Mastering data integration is a pivotal determinant for business success in today's data-centric world. This intricate procedure for synthesizing disparate data streams into a coherent framework propels organizations toward insightful decision-making, operational excellence, and competitive superiority. As the volume and complexity of data burgeon, the urgency for adept data integration escalates, underscoring its role as a critical infrastructural element in the digital business landscape. If you want to always be on the cutting edge of technology, book a call.
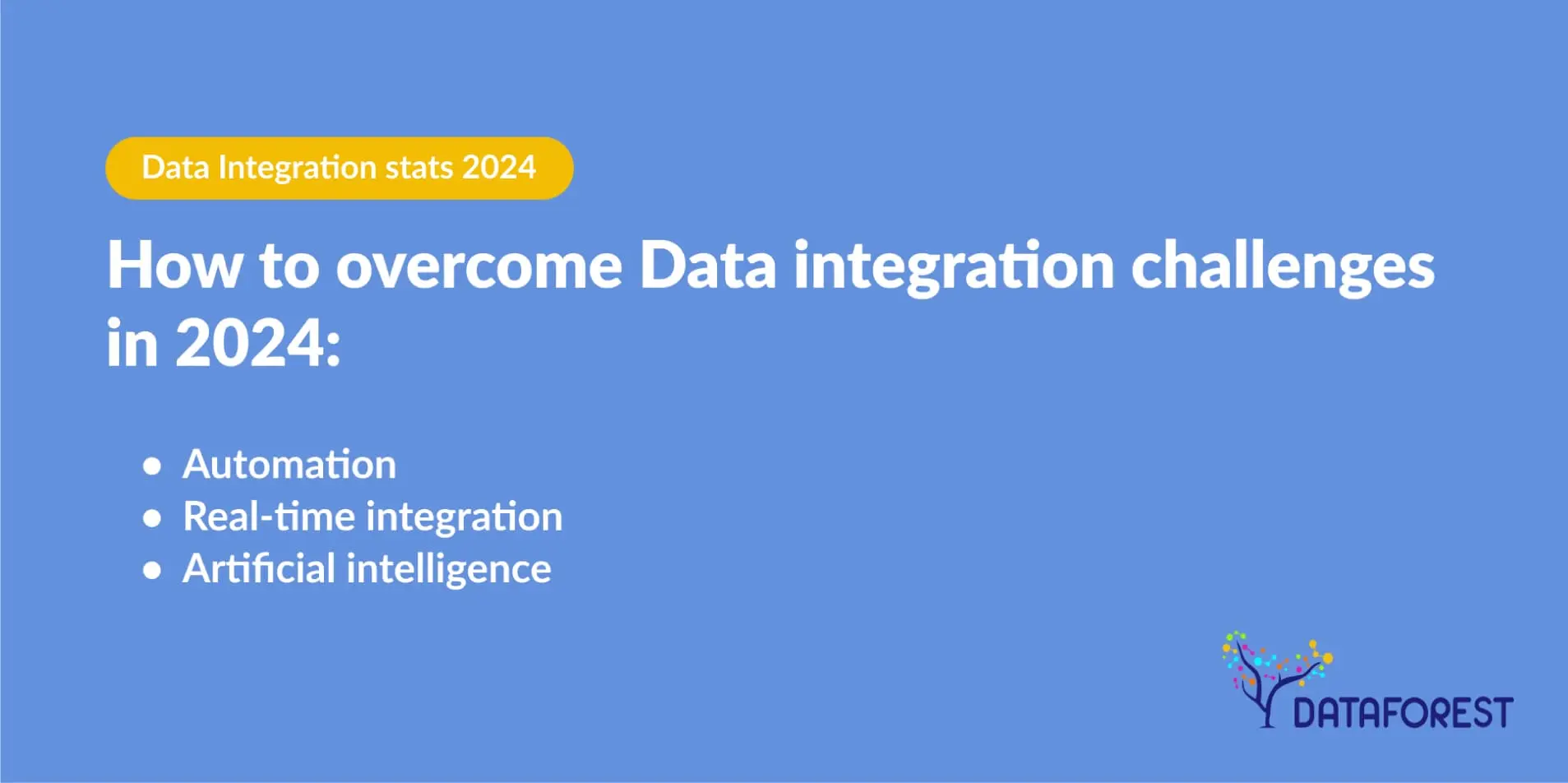
https://actioner.com/guides/data-integration-statistics
As of 2024, the data integration market is experiencing significant growth and evolution. The global market reached around USD 13.60 billion in 2023 and is expected to grow at a CAGR of 11.9% between 2024 and 2032, reaching approximately USD 37.39 billion by 2032.
