The concept of "big data" has transcended its buzzword status to become an indispensable asset for driving innovation and competitive advantage.
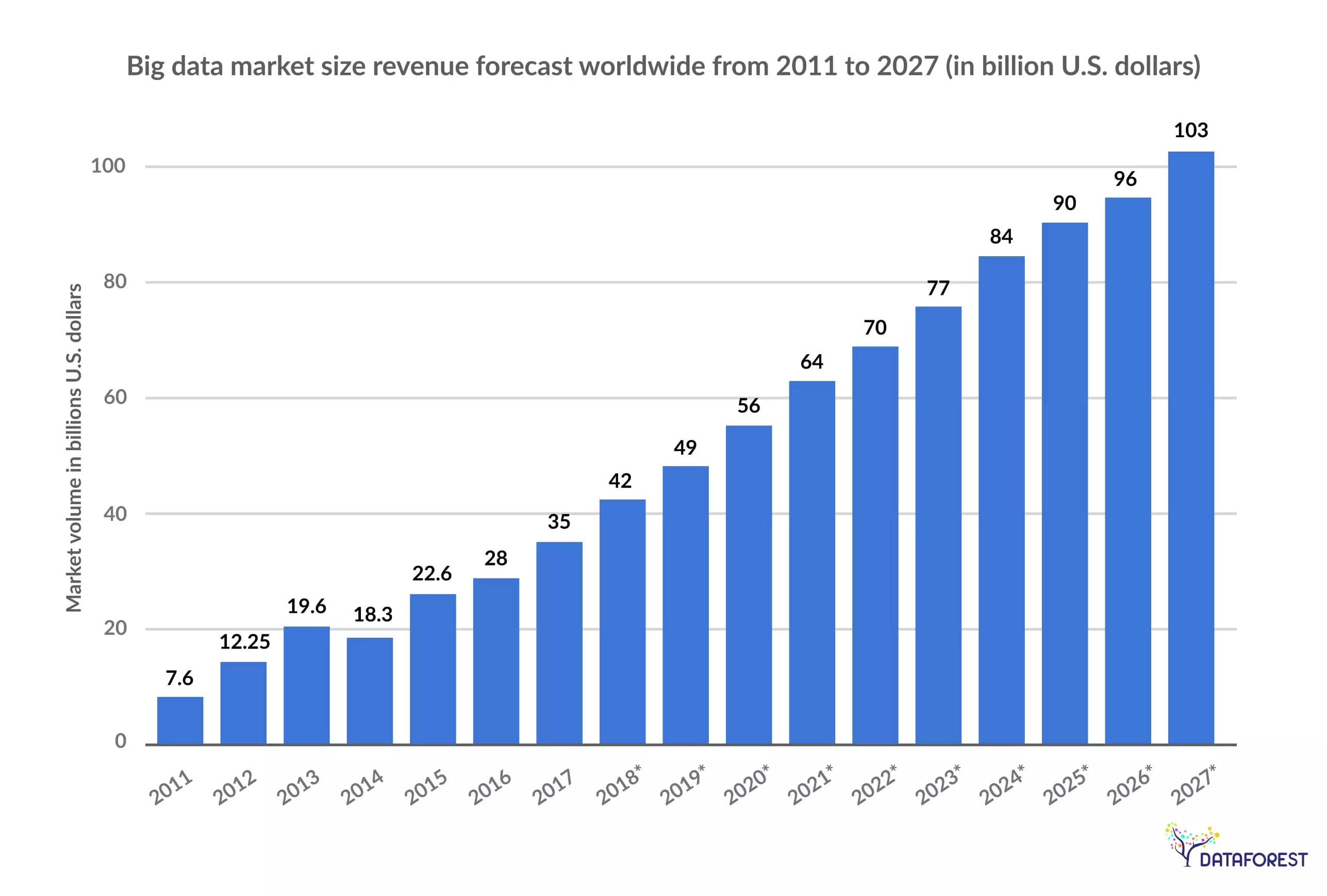
Source: Statista
With the big data analytics market on track to burgeon to $349.56 billion by 2024 and the anticipation of global data generation reaching a staggering 181 zettabytes by 2025, the criticality of big data integration in the strategic arsenal of businesses is more pronounced than ever. We know how to handle Big Data; arrange a call and you will know too.
