Some people fill the desktop with papers, while others neatly arrange them in piles. But this does not mean that one of them works better. It's just that everyone uses the approach that suits him. It's the same with data storage: in some cases, structured storage is more suitable; in others — the data is in its raw form.
Data Warehouses and Data Lakes Share Common Benefits
Both data warehouses and data lakes provide a centralized repository for storing and managing large volumes of data. Consolidating data from various sources into a single location gives businesses a unified view of data, making it easier to access, analyze, and derive insights. For the same purpose, you can book a call to us.
Making smart decisions
Modern businesses collect much information about their customers, operations, and market. Data management and analytics organize and make sense of all that data, helping companies to understand what's happening, why it's happening, and what might happen in the future. This knowledge helps businesses make smarter decisions and take action to success.
By examining data, businesses find patterns, trends, and hidden insights they might have missed otherwise. They reveal new ways to improve products, reach more customers, reduce costs, and even create innovative solutions. It makes businesses stay competitive and search for new ways to grow.
Data warehouses and data lakes concepts
The conflict between a data lake and a data warehouse in big data technologies arises due to differences in their underlying concepts and approaches to data management and analytics.
Depending on the tasks and characteristics of the project, data scientists choose one of the options or use both. Book a call, get advice from DATAFOREST, and move in the right direction.
The correct data management approach
The data lake is like a messy room where you dump all kinds of things without much organization. On the other hand, the data warehouse is similar to the organized cupboard with labeled shelves. When developing a data storage project, consider your wishes and capabilities.
- Data lakes allow flexibility by accepting data without strict rules, while data warehouses require structured and organized data with predefined schemas.
- Data warehouses integrate data from various sources into a central repository, ensuring consistency and accuracy. Data lakes store data as-is, without any enforced integration or consolidation.
- Data warehouses are designed for structured querying and reporting, providing fast and efficient access to organized data. Data lakes are more suited for exploratory analysis and processing large volumes of diverse and unstructured data.
- Data warehouses prioritize data governance, quality controls, and security measures to ensure data accuracy and compliance. Data lakes may need more built-in governance controls and require additional effort to implement proper data governance practices.
A data lake and a data warehouse can be used together in a complementary way to address various data management and analytics needs.
Data Warehouse Features
Imagine you have a big closet where you store all your clothes. Sometimes it's hard to find the exact shirt or pair of pants you want because everything is mixed up. That's where a data warehouse comes in. It is like an organized closet specifically designed to store structured data. The information is well-organized and follows a specific format, like data in tables with rows and columns.
The task is to facilitate data retrieval
The data warehouse aims to make it easy for businesses to find and analyze structured data.
It combines data from databases, customer records, or sales systems into one central place. Just like how you neatly fold and arrange your clothes in the closet.
- With data neatly organized, it's much easier to find the information. Businesses can locate specific data for analysis or reporting. It is also about cloud data warehouses.
- Data warehouses make it simple to analyze trends and patterns in the data. A business can quickly see which products sell the most, which regions have the highest sales, or how customer preferences change over time.
- Structured data in a data warehouse allows businesses to create reports and make informed decisions. It's like summarizing all your clothes to decide which outfits you want to wear.
- A data warehouse ensures that data is consistent and accurate. You keep your clothes clean and well-maintained, and the data warehouse maintains data quality by eliminating duplicates, errors, and inconsistencies.
A data warehouse architecture helps businesses keep their data in order, makes it easy to find and analyze, and supports better decision-making.
