In the competitive digital marketplace, personalized product recommendations are vital in shaping consumer behavior and driving brand loyalty. Notably, 78% of customers are more inclined to repeat purchases from brands that offer a personalized approach, as highlighted by McKinsey's report on personalization. Such compelling statistics underscore personalized product recommendations' transformative impact on customer retention and sales.
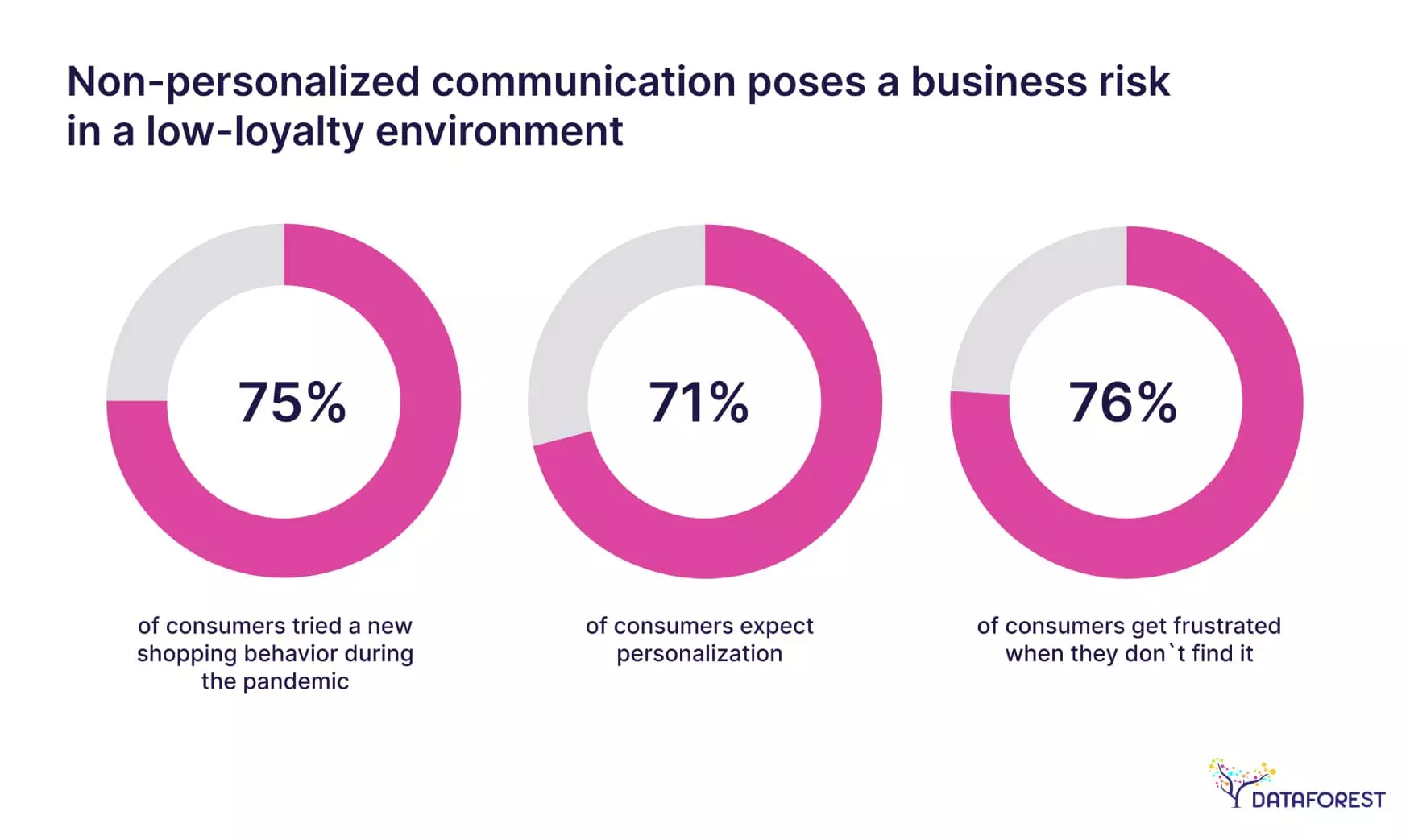
Indeed, the efficacy of personalized product recommendations is substantial. Monetate's study reveals that 75.5% of businesses see a positive return on investment from personalization initiatives, a sentiment echoed across various industries.