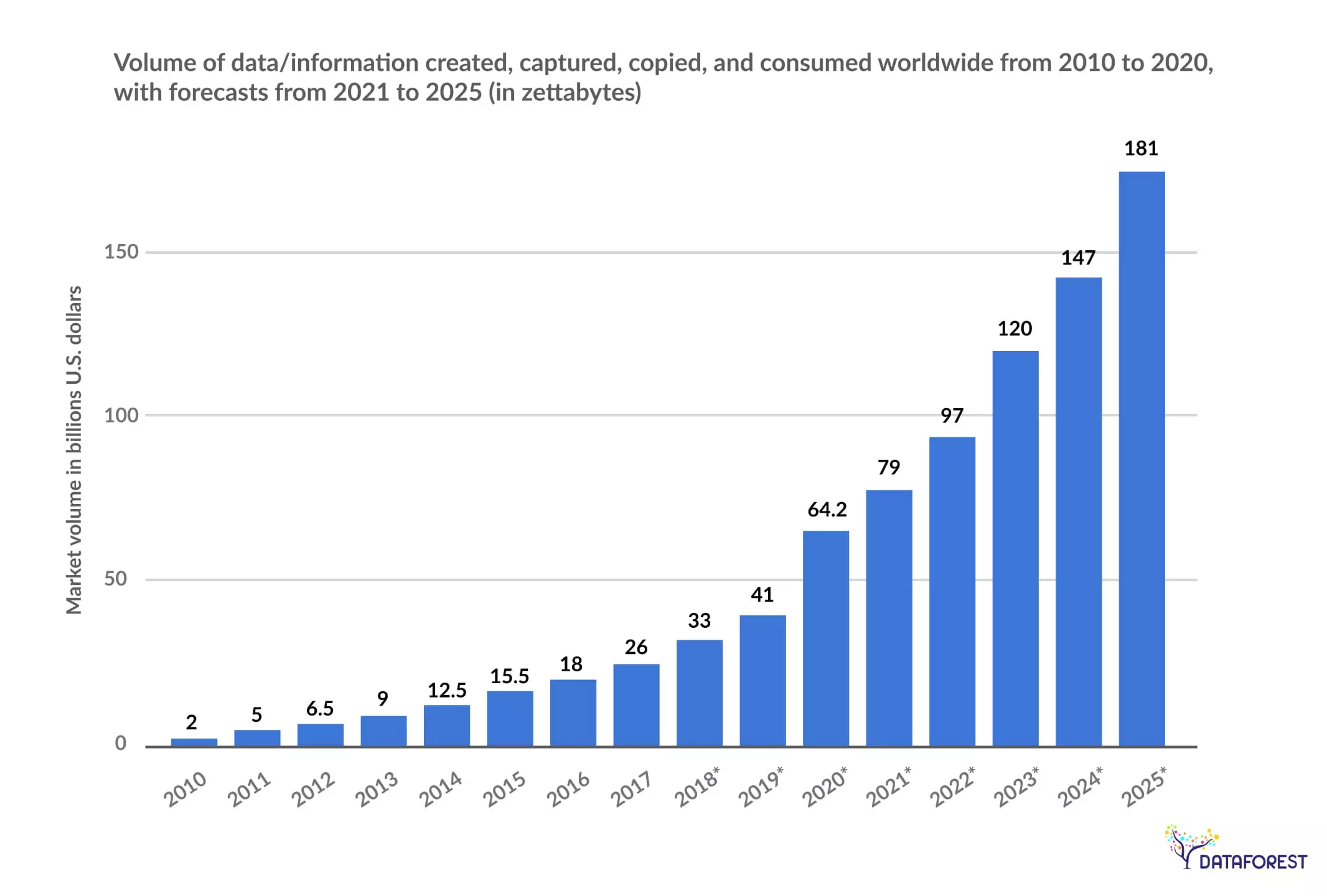
Source: Statista.com
This surge in data, propelled by the global digital footprints of internet users, accentuates the urgency for enterprises to adeptly orchestrate and amalgamate extensive datasets to unearth actionable insights and sustain a competitive vanguard. The quintessence of tapping into this colossal potential pivots around the meticulous execution of big data integration. This multifaceted and imperative venture demands a deep comprehension and an advanced skill set.