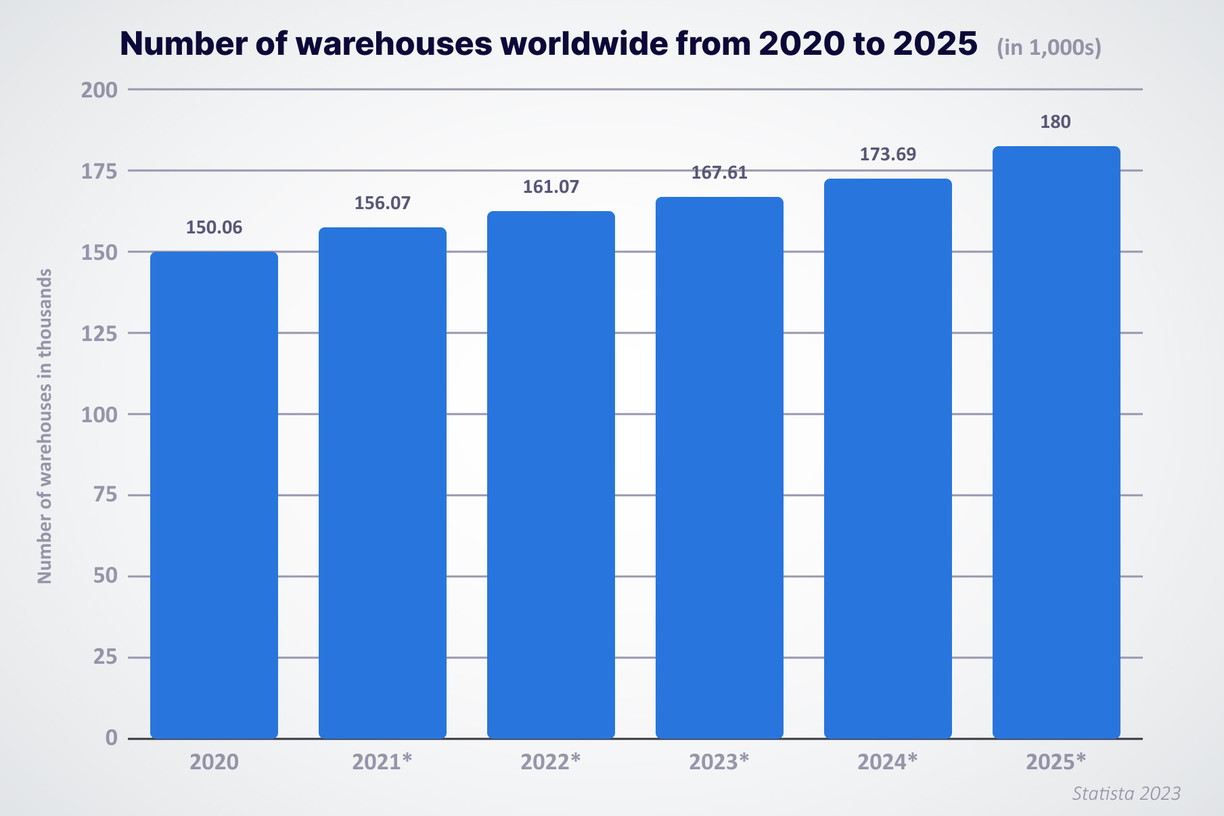
Important characteristics and components
Data warehouses often use a dimensional model to organize data for analysis. It includes dimensions (categories, time, product, or location) and facts (numeric measures like sales or quantity). It provides a structure for analyzing data from different angles.
Going through the ETL process
The ETL process in a data warehouse resembles preparing, organizing, and moving data from different sources into central storage so that it can be easily analyzed and understood.
- Extract: Think of gathering information from different places — databases, spreadsheets, or even text files.
- Transform: Making changes to the data to ensure it is in a consistent format and can be easily analyzed.
- Load: Moving it into a central storage location where it can be easily accessed and analyzed.
The ETL process is a conveyor belt that takes raw data, cleans it up, and puts it in the right place in the data warehouse.
Creating a blueprint or a plan
The schema design in a data warehouse provides a framework for organizing, structuring, and connecting the data. Before constructing a house, you need a blueprint outlining the layout, room sizes, and connections between different parts. The schema design defines the tables, columns, and their relationships, like rooms and doors in a house. As a house has walls, floors, and ceilings, a data warehouse design defines the structure of the data. It would help if you also designed a home with convenient access to rooms and functionalities.
Special functions to explore data
OLAP (Online Analytical Processing) capabilities in a data warehouse are special tools that allow businesses to analyze and explore their data flexibly and interactively. OLAP produces data exploration, multi-dimensional analysis, and aggregation. OLAP lets companies slice the data by selecting a specific dimension or attribute and dice it by further breaking it into smaller parts. OLAP capabilities support interactive reporting and making a customizable dashboard to interact with and manipulate the data in real time.