With the ascent of ecommerce and shoppers' readiness to exchange behavioral data for a more streamlined shopping experience—90% according to a SmartHQ survey—the timing is ideal for online retailers to incorporate personalized product recommendations. Such recommendations are correlated with increased time on site and reduced price comparison on platforms like Amazon, indicating a strong customer preference for customized shopping experiences.

https://helloretail.com/ecommerce-personalization-in-2024/
Personal value is a significant currency in today’s market, with around 84% of customers emphasizing the importance of being recognized as individuals to gain their business. McKinsey further enlightens us on the potential of personalization in fostering customer loyalty, which reduces conversion costs and fortifies long-term consumer relationships.
The advancements in AI have revolutionized ecommerce, and as 2025 unfolds, the trend only intensifies. G2's insights on ecommerce personalization software indicate that AI-driven tools achieve ROI milestones more swiftly than their non-AI counterparts.
As Chrome phases out third-party cookies at the beginning of 2024, Google's Tracking Protection signals a shift towards more privacy-conscious user tracking. Retailers must stay alert and compliant with evolving data protection regulations.
Echoing this sentiment, a Bolt survey from December 2023 highlights that over 70% of US digital retailers anticipate AI-driven personalization to impact their business in the forthcoming year significantly. This places hyper-personalization on par with omnichannel strategies, underscoring its critical role in the future of digital retail.

https://www.emarketer.com/content/hyper-personalization-explainer-2024
DATAFOREST delves deep into the essence of product recommendations ecommerce, elucidating their indispensable role in the modern world and outlining the blueprint for businesses to harness this powerful tool effectively.
Elevating Ecommerce Through Personalized Product Recommendations
In the fiercely competitive nature of online retail, personalized product recommendations emerge as a critical element for distinguishing one ecommerce business from another. These recommendations do more than enhance user engagement; they significantly raise conversion rates and help build lasting brand loyalty. This exploration focuses on the pivotal role of personalized product recommendations in digital commerce, emphasizing their importance for a thriving business model.
Understanding the Impact of Personalized vs. Generic Product Recommendations
The distinction between generic and personalized product recommendations is crucial for maximizing customer engagement and boosting conversion rates.
- Generic Product Recommendations: These recommendations often fail to connect personally with users, as they are not tailored to individual needs. This one-size-fits-all approach usually results in a bland shopping experience that does not leverage dynamic customer data, thereby missing opportunities to enhance engagement and escalate ecommerce sales. The lack of personal touch means these recommendations do not resonate deeply with customers, leading to potential disinterest and reduced purchase likelihood.
- Personalized Product Recommendations: Contrasting sharply with generic approaches, personalized recommendations harness advanced algorithms and data analytics to craft offers uniquely suited to each customer. These sophisticated systems predict and deliver highly relevant product suggestions by tapping into specific data points like previous purchases, browsing habits, and individual preferences. This targeted strategy profoundly enhances user interaction on ecommerce sites, making shopping experiences more engaging and increasing the likelihood of conversions.
Boosting Customer Experience and Loyalty with Personalized Ecommerce Tactics
Personalized product recommendations significantly influence immediate sales, customer experience, and brand loyalty.
- Enhancing the Customer Experience: These strategies transform online shopping into a highly responsive and personalized journey by offering recommendations that feel tailor-made. Customers enjoy an effortless and satisfying experience explicitly curated for them. This high level of personalization shows a deep comprehension of customer desires and preferences, markedly improving the overall shopping experience.
- Cementing Brand Loyalty: Providing consistent, customized recommendations helps ecommerce sites demonstrate their commitment to fulfilling individual customer needs. This ongoing personal engagement fosters stronger customer relationships, making consumers more likely to return to a platform that recognizes and caters to their unique tastes and preferences.
