The efficient management and utilization of data is a cornerstone for businesses and organizations. This critical task is anchored in Data Life Cycle Management (DLCM), an exhaustive strategy that governs the total journey of data from its birth to its eventual retirement. DLCM surpasses mere data storage; it's an elaborate practice surrounding data creation, maintenance, use, and disposal. This procedure is essential for maximizing data value and ensuring its security and compliance. We know how to handle Big Data; arrange a call and you will know too.
The Business Intelligence (BI) market is projected to reach $44.34 billion by 2030, growing at a CAGR of 7.91% from 2023-2030, highlighting the increasing extent of data lifecycle management in diverse industries. The Data Center Life Cycle Services market report presents vast data on market introduction, segmentations, and expansion prospects. Comprehending DLCM is vital in our data-driven age. It allows organizations to handle their data efficiently, ensuring that practical information is handy, secure, and used effectively while adhering to legal and regulatory requirements.
In this piece, DATAFOREST will examine the various stages of the data life cycle, its management strategies, and its pivotal role in enriching business operations.
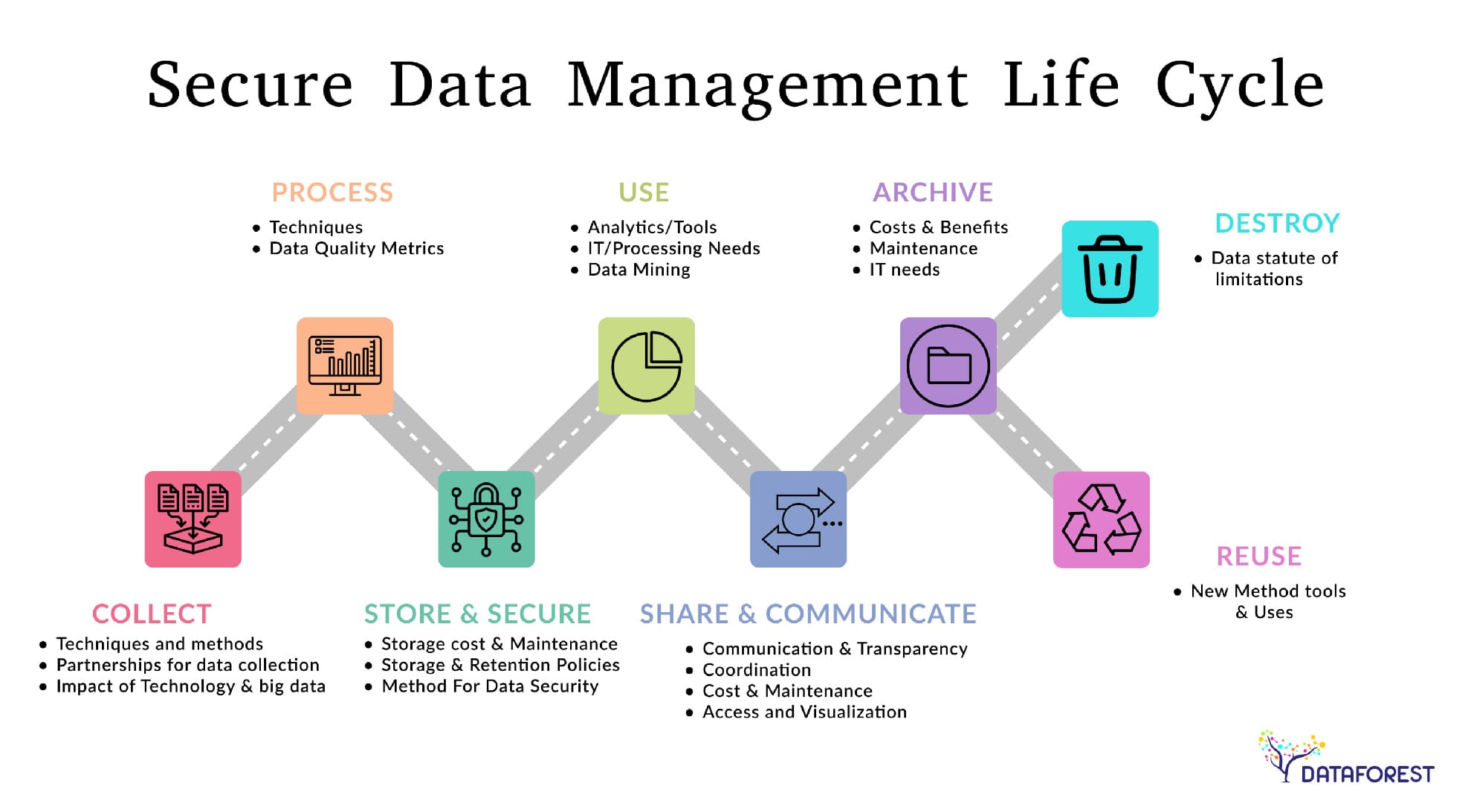
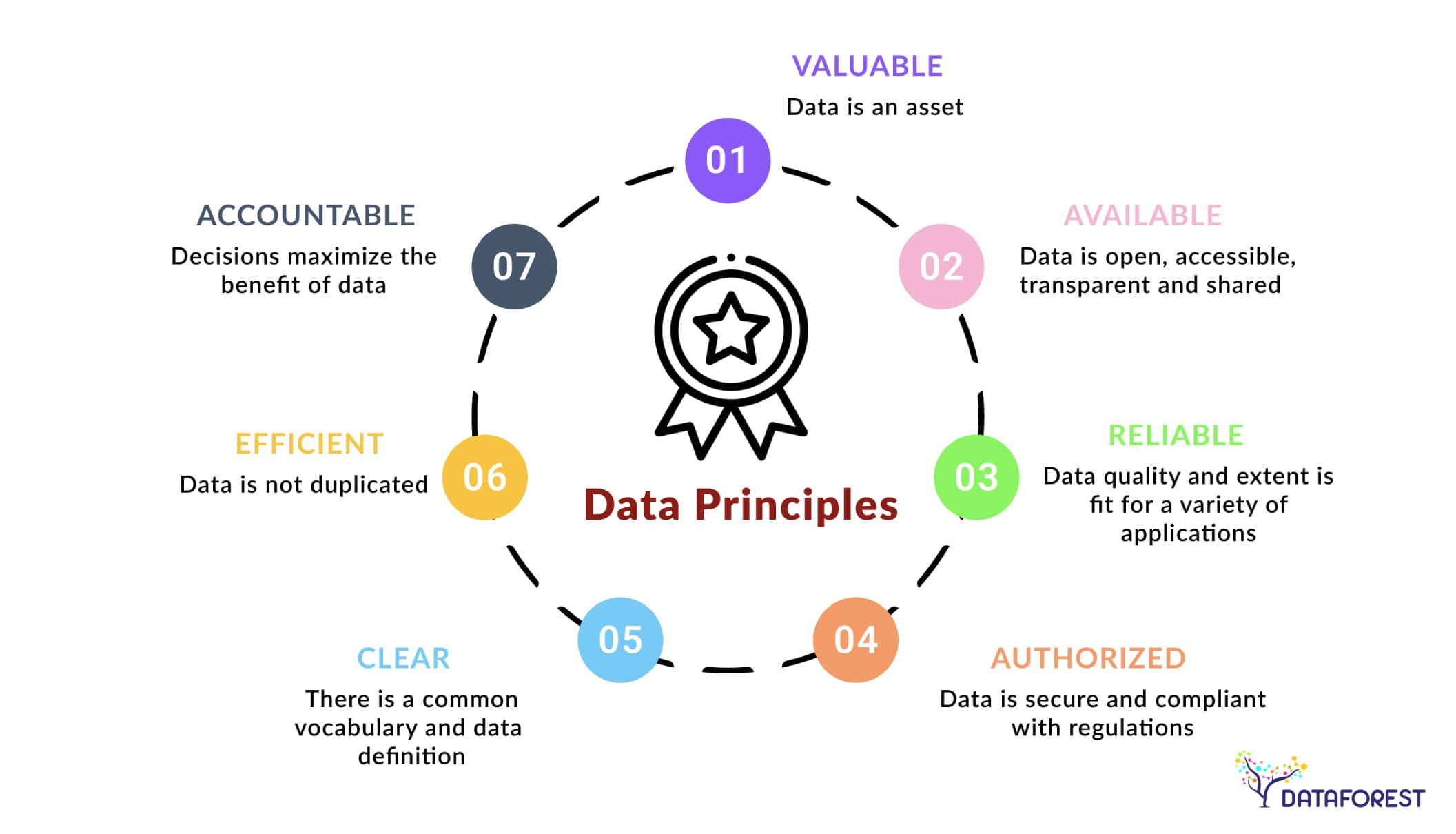
https://www.mdpi.com/2079-8954/11/8/380
Guiding the Data Life Cycle for Business Excellence
Exhaustive Resume of Data Life Cycle Management
Data Life Cycle Management (DLCM) embodies a structured methodology to administer data throughout its lifespan in a business setting.