Commonly used benefits
Data warehouses offer several benefits that are commonly used in various scenarios. Centralized and structured data, historical data preservation, and improved performance are among them. Typical use cases are business intelligence, decision support systems, market or financial analysis, and regulatory compliance. They provide a solid foundation for businesses to harness the power of their data and derive meaningful insights for success.
Data Lake for All Kinds of Data
A data lake is like a big, flexible storage pool that holds all kinds of data, regardless of its structure or format. It's designed to handle diverse and unstructured data more fluidly and flexibly.
Like a real lake
Imagine a lake where you store all sorts of things: rocks, plants, fish, coins, or even toys. Each item has a different shape, size, or type, and they coexist in the same lake without any strict organization. Similarly, a data lake allows businesses to store different types of data — text files, images, videos, social media posts, sensor data, or log files — in their original form without imposing a specific format. The purpose of a data lake is to provide a central repository for businesses to store and analyze diverse data without the need for upfront structuring.
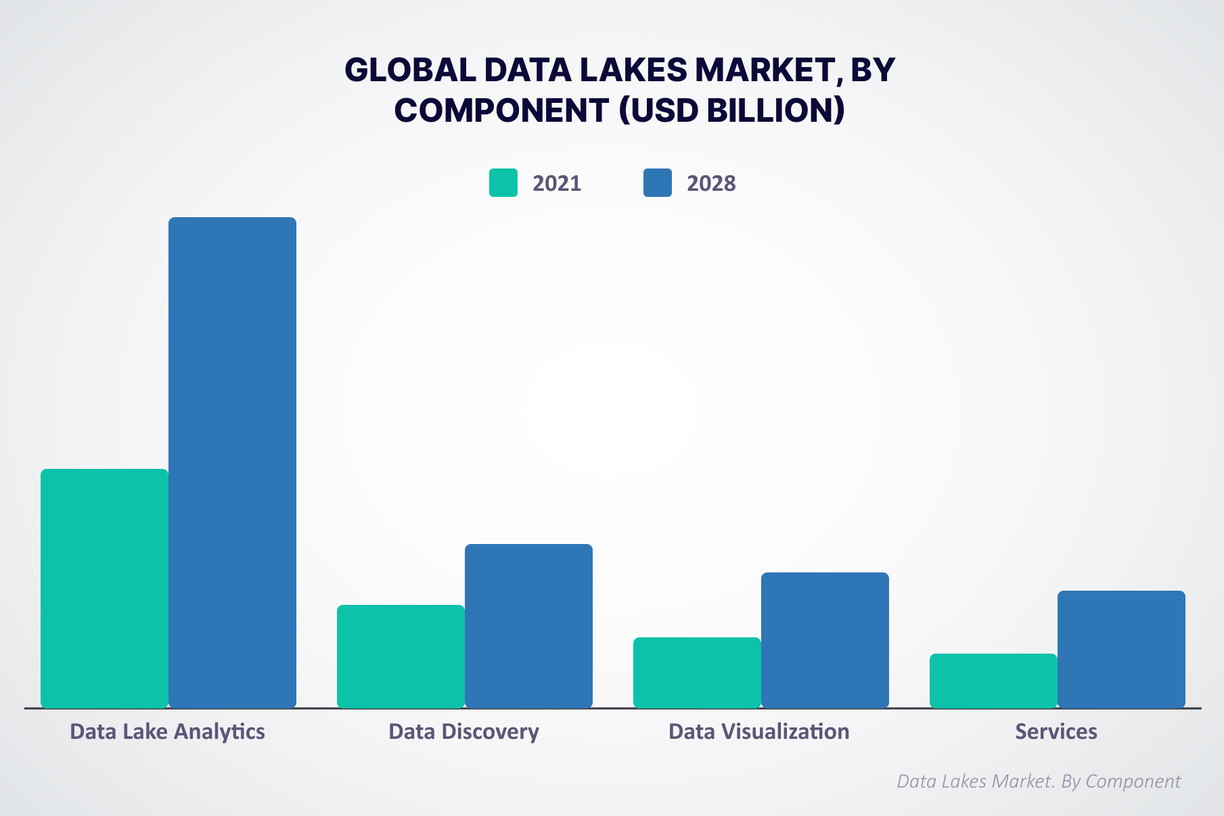
Data Lakes Market, By Component
Handling diverse data
The storage layer is where the data is physically stored in the data lake. It typically uses scalable and distributed storage systems like Hadoop Distributed File System or cloud-based services. The storage layer ensures durability, availability, and easy access to the data.