Additionally, personalized product recommendations drive an iterative enhancement process within ecommerce operations. Companies can refine their recommendation algorithms by collecting and analyzing customer feedback and behaviors to ensure continued relevance and attractiveness. This cycle of ongoing refinement maintains brand pertinence. It cultivates a dynamic, enduring relationship between the ecommerce business and its customers, promoting repeated visits and building a community of committed followers.
Key Technologies Behind Personalized Product Recommendations
Creating personalized product recommendations entails deploying sophisticated algorithms and technologies that meticulously analyze vast data sets to craft suggestions uniquely suited to each customer. Here’s a detailed look at these critical components:
- Collaborative Filtering: This technique stands out in the realm of personalized recommendations. It examines user behavior patterns and preferences across a collective to predict potential interests. By leveraging data such as purchase histories, browsing habits, and user ratings, these algorithms pinpoint products that align with user tastes and are influenced by the preferences of similar profiles.
- Data Analytics and Machine Learning: To further understand and implement these advanced predictive techniques, services like DATAFOREST's predictive analytics as a service can be invaluable. They offer tailored solutions that harness the power of data science to enhance your recommendation systems, ensuring they are as effective as possible in driving user engagement and sales.
In sum, personalized product recommendations are more than just a mechanism to boost immediate sales; they are an integral strategy for increasing customer satisfaction and loyalty. By weaving personalized recommendations into their broader ecommerce strategy, businesses can deliver a more compelling, efficient, and delightful shopping experience, securing long-term growth and a robust competitive advantage in the online marketplace.
How are the Personalized Product Recommendations Working?
Personalized product recommendations have revolutionized e-commerce by enhancing customer engagement and driving sales. This transformative strategy is rooted in a complex interplay of algorithms, data analytics, machine learning, and artificial intelligence (AI). This section delves into the intricate mechanisms that drive personalized product recommendations, spotlighting how these technologies synergize to provide tailored shopping experiences that resonate deeply with individual users.
Key Technologies Behind Personalized Product Recommendations
Creating personalized product recommendations entails deploying sophisticated algorithms and technologies that meticulously analyze vast data sets to craft suggestions uniquely suited to each customer. Here’s a detailed look at these critical components:
- Collaborative Filtering: This technique stands out in the realm of personalized recommendations. Collaborative filtering examines user behavior patterns and preferences across a collective to predict potential interests. By leveraging data such as purchase histories, browsing habits, and user ratings, these algorithms pinpoint products that align with user tastes and are influenced by the preferences of similar profiles.
- Content-Based Filtering: Focusing on product attributes, this method recommends items by analyzing features like category, brand, and price. It tailors suggestions to mirror the interests shown in past user interactions. It constructs a detailed profile of user preferences, enabling it to offer products that genuinely match individual tastes.
- Matrix Factorization: This statistical technique decomposes user-item interaction matrices to discover latent features that predict user preferences. Our approach to matrix factorization is outlined in our offerings on generative AI, which provides a deeper understanding of how we utilize this technology to refine product recommendations.
- Deep Learning: As a cutting-edge facet of personalized recommendations, deep learning processes complex, unstructured data such as images and text to decode user preferences. Training on extensive datasets enables these models to capture nuanced patterns, substantially elevating the accuracy of personalized product recommendations. Insights into how deep learning is applied to personalize product recommendations can be explored in our article on AI foundation models for significant business innovation.
Tailoring Recommendations with Data Science and AI
Data analytics, machine learning, and AI are pivotal in crafting personalized product recommendations. These technologies collectively refine the recommendation process through the following stages:
- Data Collection and Processing: This initial phase aggregates extensive data from user interactions, purchasing patterns, and demographic insights. Advanced data analytics transforms this raw data into a structured form ready for deeper analysis.
- Feature Engineering: In this crucial step, relevant data attributes are extracted to shape the training datasets for machine learning models. This might include variables like frequency of purchases or preferred product categories, which are instrumental in developing models that accurately predict user preferences.
- Model Training and Optimization: Algorithms such as collaborative filtering and deep learning are trained on historical data to discern complex patterns between users and products. These models are finely calibrated through cross-validation and hyperparameter tuning to minimize errors and optimize recommendation accuracy.
- Real-Time Recommendation Generation: Deployed models generate real-time personalized recommendations, adapting to ongoing user interactions. This dynamic process ensures that each recommendation is timely and relevant, enhancing user experience and engagement.
