So, you've got a shiny new fitness tracker, and you're racking up steps. But did you know your little wrist buddy might be a game-changer for your health insurance? We're talking about the nifty and slightly nosy world where tech meets healthcare. These gadgets are part of a bigger picture, where your jogs around the block could lead to a lighter insurance bill. Join us in exploring this digital mix of health, data science, and dollars.
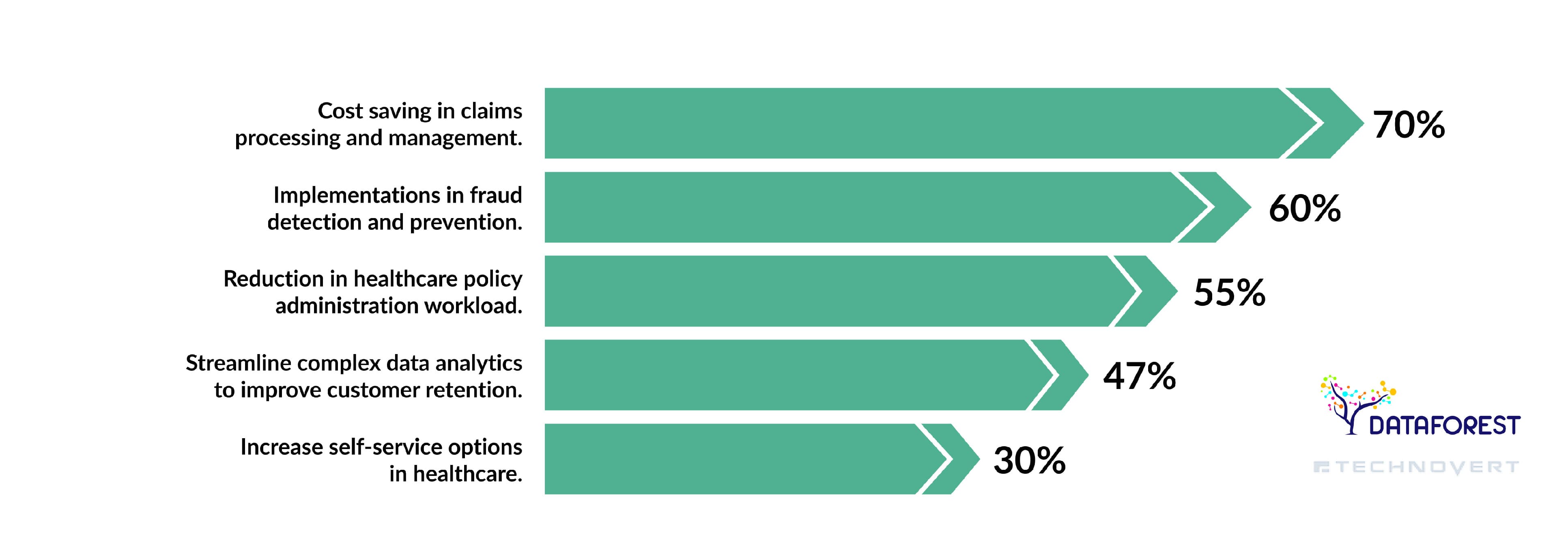
Key Statistics of Emerging Technologies in Health Insurance
Data Science and Insurance — No More Guesswork
You're in a world where your insurance isn't just a roll of the dice or some one-size-fits-all deal. That's what data science is making happen. Think about how we used to do things. Insurance was like shooting in the dark, kinda guessing based on what happened in the past. We have all this info at our fingertips – from your morning jog routine to your driving habits. It helps insurers get a picture of what risks they're dealing with.
Imagine getting insurance that actually gets you, tailored just for your lifestyle. That's the personal touch data science brings. It's like your insurance company isn't some faceless corporation anymore; they're more like a buddy who knows what you need and when you need it.
AI turns data science in insurance into a powerhouse, making insurance smarter, more personal, and just plain better at what it does. It's an exciting time to be in the field.
