Generative AI is a branch of artificial intelligence focused on creating new data and content that mimics real-world examples, from text and images to music and video. It leverages advanced machine learning models to learn the underlying patterns and distributions of the data it's trained on. This enables the generation of novel outputs that, while entirely new, are indistinguishable from authentic data. The technology has many applications, including content creation, design, personalized experiences, and data augmentation, transforming industries by fostering innovation. If you think this is your case, then arrange a call. Generative AI also poses ethical and societal challenges, particularly concerning authenticity, copyright, and potential misuse. Despite these challenges, its development is being propelled forward by significant research and investment, reflecting its potential to revolutionize how we interact with digital content. As it evolves, generative AI blurs the lines between human and machine creativity.
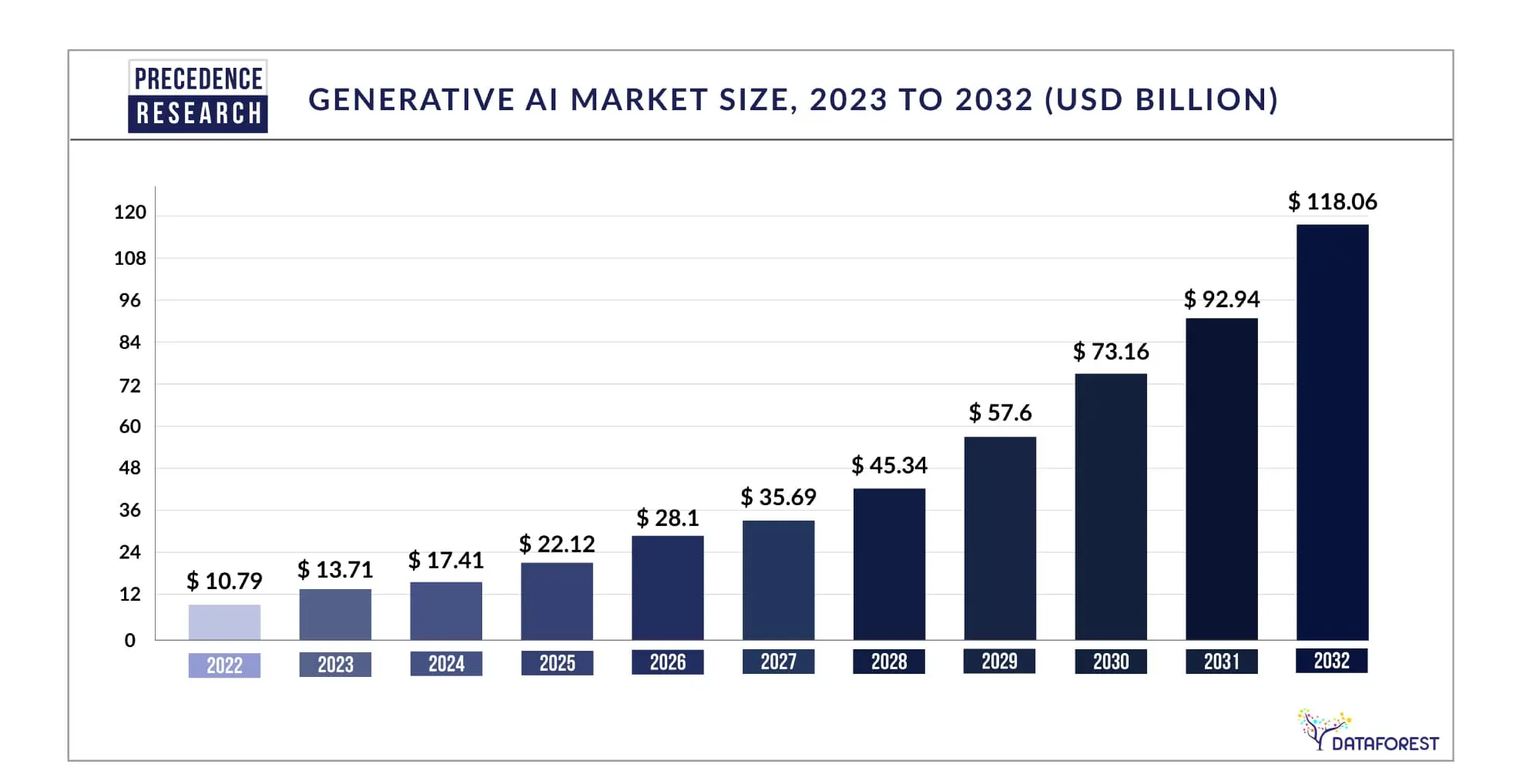
Generative AI—Creative Machines
Generative AI is a subset of artificial intelligence technologies designed to generate new data and content that mimic the characteristics of real-world examples. This includes but is not limited to text, images, music, videos, and even complex data structures. The core objective of generative AI is to understand and replicate the distribution and patterns of the input data it is trained on, enabling it to produce novel creations that resemble the original data convincingly.
