Alternative credit scoring has revolutionized the financial sector by offering enhanced insights into consumer financial behavior and bolstering risk management practices. Traditional credit scoring methods often fail to encapsulate consumers' broader financial profiles, so alternative credit scoring provides a vital bridge. It extends financial inclusivity and refines the accuracy of credit assessments, heralding a new era in economic evaluations.
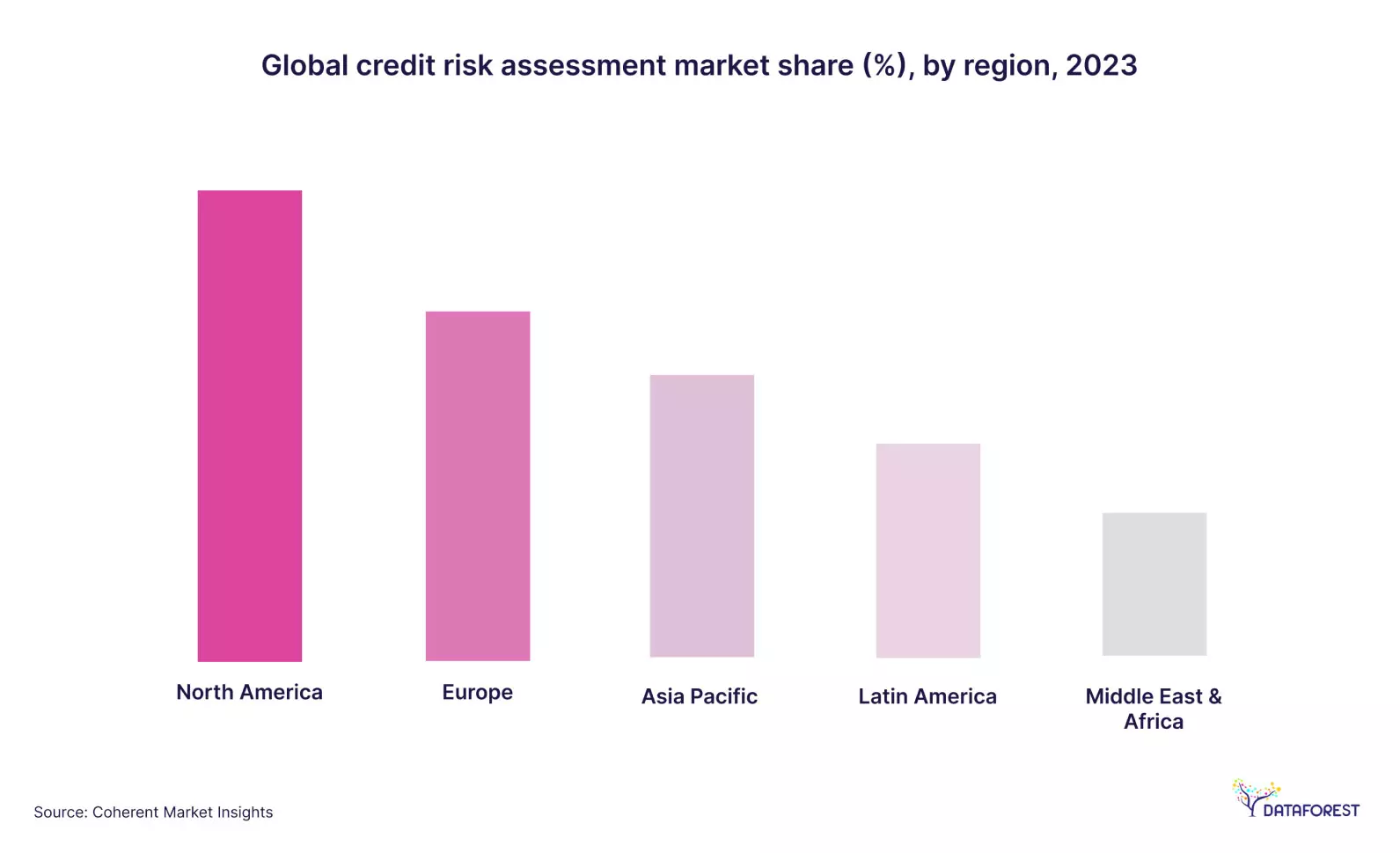
According to the comprehensive Coherent Market Insights report analysis, the credit risk assessment market is poised for significant expansion. From a valuation of US$ 7.31 billion in 2024, it is expected to surge to US$ 18.43 billion by 2030, growing at a compound annual growth rate (CAGR) of 14.1%. This growth is propelled by the escalated integration of big data and analytics alongside an increased volume of credit lending. The dominance of software in this sector, underpinned by sophisticated AI and ML technologies, underscores a pivotal shift towards more dynamic and robust credit scoring practices, particularly in regions like North America, where early technology adoption and stringent regulatory frameworks shape the market.