Defining Data Science in The Insurance Industry
Data science in insurance is about extracting insights and knowledge from data. It involves various disciplines: statistics, machine learning, predictive analytics, and AI generally.
Data Science for Insurance: Relevance
The application of data science in the insurance industry is like a goldmine. It swims in data — from personal details to claims history. The trick is to sift through this data, find patterns, learn from them, and make predictions. It's all about using this data to make smarter decisions, whether about pricing policies, assessing risks, or understanding customer needs.
Key Concepts and Methodologies for Insurance Data Scientists
- Predictive modeling is like the bread and butter of data science in insurance. Using historical data, predictive models forecast future events.
- Think of machine learning as teaching computers to learn from data. In insurance, this could mean algorithms that improve over time at detecting fraud or assessing risk.
- Data mining involves digging through large sets of data to find relevant patterns. Data science uses insurance cases; it could identify common factors in claims.
- Agents can use statistical models to estimate the risk associated with insuring an individual or business. It's about predicting the 'what ifs.'
Data Science in Insurance — Decision-Making
- Here, data science in insurance is used to evaluate the client's risk. Insurers set premiums that accurately reflect the risk by analyzing age, health, driving records, etc.
- Data science in insurance streamlines the process. By automating analysis using AI and ML, insurers determine the legitimacy of a claim the likely cost, and process payments faster.
- Data science in insurance helps understand customers better. By analyzing customer data, companies tailor services, offer personalized policies, and engage with customers more meaningfully.
Redefining Relationships — Data Science for the Insurance Sector
Insurance data science has changed risk assessment and pricing through predictive modeling, turning historical data into foresight. It also plays a pivotal role in detecting fraud, personalizing insurance products, predicting customer churn, optimizing claims processing, and transforming traditional insurance into a highly efficient industry.
Predictive Modeling for Data Science in Insurance
Predictive modeling in risk assessment and pricing within the data science in insurance requires analyzing vast amounts of historical data — ranging from personal client information to broader economic indicators — to predict future risk and determine appropriate insurance premiums. By identifying patterns and correlations in past events, insurers more accurately forecast potential risks, ensuring that pricing is fair for the actual risk involved in the context of data science in insurance.
A Shield Against Fraud and Deception
Fraud detection and prevention due to data science in insurance, powered by advanced AI analytics, leverage sophisticated algorithms and machine learning techniques. Insurers analyze patterns and anomalies in vast datasets of claims history and customer interactions. It identifies potentially fraudulent activities before they impact the bottom line, effectively saving millions and maintaining the integrity of insurance operations. This proactive data science in insurance approach deters fraud and streamlines the claims process.
Tailoring Insurance Through Behavioral Insights
Based on customer behavior, personalized insurance products and pricing represent a paradigm shift in the insurance industry, driven by the nuanced understanding of individual customer profiles. By harnessing data science, insurers analyze behavioral factors like driving habits, health routines, and lifestyle choices. This analysis enables them to tailor insurance products and pricing to match each customer's specific risk profiles and preferences. Customers receive more relevant and cost-effective insurance coverage.
Mastering Customer Churn with Data Science in Insurance
Customer churn prediction and retention strategies in the insurance industry call for using data science to identify patterns and indicators that signal when a customer might leave. Insurers predict which clients are at risk by analyzing customer interactions, policy renewals, claims history, and feedback. With these insights from data science in insurance, they proactively implement targeted customer retention strategies, such as personalized offers, improved customer service, or policy adjustments, to address those customers' specific needs and concerns.
The Analytics Edge in Insurance Claims Optimization
Claims analytics and settlement optimization harness the power of data science in insurance to streamline the claims process, making it more efficient and customer-friendly. This approach analyzes vast amounts of claims data to identify insights that improve the speed and accuracy of claims processing. Advanced algorithms assess claim validity, estimate appropriate settlement amounts, and even automate certain process aspects. This not only accelerates claim resolution but also ensures fair and consistent settlements. By optimizing the claims process, insurers can significantly enhance customer satisfaction, while data science in insurance also reduces operational costs and mitigates the risk of over or underpaying claims.