This approach recognizes data as a critical asset, necessitating meticulous management at every phase. DLCM integrates diverse procedures and protocols to manage data flow within an organization efficiently, securely, and in compliance with regulations. It's a strategy centered around maintaining data integrity, accessibility, and protection while adhering to legal and ethical standards. If you want to always be on the cutting edge of technology, book a call.
The direct aim of DLCM is to maximize data utility, mitigate risks, and curtail costs linked to data management. It's a holistic view that extends beyond simple data storage, encompassing a comprehensive range of activities that enhance organizational value and stakeholder benefits.
6 Key Stages of Life Cycle in Data Science
The life cycle of data unfolds through several pivotal stages, each integral to effective data management. These stages are:
- Generation: The birth of data, either produced internally or sourced externally. This initial phase is about identifying the data's nature, format, and origin.
- Storage: Safeguarding the data in a secure, retrievable manner. Decisions here revolve around the storage location, format, and data redundancy measures.
- Utilization: Employing the data for its intended purpose, be it analysis, processing, or review.
- Distribution: Sharing data while respecting privacy, security, and adherence to data-sharing policies and legal requirements.
- Archiving: Preserving data not in active use but may be needed later for reference or regulatory compliance. Effective archiving ensures long-term data preservation and access.
- Disposition: Secure and definitive elimination or destruction of data when it's no longer necessary or legally mandated to retain.
Each stage demands diligent management to maintain the data as a valuable resource for the organization throughout its lifecycle.