Data Ingestion
Data ingestion is the process of bringing data into the data lake. It involves collecting data from various sources such as databases, applications, IoT devices, or external sources and loading it into the lake. It's like pouring water from different streams into the pool.
Schema on read
In a data lake, the data is not structured upfront. Instead, the structure is applied when the data is read or analyzed. It means businesses define and modify the system as needed during the analysis. It provides flexibility for exploration without strict schemas.
Massive data
A data lake accommodates petabytes of info, making it suitable for storing and managing large and growing data volumes. Unlike traditional data storage approaches, a data lake does not require strict schemas or predefined structures.
Data Lake benefits and cases
Data lakes provide flexible storage adapting businesses to evolving data requirements and easily incorporating new data sources. The scalability allows to store and analyze large amounts of data without worrying about capacity constraints. Storage options minimize infrastructure costs associated with data management. Hidden insights empower data scientists and analysts to extract valuable business patterns. Data scientists also access the data lake to build sophisticated models.
The typical use cases of data lakes are:
- Big Data Analytics
- Data Science and Machine Learning
- Internet of Things (IoT) Analytics
- Data Exploration and Discovery
- Data Integration and Data Hub
Data lakes certify to unlock the value of data and gain decision-making.
Choosing Between Data Warehouse and Data Lake
Choosing between a data warehouse and a data lake depends on data structure, use cases, processing needs, integration requirements, governance, security, and cost considerations. Understanding these key features will help you make an informed decision aligning with your data requirements and business objectives.
What to start from
When deciding between a data warehouse and a data lake, consider factors like data structure, sources, analysis needs, data volume, scalability, processing speed, governance and security requirements, and cost implications. Understanding these factors will help you choose the most suitable option for your data storage, management, and analysis needs.
Data types and sources
When deciding between a data warehouse and a data lake, there are two factors to consider in data types and sources context.
- Consider the types of data you will be working with; if it is mostly structured, like spreadsheets or databases with organized rows and columns, a data warehouse is well-suited for storing and analyzing this data. A data lake is better if your data is diverse and includes unstructured or semi-structured data.
- If applications or systems generate your data, and you need to integrate it into a unified format for analysis, a data warehouse can handle this task efficiently. If your data comes from multiple sources with varying formats and structures, a data lake allows you to store the data as-is without upfront transformations.
Data quality and governance
- A data warehouse is suitable if you require high data quality with strict validation, cleansing, and consistency checks. Data warehouses have predefined schemas and integration processes that ensure data quality standards are met. They provide a structured environment where data is transformed before being loaded.
- The strict governance policies, compliance regulations, or specific data access controls are for enterprise data warehouses. It offers robust features for enforcing governance practices and provides mechanisms to manage access privileges, data lineage, auditing, and data versioning, ensuring data governance and compliance.
Scalability
- A data lake provides scalable storage options for storing large amounts of data. It accommodates massive quantities of data without sacrificing performance or incurring high costs. Data warehouses can also scale but require additional effort and resources to handle rapidly growing data volumes.
Analytics and Reporting
- A data warehouse is designed for structured reporting, predefined queries, and business intelligence. It provides optimized schemas that enable efficient querying. On the other hand, a data lake offers greater flexibility if you require more exploratory analysis, advanced analytics, and the ability to work with unstructured data.
- A data warehouse provides a structured environment to support metrics, dimensions, and data aggregations. It also offers predefined data models and pre-calculated aggregates that speed up reporting processes. Data lakes can also support reporting but require additional data processing and modeling steps to achieve the desired outputs.
Understanding these factors will help you choose the most suitable option that aligns with your analytics and reporting goals.
Ten steps to reach business suitability
When evaluating the suitability of a data warehouse or a data lake for your business, it's important to consider ten factors.
- Identify your data requirements
- Assess your analytical needs
- Evaluate scalability
- Consider data integration and transformation
- Analyze data governance and security needs
- Rate agility and flexibility
- Consider cost implications
- Gauge available resources and expertise
- Seek input from stakeholders
- Pilot and iterate
These comprehensive evaluations will help you make an informed decision that aligns with your business requirements.