Data Sources in Insurance
Insurers don't just collect data for the heck of it; they're like chefs sifting through ingredients, looking for the perfect combination to whip up policies as unique as the individuals they cover. This data-driven approach is a far cry from the old-school method of one-size-fits-all. It's all about getting a 360-degree view of the risk landscape. Here are some critical sources for collecting data for data science in insurance.
- Customer Data and Demographics
Imagine insurers as personal tailors of financial safety. They look at your age, job, health, and even your hobbies — it's building a profile in a dating app, but for insurance. This data helps them create a policy that fits your life like a glove. Data science in insurance is about understanding your day-to-day life. This level of personalization ensures that you’re not overpaying for coverage you don’t need or missing out on coverage that could be crucial.
- Telematics and Internet of Things (IoT)
This is where insurance steps into the future. Your car, smartwatch, or home gadgets are constantly chattering away, sending out data streams. It's a goldmine of information. For instance, your car's telematics tell insurers how fast you drive, how hard you brake, or how smoothly you take corners. Similarly, your fitness tracker's data might show how often you exercise. It’s for data science in health insurance, like a personal diary that insurers read to understand your habits, and in return, they can offer discounts or incentives for safe and healthy living.
- Social Media Data for Customer Sentiment Analysis
Here, insurers turn into social butterflies fluttering around the vast gardens of social media. They watch for what's buzzing: what customers love, what grinds their gears, and the general vibe about insurance topics. This insight is taking the pulse of public opinion. Data science in insurance helps to stay in touch with customer needs and expectations, tweak their marketing strategies, and even develop new products that resonate with current trends.
- Third-Party Data for Risk Assessment
These sources provide a different tune for data science in insurance, adding depth to the insurer's understanding. Credit history gives a peek into financial reliability, while public records can reveal past incidents that might flag potential risks. Weather data can be crucial for property insurance predicting natural disaster risks. This ensemble of external data harmonizes with internal data, enriching the risk assessment process.
- Historical Claims Data for Trend Analysis
Insurers dive into archives of past claims to detect patterns and trends. Data science in insurance does detective work, looking for clues that could predict future events. For example, a spike in car thefts in a particular area or a trend in health claims during certain seasons. This historical playbook helps set premiums more accurately and anticipate future trends, ensuring insurers are always one step ahead.
Overcoming Challenges in Insurance's Data Science Integration
Integrating data science into the insurance industry is challenging; it comes with challenges.
Data Science in Insurance — Navigating the Data Deluge
- Data Quality and Accuracy
Imagine trying to complete a jigsaw puzzle, but some pieces need to be included or fit right. That's what it's like dealing with data quality issues. Insurers often need help with complete, accurate, and updated data. If the data isn't clean, its insights can be misleading and destroy trust in data science in insurance.
- Data Privacy and Security Concerns
With significant data comes great responsibility for data science in insurance. Customers are entrusting their personal info to insurers, and any breach could be catastrophic, like leaving the vault door open with a sign saying ‘Come on in!’. Insurers need to ensure that the data is as secure as a fortress, safeguarding against unauthorized access, which is no small feat in this era of cyber threats.
- Regulatory Compliance and Ethical Considerations
Navigating the maze of regulations and ethical concerns is like walking a tightrope while juggling. Different regions have their own rules around data usage (think GDPR in Europe). Insurers must be vigilant to stay compliant, balancing data science with ethical considerations, like ensuring algorithms don't inadvertently discriminate against certain groups within data science in insurance.
- Integration with Legacy Systems
It is like trying to fit a square peg into a round hole. Many insurance companies have been around for decades, running on outdated systems. Integrating cutting-edge data science tools with these antiquated systems is a Herculean task. It requires time and investment, not to mention the technical headaches that come with it.