What Is Alternative Credit Scoring?
Alternative credit scoring transcends traditional boundaries by incorporating diverse and unconventional data sources, such as rental payment histories and incomes from the gig economy. This broadened lens allows lenders to gain a more nuanced understanding of creditworthiness, which is especially beneficial for those with sparse traditional credit histories. It opens up new vistas of financial opportunity, making credit more accessible to a broader segment of the population, particularly the underbanked or those new to credit.
The operational framework of alternative credit scoring comprises two key phases: data acquisition and data integration. Lenders can obtain alternative data through direct submissions from applicants or via technological solutions like Plaid, which offers real-time data access. This flexibility in data handling allows lenders to tailor their scoring models with varying degrees of reliance on alternative data, thereby enhancing the inclusivity and precision of credit scoring systems.
Adopting alternative credit scoring models has profound implications for the lending industry. By leveraging types of alternative data in credit scoring, lenders can mitigate risks more effectively and offer more equitable credit opportunities. This shift supports financial institutions in managing their risk exposure and empowers a broader range of borrowers by providing them with fair access to credit. Ultimately, alternative credit scoring democratizes financial services, fostering a more inclusive financial ecosystem.
As we look to the future, the role of alternative credit scoring in reshaping financial landscapes remains undeniably significant. With its capacity to integrate diverse data points and provide a holistic view of an applicant's economic health, alternative credit scoring models are set to become the cornerstone of modern credit systems. For businesses, staying abreast of these developments is beneficial—it's essential for maintaining competitiveness and innovating in an increasingly data-driven market.
This detailed exploration by DATAFOREST of alternative credit scoring elucidates its pivotal role in modern finance, offering businesses and lenders actionable insights and a strategic edge in a competitive marketplace. As the financial industry continues to evolve, integrating alternative data in credit scoring will undoubtedly play a critical role in shaping the future of lending.
Exploring Alternative Credit Scoring Mechanisms
Decoding the Complexities of Alternative Credit Scoring Models
Alternative credit scoring significantly diverges from conventional credit evaluation methods by leveraging various non-standard data sources. At the core of alternative credit scoring are advanced algorithms that scrutinize varied data elements to determine creditworthiness more precisely.
Conventional credit scoring models primarily depend on data from credit bureaus, such as credit card activity, repayment history, and existing debts. Though these indicators are informative, they only offer a limited perspective on an individual’s or company's financial conduct. Alternative credit scoring adopts an expansive approach, considering aspects often overlooked by traditional credit reports.
A fundamental aspect of alternative credit scoring is its utilization of unconventional data sources. These include utility payments, rental history, employment details, and social media behavior. By integrating a broader spectrum of data points, alternative credit scoring models can craft a more detailed portrait of a person's financial habits and credit status.
For instance, utility payments can reveal much about a person's reliability and fiscal discipline. Regular, timely payments suggest a lower credit risk, while delayed payments pose concerns. Similarly, a person's rental history can reflect their consistency in fulfilling financial commitments, like timely rent payments.
Social media behavior is another unconventional resource that alternative credit scoring models may assess. While atypical, social media can offer insights into a person's lifestyle, hobbies, and expenditure patterns. Frequent mentions of extravagant trips or high-end purchases indicate more discretionary spending, which could influence credit risk evaluations.
Beyond examining individual data points, alternative credit scoring algorithms employ sophisticated analytics to detect patterns and relationships within the data. Machine learning plays a pivotal role here, allowing the models to evolve from historical data and predict future behaviors.
Effectively Assessing Credit Risk with Alternative Credit Scoring
To illustrate the practical application of alternative credit scoring, let’s examine a hypothetical scenario:
Consider Sarah, a small business owner aiming to grow her enterprise by purchasing new equipment. Despite her business’s profitability and longevity, Sarah’s limited credit history could hinder her from meeting traditional lenders’ stringent criteria.
Here, alternative credit scoring could be instrumental for Sarah. Rather than solely focusing on her credit score, lenders could explore various data sources to gauge her creditworthiness more thoroughly.
To scrutinize her cash flow and revenue patterns, lenders might evaluate Sarah's business banking statements. Analyzing the trends in Sarah’s financial transactions could provide deep insights into her business’s fiscal stability and capacity to repay the loan.
