With Traditional BI, you'd bug the IT guys to pull some numbers and then wait forever for them to make a fancy spreadsheet. By the time you get it, the sale's over! With Self-Service BI by artificial intelligence, you hop on a computer, grab the data you need, and the software does most of the work. It finds patterns, makes graphs, and tells you what's probably causing the problem. Self-service BI with AI gives everyone superpowers to understand their data. Book a call, get advice from DATAFOREST, and move in the right direction.
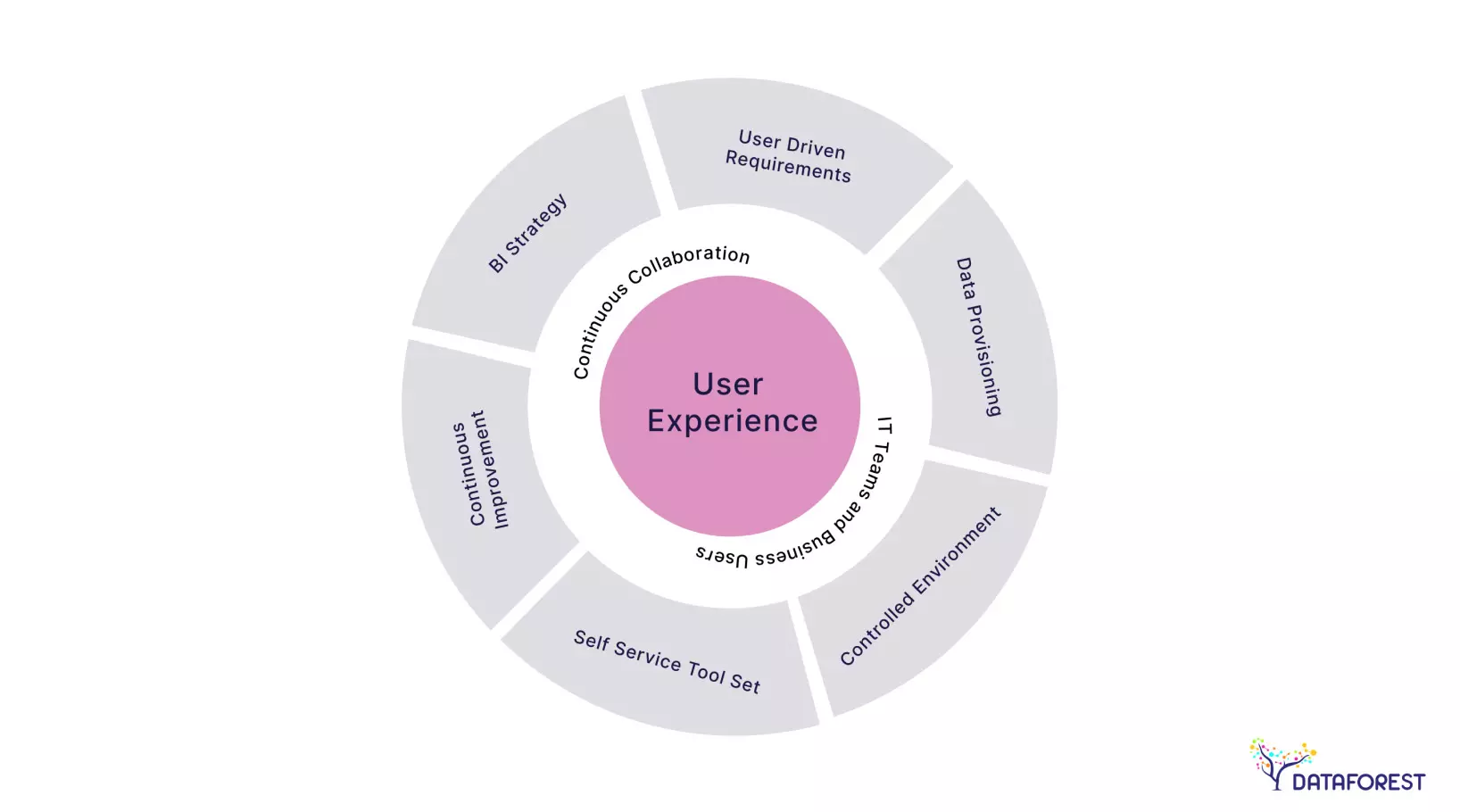
Key Features of Traditional BI
Traditional Business Intelligence (BI) refers to the earlier data analysis and reporting approach, where IT departments primarily handled data management and insight generation.
