Scaling AI is taking artificial intelligence (AI) models and effectively implementing them in the real world across a broader range of tasks, users, and applications. It's about building a powerful AI model and making it work seamlessly within a large organization or for a broader audience. Scaling AI is moving beyond a single project and integrating AI across various aspects of an organization. When scaling AI, the focus is on efficiently handling a larger volume of data and tasks. It's also ensuring that AI models are practical and can be effectively deployed in real-world scenarios. Picture you've built a powerful race car (the AI model). Scaling AI is turning this car into a production vehicle that can be manufactured efficiently, driven by a wider range of people, and functions on various terrains.

Leaders Scale More AI Use Cases More Quickly
Essential Steps for Scaling AI Systems
In scaling AI, building a robust AI foundation is constructing a sturdy and secure building before adding multiple floors. It's the crucial first step that ensures an AI system can effectively grow and handle the demands of real-world implementation.
The Pillars of Trust
- High-quality data is the first pillar of scaling AI. A solid data infrastructure ensures that the data used to train and run AI models is accurate, complete, and relevant. It involves:
Data Cleaning: Eliminating errors, inconsistencies, and missing values from the data.
Data Validation: Verifying the accuracy and integrity of the data.
Data Governance: Establishing procedures to ensure the consistent usability of data across the organization.
- Accessibility is the second scaling AI pillar. A well-stocked pantry wouldn't be helpful if locked away, so readily available data is crucial. A good data infrastructure provides:
Easy Access: Enabling authorized users to retrieve the data they need quickly and efficiently. This can involve tools for data discovery, self-service analytics, and robust search functionalities.
Standardization: Ensuring data is formatted consistently across different systems and departments makes it easier to integrate and analyze.
Scalability: The ability to handle increasing volumes of data as your needs grow.
- Unfortunately, data breaches and cyberattacks are a constant threat. A solid data infrastructure is a guardian at the gate, protecting valuable information within scaling AI. Here's how:
Encryption: Data encryption scrambles sensitive information, making it unreadable to unauthorized users even if it's intercepted.
Access Controls: Limiting access to data based on user roles and permissions helps prevent unauthorized access.
Data Monitoring: Monitoring data activity for suspicious behavior can help identify and mitigate potential security threats.
Cloud Computing and Scaling AI
Traditional on-premises data centers can be inflexible and expensive to scale up or down as your AI needs to evolve. Cloud computing offers a dynamic solution for scaling AI. You can access vast computing resources (like processing power and storage) on-demand, providing precisely what you need when needed. Training complex AI models often requires significant processing power. Cloud providers offer access to state-of-the-art HPC clusters, packed with high-performance CPUs and GPUs, that accelerate the training process. Cloud computing eliminates the upfront costs of maintaining physical hardware. You only pay for the resources you use, making it a budget-friendly option for organizations of all sizes.
Cloud platforms provide access to powerful virtual machines specifically designed for AI workloads. These pre-configured environments come equipped with the necessary tools and libraries, allowing models to be trained quickly and efficiently. Cloud storage solutions offer a secure platform to store massive amounts of data, which is essential for training and deploying AI models for scaling AI. Cloud providers also offer tools for data management, simplifying data cleansing and transformation.
Once trained, AI models need to be deployed into production environments. Cloud platforms provide tools for containerization and orchestration, making it easy to deploy and manage AI models at scale. Cloud services like serverless computing can be leveraged to deploy AI models without managing servers, further simplifying the process.
Cloud platforms facilitate collaboration among stakeholders in scaling AI and development. With an internet connection, teams can access and work on shared datasets and models from anywhere. Cloud providers are constantly innovating tools and services specifically designed for AI development.
Orchestration and Automation for Scaling AI
Teams unlock the true potential of scaling AI by embracing MLOps and automation. These tools empower them to manage complex AI deployments efficiently, achieve faster results, and gain an advantage in artificial intelligence.
The Scaling AI Conductor
Scaling AI integrates and manages a complex ecosystem of AI models, each potentially serving different purposes and interacting with various data sources. AI orchestration platforms offer tools to streamline the management of multiple AI models.
Workflow Automation: These tools automate the various stages of the AI lifecycle, from data pre-processing and model training to deployment and monitoring. This reduces manual effort when scaling AI and ensures consistency across models.
Resource Management: Orchestration platforms allocate resources like processing power and storage based on each model's specific needs.