- Continuous Learning and Adaptation: Personalized recommendation systems are designed to evolve, learning continuously from new user data and feedback. Modern AI techniques facilitate the ongoing refinement of these models, ensuring they stay adequate and relevant as user preferences and behaviors change.
By integrating these sophisticated technologies, personalized product recommendations transform e-commerce experiences, making them highly relevant, engaging, and effective at driving user satisfaction and loyalty. This approach personalizes the shopping journey and significantly boosts engagement and conversion rates, positioning it as a vital strategy in modern digital commerce.
The client identification case involved using advanced analytics and AI technologies to build a recommendation and customer behavior tracking system for a restaurant chain. It highlights how data insights can enhance customer loyalty and improve service quality by personalizing user experiences. Although it's focused on a restaurant setting, the underlying principles of customer data analysis and personalized recommendations apply to ecommerce environments.
Understanding the Types of Personalized Product Recommendations
Personalized product recommendations utilize advanced data analytics and algorithms to offer tailored suggestions that enhance user experience, engagement, and sales conversion rates. Each type leverages specific data and techniques to create a personalized shopping journey.
Unpacking Behavior-Based Recommendations
Behavior-based recommendations harness user-generated data from various digital interactions to create customized product suggestions. This method leverages a user's historical online behaviors, such as browsing patterns, purchases, and product ratings, to forecast future buying preferences and suggest relevant products.
Mechanics of Behavior-Based Recommendations
These recommendation systems collect and analyze detailed user interaction data to construct a profile that reflects personal tastes and preferences. For instance, if a customer frequently purchases novels in the mystery genre, the system might suggest newly released mystery books or related genres like thrillers.
Advantages of Behavior-Based Recommendations
- Tailored Experiences: These recommendations offer a customized shopping experience by aligning product suggestions with individual user behaviors and preferences.
- Contextual Relevance: They ensure the relevancy of the recommendations, increasing the user’s likelihood of engagement.
- Boost in Engagement: Behavior-based recommendations keep users engaged by providing relevant suggestions and encouraging them to spend more time on the platform.
- Conversion Enhancement: Personalized recommendations effectively convert browsing into sales by showcasing products users are likelier to purchase based on their past behaviors.
Decoding Contextual Recommendations
Contextual recommendations provide personalized product suggestions by considering the real-time context of user interactions, including factors like geographic location, device used, and the time of day.
Functionality of Contextual Recommendations
These systems use real-time data to offer product suggestions that suit the user's current situation—for example, suggesting umbrellas and raincoats on a rainy day or promoting a local event when the user is nearby.
Benefits of Contextual Recommendations
- Situational Relevance: Recommendations are dynamically aligned with the user's current context, making them highly relevant and likely to be acted upon.
- Timely Suggestions: These recommendations consider elements like time and location and offer timely suggestions that meet immediate user needs.
- Enhanced User Experience: They contribute to a seamless and intuitive user experience by adapting to the user's current environment.
- Conversion Optimization: Timely and relevant suggestions can lead to higher conversion rates, as they are more likely to meet the user's immediate needs.
Exploring Similar Product Recommendations
Similar product recommendations introduce users to products that share characteristics with items they have shown interest in, aiding discovery and comparison.
How Similar Product Recommendations Function
By analyzing product attributes such as brand, size, color, and style, these systems identify and suggest similar items to those the user has previously liked or purchased.
Advantages of Similar Product Recommendations
- Enhanced Discovery: They help users discover new products similar to those they like, broadening their shopping experience.
- Effective Cross-Selling: These recommendations encourage users to explore complementary products, enhancing potential sales.
- Improved Shopping Satisfaction: By suggesting similar items, they enhance the user's shopping experience, making it easier to find desired products.
- Sales Increment: They can increase sales by suggesting additional items that align with the users' established preferences, effectively using their past behavior to guide future suggestions.
Each type of personalized product recommendation plays a crucial role in modern ecommerce strategies. By understanding and implementing these customized recommendation systems, businesses can significantly enhance the online shopping experience, leading to higher engagement, satisfaction, and sales. This holistic personalization approach meets customer expectations and drives business growth through improved ecommerce metrics.