Moreover, lenders could factor in alternative data, such as Sarah’s social media interactions and customer reviews. Positive client feedback and active participation on social platforms could suggest a robust customer base and strong market presence, enhancing her creditworthiness.
By adopting alternative credit scoring, lenders can make more nuanced lending decisions, considering Sarah’s comprehensive financial landscape rather than her credit score alone. This approach benefits Sarah by improving her likelihood of loan approval and helps lenders minimize risk by evaluating a more comprehensive array of factors.
By delving deep into the mechanics and effectiveness of alternative credit scoring, we uncover how these innovative models open new avenues for credit access, particularly for underbanked populations and small businesses, thereby transforming the lending landscape. This insight-packed exploration reveals the transformative potential of alternative credit scoring in the financial industry, particularly in enhancing inclusivity and precision in credit assessments.
Revealing the Nuances of Traditional vs. Alternative Credit Scoring
In-depth Comparative Analysis
- Challenges with Traditional Credit Scoring:some text
- Dependence on Narrow Data Points: Traditional credit scoring primarily sources data from credit bureaus. This method focuses on a narrow set of financial indicators like credit card use and loan repayment histories, potentially missing broader financial behaviors. Such an approach may fail to capture a complete picture of an applicant's fiscal responsibility, from utility bill payments to rental histories, which are pivotal in alternative credit scoring.
- Systemic Exclusions: Traditional credit scoring systematically excludes certain groups—such as young adults, newcomers, and underserved populations lacking robust credit histories—from accessing credit. These potentially creditworthy individuals are disadvantaged by traditional models that do not account for their financial management skills.
- Rigidity in Assessment: Traditional credit scoring models' inflexibility often requires accommodating unique financial situations or non-standard income patterns, like freelancers or part-time workers. This rigidity can lead to skewed or unfair credit evaluations, failing to consider an individual's financial landscape.
- Benefits of Alternative Credit Scoring:some text
- Greater Inclusivity: Alternative credit scoring broadens the evaluation framework to include a variety of financial indicators such as rent and utility payment histories, mobile phone usage, and even social media activities. This inclusivity allows for a more holistic view of a person's financial behavior, significantly enhancing the accessibility of credit for broader segments of the population.
- Adaptive and Flexible: Unlike the static models used in traditional credit scoring, alternative credit scoring offers the flexibility to adapt and refine criteria based on emerging data insights. This adaptability makes alternative credit scoring more responsive to different borrower profiles and allows it to evolve with changing economic landscapes.
- Enhanced Predictive Capabilities: Alternative credit scoring harnesses advanced analytics and machine learning technologies to process vast and complex datasets. These technologies enable the discovery of nuanced patterns and correlations that may not be observable through traditional methods, thereby improving the prediction accuracy of credit risk assessments.
- Expansion of Credit Access: By integrating diverse data points, alternative credit scoring facilitates credit access for individuals and businesses previously marginalized by traditional systems. This expansion is crucial for fostering financial inclusion and empowering a larger demographic to engage with the economy through enhanced access to financial services.
In essence, alternative credit scoring democratizes the credit evaluation process by integrating more dynamic, diverse, and inclusive data points, significantly contrasting with the often restrictive and narrow scope of traditional credit scoring. This shift aligns with modern financial behaviors and emerging digital footprints. It champions a more equitable financial ecosystem, ensuring that more individuals and businesses can obtain the credit they rightfully qualify for. Through this more profound analysis, it's clear that alternative credit scoring is not just an innovation in credit assessment but a necessary evolution to meet the demands of today's diverse economic participants.
What Are Alternative Credit Scoring Models?
Alternative Credit Scoring Models: Machine Learning to Psychometric Evaluations
Alternative credit scoring models are revolutionizing the approach to determining creditworthiness. They transcend traditional methods by utilizing diverse data sources and sophisticated analytical techniques. From machine learning algorithms to psychometric assessments, each model varies in approach and application, providing distinct benefits and facing unique challenges.
1. Machine Learning in Alternative Credit Scoring:
Machine learning algorithms are pivotal in developing alternative credit scoring models, enabling the processing of vast and intricate datasets to unearth patterns and predict credit risk with precision. These algorithms apply techniques from supervised to unsupervised learning, adjusting and evolving to better forecast future financial behaviors.
Applications:
- Broad Data Analysis: Machine learning in alternative credit scoring models analyzes extensive data types, from financial transactions to social media engagements, refining the accuracy of credit risk assessments.