Machine learning models such as Generative Adversarial Networks (GANs) and Variational Autoencoders (VAEs) are at the heart of generative AI. These models are trained on vast amounts of data, from which they learn the intricate patterns and structures inherent to the data set. GANs, for instance, operate through a dynamic process involving two models: a generator that creates data and a discriminator that evaluates its authenticity. This competition drives the generator to produce increasingly accurate representations of the original data.
Generative AI has the power to innovate, creating entirely new content that, while inspired by its training data, is original. This makes it invaluable across myriad applications, from digital art and music composition to the design of novel chemical compounds and the creation of realistic virtual environments. Gen AI is reshaping industries by enabling personalized content creation, automating design processes, and enhancing creative endeavors. It offers a blend of efficiency and creativity, opening up new possibilities for scale customization and exploring creative frontiers with reduced time and cost.
Types of Generative AI Models
Each of these models contributes uniquely to generative AI, offering tools to tackle complex problems by understanding and generating data in innovative ways. Their applications range from creating realistic images and personalizing user experiences to modeling language and enhancing predictive analytics. Schedule a call to complement reality with a profitable solution.
Generative Adversarial Networks (GANs)
GANs consist of two neural networks, the generator and the discriminator, which are trained simultaneously through adversarial processes for generative AI. The generator creates data (such as images) to mimic the training dataset, while the discriminator evaluates the authenticity of the generated data against actual data. This competition drives the generator to produce increasingly realistic outputs. GANs are particularly renowned for their ability to generate highly realistic images and videos.
Variational Autoencoders (VAEs)
VAEs are based on encoding input data into a compressed representation and then reconstructing the input data from this representation. Unlike traditional autoencoders, VAEs introduce randomness in the gen AI encoding process, generating a distribution for each encoded feature. This allows VAEs to generate new data points by sampling from these distributions. VAEs are widely used for tasks that require understanding the data's latent structures—image generation and anomaly detection.
Autoregressive Models
Autoregressive models generate data sequences by predicting each new piece of data based on the preceding ones. They operate under the assumption that the probability of observing a sequence can be decomposed into the product of conditional probabilities. Each element is predicted one at a time, with previous outputs serving as the context for subsequent predictions. This gen AI model type is used in natural language processing and speech synthesis, where the sequential nature of the data is crucial.
Flow-Based Models
Flow-based generative AI models, also known as invertible neural networks, learn data distribution through a series of invertible transformations. These models are designed to map complex data distributions to and from a simpler distribution, typically Gaussian. The invertibility ensures that no information is lost during transformation, allowing for the exact calculation of likelihoods and the generation of high-quality samples. Flow-based models are praised for their ability to efficiently generate data and calculate likelihoods, making them useful for tasks requiring detailed density estimation.
Energy-Based Models (EBMs)
EBMs learn a scalar energy function for each configuration of the input variables, where lower energy values are associated with more probable configurations. The model is trained to adjust the energy landscape so that observed data points are assigned lower energy (and thus higher probability) than unobserved configurations. Generating new data involves finding configurations that minimize the energy function, often through techniques like Markov Chain Monte Carlo (MCMC). With generative AI, EBMs are versatile and can be applied to various tasks—image generation, reconstruction, and denoising.
Foundation Models
They can be considered a type of Generative AI model with a broader scope and capability. Foundation models are large-scale machine learning models pre-trained on vast amounts of data, enabling them to understand and generate human language, among other capabilities. They serve as a foundational layer upon which various specific tasks can be performed, including but not limited to content generation, translation, summarization, and question-answering. The term is often associated with models like GPT (Generative Pre-trained Transformer), which can generate coherent and contextually relevant text across various domains. These models are trained on diverse internet text, allowing them to develop a broad understanding of human language, knowledge, and creativity. As such, foundation models encompass generative capabilities but extend beyond mere data generation to include tasks that require understanding, reasoning, and interaction.
Large Language Models
At a basic level, LLMs are a new way of representing language in a high-dimensional space with many parameters, a representation you create by training massive quantities of text. With large language models, we are taking lots of data and representing it in neural networks that simulate an abstract version of brain cells. Layers and layers of connections with trillions of parameters. And suddenly you can start to do some fascinating things. You can discover patterns so detailed that you can predict relationships with confidence. This word is most likely connected to this next word. These two words are most likely followed by a specific third word, repeatedly building up, reassessing, and repeatedly predicting until something new is written by generative AI.