Maximizing Impact with Personalized Product Recommendations Across Digital Platforms
Personalized product recommendations are integral to modern digital marketing strategies. They provide tailored experiences that resonate deeply with individual customers across varied platforms. Implementing these recommendations effectively involves strategic planning and a deep dive into customer data and behaviors, enhancing user experience on e-commerce sites, mobile apps, and email campaigns.
Implementing Personalized Product Recommendations Strategically
Personalized Product Recommendations on E-commerce Sites
- Enhanced Product Page Engagement: Implement customized product recommendations on individual product pages, utilizing users' browsing and purchase history to suggest relevant or complementary items. This tactic enhances the user experience and increases the potential for upselling.
- Optimized Cart Recommendations: Leverage personalized product recommendations during checkout to suggest additional items that enhance or complement the user’s current selections, effectively increasing the average order value.
- Customized Homepage Displays: Personalize the homepage to display products or promotions specifically targeted based on the user's previous interactions and preferences, such as highlighting trending products or personalized discounts.
Personalized Product Recommendations via Mobile Apps
- Seamless In-App Recommendations: Integrate personalized product recommendations within the app’s interface. This ensures a cohesive and engaging user experience by directly displaying products tailored to the user's past preferences on the home or category screens.
- Targeted Push Notifications: Utilize personalized product recommendations through push notifications, informed by user behavior and preferences, to promote timely offers or suggest products that align with users' interests and past activity.
- Engaging Interactive Elements: Embed interactive elements like quizzes or surveys within the app to collect user preferences and generate more accurate personalized product recommendations, enhancing user engagement and data collection.
Enhancing Email Campaigns with Personalized Recommendations
- Dynamic Email Content: Incorporate personalized product recommendations into emails, tailoring content based on the user’s past behaviors and preferences to showcase products that echo previously viewed or purchased items.
- Utilizing Behavioral Triggers: Employ personalized product recommendations in emails triggered by specific behaviors, such as cart abandonment or past browsing activities, to remind users of their interests and nudge them towards conversion.
- Strategic List Segmentation: Segment email marketing lists based on detailed criteria such as user demographics, past behaviors, and engagement levels to deliver highly targeted and relevant personalized product recommendations.
Case Studies Highlighting Effective Personalized Recommendations
- Amazon's Precision Targeting: Amazon excels in personalized product recommendations by analyzing extensive data on user interactions, enabling features like "Customers who bought this also bought" and personalized browsing suggestions, significantly boosting user engagement and sales.
- Spotify’s Curated Experiences: Spotify uses personalized product recommendations to craft user-specific playlists and music suggestions based on detailed analysis of listening habits, enhancing user satisfaction and retention.
- Netflix’s Custom Content Suggestions: Netflix provides personalized product recommendations for TV shows and movies by analyzing viewing histories and user ratings, offering tailored content that increases viewer engagement and subscription loyalty.
Best Practices for Optimizing Personalized Product Recommendations
- Comprehensive Data Analysis: Collect and scrutinize data from all user interactions across platforms to understand behaviors and preferences deeply. Employ advanced analytics to spot trends and inform personalized product recommendations.
- Continuous Optimization through Testing: Regularly test and refine recommendation algorithms and user interfaces to determine the most effective strategies for engaging users and converting sales.
- Prioritize Relevance and Value: Ensure personalized product recommendations are relevant and valuable, enhancing the user's experience and encouraging further interaction and conversion.
- Uphold Privacy and Transparency: Maintain transparency about data usage and honor privacy preferences. Communicate data-handling practices and provide users with straightforward privacy controls.
- Monitor and Adjust Based on Performance Metrics: Track performance indicators like click-through and conversion rates from personalized product recommendations to assess effectiveness and make data-driven adjustments for continual improvement.
By integrating these strategies, businesses can fully leverage personalized product recommendations to enhance user engagement, increase sales, and provide a seamless and enjoyable digital shopping experience.
Harnessing Personalized Product Recommendations for Enhanced Engagement, Conversion, and Performance
Personalized product recommendations are critical tools for modern businesses aiming to elevate customer engagement, increase conversions, and boost overall performance. These recommendations, tailored through sophisticated algorithms and comprehensive data analysis, create compelling user experiences that can significantly uplift satisfaction, loyalty, and revenue streams.