In traditional BI, data is stored and managed in a centralized location, often a data warehouse. This means that IT teams are responsible for:
Data Collection: Gathering data from various sources (e.g., databases, spreadsheets, applications).
Data Cleaning: Ensuring data accuracy, consistency, and completeness.
Data Transformation: Converting data into a suitable format for analysis.
Data Storage: Storing data in a centralized repository for easy access.
Traditional BI relies heavily on data analysts and IT experts to extract meaningful insights from data. These experts:
Build Reports: Create static reports based on specific business requirements.
Develop Dashboards: Design interactive visualizations to monitor key performance indicators (KPIs).
Conduct Analysis: Perform complex data analysis to uncover trends and patterns.
While this approach ensures the quality of insights, it can be time-consuming and limits business users' ability to explore data independently.
Self-Service BI – Putting Data in Hands
Self-service BI is a modern approach to data analysis that gives everyday business users the tools to explore and analyze data independently. Unlike traditional BI, it puts control in the hands of the people who need the insights most.
Self-service BI tools are designed with the non-technical user in mind.
Intuitive Interfaces: Simple drag-and-drop functionality and easy-to-understand visualizations.
Pre-built Templates: Ready-made reports and dashboards to jumpstart analysis.
Natural Language Processing: The ability to ask questions in plain language to get answers.
By breaking down barriers between data and users, self-service BI:
Speeds Up Insights: Business users quickly explore data and find answers without waiting for IT.
Fosters Data Literacy: Encourages a data-driven culture by empowering everyone to use data effectively.
Improves Decision Making: Allows faster, more informed decisions based on real-time data.
Traditional vs. Self-Service BI: A Head-to-Head Comparison
A comparative analysis of Traditional and Self-Service BI examines the key differences and similarities between these two approaches to data analysis. By comparing factors such as user control, speed, cost, and scalability, organizations determine the best fit for their specific needs.
User Autonomy and Flexibility
Traditional BI: Users have limited autonomy. IT controls data access and analysis, restricting user flexibility.
Self-Service BI: Offers high levels of user autonomy. Business users explore data independently, creating customized reports and dashboards.
Speed of Deployment and Decision-Making
Traditional BI: Deployment is time-consuming because IT is involved in every step. Decision-making is often delayed as users rely on IT for insights.
Self-Service BI: Enables faster deployment and time-to-insight. Business users quickly access and analyze data, accelerating decision-making.
Scalability and Cost Efficiency
Traditional BI: Scaling can be challenging as demand for reports and analysis grows. IT resources may become stretched thin, leading to increased costs.
Self-service BI is more scalable, accommodating increasing user demands without overwhelming IT. Reducing IT involvement in routine tasks can lead to cost reductions.
If you think this is your case, then arrange a call.
Choosing the Right BI Tool
Selecting the optimal BI tool for your business involves carefully evaluating your specific needs, considering various factors, and making informed comparisons.
Evaluation Process:
- Clearly articulate your business goals and data needs.
- Explore available options based on your requirements.
- Select a few promising tools for further evaluation.
- Test the shortlisted tools with real-world data and use cases.
- Compare the performance of each tool and choose the best fit.
Considerations for Small Enterprises
- Limited resources often necessitate cost-effective solutions with a focus on core functionalities.
- User-friendly interfaces and minimal training requirements are crucial due to smaller IT teams.
- Cloud-based solutions are often preferred for flexibility, scalability, and reduced upfront costs.
- Data volume and complexity – typically handle smaller and less complex datasets.
- Prioritize tools that provide insights into KPIs directly relevant to the business.
Considerations for Large Enterprises
- Vast amounts of data from multiple sources require data integration and processing capabilities.
- The tool should accommodate growth in data volume, user base, and analytical demands.
- Strict data protection and regulatory requirements necessitate advanced security features.