Transformers
A transformer is an advanced machine learning model designed to handle sequential data, like text or time series, with a unique architecture focusing on relationships between elements in the sequence. It uses 'attention mechanisms' to weigh the importance of different parts of the data, allowing it to efficiently process and interpret large amounts of information within generative AI.
Transformers are the scaffolding that allows architects to build towering skyscrapers. They form the backbone of large language models like GPT (Generative Pretrained Transformer). By efficiently processing vast amounts of text data, they have enabled the creation of these 'foundation models'—large, general-purpose models that can be fine-tuned for a wide variety of specific tasks.
Diffusion Models
Diffusion models are a cutting-edge class of generative models that have rapidly ascended to prominence within artificial intelligence, particularly in image generation. At their core, diffusion models begin by adding noise to a dataset of images, gradually transforming them into pure, unstructured chaos. However, the true ingenuity of diffusion models lies in their reverse engineering prowess. They learn to meticulously remove this noise, step by step, revealing a coherent image that mirrors the characteristics of the original dataset for generative AI.

Statistics of the Generative AI adoption in business
How Generative AI Works
Generative AI operates through a sophisticated process that involves learning from vast datasets to produce new content that mimics the original data. This process can be divided into several key stages: data collection, model training, generation, and refinement.
Generative AI Principles and Algorithms
- The first step involves gathering a large and diverse dataset from which the model will learn. Depending on the desired output, this dataset could include text, images, audio, or video. The quality and variety of this data are vital, as they lay the foundation for the gen AI model's understanding.
- During training, the generative AI model is exposed to the collected data and learns to recognize patterns, structures, and relationships. This is where specific algorithms, such as Generative Adversarial Networks (GANs) or Variational Autoencoders (VAEs), come into play. These algorithms help the model approximate the input data distribution and understand how to generate similar data.
- Once trained, the gen AI model can start generating new content. This is done by sampling from the learned distribution or by taking an input (in the case of conditional generation) and producing an output that matches the characteristics of the training data. The generation process can be iterative, with the model refining its outputs based on feedback or additional inputs.
- The refinement stage involves fine-tuning the generated outputs to improve their quality, realism, or adherence to specific criteria. This can be achieved through further training, the application of additional generative AI algorithms, or manual adjustments by human operators. Refinement is critical for applications requiring high precision or those operating in sensitive contexts.
Examples of Applications
- Text Generation: Generative AI models like GPT can produce coherent and contextually relevant articles, stories, code, and poetry. This capability is widely used for content creation, automated journalism, and conversational AI.
- Image Synthesis: Using models such as GANs, generative AI can create realistic images and artworks from scratch or modify existing images. Applications include virtual fashion design, video game environment creation, and deepfake prevention.
- Music Composition: Generative AI can generate new music compositions by learning from a dataset of songs and understanding patterns in melody, rhythm, and harmony. This has implications for entertainment, therapeutic uses, and aiding composers in exploring new musical ideas.
- Drug Discovery: In the pharmaceutical industry, generative AI models can propose new molecular structures for drugs that are likely effective against specific diseases, accelerating drug discovery.
- Personalized Content: From customizing digital experiences to generating personalized learning materials, generative AI can tailor content to individual preferences and needs, enhancing user engagement and satisfaction.
Generative AI's applications, ranging from creative arts to scientific research, demonstrate its potential to mimic human creativity and extend it, offering solutions that were previously beyond reach.
Generative AI is Important for Businesses
Generative AI is transforming the business landscape by unlocking new creativity, efficiency, and personalization levels. Its importance is multifaceted, touching everything from innovation and content creation to bespoke customer experiences.
Creativity and Innovation
By generating novel ideas, designs, and solutions, gen AI enables companies to explore uncharted territories without the constraints of human bias or traditional thought patterns. This can lead to developing groundbreaking products, services, and business models, keeping companies at the cutting edge of their industries. For instance, generative AI can assist in designing unique product features or identifying new market opportunities, fostering a culture of innovation.
Content Generation
Generative AI streamlines the content creation, producing high-quality, engaging content at scale. Whether writing articles, creating marketing materials, or generating images and videos, gen AI can significantly reduce the time and resources required, allowing businesses to maintain a robust online presence. This is especially crucial for marketing campaigns, social media engagement, and keeping websites fresh and up-to-date.
Tailoring to Individual Preferences and Needs
Generative AI enables businesses to offer personalized experiences to their customers by understanding and anticipating individual preferences and needs. From personalized marketing messages to customized product recommendations, gen AI can analyze customer data to deliver highly targeted content and services. This enhances customer satisfaction and loyalty, increases the effectiveness of marketing efforts, and boosts sales.