Presented by DATAFOREST, this discourse ventures into the nuanced realm of big data integration, shedding light on its paramount significance for entities poised to navigate and flourish in the rapidly transforming, data-driven business ecosystem.
Businesses can pivot data into actionable intelligence by deploying big data integration techniques and architectures, capitalizing on data lakes and warehouses, and facilitating ETL (Extract, Transform, Load) processes. This metamorphosis propels decision-making and amplifies organizational efficiency by refining data management practices and technological infrastructures.
This article offers a comprehensive investigation of the cruciality of big data integration, the impediments it navigates, and methodologies for its efficacious application within the intricate data landscapes of today. By centering on big data integration, enterprises can adeptly harness the insights for fostering innovation, operational excellence, and an enduring competitive edge.
What is Big Data Integration and why is it essential?
Big data integration embodies amalgamating and harmonizing extensive volumes of varied and intricate data from diverse origins into a coherent format ripe for analysis and application. This synthesis empowers organizations to attain holistic insights and extract value from their voluminous data reserves.
In the interconnected milieu of the modern era, entities accumulate data from an array of origins, encompassing internal systems, external databases, social media, IoT gadgets, and beyond. Nonetheless, this data frequently resides in disparate formats and locales, posing considerable challenges regarding accessibility, uniformity, and utility.
Big data integration confronts these challenges head-on by streamlining the aggregation, processing, and analysis of data from myriad origins. By weaving data from various streams into a centralized repository, entities can dismantle silos, enhance data quality, and unlock precious insights that catalyze business growth and innovation.
Overcoming the Challenges of Big Data Integration
Despite its promising advantages, big data integration introduces several hurdles for organizations. The foremost challenge is the overwhelming volume of data generated and amassed by businesses in the current digital age. With the exponential development of digital technologies and the internet, the volume of data burgeons incessantly exerts substantial pressure on organizations to manage and process this data efficaciously.
Additionally, the diversity of the data generated, encompassing structured and unstructured forms from various sources, complicates the integration process. While structured data, such as transactional records and customer databases, is relatively manageable, unstructured data, including textual content, images, and multimedia, poses a formidable challenge due to its complex and heterogeneous character.
Another pivotal challenge in big data integration is ensuring data quality and consistency. With data emanating from multiple sources, the risk of discrepancies, errors, and duplications looms, potentially compromising the precision and reliability of insights derived from the data. Organizations must institute stringent data governance protocols and quality assurance mechanisms to mitigate these issues and preserve the integrity of their data assets.
Moreover, data security and privacy stand at the forefront of concerns in big data integration endeavors. As organizations amalgamate vast volumes of data from disparate sources, adherence to data protection regulations and safeguarding sensitive information against unauthorized access, breaches, and exploitation become paramount.
Amplifying Benefits of Big Data Integration
Refining Decision-Making: The Crucial Role of Unified Data Insights
The process of big data integration is transformative for organizations, enabling access to a comprehensive dataset panorama that equips decision-makers with the critical insights required for strategic planning and informed execution. By amalgamating data from a diverse array of sources into a singular, coherent format, big data integration illuminates patterns, trends, and correlations that remain obscured in isolated data analysis. This enriched perspective bestowed by big data integration fosters precision in decision-making, catalyzing enhanced business outcomes and elevating organizational performance to new heights.
Deepening Analytical Insights: The Fusion of Varied Data Streams
Big data integration stands at the forefront of enriching organizational analytics, merging diverse data streams—including structured and unstructured sources—to unlock profound insights into operations, customer dynamics, and evolving market trends. This dismantling of data silos through big data integration and the strategic amalgamation of information from disparate sources allows organizations to reveal hidden patterns, pinpoint anomalies, and distill invaluable insights. Such augmented analytical capabilities, a direct outcome of big data integration, empower organizations to seize opportunities and refine processes with unprecedented effectiveness.