Model Versioning and Governance: These tools track different versions of each model, enabling rollbacks for scaling AI if necessary. They enforce governance policies to ensure the responsible use of AI.
Monitoring and Observability: Orchestration tools provide centralized dashboards for monitoring the performance and health of all your AI models. They identify potential issues like bias or performance drift and trigger corrective actions.
Automation Advantage in Scaling AI
Scaling AI isn't just about building powerful models; it's about seamlessly integrating them into the real world. By automating repetitive tasks and streamlining workflows, organizations significantly accelerate AI deployment and integration into business processes, unlocking the true potential of AI at scale.
Automated Data Pipelines: They automate data cleaning, transformation, and feature engineering, ensuring consistent and high-quality data for training and deploying AI models.
AutoML and Hyperparameter Tuning: Leveraging automated machine-learning (AutoML) techniques reduces the time required for training, optimizing model performance efficiently and scaling AI.
Infrastructure as Code (IaC): IaC tools allow you to define and automate deploying and managing the infrastructure needed for your AI models. This lowers the risk of errors and simplifies scaling AI.
API Integration and Automation Platforms: These platforms enable seamless integration of AI models with existing business applications through APIs. Automation workflows trigger AI model predictions based on specific events or data changes within business processes.
Operational Efficiency with Scaled AI
These real-world examples showcase how AI orchestration and automation empower businesses to achieve operational efficiency with scaling AI.
Inventory Management with AI Orchestration
A retail chain struggled with maintaining optimal stock levels across a vast network of stores. Manual forecasting and ordering processes were inefficient and prone to errors, leading to stockouts and overstocking. The company implemented an AI orchestration platform that automated data collection and processing, training and deploying AI models for demand forecasting, and automated order generation and communication with suppliers based on predicted demand.
As a result of scaling AI, they have reduced stockouts, lower inventory carrying costs, and faster response times to changing market trends. The scalable solution has led to an expanding network of stores.
Quality Control with AI Automation
A manufacturing company faced challenges in maintaining consistent product quality with manual inspections. The process was time-consuming, prone to human error, and couldn't scale efficiently with increased production volume. The company deployed an automated AI system for visual inspection: high-resolution cameras and AI models trained on a vast dataset of defect-free and defective products and automated flagging of defective products.
It improved product quality by identifying defects with greater accuracy, increased production efficiency by reducing the time spent on manual inspections, and a scalable solution for handling higher production volumes without compromising quality. Also, reduce labor costs associated with manual inspections within scaling AI.
The Human Factor in Scaling AI
Scaling AI effectively requires a deliberate focus on building a robust talent pipeline and cultivating a company culture that fosters innovation and collaboration.
Assembling the Dream Team
- Data scientists transform raw data into valuable insights. They are adept at wrangling massive datasets, cleaning them, and extracting the hidden patterns that fuel AI models when scaling AI.
- AI engineers are the architects who bring these insights to life. They translate the data-driven discoveries into functional code, deploying the AI models that power intelligent applications.
- Domain experts bridge AI's abstract world and the industry's specific realities. They ensure that scaling AI addresses the challenges and opportunities of the specific domain.
Cultivating an AI-Fueled Mindset
A thriving AI-driven culture prioritizes continuous learning, encouraging employees to embrace new technologies, attend workshops, and stay abreast of the latest advancements. Breaking down silos between departments is essential. Working with data scientists, engineers, and domain experts will produce more effective solutions by scaling AI. Foster a collaborative environment where these diverse skill sets interact freely. Encourage employees to experiment with new AI applications, challenge the status quo, and not be afraid of failure. A culture that embraces calculated risks and fosters a "learn by doing" mentality is crucial in achieving breakthroughs.
Building AI Workforce
Attracting and retaining top AI talent is a competitive endeavor in scaling AI. Don't underestimate the potential of your existing workforce. Invest in training programs to equip current employees with the necessary AI skills. This approach strengthens loyalty and leverages existing institutional knowledge when scaling AI. Target AI specialists in your field. Offer competitive salaries, highlight your innovative culture, and showcase the impact their work will have. Building strong relationships with universities and research institutions is a valuable source for identifying top talent.
Scaling AI with Ethics and Governance
Responsible practices and robust governance frameworks are the cornerstones of trust when scaling AI. Biased data sets lead to discriminatory outcomes, so implementing robust data cleansing practices and fairness checks throughout the AI development lifecycle is essential.