Integrating Data Science Project Life Cycle Best Practices
- Embrace Cloud Solutions: Leveraging cloud technology for scalability, efficiency, and enhanced data storage and processing security.
- Implement Robust Data Governance: Establishing comprehensive governance policies for data quality, compliance, and lifecycle management.
- Optimize through Analytics: Utilizing analytics to derive meaningful insights from data, enhancing decision-making and business strategies.
- Prioritize Data Security: Ensuring encryption and robust security measures across the data science life cycle, from creation to disposal.
- Enhance Efficiency with Integration: Streamline the integration of various data sources and formats for a more cohesive data management approach.
- Focus on Scalability and Quality: Building scalability into the big data life cycle, ensuring high-quality data management even as data volume grows.
Data Life Cycle Management is key in ensuring efficiency and security in business. By meticulously managing individual stages of the data life cycle, the corps can protect their data assets and harness them as influential tools for growth and innovation.
Harnessing DLCM for Enhanced Business Performance
Revealing DLCM's Function in Streamlining Operations
Data Life Cycle Management is fundamental in enhancing operational efficiency, and by integrating a robust strategy, organizations seek precision in their data handling. Each phase of the data life cycle, from creation to disposal, is meticulously managed, ensuring data accuracy, accessibility, and security. Through DLCM, businesses can eliminate redundant processes, minimize errors, and maintain data consistency across all departments.