Crafting a Unified Operational Vision: The Comprehensive Impact of Integration
Through big data integration, organizations achieve a holistic understanding of their operational ecosystem and customer engagements, providing a 360-degree view that is otherwise unattainable. Big data integration offers a panoramic view of organizational operations and the customer journey by weaving together data from sales, marketing, financials, and customer service. This all-encompassing insight, a hallmark of effective big data integration, identifies inefficiencies, streamlines processes, and delivers personalized customer experiences that enhance satisfaction and foster loyalty.
Securing a Competitive Edge: The Strategic Advantage of Integrated Data
In the fiercely competitive terrain of today's business world, the ability to adeptly leverage integrated data through big data integration bestows a significant advantage. Big data integration equips companies with the capability to exploit their data assets fully, fostering innovation, agility in responding to market shifts, and a sustainable competitive lead. By leveraging the strategic insights derived from big data integration, organizations can unearth new opportunities, optimize operational efficiency, and distinctively position themselves in the marketplace, securing a robust competitive advantage.
Proactively Managing Risks: The Protective Power of Big Data Integration
The strategic application of big data integration extends into risk management and mitigation, offering organizations a potent tool for identifying and preemptively addressing potential threats. Through the continuous, real-time monitoring of vast datasets, big data integration allows businesses to anticipate and counteract emerging issues. For instance, a financial institution leveraging big data integration for analytics can detect anomalous transactions indicative of fraud, enabling swift, informed action to avert significant losses. This proactive stance on risk management, underpinned by big data integration, safeguards organizations against unforeseen challenges, securing their operational integrity and customer trust. If you want to always be on the cutting edge of technology, book a call.
Elevating Organizational Capabilities through Big Data Integration
The essence of big data integration lies in its capacity to transform how organizations leverage their vast data landscapes to foster business growth and innovation. By meticulously integrating an extensive array of datasets from myriad sources, big data integration aims to crystallize a series of pivotal objectives:
- Streamlining Data Aggregation: At the heart of big data integration lies the endeavor to amalgamate data scattered across diverse sources into a centralized repository. This harmonization effort by big data integration not only dismantles data silos but also crafts a comprehensive data tableau, offering a unified perspective of organizational data assets.
- Elevating Data Integrity: A cornerstone of big data integration is enhancing data quality and uniformity. By meticulously applying data cleansing, validation, and enrichment protocols, big data integration ensures the data's accuracy, reliability, and timeliness, empowering organizations to base their decisions on solid, insight-driven foundations.
- Enhancing Data Utilization: Big data integration is instrumental in making data more accessible and actionable across the organizational spectrum. Big data integration democratizes data exploration by furnishing decision-makers with self-service access to integrated data via intuitive interfaces and analytical tools, facilitating insight generation and strategic decision-making.
- Optimizing Operational Efficiency: By automating and refining data management practices, big data integration significantly streamlines the processes involved in data collection, processing, and analysis. This operational streamlining, in turn, accelerates decision-making, boosts business agility, and propels organizational efficiency to new heights.
Key Components of Big Data Integration
Navigating the complexities of big data integration necessitates a deep dive into its architectural framework, which is built on several fundamental components that ensure the seamless assimilation and utilization of massive data volumes. The key pillars of big data integration architecture include:
- Data Sources: The foundation of big data integration is the wide array of data sources that populate the data landscape, ranging from conventional databases and enterprise applications to cutting-edge IoT devices and online platforms. Big data integration confronts the challenge of heterogeneous data formats and structures head-on, deploying advanced connectors, APIs, and scripts to seamlessly extract and funnel data from these disparate sources into a consolidated repository for subsequent processing.
- Data Processing: Following data collection, the next phase involves the intricate processing and transformation of data into a format primed for analysis. This stage, pivotal in the big data integration process, encompasses data cleansing, normalization, aggregation, and enrichment, employing a suite of technologies and methodologies like ETL processes, Apache Hadoop for batch processing, and Apache Kafka for stream processing, aiming to optimize data quality and consistency.