Transparency is another cornerstone of ethical AI. Understanding how AI models arrive at their decisions is crucial for ensuring accountability. Scaling AI necessitates explainable AI (XAI) techniques that shed light on the inner workings of these models.
Privacy concerns also become prominent as AI collects and analyzes vast data. Strong data privacy practices, adhering to relevant regulations, and obtaining informed consent from users ensure ethical data collection when scaling AI.
Here are three key aspects of a practical AI governance framework:
- Well-defined roles and responsibilities for AI development and deployment are essential. This ensures accountability and prevents potential misuse.
- AI systems must comply with existing regulations and industry standards. Regular audits and risk assessments help mitigate potential compliance issues when scaling AI.
- Identifying potential risks associated with bias, privacy breaches, and unintended consequences is essential for responsible AI development.
Responsible use is critical to building trust with stakeholders—employees, customers, investors, and the public. By being transparent about how AI works and the data it uses, companies alleviate user concerns about "black box" algorithms. Taking ownership of the outcomes produced by AI systems demonstrates accountability. Focusing on developing AI solutions that address real-world challenges and create positive societal impacts reinforces the value proposition of scaling AI.
Scaling AI—Fueling Continuous Innovation
Scaling AI necessitates an agile approach that embraces continuous innovation. By staying fluid and adaptable and fostering a culture of experimentation, companies leverage AI as a springboard for ongoing improvement and market leadership.
The ability to adapt to market changes, technological advancements, and user feedback maintains a competitive edge. Markets are dynamic, and user needs evolve. Agile AI development allows companies to adapt solutions to address emerging market requirements. The field of AI is constantly in flux. Scaling AI development enables the integration of new techniques and tools. Agile methodologies allow for continuous feedback loops. User feedback is incorporated quickly, and AI solutions remain relevant.
A thriving AI development environment fosters a culture of experimentation and iterative improvement. Encouraging exploration and experimentation allows for the testing of new ideas and approaches. Scaling AI methodologies prioritize breaking down projects into smaller, iterative cycles. This allows for continuous refinement based on data, user feedback, and ongoing testing. A culture that embraces calculated risks and learns from failures fosters a more innovative environment.
No single entity possesses a monopoly on AI expertise. Building a collaborative ecosystem with partners significantly enhances scaling AI capabilities. Collaboration with other AI developers promotes cross-pollination of ideas. It also allows companies to leverage the complementary skill sets of different partners. This fills knowledge gaps and expedites the development process. Partnering with other players can enable access to shared resources, such as data sets and computing power, accelerating AI development and innovation.
