and Analytics Amplify Supply Chain Efficiency in Agriculture?
Big data and analytics have a transformative impact on improving the efficiency of agricultural supply chains, showcasing the power of data science in agriculture. By analyzing vast amounts of data related to transportation, storage, and distribution, analytical tools provide valuable insights for decision-making. These insights help minimize waste, optimize resource allocation, and ensure fresh produce reaches consumers. Real-time data tracking adds transparency to the supply chain, facilitating smooth operations between farmers, distributors, and retailers.
How Does Data Visualization Assist in Interpreting and Applying Agricultural Insights?
Data visualization is an essential tool in agricultural data science, allowing complex data to be presented in a visual and easily understandable format. For example, a heatmap can show variations in crop health over time, enabling farmers to identify problematic areas in their fields. This visualization helps stakeholders make quick and informed decisions regarding interventions, such as weed control or managing produce weight. Data visualization promotes effective communication and collaboration among all agricultural stakeholders, leading to improved decision-making and outcomes.
Incorporating various data science applications in agriculture, from disease prediction to supply chain optimization, sets the stage for a future of enhanced efficiency, sustainability, and productivity in the sector.