- Pattern Recognition: These algorithms excel in detecting subtle financial behaviors and correlations that traditional methods might overlook, thereby enhancing prediction accuracy.
- Customization for Specific Markets: Alternative credit scoring models powered by machine learning can be customized for particular industries or demographic groups, aligning more closely with specific business objectives.
2. Psychometric Assessments in Alternative Credit Scoring:
Psychometric assessments in alternative credit scoring utilize psychological tests to evaluate traits and behaviors correlated with financial responsibility. This innovative approach considers personality aspects like risk tolerance and financial discipline, which traditional credit reports might not reflect.
Applications:
- Deeper Behavioral Insights: Psychometric assessments illuminate financial behaviors and attitudes, providing a layered understanding of an individual's creditworthiness.
- Holistic Credit Evaluation: Integrating psychometric data helps lenders make more nuanced credit decisions, enhancing their ability to judge an applicant's suitability for credit.
- Fraud Detection: These assessments also assist in identifying potential fraud, thereby reducing financial risks for lenders.
3. Utilizing Alternative Data in Credit Scoring:
Alternative credit scoring models incorporate non-traditional data—such as rental payments and utility bills—which provide insights into a person's financial habits. This approach mainly benefits those with sparse or no traditional credit data.
Applications:
- Comprehensive Credit Views: Alternative data enriches understanding of an individual's complete financial profile, especially for those previously invisible under traditional models.
- Increased Credit Accessibility: By evaluating broader data points, alternative credit scoring models enhance financial inclusion, enabling access for underrepresented groups.
- Market Trend Identification: These models help lenders spot emerging trends and craft innovative financial products tailored to meet evolving customer needs.
Tailoring Alternative Credit Scoring Models:
Businesses can adopt alternative credit scoring models or develop bespoke ones tailored to their needs. Custom models can integrate unique industry data, address particular demographic and financial behaviors, or focus on defined risk factors.
Strategic Customization:
- Industry-Specific Models: Custom models can cater to unique sector requirements, incorporating relevant data like vehicle maintenance records for auto lenders or healthcare expenses for medical financing.
- Demographic-Focused Models: Tailoring models to particular demographic groups helps address specific financial characteristics, such as considering gig economy impacts on millennials or retirement income for older adults.
- Risk Prioritization: Businesses might configure models to emphasize certain risk aspects more heavily based on their risk appetite, enabling more aligned credit decision-making. For further insights into how these innovative credit scoring models are applied across various industries, explore our Data insights & Forecasting and Business process automation solutions. These resources illustrate the practical applications of alternative credit scoring models in enhancing predictive accuracy and operational efficiency across sectors.
- Collaborative Model Development: Partnerships with fintech firms or data science specialists can lead to the development of robust, data-rich credit scoring models. Businesses interested in such collaborations should consider our marketing automation and supply chain automation services, which showcase the potential of integrated data-driven approaches to optimize business processes.
This comprehensive investigation of alternative credit scoring models underscores their critical role in modern financial systems, offering a more inclusive, precise, and adaptable approach to credit assessment.
Exploring Types of Alternative Data for Credit Scoring
Integrating Utility and Rental Payments into Credit Assessments
Utility and rental payments are crucial to alternative credit scoring, shedding light on an individual's financial discipline. By incorporating these payments, alternative credit scoring models provide lenders with enriched insights into borrowers' ability to manage their financial obligations effectively.
- Utility Payments: In alternative credit scoring, utility payments, such as those for electricity and gas, serve as reliable indicators of financial stability. Regular, punctual utility payments can suggest a low credit risk, enhancing a borrower's creditworthiness profile. Conversely, inconsistencies in these payments highlight potential credit risks, making utility payment history a valuable tool in alternative credit scoring.
- Rental Payments: Similarly, rental payment history is a potent indicator within alternative credit scoring. Timely rent payments signify a commitment to financial responsibilities, suggesting a lower risk of default. This data enriches the credit scoring process, offering a fuller understanding of an individual's economic health.
Leveraging Bank Account Data and Alternative Financial Services
Exploring bank account activity and using alternative financial services can significantly augment the efficacy of alternative credit scoring by providing a detailed picture of an individual's economic landscape.
- Bank Account Data: Insights from bank account data, including deposit regularity, spending habits, and account balance stability, are integral to alternative credit scoring. This data helps lenders evaluate financial stability and predict creditworthiness more accurately, which is crucial in reducing default risks.
- Alternative Financial Services Usage: Payday loans and check cashing can indicate financial distress. Alternative credit scoring models that analyze these behaviors can better understand risk profiles, especially for individuals whom traditional credit systems might overlook.