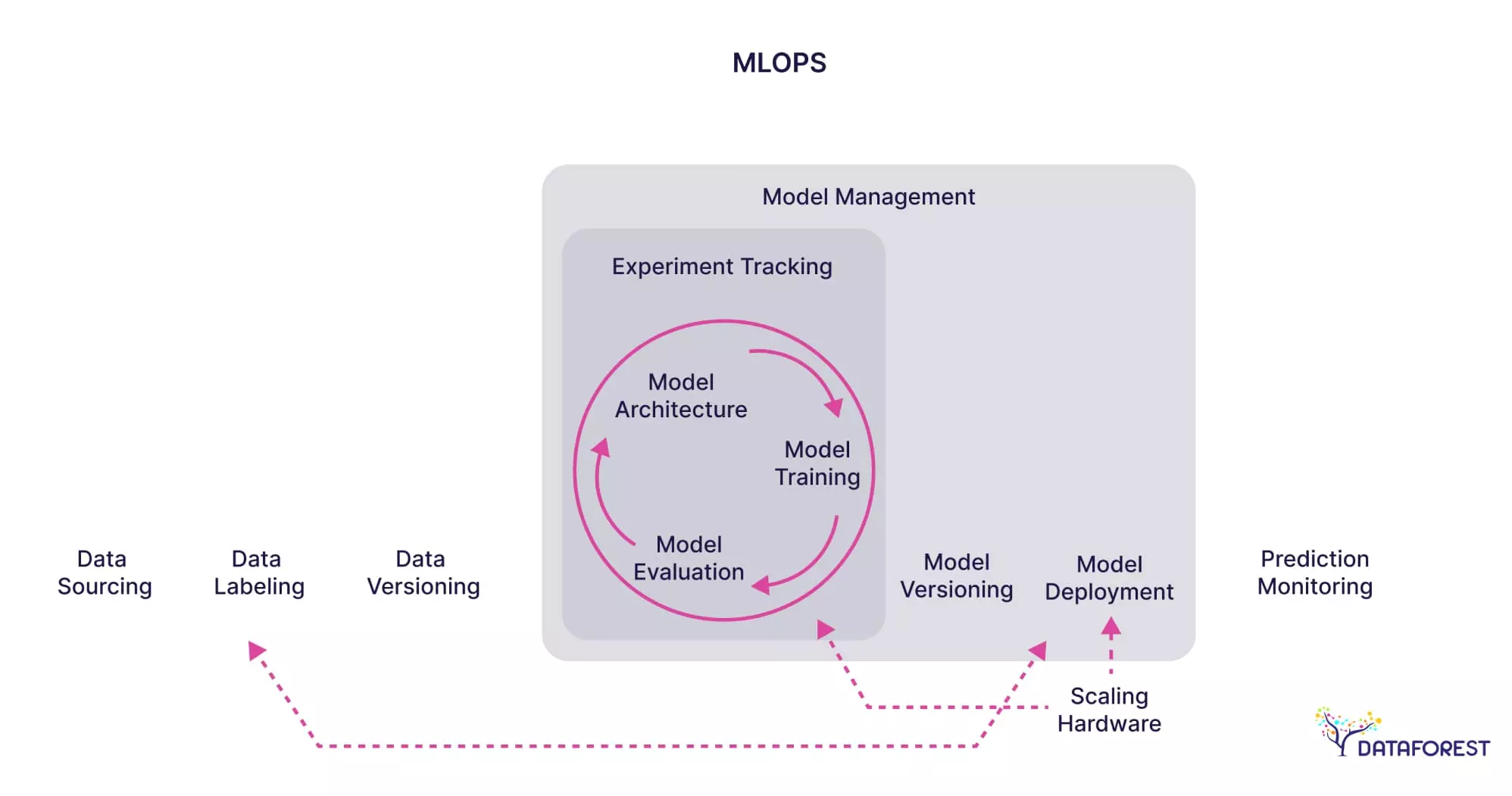
Scaling AI: A Multi-Faceted Approach
A tech provider addresses a business's pain points when scaling AI by offering a multi-pronged approach. As such, DATAFOREST bridges the gap by offering access to a team of skilled data scientists, AI engineers, and domain experts, alleviating the burden of internal recruitment and upskilling. The provider implements AI governance frameworks, ensuring compliance with regulations. It
also guides the business in adopting agile methodologies that promote continuous learning, iteration, and adaptation to market changes. The provider gives access to the necessary computing power, data management tools, and AI development platforms to handle the demands of scaling AI initiatives. Please fill out the form, and let's scale our relationship to the cooperation level.
FAQ
What are the first steps to scaling AI in my organization?
The first steps to scaling AI in your organization involve building a solid foundation. Ensure high-quality, secure data with clear access protocols. Then, explore cloud computing for its scalability and efficiency in handling the demands of large-scale AI initiatives.
How can I assess the readiness of my business for AI scaling?
To assess your AI scaling readiness, evaluate your data infrastructure. Ensure you have high-quality, well-organized data to access and manage efficiently. Consider your talent pool. Do you have the necessary expertise in data science, AI/ML engineering, and relevant domain knowledge to develop and maintain AI models?
What are the common pitfalls in scaling AI, and how can they be avoided?
Common pitfalls in scaling AI include relying on poor-quality data and needing a culture of experimentation. Avoid these by prioritizing clean, secure data and fostering an environment that encourages trying new ideas and learning from failures.
How do AI ethics and governance contribute to sustainable scaling AI?
AI ethics and governance ensure responsible development and use of AI, mitigating bias and privacy risks. This builds trust with stakeholders, fostering long-term adoption and success for scaling AI initiatives.
Can small to medium businesses also benefit from scaling AI strategies?
Small and medium businesses can leverage scaling AI strategies by focusing on pre-built, industry-specific solutions and utilizing cloud-based AI platforms that offer affordability and scalability, allowing them to compete with more prominent players.
What metrics should be used to measure the success of scaling AI initiatives?
When measuring the success of scaling AI initiatives, consider technical and business impact metrics. Track technical performance indicators like model accuracy and efficiency alongside business-oriented metrics like increased revenue, cost reduction, or improved customer satisfaction.