In terms of data integration practices and strategies, a survey of over 600 Chief Data Officers (CDOs) reveals some key insights. The primary emphasis 2024 is on trusted data as a foundation for delivering trusted AI. For organizations to gain value from generative AI, data must be managed carefully, ethically, and responsibly. Another important aspect the CDOs highlight is the need for integrated data management solutions to facilitate AI adoption. Many organizations focus on enhancing data quality, privacy, integration, and engineering to improve overall data governance and streamline operations. These efforts are critical in supporting data strategy priorities and making the most of generative AI technologies.
The data integration process, in its essence, is the strategic amalgamation of data from varied origins, transformed and harmonized to forge a single, accessible repository. This endeavor stretches beyond mere data merging; it orchestrates aligning formats, rectifying disparities, and refining data quality. By navigating the intricate data integration steps, businesses unveil a streamlined, reliable data foundation conducive to strategic analysis and intelligence.
Deciphering the Data Integration Process
What is the Data Integration Process?
The data integration process, at its core, signifies the meticulous convergence of diverse data sets into a cohesive entity. This convergence encompasses the extraction of data from myriad sources, its transformation into a standardized format, and its ultimate consolidation. Such a process transcends simple aggregation, embodying the nuanced reconciliation of data characteristics to ensure uniformity and applicability. It's a comprehensive journey, integrating data cleansing, transformation, and enrichment elements, culminating in a singular, dependable data ecosystem. Select what you need and schedule a call.

Advantages of a Proficient Data Integration Process
Embarking on a proficient data integration project plan yields substantial rewards:
- Strategic Decision-Making: A consolidated data panorama empowers organizations with holistic insights, driving nuanced, data-informed decisions.
- Operational Streamlining: The efficiency gains from a unified data source are manifold, eliminating redundancy and optimizing resource allocation.
- Quality Data at the Forefront: Integral to the data integration process is data refinement, ensuring its accuracy, consistency, and trustworthiness.
- Enhanced Customer Understanding: Businesses can deeply understand customer dynamics through unified data, enhancing engagement and satisfaction.
- Adaptability and Growth: A well-architected pipeline data integration lays the groundwork for scalability, accommodating new data sources and evolving business needs with agility.
- Compliance and Governance: A standardized data repository aids in adhering to regulatory demands, reinforcing data governance frameworks.
The strategic execution of a data integration plan is not merely a technical endeavor but a business imperative. It enables organizations to navigate the intricacies of modern data landscapes with finesse, transforming raw data into a strategic asset.
Data Integration Steps
In data-driven decision-making, mastering the data integration process is paramount for organizations aiming to harness the full spectrum of insights and value from disparate data sources. This intricate process entails a series of meticulously orchestrated steps designed to unify, refine, and render data from diverse origins accessible for strategic analysis. Below, we delve into these pivotal data integration process steps, offering a roadmap for executing a thriving data integration process that elevates data utility and business intelligence.