DLCM's automation aspect plays a crucial role in optimizing data processing. By automating the data life cycle, businesses can significantly reduce manual labor, saving time and costs. This automation enhances data quality, reduces human error, and facilitates quicker response to market trends and customer demands. Effective DLCM is a game-changer for businesses aiming for agility and competitive advantage.
DLCM's Influence on Decision-Making and Efficiency
DLCM's impact on business decision-making, resource allocation, and operational speed is substantial. Managing the data life cycle effectively equips decision-makers with high-quality, pertinent data, leading to more accurate and informed decisions. DLCM ensures that crucial data is readily available, enabling swift and efficient decision-making processes.
Resource allocation benefits greatly from DLCM. Organizations can strategically channel resources by understanding the significance and relevance of data at various stages of the data life cycle, focusing more on critical data aspects. This strategic approach to managing the data life cycle aids in cost optimization and boosts the return on data management investments.
Operational speed is another critical area where DLCM makes a marked difference. With streamlined data life cycle processes, data flows seamlessly within the organization, reducing delays and improving overall operational agility. This enhanced flow of information, governed by effective DLCM, allows for faster adaptation to both internal and external challenges, solidifying a business's dynamic capability.
Strengthening Business Through DLCM
DLCM: A Shield for Data Security and Regulatory Alignment
In the realm of business, Data Life Cycle Management (DLCM) stands as a sentinel for data security and compliance with regulatory standards. DLCM is pivotal in safeguarding data throughout its life cycle, ensuring adherence to various regulations like GDPR and HIPAA. Incorporating DLCM into business practices allows for systematic data handling, conforming to legal and industry norms.
The protocols in DLCM are designed to protect sensitive and personal data, ensuring that such information is accessible only to those with proper authorization and used in line with privacy laws. For instance, under GDPR, DLCM helps businesses protect personal data and uphold individual privacy rights by tracking data access and maintaining comprehensive audit trails. In the context of HIPAA, DLCM assures the secure storage, access, and sharing of healthcare data, preserving the confidentiality and integrity of patient information.
DLCM's Critical Role in Thwarting Data Breaches and Loss
DLCM's contribution to preventing data breaches and loss is invaluable. By managing the data life cycle effectively, organizations can mitigate the vulnerabilities that frequently lead to breaches. DLCM encompasses rigorous evaluations of data storage, access controls, and transmission methods, identifying and rectifying any security gaps.
Effective data life cycle management components include consistent backups and disaster recovery plans, which are essential for preventing data loss. These measures guarantee data recovery and business continuity in case of system failures or cyber-attacks. Additionally, DLCM's continual monitoring of the data life cycle aids in swiftly detecting anomalies that could signal breaches, enabling prompt action.

DLCM in Identifying and Mitigating Security Risks
Identifying and mitigating security risks is a cornerstone of DLCM. This process entails ongoing monitoring and analysis of data collection, storage, access, and disposal. DLCM aids in pinpointing patterns or irregularities indicative of security risks, allowing for preemptive action against potential vulnerabilities.
Regular revisions and updates to data policies and practices are integral to DLCM, ensuring resilience against evolving security threats and regulatory shifts. This dynamic approach keeps an organization's data management strategies robust and up-to-date. Furthermore, employee training and awareness programs, as part of DLCM, are essential in ensuring comprehensive understanding and compliance with data security responsibilities, significantly reducing the risk of data breaches.
Data Life Cycle Management Complexities
Overcoming Obstacles in Data Life Cycle Management Adoption
Adopting data life cycle management within an organization often encounters several challenges. A primary concern is the technical complexity involved. Establishing a data life cycle management system requires an in-depth understanding of diverse technologies and their integration into the existing IT infrastructure, a task that becomes more daunting as the volume and diversity of data proliferation.