Combining Elements of Both Data Warehouse and Data Lake
Integration and hybrid approaches refer to combining elements of a data warehouse and a data lake to leverage their strengths. The integration approach involves integrating a data warehouse and a data lake to create a unified data platform. The hybrid approach means creating a hybrid data architecture that combines data warehouse and data lake elements into a single solution.
A single solution that benefits from both
Imagine you have two tools: a toolbox and a toy box. The toolbox has all the tools you need for a particular task, like a screwdriver and a hammer. The toy box, however, has a variety of toys you can play with. Now, let's say you want to build something using specific tools from the toolbox, but you also want to incorporate some fun and unique elements from the toy box. To do this, you can integrate the toolbox and the toy box. You take the necessary tools from the toolbox to complete the construction work and add the exciting toys from the toy box to make it more interesting and enjoyable.
Integrating data warehouses and data lakes for a hybrid approach works similarly. The data warehouse is like the toolbox, which provides structured data for reporting and analysis. The data lake is like the toy box, which holds diverse and unstructured data. By integrating the two, you can use the data warehouse for specific structured analysis and reporting needs, and you can also tap into the data lake to explore and uncover insights from diverse and unstructured data. It's like combining the best features of both worlds to get a more comprehensive and powerful solution for your data needs.
Data pizza
Imagine you are making a pizza. You have a pizza crust, which represents your data warehouse, and you have various toppings, which represent your data lake.
Scenario 1: Structured Reporting with Special Toppings
In this scenario, you want to create a traditional pizza with predefined toppings, like cheese, pepperoni, and mushrooms. You use the pizza crust (data warehouse) to provide the base structure for structured reporting. The predefined toppings (structured data) are stored in the data warehouse, allowing you to create consistent and structured reports.
However, you also want to add special toppings like pineapple or jalapeños, which are unique and not part of the traditional recipe. These toppings represent diverse data that is not fit into the data warehouse. In this case, you can force the data lake to store special toppings. It allows to experiment with different combinations and flavors (data exploration and analysis) that do not conform to the predefined structure of the data warehouse.
Scenario 2: Data Enrichment and Experimentation
In this scenario, you have a pizza crust (data warehouse) that provides a solid foundation for structured reporting and analysis. However, you want to enhance your pizza with new flavors and experiment with different toppings to cater to customer preferences.
You use the data lake to store additional toppings and ingredients that are not yet ready for structured reporting. You can experiment with new data sources, such as social media feeds or customer reviews, and extract valuable insights from them.
The integration and hybrid approaches permit companies to combine the strengths of both solutions, creating a more adaptable data platform.
Big house, toy train track, and cake
Here are some simple examples of hybrid architectures and how they address different data management needs.
Staging and Integration
Imagine you have a big house with multiple rooms. You decide to use one room as a data warehouse and another as a data lake. Whenever you bring in new items, you first place them in the data lake room. It represents the raw and diverse data that you collect from various sources. You transfer those items to the data warehouse room when you need to organize them for specific purposes. It represents that the processed data is ready for analysis.
Extract, transform, load (ETL) pipeline
Imagine you are building a toy train track. You have different types of tracks and connectors to create a customized layout. In this analogy, the tracks represent the data warehouse, and the connectors represent the data lake. You start by laying down the primary tracks, representing your data warehouse, to create the main structure of the train track. These tracks ensure stability and provide the foundation for structured reporting.
Real-time analytics and historical analysis
Imagine you are baking a cake. You have a mixing bowl and an ingredient tray. The mixing bowl represents your data warehouse, and the ingredient tray means your data lake.
You start by putting the main ingredients in the mixing bowl to create the base of your cake. These ingredients are structured and ready for immediate use, representing the data warehouse's real-time analytics. You can access the ingredient tray (data lake) for additional variations to add different ingredients.
Data Security and Compliance in Data Warehouse and Data Lake
Data security indicates protecting your data from unauthorized access, similar to securing valuable belongings in a safe. Compliance calls for adhering to regulations related to data management, like following the game rules. A data warehouse provides a structured and secure environment, making security and compliance easier. While more challenging, a data lake can still be secured and made compliant through proper measures and governance practices.
Reliable and by the rules
Let's break down data security and compliance concepts in simple terms.