- Data Storage: The choice of data storage solutions is critical in big data integration, dictating where and how integrated data is archived for future analytics. The spectrum of storage options ranges from traditional databases to scalable data lakes and NoSQL databases, each catering to different organizational needs. Data lakes, in particular, have emerged as a versatile and scalable storage paradigm, enabling raw data retention in its original format for comprehensive analysis.
- Data Delivery: The culmination of the big data integration process is delivering processed and integrated data to end-users, facilitated by sophisticated dashboards, visualization tools, and APIs. This component is essential for enabling interactive data exploration and insight extraction, supported by various business intelligence platforms and data visualization tools that transform integrated data into actionable business intelligence.
By meticulously addressing these architectural components, organizations can harness the full potential of big data integration, transforming vast and diverse data streams into a strategic asset that drives innovation, enhances decision-making, and secures a competitive advantage in the digital marketplace.
Advancing Big Data Integration with Cutting-Edge Technologies
Big data integration stands as a cornerstone for businesses aiming to leverage the immense power of their data. The sophistication and volume of the data involved necessitate sophisticated technologies designed to streamline the integration process. Among these, ETL processes, data pipelines, and data lakes are pivotal, each playing a unique role in enhancing the efficiency and effectiveness of big data integration efforts.
The Pivotal Role of ETL in Data Integration
ETL processes form the backbone of big data integration, enabling the meticulous collection, refinement, and assimilation of data from many sources into a singular, coherent system or data warehouse. The stages of ETL—Extract, Transform, Load—each serve a critical function:
- Extract: This initial phase involves the retrieval of data from various sources, whether they be databases, files, APIs, or live streams. ETL tools equipped with connectors and adapters are crucial here, as they streamline the extraction process, ensuring efficiency and reliability.
- Transform: Post-extraction, the data is subject to a series of transformations aimed at standardizing and enriching the dataset. These transformations are vital for cleansing data, rectifying inconsistencies, integrating additional valuable information, and preparing the data for insightful analysis.
- Load: The final stage sees the transformed data deposited into the designated system or data warehouse. Through careful management, ETL tools guarantee the data's integrity and consistency, setting the stage for subsequent analysis and insight generation.
ETL's role in big data integration is indispensable, offering a structured and efficient method for managing data movement and processing complexities.
Navigating the Complexity of Data Pipelines in Big Data Integration
Data pipelines are vital in extensive data integration, meticulously orchestrating the data journey across complex landscapes, often in real-time or near-real-time, to cater to the evolving needs of dynamic data environments. These intricate networks function as the foundational infrastructure, meticulously guiding data from its inception point through a series of transformative stages until it reaches its ultimate destination, ready for analytical consumption.
Technological advancements such as Apache NiFi and Apache Airflow, alongside cloud-powered solutions like AWS Data Pipeline and Google Cloud Dataflow, play a pivotal role in erecting these robust and scalable data conduits. They equip organizations with the essential tools for ingesting, refining, and disseminating data with unparalleled efficiency, bolstering real-time analytics and fortifying decision-making processes. The strategic deployment of data pipelines significantly propels the capabilities of extensive data integration services, ensuring a continuous, automated stream of data that is primed for analysis and actionable insights.
Harnessing the Potential of Data Lakes for Big Data Integration
Data lakes have emerged as a beacon of innovation in data storage and management, presenting a versatile and scalable refuge for the vast oceans of data that modern organizations grapple with. Unlike their traditional counterparts, data lakes embrace data in its most primal form, offering sanctuary to an extensive spectrum of data types, from the rigorously structured to the wildly unstructured.
The adoption of data lakes signifies a bold departure from the limitations inherent in conventional storage paradigms, heralding a new era of unified data storage solutions. This transformative approach democratizes access to data for comprehensive analysis and significantly curtails the financial burdens associated with data storage and management.