- Tailoring the tool to specific business needs and integrating it with existing systems is crucial.
- Require tools that support complex data modeling, predictive analytics, and machine learning.
Balancing Data Governance with User Freedom
- Striking the right balance between data governance and user freedom is a critical challenge for many organizations. Too much governance stifles innovation and slows decision-making, while too little leads to data quality issues and security risks.
- Clear Communication: Effectively communicate data governance policies and their rationale to users.
- User-Centric Design: Design governance processes with user needs in mind.
- Continuous Improvement: Regularly review and refine governance policies based on user feedback and changing business needs.
- Technology Enablement: Leverage data management and governance tools to automate tasks and reduce administrative burden.
- Risk Assessment: Evaluate the potential risks of different governance approaches and implement appropriate controls.
- For example, establish a centralized data catalog with clear metadata and quality standards. It will empower users to search and discover data independently, promoting data literacy.

Self-Service BI is Dominating the Market
Technology vendors such as DATAFOREST are overwhelmingly focusing on self-service BI solutions. Businesses recognize the value of empowering employees at all levels to access and analyze data. Self-service BI enables faster, more informed decisions while reducing reliance on IT for data analysis, leading to significant cost savings. As for us, promoting data literacy across the organization is a key business objective. Please complete the form and try the power of both Traditional and Self-Service BI approaches.
FAQ
What are the main differences between traditional BI and self-service BI?
Traditional BI is centrally managed by IT, requiring technical expertise and often resulting in slow insights delivery. Self-service BI empowers business users to access and analyze data independently, accelerating decision-making and fostering a data-driven culture.
How does self-service BI enhance user flexibility compared to traditional BI?
Self-service BI grants users direct control over data exploration and analysis, eliminating the need to rely on IT for every request. This empowers them to experiment with different data perspectives and create custom reports, fostering a more agile and responsive approach to decision-making.
What are the cost implications of implementing traditional vs. self-service BI?
Traditional BI often incurs high upfront costs due to complex infrastructure, specialized IT resources, and ongoing maintenance. Self-service BI typically has lower initial costs and ongoing expenses as it relies on user self-sufficiency and cloud-based solutions.
Which BI model is more suitable for small businesses and why?
Self-service BI is generally more suitable for small businesses. It aligns with their need for rapid insights, cost-efficiency, and user empowerment. With limited IT resources, small businesses benefit from tools that allow employees to independently explore data and make data-driven decisions.
Can traditional BI and self-service BI be integrated within the same organization?
Traditional and self-service BI can be integrated within the same organization. Traditional BI can handle complex data modeling and governance, while self-service BI empowers users with data exploration and visualization. By combining these approaches, organizations achieve a balance between data control and user autonomy, maximizing the value of their data.
What are the key features to consider when choosing a BI tool?
When selecting a BI tool, prioritize data integration capabilities, user-friendliness, and scalability. A robust tool should seamlessly connect to various data sources, offer intuitive interfaces for users of all technical levels, and accommodate growing data volumes and user needs.
How does self-service BI empower non-technical users?
Self-service BI empowers non-technical users by providing intuitive interfaces and pre-built tools that require minimal technical expertise. This enables them to explore data independently, create visualizations, and uncover insights without relying on IT support, fostering a data-driven culture within the organization.
What are the data governance considerations in self-service BI?
Data governance in self-service BI requires a balance between control and freedom. Companies must establish data quality standards, implement access controls, and monitor data usage to ensure data integrity and security. Clear communication of data governance policies is essential to foster user compliance and trust.
How does the scalability of BI tools impact business growth?
The scalability of BI tools is crucial for business growth. A scalable BI tool can handle increasing data volumes and user numbers without compromising performance, allowing businesses to expand operations and gain deeper insights. Conversely, a tool with limited scalability can hinder growth by becoming a bottleneck as the organization expands.
What should businesses prioritize when transitioning from traditional to self-service BI?
Businesses transitioning from traditional to self-service BI should prioritize user training and adoption, data quality and governance, and the selection of a suitable self-service BI tool. These elements are crucial for ensuring a smooth transition and maximizing the benefits of the new approach.