- Data Security
In a data warehouse, it's like putting precious items in a locked safe, accessible only to those with the right key or combination. It has strong security measures in place to protect your structured data. The data is organized, controlled, and accessed by authorized users. On the other hand, a data lake is like an open storage area. It may store raw and diverse data in its original form, making securing it more challenging. However, measures are implemented to protect the data lake from unauthorized access.
- Compliance
Imagine there are rules that you need to follow while playing a game. And its name is data management. With a data warehouse, compliance is often easier to achieve. It provides a structured environment where data governance is enforced. Since data lake stores raw data, ensuring compliance involves additional efforts. But with proper data governance practices and security controls, compliance can still be achieved. It’s like playing with fewer predefined rules, but you can ensure compliance.
Privacy and regulatory compliance
Data privacy refers to protecting the personal or sensitive information of individuals. It’s similar to keeping personal secrets or private information confidential. Regulatory compliance notes following laws, regulations, and industry standards related to data management. This touch can be compared to obeying the game rules to ensure fairness and adherence to legal requirements.
The protection practices
By implementing verified security strategies, firms enhance their data protection in both data warehouses and data lakes, safeguarding it from breaches and potential risks.
Data warehouse security
- Implement strong access controls to ensure only authorized individuals can access the data warehouse.
- Employ encryption techniques to protect data at rest and during transmission.
- Carry out monitoring tools to track access and activities within the data warehouse.
- Apply data masking techniques to obfuscate sensitive data when it's unnecessary for reporting or analysis.
Data lake security
- Classify data in the data lake based on its sensitivity level.
- Enact authentication mechanisms to control who can modify the data in the data lake.
- Fulfill encryption techniques to protect data at rest and in transit within the data lake.
- Perform anonymization techniques to protect personally identifiable information (PII) stored in the data lake.
- Establish strong data governance practices to enforce data access, quality, and lifecycle management policies.
Data Warehouse and Data Lake are Library and Secondhand Bookshop
In a data warehouse, data is stacked and accounted for like books in a library. And in the secondhand market (data lake), you can find much more interesting tomes, with signatures and photographs forgotten inside. But people need to take these features into account initially; they appear only by chance when turning over the pages of an old book.
Deriving insights
Analytics with a data warehouse:
- A data warehouse allows analysts to perform structured analysis on organized data.
- Since a data warehouse retains historical data, analysts examine past patterns.
- Data warehouses calculate aggregated sales totals, customer counts, or average values.
Analytics with a data lake:
- Data lakes allow analysts to explore a wide range of data without predefined schemas.
- A data lake accommodates diverse data sources, allowing integration and analysis of different types of data.
- Data lakes support advanced analytics techniques like machine learning, natural language processing, and predictive modeling.
Both data warehouses and data lakes play important roles in supporting analytics and deriving insights, but they cater to different needs and types of data.
Types of analytics and reporting
- Data Warehouse:
- Support descriptive analytics, which summarizes historical data to understand past events.
- Enable diagnostic analytics, which analyzes data to know why something happened.
- Offer reporting capabilities, which generate predefined reports based on specific business requirements.
- Data Lake:
- Support exploratory analytics, which explores raw data to uncover patterns.
- It is suitable for predictive analytics, which uses historical data to make predictions.
- Facilitate ad hoc reporting, allowing the generation of quick custom reports based on on-the-fly analysis.
Data warehouses and data lakes provide distinct analytical capabilities, catering to different analytical needs and enabling businesses to gain insights from their data.
Supporting efficient analytics
Data modeling and schema design optimize the structure for efficient querying and analysis in a data warehouse. In a Data Lake, data modeling focuses on metadata management, and schema design provides flexibility for handling diverse and unstructured data sources. Both approaches aim to organize and structure data to support efficient analytics and enable users to derive insights from the data.
Data Warehouse and Data Lake Application Branches
The specific use cases and benefits will vary depending on the industry, company, and their particular data management and analytics needs.
Data Warehouse Use Cases
- Sales and Marketing Analysis: Companies use data warehouses to analyze sales data, customer behavior, and marketing campaigns.
- Financial Reporting and Analysis: Data warehouses are commonly used in FinTech to consolidate and analyze financial data.
- Supply Chain Management: Data warehouses consolidate data from inventory systems, production databases, and logistics platforms.