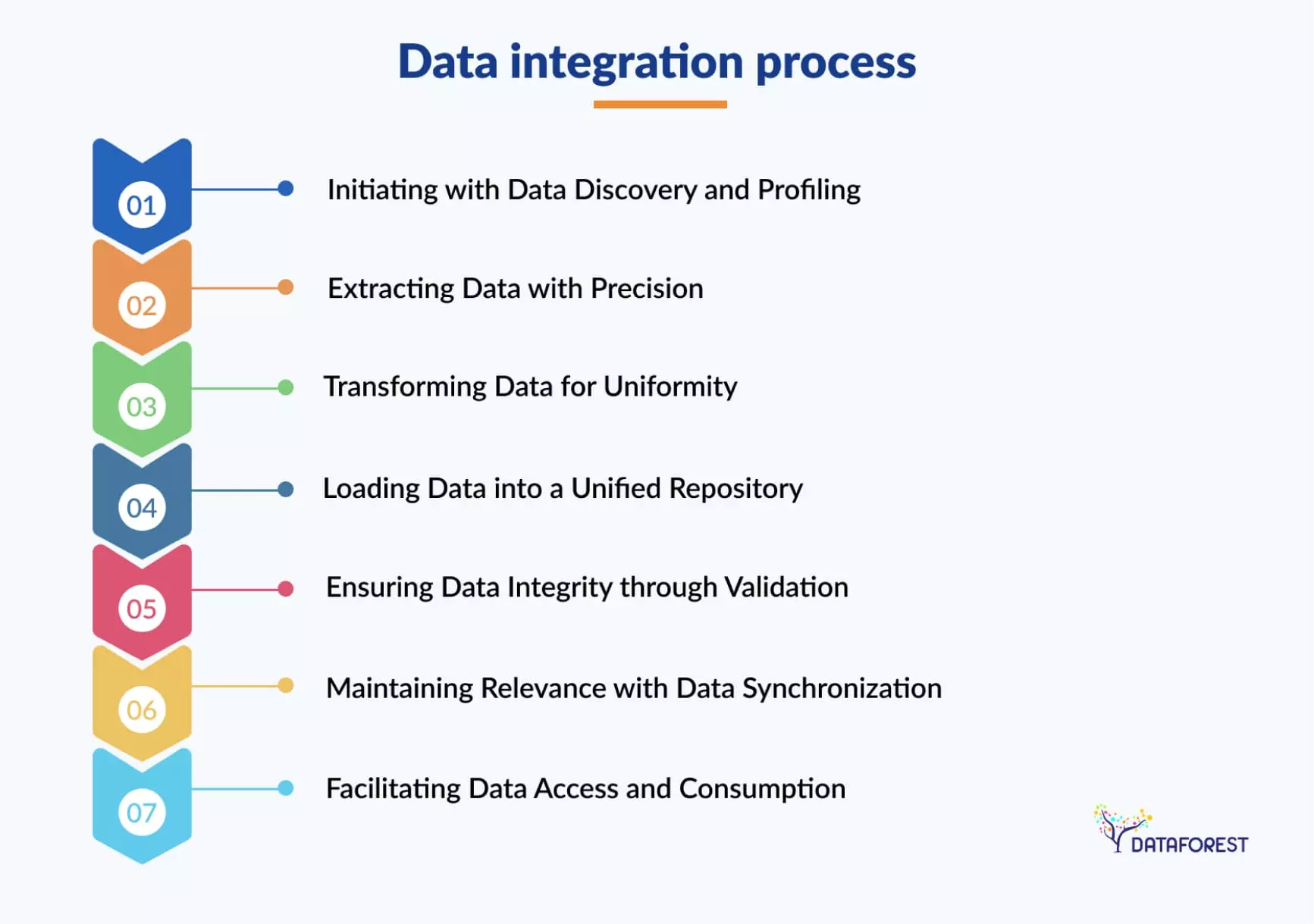
1. Initiating with Data Discovery and Profiling
The journey begins with data discovery and profiling, a foundational step in the data integration process, akin to charting the terrain before embarking on an expedition. This phase implicates a deep dive into the data landscape, identifying the myriad streams that feed into the organization's informational ecosystem. Here, the focus is on understanding the nuances of each data source—its structure, quality, and peculiarities—laying the groundwork for a seamless integration.
Imagine, for instance, a financial institution integrating data from diverse customer interaction points. The discovery phase would entail mapping data from online banking platforms, customer service interactions, and transactional systems, assessing each for format consistency, integrity, and completeness.