- Building A Data-Driven Culture for Data Science in Insurance
This is about changing the mindset. It’s one thing to have the tools and the data, but another to get everyone on board. It’s turning a cruise ship; it doesn’t happen on a dime. Building a data-driven culture means fostering an environment where data is at the heart of every decision, and that requires training, change management, and sometimes a shift in company values.
While integrating data science in insurance holds tremendous promise, the path is strewn with hurdles. These challenges require technological savvy, strategic foresight, and a deep commitment to ethical and responsible data usage.
Cases Of Solving Challenges of Data Science in Insurance
- A health insurance company faced data quality issues and resolved them by implementing an AI-driven system to continually assess and clean data.
- An auto insurer addressed privacy concerns by deploying an advanced encryption and user authentication system, significantly reducing the risk of data breaches in data science in insurance conception.
- To comply with GDPR, a European insurer developed a compliance framework that became part of their core operations, ensuring every data process met regulatory standards.
- A legacy insurer overcame system integration challenges by adopting a hybrid cloud approach, allowing them to gradually migrate their processes without disrupting existing operations and with no problems for data science in insurance.
- Data analytics in a life insurance company struggling with culture change initiated a company-wide data awareness program, integrating data-driven decision-making into their core values and daily operations.
In each of these scenarios, the insurers didn't just overcome challenges; they used them as stepping stones to innovate and improve their operations with data science in insurance.
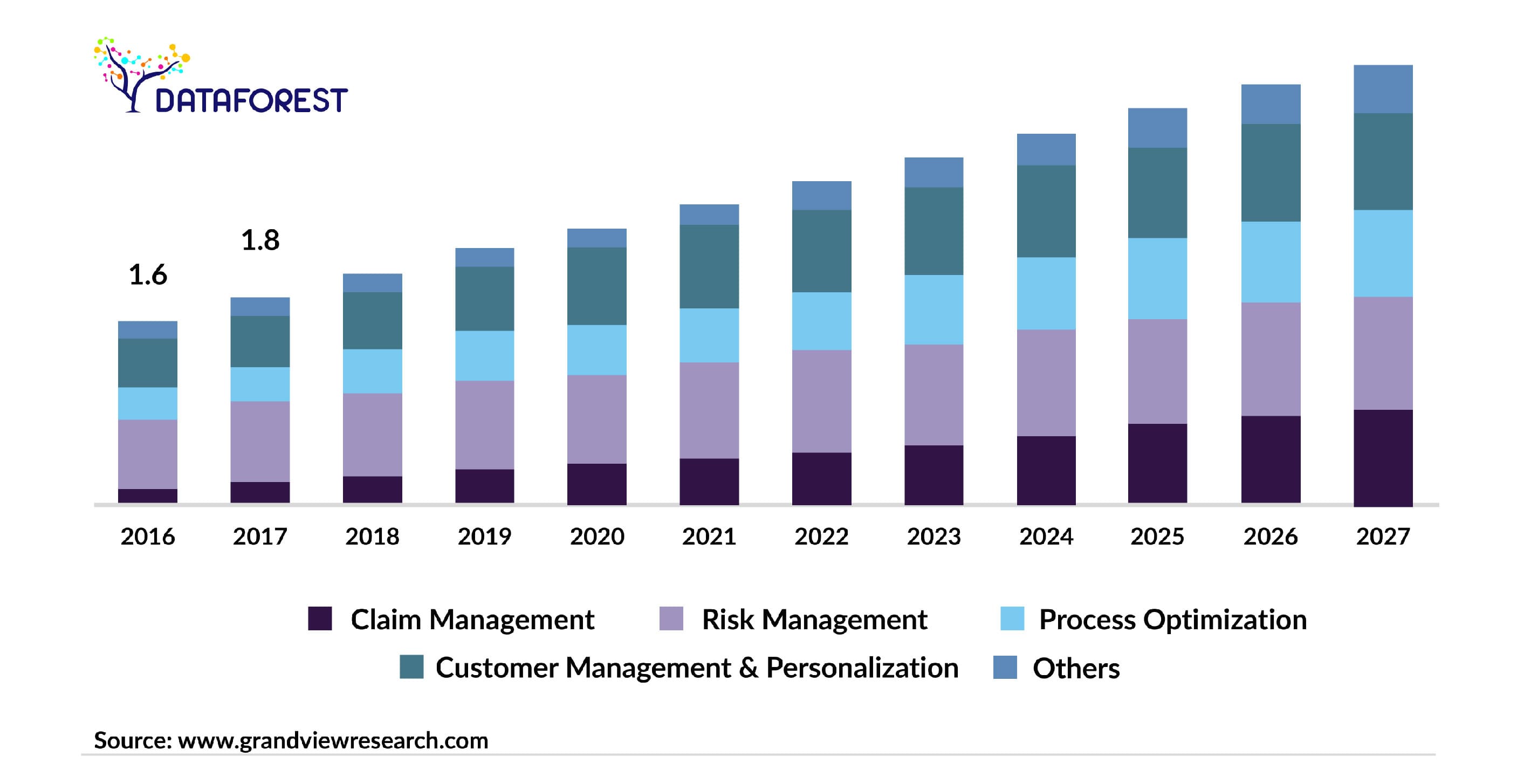
US Insurance Analytics Market Size, by application, 2016-2027 (USD Billion)
Data Science in Insurance: Driving Efficiency and Transforming Operations
Implementing data science in the insurance sector is like turbocharging an engine — it significantly boosts efficiency across various facets of the industry. The role of data science providers (such as DATAFOREST) in this transformation is crucial. They are the architects and builders of the data infrastructure. They offer the tools, technologies, and expertise necessary to harness the power of data science. They work closely with insurance companies to understand their needs and challenges and tailor solutions to meet these requirements. This collaboration is a two-way street, with insurers providing the domain knowledge and data and data science providers bringing in the technological muscle. Please complete the form, and we'll start walking down this street.
FAQ
How does data science contribute to the detection and prevention of insurance fraud?