Data Lake Use Cases
- Customer 360 and Personalization: Data lakes are created a comprehensive view of customers by integrating data from various sources like CRM systems, social media, customer support interactions, and website logs.
- Internet of Things (IoT) Analytics: With the rise of IoT devices, data lakes store and analyze data generated by sensors, machines, and connected devices.
- Data Science and Analytics: Data lakes provide a foundation for data scientists and analysts to perform advanced analytics, machine learning, and AI modeling.
These are just a few examples of how data warehouses and data lakes have been successfully implemented in real-world scenarios.
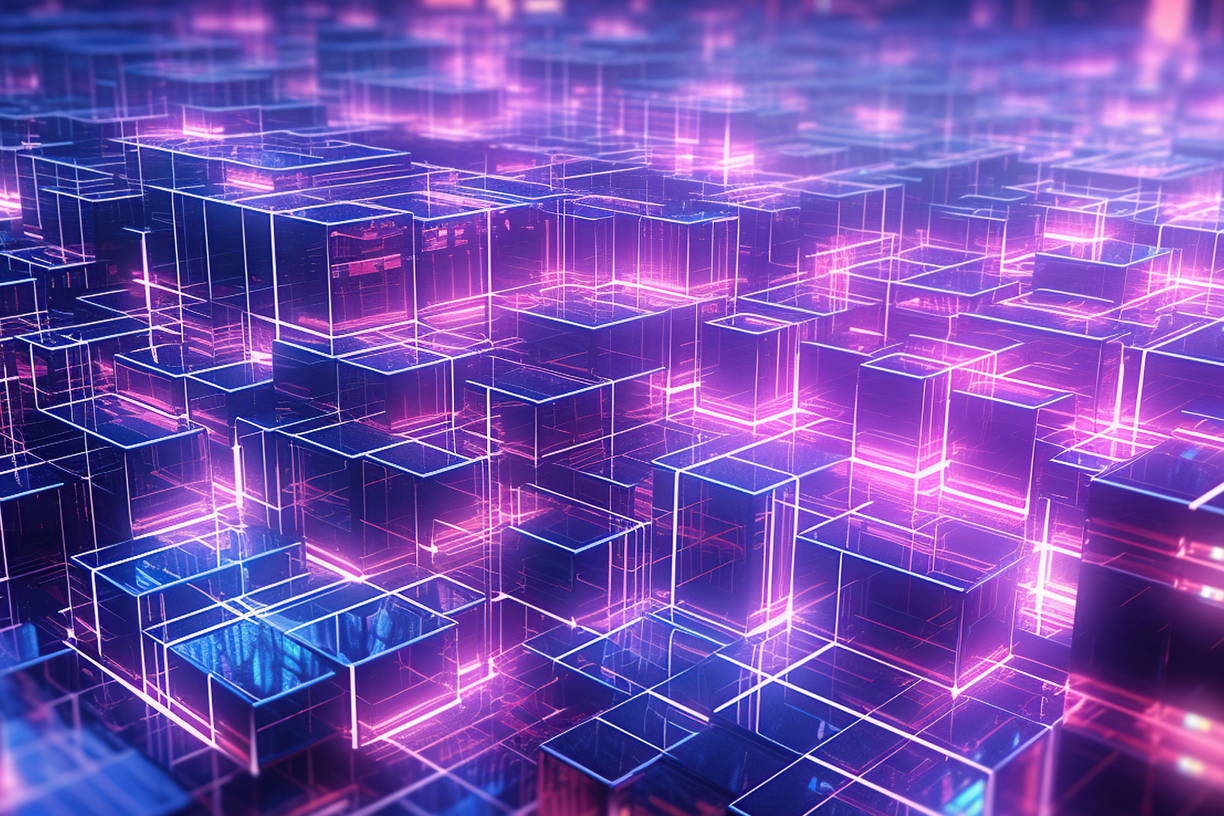
Some specific benefits and outcomes
Some famous brands have successfully implemented data warehouses or data lakes.
Data warehouses
- Amazon, one of the world's largest e-commerce companies, leverages data warehouses to analyze customer behavior, purchasing patterns, and inventory management.
- A multinational retail corporation, Walmart uses data warehouses to analyze sales data, inventory levels, and customer preferences.