Strategic Audience Segmentation and Personalized Content Delivery
Effectively segmenting your audience and personalizing content are foundational to maximizing the impact of personalized product recommendations. Here’s a deeper look into how strategic segmentation and tailored content can escalate user engagement and optimize performance:
- Precision in Audience Segmentation: By categorizing your audience into well-defined segments based on detailed criteria—such as product preferences, browsing habits, purchase history, and demographics—you can craft personalized product recommendations that are exceptionally relevant and engaging.
- Customized Content Recommendations: Offering personalized content recommendations that align with individual user interests, preferences, and historical interactions can significantly enhance user engagement. This approach encourages further exploration of your offerings and builds a deeper connection with your audience.
- Dynamic Personalization of Content: Implement dynamic content personalization to adapt personalized product recommendations in real-time, reflecting the user’s current behaviors and situational context. This method ensures the recommendations remain pertinent and compelling, enhancing user engagement and satisfaction.
Enhancing User Experience with Tailored Recommendations
Personalized product recommendations are instrumental in refining the user experience by providing content that is not only relevant but also engaging and user-specific. Here’s how they can transform the user experience:
- Integrated User Journeys: Seamlessly embed personalized product recommendations across various customer touchpoints—on websites, mobile apps, or during email interactions. Ensure these recommendations are intuitive and enrich the overall user experience without overwhelming it.
- Predictive Personalized Recommendations: Employ predictive analytics to foresee user needs and offer personalized product recommendations that resonate with their anticipated requirements and interests. This proactive approach satisfies existing needs and captivates users by addressing their unexpressed desires.
- Engagement through Interactivity: Enhance the discovery process with interactive elements such as quizzes and surveys that tailor personalized product recommendations. This engagement fosters a more enjoyable and memorable exploration, making the customized recommendation process an integral part of the user experience.
Boosting Conversion Rates through Targeted Recommendations
Personalized product recommendations profoundly improve conversion rates by strategically guiding users through their purchase journeys. Here’s how these recommendations can be optimized to drive conversions:
- Effective Cross-Selling and Upselling: Utilize personalized product recommendations to intelligently cross-sell related products and upsell higher-tier items that align with the user’s preferences and previous purchases. Strategically positioning these recommendations at crucial decision-making points can significantly enhance the average order value and elevate revenue.
- Recovering Abandoned Carts: Implement personalized product recommendations to address abandoned shopping carts by reminding users of forgotten items and suggesting additional products or incentives. This targeted approach re-engages users and encourages them to revisit and complete their purchases.
- Personalized Promotional Strategies: Tailor promotions and discounts to match each user's unique buying behaviors, preferences, and history. Offering personalized discounts on items left in carts or exclusive deals based on user activity can dramatically increase conversion chances, fostering immediate sales and long-term customer loyalty.
By leveraging personalized product recommendations across these strategic areas, businesses can meet their customers' diverse needs and drive significant improvements in engagement, conversion rates, and overall business performance. This comprehensive approach ensures that each customer interaction is optimized for satisfaction and sales, making personalized product recommendations a cornerstone of successful digital marketing and e-commerce strategies.
Enhancing ROI through Metrics and Optimization of Personalized Product Recommendations
The success of personalized product recommendations hinges on meticulously measuring their impact and continuously refining the strategies based on insightful analytics. This approach allows businesses to accurately gauge the effectiveness of their recommendation engines and optimize them to maximize engagement, conversions, and revenue. This section delves into the critical metrics for evaluating personalized product recommendations and outlines practical strategies for A/B testing, ongoing optimization, and sustained performance enhancement.
Vital Metrics for Assessing Personalized Product Recommendations
- Click-Through Rate (CTR): This metric tracks the percentage of users who click on personalized product recommendations compared to the total number of views. A robust CTR signifies that the customized product recommendations resonate well with users, prompting them to explore further.
- Conversion Rate: This key performance indicator measures the proportion of users who complete a purchase or another predefined action after engaging with personalized product recommendations. An optimized conversion rate underscores the effectiveness of these recommendations in navigating users through the sales funnel.