Leveraging avant-garde technologies such as Apache Hadoop and Apache Spark and cloud-based data lake services, including Amazon S3 and Azure Data Lake Storage, enterprises can now establish formidable data lakes. These technological marvels are the cornerstone for groundbreaking data analytics and insight-driven decision-making, empowering organizations to navigate the complexities of extensive data integration with confidence and clarity.
Elevating Big Data Integration: A Strategic Blueprint for Excellence
Harnessing the power of big data is a paramount goal for forward-thinking organizations, necessitating a robust, multi-dimensional strategy for big data integration. This strategy involves a harmonious blend of cutting-edge methodologies and technologies crafted to ensure a seamless, efficient data ecosystem. Central to achieving this are key strategies like batch processing, real-time integration, and innovative hybrid models, each tailored to meet the distinct needs and challenges of big data dynamics.
Core Strategies for Enhanced Big Data Integration
- Batch Processing: A cornerstone for managing vast data volumes, batch processing optimizes data handling by scheduling operations, ideal for non-urgent tasks.
- Real-Time Integration: Essential for scenarios demanding swift insights, this data strategy ensures data is processed instantaneously, supporting critical decision-making.
- Hybrid Models: Merging the best of batch and real-time processing, hybrid models offer a versatile framework for dynamic data integration, adapting to varying organizational demands.
Implementing these strategies addresses the complex challenges inherent in big data integration, facilitating the seamless amalgamation of disparate data sources into a cohesive whole.
Comprehensive Insights Table for Strategic Big Data Integration
Delve into the strategic nuances of big data integration with the following table, outlining key strategies, examples, KPIs, and the tools or technologies best suited for each approach. This detailed overview serves as a practical guide for organizations striving to master big data integration:
Upgrading Your Data Mastery with DATAFOREST
DATAFOREST big data integration company and a provider of big data integration services, can help unlock the vast potential embedded within complex data ecosystems. The journey of melding and refining enormous datasets from many sources, known as data integration in big data, embodies both a formidable challenge and an unprecedented opportunity.
Is your organization poised to transcend the conventional boundaries of data management and unlock the transformative power of big data integration? Discover how DATAFOREST's big data integration services can elevate your data strategies from mere concepts to engines of growth and innovation. Initiate your journey towards unparalleled data mastery by contacting us today, and together, we'll transform your data challenges into pillars of success.
FAQ
How can organizations ensure data quality during integration?
Organizations must adopt comprehensive data management practices to safeguard data quality throughout the integration process. This involves rigorous data profiling, thorough cleansing, precise validation, and implementing a solid governance framework. Utilizing automated tools for data management and conducting regular quality checks are essential strategies to ensure data accuracy, consistency, and reliability of big data integration initiatives.
How do serverless architectures impact Big Data Integration?
Serverless architectures significantly influence big data integration by offering a scalable and cost-effective way to manage data workloads. By eliminating the need for infrastructure oversight, these architectures facilitate a more streamlined integration process, allowing organizations to focus on developing and deploying sophisticated data processing workflows. This approach optimizes efficiency and enhances the agility of big data integration efforts.
How can businesses get started with Big Data Integration?
Embarking on big data integration begins with strategically assessing the organization's data needs. This includes selecting the right integration tools and technologies, defining clear and effective processes, establishing robust data governance measures, and ensuring thorough testing. Continuous monitoring and optimization are crucial for maintaining the effectiveness of the big data integration framework, ensuring it remains responsive to evolving data requirements.
How does Big Data Integration differ from traditional data integration?
Big Data Integration stands apart from traditional data integration by its ability to manage and process vast volumes of data, accommodate various data types, and handle the rapid velocity at which data is generated and collected. Addressing these challenges necessitates specialized tools and innovative approaches, making big data integration a more complex but crucially important endeavor for harnessing the full potential of data in today's digital age.
How can organizations maintain data security during integration?
Ensuring data security during the integration process requires a multi-layered security strategy. This contains data encryption in transit and at rest, the implementation of stringent access control measures, the masking of sensitive information, and the maintenance of comprehensive audit trails. Regular security audits and assessments are also vital for identifying vulnerabilities and reinforcing the security posture of big data integration practices, ensuring data protection against unauthorized access and breaches.