- Netflix, a leading streaming entertainment service, relies on data warehouses to analyze viewer preferences, content performance, and user engagement.
Data lakes
- Uber, the ride-hailing and food delivery platform, manipulates data lakes to analyze vast amounts of real-time data generated by drivers, riders, and delivery partners.
- Airbnb, an online marketplace for accommodations, uses data lakes to analyze user behavior, property listings, and booking patterns.
- Spotify, a popular music streaming platform, relies on data lakes to analyze user listening habits, music preferences, and playlist creation.
Each brand has unique use cases based on its industry, business model, and data management requirements.
Industries examples
Data Warehouses and Data Lakes Work as Named
The warehouse is designed to store specific items in predefined locations for easy retrieval. The data enters the lake rawly without predefined organization or structure, such as rivers and streams.
These two data stores work as metaphorically as they are called. The data science and data engineering specialists DATAFOREST has are well versed in the features of each storage structure and are always ready to get the most out of each separately or can combine solutions if necessary. The choice between tools implies the flexibility of decisions; the greater the choice, the greater the flexibility. Using it is a matter of skill.
If you have a complex project regarding data storage conditions or are curious about what you have read, fill out the form, and we will talk in more detail. We are always glad to have new and interesting cooperation.
FAQ
What are the main differences between a data warehouse and a data lake?
The main differences between a data warehouse and a data lake are their data structure, storage approach, and analytics capabilities. A data warehouse stores structured data with a predefined schema optimized for SQL-based querying and reporting. In contrast, a data lake stores raw and diverse data, including structured, semi-structured, and unstructured formats, allowing for flexible data exploration and advanced analytics beyond SQL.
How does a data warehouse handle structured data, and what are its advantages?
A data warehouse handles structured data by organizing it into a predefined schema, enforcing data consistency, and optimizing it for SQL-based querying. Its advantages lie in its ability to provide a structured and controlled data analysis environment, ensure data integrity, and deliver fast and efficient access to structured data.
What types of data are suitable for storage in a data warehouse?
Data warehouses are suitable for storing structured and relational data that follows a predefined schema. It includes transactional data, customer information, sales data, financial records, and other data with a well-defined structure and clear relationships.
How does a data lake handle unstructured and diverse data?
A data lake handles unstructured and diverse data by storing it in its raw form without the need for immediate structure or predefined organization. It accommodates a wide range of data types. With its flexible schema-less nature, a data lake allows for the exploration, analysis, and processing of diverse data sets, empowering to uncover valuable insights and support advanced analytics use cases.
What examples of unstructured data can be stored in a data lake?
Unstructured data stored in a data lake include text documents, social media posts, emails, sensor data, log files, audio recordings, video files, and images. These data types do not conform to a fixed schema or structure, making them challenging to store and analyze in traditional relational databases. By keeping such unstructured data in a data lake, firms leverage advanced analytics techniques to derive meaningful insights.
Can a data lake support real-time data processing and analytics?
By integrating real-time data streams into the data lake, companies derive insights from data as it arrives, enabling timely decision-making and immediate response to events. Real-time processing frameworks like Apache Kafka and Apache Flink to ingest, process, and analyze streaming data within the data lake environment, allowing for real-time analytics.
What are the typical use cases where a data warehouse better fits a business?
A data warehouse is a better fit for a business when there is a need for structured data analysis, standardized reporting, and business intelligence. Typical use cases include sales analysis, financial reporting, inventory management, and regulatory compliance, where structured data from various sources must be consolidated and analyzed in a structured and controlled environment.
In which scenarios would a data lake be more suitable than a data warehouse?
A data lake is more suitable than a data warehouse in scenarios where there is a need to store and analyze diverse, unstructured, and raw data. It is ideal for exploratory analysis, data science, and advanced analytics use cases. Typical scenarios include data exploration, machine learning, sentiment analysis, IoT data analysis, and scenarios where the data sources and analysis requirements are evolving and require flexibility.
How do data warehouses and data lakes support advanced analytics and machine learning?
Data warehouses support advanced analytics and machine learning by providing structured and well-organized data that can be easily queried and analyzed using SQL-based techniques. Data lakes offer the flexibility to store raw data, which is used for exploratory analysis, data preprocessing, and training large-scale machine learning models that require extensive data transformation and feature engineering.