- Revenue Impact: Monitoring the revenue generated from interactions with personalized product recommendations helps quantify their direct financial benefits. This metric is crucial for evaluating the return on investment (ROI) and justifying the allocation of resources toward enhancing recommendation systems.
- Engagement Metrics: Metrics such as time on site, pages visited per session, and bounce rate are vital for understanding how personalized product recommendations influence overall user engagement and satisfaction. Insights from these metrics guide improvements in user experience and content relevance.
- Customer Lifetime Value (CLV) calculates the total value a customer contributes over their entire relationship with a brand. Focusing on enhancing CLV through personalized product recommendations can drive sustained profitability by fostering customer loyalty and repeat business.
Effective Strategies for A/B Testing and Continuous Enhancement
- A/B Testing: Employ A/B testing to experiment with various elements of personalized product recommendations, such as algorithm changes, content placement, and visual appeal. This systematic approach helps identify the most effective configurations that maximize user engagement and business outcomes.
- Optimization of Recommendations: Regularly analyze performance data to refine the algorithms and strategies underpinning personalized product recommendations. Tailoring these elements to align with user behavior and preferences ensures continued relevance and effectiveness.
- Commitment to Continuous Improvement: Adopt a proactive stance on continuously monitoring and adjusting personalized product recommendations. This adaptive strategy responds effectively to evolving market trends, consumer behaviors, and technological advancements, ensuring the recommendations remain impactful.
- Diverse Personalization Tactics: Experiment with various personalization techniques, including collaborative filtering, content-based filtering, and hybrid models. Each method has its strengths and can be tailored to different segments of your audience to optimize the impact of personalized product recommendations.
- Leveraging User Feedback: Incorporate direct feedback from users to refine personalized product recommendations. Surveys, user testing sessions, and feedback forms are invaluable tools for gaining insights into user preferences and pain points, which can inform further refinements to the recommendation system.
By integrating these metrics and strategies into their operations, businesses can create a dynamic and responsive framework for personalized product recommendations that boost immediate sales and enhance customer satisfaction and loyalty in the long term. This strategic approach ensures customized product recommendations remain a powerful tool for achieving competitive advantage and driving sustainable business growth.
Navigating the Complexities of Personalized Product Recommendations
While personalized product recommendations offer substantial benefits for enhancing user engagement and sales, they also introduce significant challenges that can impact their effectiveness and ethical application. Addressing these challenges head-on is crucial for maintaining the integrity of personalized recommendation systems and ensuring they deliver value responsibly.
Identifying and Mitigating Common Challenges
- Data Privacy and Trust: Data privacy is a primary concern in deploying personalized product recommendations. Mismanagement can lead to serious privacy breaches and diminish customer trust.
- Algorithmic Bias and Fairness: Algorithmic bias is another critical issue. Faulty data or algorithms can lead to unfair or discriminatory recommendations against certain user groups, potentially reinforcing negative stereotypes or perpetuating inequalities.
Strategic Solutions and Proactive Measures
- Ensuring Transparency and Consent: Businesses must operate transparently by clearly communicating to customers how their data is being used to generate personalized product recommendations. Gaining users' trust begins with explicit consent mechanisms and straightforward options for customers to manage their preferences.
- Prioritizing Data Security: Robust security measures must be implemented to safeguard customer data in personalized product recommendations. This includes employing state-of-the-art encryption, stringent access controls, and advanced data anonymization techniques to protect data integrity and comply with rigorous regulatory requirements.
- Committing to Ethical Data Practices: Companies should adhere to ethical guidelines for the use of data in personalized product recommendations. This includes refraining from collecting unnecessary or overly sensitive data and ensuring that all data use adheres to established privacy standards and respects customer rights.
- Promoting Diversity and Algorithmic Fairness: To combat bias in personalized product recommendations, designing and testing algorithms emphasizing fairness and diversity is essential. Regular audits should be conducted to identify and address biases, ensuring that all users receive fair and equitable treatment.