2. Extracting Data with Precision
Following discovery, the data integration process advances to data extraction. This critical step requires pulling data from its native repositories, which demands precision and sensitivity to avoid disrupting the source systems' functionality. The challenge lies in efficiently marshaling data from its silos, ensuring it's primed for the subsequent transformation phase.
Consider a multinational corporation consolidating operational data across its global subsidiaries. The extraction involves retrieving data from varied ERP systems, each with its distinct schema and data standards, preparing it for a unified corporate analysis.
3. Transforming Data for Uniformity
The heart of the data integration process lies in data transformation. The diverse data elements are standardized, cleansed, and harmonized in this stage, ensuring they speak a common language. This transformation is pivotal, turning a cacophony of disparate data into a harmonious dataset ready for integration.
An illustrative example could be an e-commerce giant integrating customer interaction data across multiple channels. Here, data from social media interactions, website visits, and purchase histories undergo normalization and deduplication, making it analyzable as a coherent whole.
4. Loading Data into a Unified Repository
With the transformation complete, data loading is the next step in the data integration process. This phase involves transferring the refined data into a central repository, such as a data warehouse, where it is stored, accessed, and analyzed. The loading must be executed to maintain data integrity and optimize for future access.
A retail chain could integrate sales data across its online and brick-and-mortar stores into a single data warehouse. This consolidation enables holistic sales performance analysis, inventory optimization, and customer data integration processes for behavior insights.
5. Ensuring Data Integrity through Validation
Data validation and quality assurance form the next critical phase in the data integration process. This step confirms that the integrated data is accurate, consistent, and high-quality. Rigorous validation checks are applied to detect and rectify any anomalies, ensuring the data's reliability for decision-making.
A healthcare provider integrating patient data across multiple care settings must ensure data accuracy and compliance with regulatory standards, validating patient information for consistency and completeness.
6. Maintaining Relevance with Data Synchronization
Given the dynamic nature of business data, synchronization and regular updates are indispensable steps of data integration. This ensures that the integrated data repository remains current, reflecting the latest changes from the source systems. Regular synchronization is crucial for maintaining the relevance and accuracy of the data.
For instance, a logistics firm integrating real-time tracking data from its fleet must regularly update its integrated data system to accurately reflect delivery statuses, vehicle locations, and logistical challenges.
7. Facilitating Data Access and Consumption
The culmination of the data integration process ensures that the integrated data is readily accessible for analysis and business intelligence. This involves making the data available in formats and through channels that support efficient consumption, analysis, and reporting.
A marketing firm analyzing consumer behavior across different campaigns would provide its analysts access to integrated data from various advertising platforms, enabling in-depth analysis to refine and optimize future marketing strategies.
Elevating Business Intelligence through Data Integration
Each step in the data integration process, from the initial discovery to enabling data access, is integral to transforming disparate data into a strategic asset. By meticulously navigating these steps, organizations can elevate their data utility, unlocking deeper insights and fostering informed decision-making.
Crafting a Data Integration Project Plan: A Strategic Framework
The cornerstone of any successful data integration initiative is a robust project plan. This plan is not just a schedule of tasks; it is a strategic blueprint that guides the orchestration of data integration steps, ensuring alignment with business objectives and operational requirements. The meaning of a data integration project plan lies in its ability to delineate the scope, define clear goals, allocate resources judiciously, and establish a realistic timeline, all while anticipating challenges and devising effective risk mitigation strategies.
Defining Objectives and Scope with Precision
A comprehensive data integration plan begins with clearly articulating objectives and scope. This clarity ensures that every step of the data integration process—from selecting the appropriate types of data integration approaches to deploying data integration pipelines—is aligned with the overarching goals. Whether the focus is on consolidating enterprise data from disparate systems or enhancing analytics through integrating IoT devices data, the objectives and scope set the direction for the entire project.
Resource Allocation: The Backbone of Execution
Effective resource allocation is crucial, integrating the expertise of data engineers, the capabilities of advanced software tools, and the infrastructure for data storage and processing. The delineation of team roles and the selection of technology for data propagation, data processing systems integration, or data analysis form the backbone of the project's execution plan.