Financial considerations are another significant barrier. The initial investment in technology, human resources, and training for effective data life cycle management can be substantial. For many smaller businesses, these costs can hinder the implementation of comprehensive data life cycle management strategies.
Change management also poses a critical challenge. Implementing data life cycle management typically necessitates substantial organizational processes and workflow changes. Employees may resist adapting to new data handling methods. Ensuring widespread acceptance and smoothly managing this transition is crucial for successfully adopting data life cycle management practices.
Strategies for Effective Data Life Cycle Management Implementation
To effectively navigate these challenges, several strategies can be employed:
- Begin with a Manageable Scope: Initially, implement data life cycle management on a small scale, focusing on critical data or key areas, then expand gradually. This approach helps mitigate initial costs and complexities.
- Embrace Cloud-Based Solutions: Cloud-based data life cycle management services can be more cost-effective and less complicated than building an in-house system. They also offer the benefits of scalability and flexibility.
- Prioritize Employee Training and Involvement: Educating employees about the importance of data life cycle management and involving them in the process can facilitate a smoother transition. Regular training and clear communication about changes are essential.
- Adopt a Phased Approach: Implementing data life cycle management in phases allows for ongoing assessment and adjustment, easing change management by giving employees time to adapt to new systems and processes.
- Consult with Experts: Seeking advice from data life cycle management experts or vendors can provide insights into best practices, helping to navigate technical complexities and tailor solutions to the organization's specific needs.
- Regularly Review and Update Strategies: The field of data management is constantly evolving. Regular reviews and updates to data life cycle management strategies ensure their continued effectiveness and compliance with current technologies and regulations.
Data Life Cycle Management & Technological Revolution
Arising technologies like Artificial Intelligence (AI), Machine Learning (ML), cloud computing, and the Internet of Things (IoT) are forming significant shifts. Each of these technologies brings unique enhancements and challenges to managing data life cycles.
- Artificial Intelligence's Role in Data Life Cycle Management: AI transforms data life cycle management by automating and enhancing analytics. Through AI, routine tasks in data life cycle management, such as data sorting, quality checks, and even archival processes, become more efficient and less prone to error. AI's analytical capabilities also offer deeper insights into data utilization, aiding in optimizing data life cycle processes.
- Cloud Computing's Impact on Data Life Cycle Management: Cloud computing reshapes data life cycle management by providing scalable, flexible data storage options. This technology enables businesses to handle large data volumes without extensive physical infrastructure. In data life cycle management, cloud services ensure data is stored securely and facilitate efficient data sharing and collaboration.
- Internet of Things and Data Life Cycle Management: The origin of IoT has drastically increased the volume and variety of data, presenting new challenges and opportunities for data life cycle management. Real-time data generated by IoT devices can be critical for businesses. Sufficient data life cycle management in this context involves rapidly processing and analyzing this influx of data and making swift decisions on data retention, analysis, or disposal.
These technological advancements are not just enhancing the efficiency of data life cycle management but also bringing forward new security, privacy, and compliance considerations. As data life cycle management evolves with these tech advancements, organizations must continually adapt their strategies to fully benefit from these innovations while effectively addressing the associated risks.
Establishing and Enhancing Data Life Cycle Management
Establishing or enhancing data life cycle management in an enterprise demands a well-organized approach. Beneath are key steps for businesses to navigate this journey effectively:
- Evaluate Existing Data Management: Initiate by assessing your organization's present data management state. Identify the nature of data collected, storage methods, usage, and disposal practices. This evaluation aids in pinpointing gaps in the existing data life cycle management.
- Set Data Life Cycle Management Goals: Clearly articulate your data life cycle management objectives. Goals may encompass enhancing data security, adhering to regulatory standards, or improving data accessibility and quality.
- Formulate a Data Life Cycle Management Strategy: Develop a comprehensive data life cycle management strategy based on your goals. It should detail policies, processes, and standards for data handling throughout its life cycle.
- Choose Suitable Tools and Technology: Select tools and technologies that align with your data life cycle management strategy. Options might include data management software, cloud storage solutions, or AI analytics tools.