Data science in insurance contributes to insurance fraud detection and prevention by utilizing advanced AI analytics and machine learning algorithms to identify unusual patterns and anomalies in claims data. These sophisticated tools enable insurers to swiftly pinpoint potentially fraudulent activities, reducing the incidence and impact of fraud on their operations.
What role does predictive modeling play in insurance, and how does it impact policy pricing and underwriting?
Predictive modeling in data science in insurance plays a crucial role by analyzing historical data to forecast future risks and trends, thereby informing policy pricing and underwriting decision-making. This approach allows insurers to set premiums more accurately based on individual risk profiles, leading to fairer consumer pricing and more efficient company risk management.
How can data science be used to personalize insurance products and pricing based on individual customer behavior?
Data science in insurance enables the personalization of insurance products and pricing by analyzing individual customer behavior, such as driving patterns or health habits, using advanced algorithms and machine learning. This analysis allows insurers to tailor policies and pricing to each customer's unique risk profile, offering more accurate and customized insurance solutions.
How does data science enable insurance companies to predict and address customer churn?
Data science in insurance empowers insurance companies to predict customer churn by analyzing patterns in policy renewals, customer interactions, and feedback, identifying signals that a customer may be likely to leave. Utilizing these insights, insurers can proactively implement targeted retention strategies, offering personalized incentives or addressing specific concerns to retain their clientele.
How can insurance companies build a data-driven culture within their organizations to promote data science adoption?
Insurance companies can build a data-driven culture by investing in training and resources to enhance data literacy across all levels of the organization, emphasizing the importance and value of data in decision-making processes. Additionally, fostering an environment that encourages experimentation, data sharing, and collaboration between departments can further embed data science principles into the company's core operational strategy in the context of data science in insurance.
Can data science be used to improve insurance products and services for niche markets and specialized industries?
Yes, data science in insurance can be utilized to improve insurance products and services for niche markets and specialized industries by analyzing specific trends, risks, and customer needs unique to those sectors. This targeted analysis enables insurers to develop highly tailored insurance solutions that effectively address the distinct challenges and requirements of niche and specialized markets.