Data Augmentation
Data is the lifeblood of the digital economy, but accessing high-quality, diverse datasets can be challenging. Generative AI can augment existing datasets, creating synthetic data that helps improve the performance of machine learning models without compromising privacy. This is particularly valuable in healthcare, finance, and autonomous driving, where real-world data may be scarce or sensitive. By expanding the breadth and depth of data available for training, businesses can develop more accurate and robust AI applications.
Problem-Solving
Generative AI offers powerful problem-solving capabilities, enabling businesses to efficiently identify and address complex challenges. By simulating various scenarios and outcomes, AI can help decision-makers explore strategies and their potential impacts. This can be applied to optimizing supply chains, improving product designs, or navigating legal and regulatory landscapes. The ability to rapidly generate and evaluate solutions can lead to more informed decision-making and a competitive advantage.
Generative AI’s Far-Reaching Benefits
This matrix highlights the diverse capabilities of generative AI and how they can be applied to yield tangible benefits for businesses. From enhancing creative processes and personalizing customer experiences to optimizing strategic planning and expanding market reach, generative AI is a pivotal tool in the digital transformation of industries.
Essential Criteria for Generative AI Providers
For a generative AI provider to effectively meet business needs, it must offer a suite of capabilities, support, and services that align with modern enterprises' diverse and evolving demands. DATAFOREST can deliver these essential aspects.
- A comprehensive portfolio of generative AI services, including text generation, image and video creation, design and prototyping, and data augmentation.
- Customization of AI models to suit specific business requirements, whether branding in content creation, specific design aesthetics, or unique data sets for model training.
- The generative AI content and data are highly accurate, minimizing the need for extensive human editing or correction.
- Gen AI solutions that can scale with the business, accommodating fluctuating demands and growing data volumes without compromising performance or speed.
- Data privacy and security standards, ensuring that all data used for training and generating outputs are handled in compliance with relevant regulations (e.g., GDPR, CCPA).
- Intuitive platforms and tools that non-technical users can navigate easily, reducing the barrier to entry for businesses looking to adopt generative AI.
- A collaborative approach to AI development allows businesses to provide feedback and request iterations on generated outputs.
- Ethical AI practices include transparency in how generative AI models are trained and used and providing controls to mitigate biases in generated content.
- Catering to the unique needs of different industries, offering specialized models and services for sectors such as healthcare, finance, marketing, and retail.
- Comprehensive support, training, and resources to help businesses effectively use and manage generative AI tools.
Please fill out the form; we will create new digital conveniences for your business.
FAQ
How can generative AI help my business stay ahead of the competition regarding content creation and customer engagement?
Generative AI can significantly enhance your business's content creation process by producing high-quality, diverse content at scale, allowing you to maintain a robust and engaging online presence without the extensive time and resource investment traditionally required. It enables personalized customer experiences by analyzing individual preferences and behaviors to tailor content, recommendations, and interactions, increasing customer satisfaction and loyalty. By leveraging gen AI capabilities, your business can swiftly adapt to market trends and customer needs, setting you apart from competitors with more dynamic, responsive, and personalized content strategies.
What are the potential risks associated with implementing generative AI in business operations?
Implementing generative AI in business operations can introduce risks, such as the potential for generating biased or inappropriate content due to underlying biases in the training data, raising ethical and reputational concerns. There's also the risk of dependency on gen AI content, which might compromise the originality and authenticity valued by customers. Additionally, ensuring data privacy and security becomes more complex as generative AI systems require access to vast amounts of data, heightening the risk of data breaches and misuse.
How can generative AI be integrated into our existing systems and workflows?
Generative AI can be integrated into existing systems and workflows through API connections, allowing seamless communication between AI models and your business applications for tasks like content creation, data analysis, and customer service enhancements. Customization and training of Gen AI models with your specific datasets ensure that the AI's outputs align with your business needs and maintain consistency with your brand's voice and standards. Additionally, adopting cloud-based AI solutions offers scalability and flexibility, enabling businesses to leverage generative AI capabilities without substantial upfront investments in infrastructure.
What skills or resources does my team need to leverage generative AI technologies effectively?
To effectively leverage generative AI technologies, your team needs a foundational understanding of gen AI and machine learning principles, enabling them to grasp how generative AI models work and their potential applications. Technical data science and programming skills, particularly in languages like Python, are crucial for customizing and integrating generative AI models into existing systems. Additionally, your team should possess data analysis and management skills to prepare datasets for training AI models and interpret the generated outputs for strategic use in business operations.