- Implement Data Governance Policies: Establish clear data governance policies, defining roles, responsibilities, data standards, and protocols for data access, sharing, and security.
- Educate Your Team: Provide training for your staff on data life cycle management practices and the significance of data management. Ensure they comprehend the new policies and tools in use.
- Apply the Data Life Cycle Management Plan: Implement the data life cycle management plan across the organization, starting with pilot projects or specific departments and expanding organization-wide.
- Continuously Monitor and Update: Monitor the data life cycle management processes to ensure effectiveness. Update the strategy periodically to tackle new challenges or shifts in business requirements.
- Track Progress: Establish metrics to gauge the success of your data life cycle management. Metrics could include data quality indicators, compliance levels, and efficiency metrics.
Guidance on Tool Selection, Staff Training, and Progress Tracking
- Tool Selection: When choosing data life cycle management tools, consider scalability, system compatibility, user-friendliness, and security features. Tools with analytics and reporting capabilities are advantageous.
- Staff Training: Customize training sessions for different organizational roles. Focus on aligning training content with each group's specific data management responsibilities.
- Tracking Progress: Implement a dashboard or regular reporting system to monitor data life cycle management progress. This should involve key performance indicators that match your data life cycle management objectives.
Implementing an effective data life cycle management system is a progressive journey, requiring dedication and ongoing refinement. Following these steps, businesses can establish a solid data life cycle management framework that supports their objectives and adapts to evolving data management landscapes.
Effective DLCM strategies enable organizations to maximize their data's value, driving informed decision-making and maintaining competitiveness in a data-centric world. Although challenging, the path to proficient DLCM offers substantial security, compliance, and operational efficiency rewards.
DATAFOREST, a leader in the field and data science services vendor, is an ideal partner for this journey. Our expertise in data management and analytics, as well as AI and Machine Learning, can help businesses tailor and implement effective DLCM strategies. DATAFOREST's customized solutions ensure that organizations meet their data management requirements and utilize their data as a key strategic resource, spurring growth and innovation.
FAQ
Can small businesses benefit from implementing a data life cycle?
Small businesses stand to gain immensely from implementing a data life cycle management strategy. Integrating data life cycle management can lead to enhanced decision-making capabilities, improved data quality, increased operational efficiency, and adherence to regulatory standards. For small enterprises, data life cycle management means gaining a competitive edge through more nimble and informed market responses.
How do I assess my current data management processes and identify areas for improvement?
To evaluate your existing data management processes:
- Begin by tracing the journey of data through your organization.
- Scrutinize each phase of the data life cycle: collection, storage, usage, and eventual retirement.
- Search for inefficiencies, data security risks, or redundant processes.
Assessing aspects like data quality and policy compliance will spotlight areas ripe for improvement. Utilize tools such as internal audits and employee feedback to aid in this assessment.
How can data analytics be integrated into the data life cycle?
Data analytics can be seamlessly incorporated at various stages of the data life cycle. During the data collection phase, analytics play a crucial role in assessing the relevance and quality of gathered data. In the storage and utilization phases, analytics can shed light on data access patterns and usage, guiding better data life cycle management strategies. At the disposal stage, analytics can determine the continued value of data, aiding in decisions regarding archiving or deletion.
How does cloud computing impact data life cycle management?
Cloud computing significantly transforms data life cycle management by offering scalable and flexible data storage options. Adopting cloud services in data life cycle management brings advanced security measures essential for data protection. Furthermore, cloud computing enhances collaboration and streamlines data sharing, essential aspects of effective data life cycle management.
How often should a business review and update its data life cycle strategy?
Businesses should regularly review and update their data life cycle management strategies, ideally annually or in response to significant business or technological changes. These periodic assessments ensure the data life cycle management approach stays aligned with the company's goals, compliance demands, and technological progress. Adapting the data life cycle management strategy to address new challenges, like emerging security threats or shifts in data privacy laws, is also crucial.