- Empowering Users: It is critical to empower customers to manage their data and preferences. Providing tools that allow users to adjust their privacy settings and control how their data is used for personalized product recommendations enhances user satisfaction and complies with privacy regulations. Users should be able to easily opt out of customized recommendations, giving them ultimate control over their interaction with your platform.
Businesses can effectively manage the complexities of personalized product recommendations by carefully considering these challenges and implementing thoughtful solutions. This approach enhances customer satisfaction and trust and ensures that deploying these technologies adheres to ethical standards, fostering a positive and inclusive user experience.
Elevating Personalized Product Recommendations with DATAFOREST
Personalized product recommendations are at the forefront of a transformative era in customer engagement. They can revolutionize both the approach to and efficiency of online shopping. By harnessing advanced algorithms and data analytics, businesses can provide experiences that are not merely tailored but uniquely resonant with each customer, significantly enhancing user satisfaction and conversion rates.
However, implementing personalized product recommendations is full of challenges. Concerns regarding data privacy and potential biases in algorithms necessitate a rigorous framework within which these tools operate. Businesses must navigate these complexities with a focused commitment to ethical practices, ensuring personalized product recommendations enhance customer trust and data integrity rather than compromise.
Embracing personalized product recommendations can dramatically increase the efficacy of ecommerce strategies, propelling customer engagement and boosting sales. Yet, success in this area requires more than just integrating technology; it demands a steadfast dedication to ethical practices, data security, and a transparent approach to customer interaction.
Integrating personalized product recommendations offers a promising path for companies ready to leap into the future of ecommerce. Explore the potential of personalized product recommendations with DATAFOREST’s cutting-edge solutions. Whether you want to refine your marketing strategies with data-driven insights or enhance your operational efficiency through DevOps solutions, Dataforest can elevate your business.
Harness the power of personalized product recommendations and transform your digital strategy by visiting DATAFOREST’s services. Engage with us today and ensure your enterprise remains at the forefront of the ecommerce revolution, leveraging technology to build enduring, valuable customer relationships.
FAQ
How do I ensure that personalized recommendations are ethical and compliant with data privacy regulations?
To guarantee that product recommendations in ecommerce meet ethical standards and comply with data privacy regulations, it is paramount to embody transparency with customers about the types of data being harnessed and their specific utilization. It is imperative to secure explicit consent before the collection of any personal data, ensuring that customers are thoroughly informed. Strategies such as minimizing the data gathered and employing techniques like anonymization or pseudonymization serve to shield customer privacy. By embracing these approaches, recommendations boost engagement while steadfastly adhering to legal frameworks and maintaining ethical integrity.
Are there any industry-specific considerations for implementing personalized recommendations?
Indeed, implementing personalized recommendations within various industries necessitates carefully considering industry-specific statutes, particularly in sensitive realms such as healthcare and finance. These sectors are governed by rigorous compliance mandates that demand meticulous adherence to ensure personalized recommendations effectively enhance customer engagement without breaching regulatory confines. Acquainting oneself with these legal constraints is critical to fostering a recommendations system that not only captivates but also complies.
How can I ensure the security of my customers' data when using personalized recommendations?
The security of customer data emerges as a pivotal concern when deploying recommendations personalized product systems. To fortify this data, robust encryption protocols must be implemented, stringent access controls must be enforced, and regular security audits must be conducted. Additionally, educating your team on the paramountcy of data security and establishing a proactive stance towards potential data breaches are indispensable measures to perpetuate trust and uphold the integrity of your personalized recommendations infrastructure.
How can I begin implementing personalized recommendations in my business?
Embarking on integrating personalized product recommendations into your ecommerce framework entails clearly delineating objectives and identifying requisite data inputs. Opting for appropriate algorithms and assembling or acquiring the necessary technological infrastructure are foundational steps. It is essential that each phase of the implementation process is marked by thorough testing and strict adherence to both legal standards and best practices, thereby harnessing recommendations to boost engagement significantly.
What data is necessary for creating effective personalized recommendations?
To forge effective recommendations personalized product strategies, an extensive dataset is indispensable. This dataset should encompass user demographics, behavioral inclinations, explicit preferences, and contextual insights. Aggregating and analyzing these data types enable businesses to devise precise and highly pertinent product recommendations, amplifying user engagement and enhancing overall satisfaction.