Timeline and Milestones: Mapping the Journey
The data integration process is a journey marked by significant milestones, from the initial data extraction to the intricate steps of transformation and loading. Establishing a timeline that reflects both the complexity of tasks, such as data virtualization and meta data management, and the necessity for iterative refinement is paramount. This timeline serves as a tool for tracking progress, aligning expectations, and facilitating stakeholder engagement.
Budgeting: Fueling the Project's Success
A detailed budget, encompassing everything from software licensing fees to costs associated with manual data preparation, is essential. It ensures that the data integration project has the financial backing necessary to address anticipated needs and unforeseen challenges, securing its viability.
Risk Management: Safeguarding the Project
The data integration process needs to be improved, from the technical hurdles of integrating diverse data formats to the organizational challenges of ensuring data quality. A proactive approach to risk management, identifying possible impediments and crafting contingency plans, is indispensable. This foresight minimizes disruptions and ensures the project remains on track toward its goals.
From Theory to Practice
From the intricacies of data extraction, which aims to seamlessly retrieve data from varied sources without impacting source system performance, to the challenges of data transformation, where disparate data formats are standardized and cleansed, each step demands a strategic approach. The deployment of data integration pipelines, leveraging both traditional ETL (extract, transform, and load) and modern data processing integration techniques, exemplifies the blend of art and science that is data integration.
What is the relationship between data pipelines and data integration? These pipelines are not merely conduits for data flow; they are the arteries of the data integration process, enabling the dynamic movement, transformation, and delivery of data across the enterprise. This synergy emphasizes the importance of a holistic view of the data integration process, recognizing the interdependencies and the need for coordination across various data integration life cycle stages.
Organizations can navigate this complexity by embracing a strategic approach to planning and executing data integration projects, transforming disparate data sources into a cohesive, powerful asset that drives informed decision-making and strategic advantage.
This complex undertaking involves many steps, requiring a comprehensive data integration project plan to ensure a seamless, efficient fusion of data streams. A successful data integration process's essence lies in meticulously selecting various data integration approaches, each tailored to specific business needs, data volumes, and operational demands.
Delving into Data Integration Approaches
The data integration process offers a spectrum of approaches, each with its distinct methodology and potential impact on the business’s analytical capabilities and operational agility. Understanding these approaches is critical for formulating a data integration plans that aligns with organizational goals and data strategies.
Batch Integration
Batch integration is a foundational element of the data integration process, tailored for scenarios where real-time data is not paramount. This approach, integral to the data integration process, involves aggregating data at predetermined intervals, thus optimizing for efficiency and minimizing the load on source systems. Despite its simplicity, the batch integration method within the data integration process may introduce delays in data availability, posing challenges for time-sensitive decision-making.
Real-time Integration
The data integration process is significantly enhanced by real-time integration, which ensures immediate data availability, a critical requirement for operations demanding up-to-the-minute data. This facet of the data integration process is essential for enabling dynamic decision-making and operational responsiveness. However, the complexity and resource intensity of real-time integration within the data integration process must be considered.
Hybrid Integration
Hybrid integration represents a strategic blend within the data integration process, combining the strengths of batch and real-time methods to offer a versatile solution tailored to diverse business needs. This approach enriches the data integration process by providing flexibility, allowing organizations to balance immediacy and efficiency based on specific data integration conditions.
Cloud-based Integration
Cloud computing has introduced cloud-based integration into the data integration process, offering scalability, cost-efficiency, and remote accessibility. This approach has become increasingly relevant in data integration, especially for organizations with geographically dispersed teams requiring access to integrated data. Data security and internet dependence are inherent challenges within this data integration approach.
Middleware and ETL Tools
Middleware and ETL (Extract, Transform, Load) tools are pivotal in the data integration process, facilitating the seamless extraction, transformation, and loading of data. These instruments are central to data integration, automating and simplifying complex data transformations and integrations across various systems. While indispensable for data integration, these solutions may require significant investment and specialized skills.
The data integration process, with its manifold approaches and methodologies, is a strategic imperative for businesses aiming to harness the full potential of their data assets. Whether through batch, real-time, hybrid, cloud-based, or middleware and ETL tools, data integration is fundamental for consolidating data streams, enabling advanced analytics, and driving informed decision-making. Crafting a data integration project plan that thoughtfully selects among these approaches is crucial for navigating the complexities of data integration, ensuring a cohesive, efficient, and effective integration result. This strategic engagement with the data integration process ultimately empowers organizations to achieve a competitive edge in the data-centric business environment.
In-Depth Strategies for Addressing Challenges in the Data Integration Process
To thoroughly understand and effectively manage the intricacies of the data integration process, it is crucial to delve more in-depth into the challenges and explore more comprehensive strategies. The following table presents an expanded and detailed view of the common hurdles in the data integration process, along with an enriched strategy for overcoming them:
To summarize the above
In the intricate landscape of data-driven decision-making, mastering the data integration process is a cornerstone of success for our organization. Confronting challenges such as data silos, integration complexity, and data inconsistency, we employ strategies and tools that significantly streamline the data integration process. By focusing on collaboration, standardization, and leveraging our expertise, we transform these challenges into opportunities, enhancing decision-making and operational efficiency. This proactive approach is fundamental in laying a solid foundation for analytics and business intelligence initiatives within the data integration process.
At DATAFOREST, we specialize in navigating the complexities of the data integration process. Our deep understanding of state-of-the-art integration technologies and data management positions us to offer customized solutions that effectively address the typical challenges encountered in the data integration process. Our commitment ensures that organizations benefit from high-quality, consistent, actionable data.
Tackling data silos within the data integration process, we focus on integrating disparate data sources. Our comprehensive blog post explores strategies and best practices to overcome this challenge, ensuring a seamless flow of information and thus enhancing the data integration process.
In addressing integration complexity, we demonstrate our proficiency in simplifying this critical aspect of the data integration process. Our insights into managing and integrating complex data from various sources ensure a smooth and efficient data integration.
We recommend employing advanced data cleansing tools and techniques for data inconsistency issues within the data integration process. Our guidance on choosing the right tools, akin to selecting dishes from a menu, is detailed in our informative blog article, providing valuable insights for enhancing the data integration process.
For more information about our solutions and expertise in the data integration process, interested parties are encouraged to contact us directly. Our team is equipped to offer comprehensive assistance and tailored solutions, ensuring your data integration process is streamlined and effective.
Partnering with us at DATAFOREST means navigating the intricacies of the data integration process with greater confidence and efficiency, setting the stage for data-driven success.
FAQ
How can I ensure data quality during the data integration process?
The data integration process must prioritize data quality to ensure reliability and accuracy. Implementing a robust data validation framework is essential in this process. Using data cleansing tools to rectify inaccuracies forms a cornerstone of this strategy. Automating quality checks within the data integration process helps maintain data integrity. Comprehensive data governance policies further fortify the data integration process against quality issues. Additionally, regular audits of the data post-integration are indispensable for maintaining quality and consistency throughout the data integration process.
What is the relationship between data pipelines and data integration?
Data pipelines are the lifelines of the data integration process. They act as conduits via which data flows from its sources to the target system. In the data integration process, these pipelines automate the movement and transformation of data. They ensure data is efficiently integrated into the desired format and location. This automation facilitates analysis and decision-making, underscoring the critical role of data pipelines in the data integration process.
What are the key considerations when choosing a data integration approach for my organization?
Several factors must be considered when determining the most suitable data integration approach. The volume and sophistication of data play a significant role in the data integration process. Assess whether the data integration process needs to accommodate real-time processing. Budget constraints and the existing IT infrastructure also influence the choice of the data integration method. Evaluate whether batch, real-time, or hybrid integration best suits your data needs. Cloud-based solutions should be considered for their scalability and flexibility in data integration.
What role does data mapping play in the data integration process?
Data mapping is a critical component in the data integration process. It serves as the blueprint for how data from various sources is related and transformed. In the data integration process, mapping involves outlining the relationships between data fields in the source and target systems. This step is crucial for assuring accurate and effective data translation and consolidation, making data mapping an indispensable part of data integration.