Assessing Social Media and Professional Credentials
Social media activities and professional credentials provide additional data layers for alternative credit scoring, offering a broader perspective on an individual's lifestyle and financial potential.
- Social Media and Online Behavior: In alternative credit scoring, social media usage can reveal spending habits and personal interests that reflect financial behavior. Posts about lavish expenditures may hint at higher discretionary spending, influencing credit risk assessments within alternative credit scoring frameworks.
- Education and Professional Credentials: An individual's educational background and professional achievements can also inform credit decisions. Higher education levels and professional success often correlate with financial stability, making this data invaluable for alternative credit scoring.
Benefits of Alternative Credit Scoring: Accuracy and Market Expansion
Enhancing Accuracy in Credit Decisions
Alternative credit scoring improves the accuracy of credit assessments by utilizing a diverse array of data sources beyond the conventional datasets used in traditional credit scoring.
- Reducing Bias: Alternative credit scoring models help diminish biases that traditional credit scoring might perpetuate by broadening the data considered in credit evaluations. This approach leads to fairer, more equitable credit decisions.
- Boosting Predictive Precision: Using varied data points like utility and rental payments, alternative credit scoring enhances predictive accuracy. This not only aids lenders in making informed decisions but also reduces the likelihood of defaults, benefiting the entire credit ecosystem.
Broadening Market Reach and Inclusivity
Alternative credit scoring significantly widens market reach, tapping into demographics traditionally underserved by conventional credit scoring methods.
- Expanding Credit Access: By assessing a more comprehensive range of data, alternative credit scoring models enable credit access for individuals and businesses previously excluded due to insufficient traditional credit histories. This expansion is crucial for fostering broader financial inclusion.
- Promoting Financial Inclusion: Alternative credit scoring drives financial inclusion, empowering more people and businesses to engage fully in economic activities. Access to credit is pivotal for financial stability and growth, making alternative credit scoring a key player in enhancing societal economic well-being.
Using comprehensive data and innovative approaches, alternative credit scoring models refine credit assessment processes and play a crucial role in democratizing financial services. This shift towards more inclusive and accurate credit scoring mechanisms underscores the transformative impact of alternative data in the financial sector.
Navigating the Complexities of Alternative Credit Scoring Models
Addressing Data Privacy in Alternative Credit Scoring
Data privacy is crucial in alternative credit scoring, where extensive personal data utilization is inevitable. While beneficial for comprehensive credit assessments, using diverse data sets brings significant ethical and security challenges that must be meticulously managed.
- Ethical Implications: The moral landscape of alternative credit scoring is complex due to using personal data, such as utility payments and social media activity. Consent and awareness are paramount, as individuals may need to realize their data is being used to determine creditworthiness, raising significant concerns about privacy and ethical data usage.
- Ensuring Transparency and Compliance: To navigate the ethical dilemma, transparency with consumers about data usage, along with stringent adherence to privacy laws such as GDPR or FCRA, is crucial. This ensures that alternative credit scoring practices are lawful but also fair and transparent, fostering trust and credibility.
- Prioritizing Data Security: With the high stakes of personal data management, ensuring robust cybersecurity measures is non-negotiable. Protecting sensitive data against breaches and unauthorized access is essential to maintaining the integrity and trustworthiness of alternative credit scoring systems.
Overcoming Challenges of Model Interpretability
The complexity of models used in alternative credit scoring, particularly those based on machine learning, presents significant interpretability challenges. These complex models, while powerful, can often be opaque, making it difficult for users to understand how decisions are made.
- Decoding Complex Algorithms: The advanced mathematical models at the heart of alternative credit scoring, such as machine learning algorithms, can be incredibly complex and opaque. This complexity can obscure the decision-making process, making it hard for users and regulators to understand how credit decisions are derived.
- Enhancing Model Transparency: Ensuring that alternative credit scoring models are interpretable is crucial for validation and trust. Clear explanations of how models function and make decisions require ongoing efforts to simplify and demystify complex algorithms.
- Navigating Regulatory Landscapes: Regulatory scrutiny of alternative credit scoring intensifies, demanding greater transparency and fairness. Meeting these regulatory demands requires a proactive approach, ensuring that alternative credit scoring models are effective, equitable, and understandable to all stakeholders.
By addressing these critical challenges, alternative credit scoring can realize its potential to provide more inclusive and accurate credit assessments.
The Future of Credit Assessment
In a world where financial inclusivity is paramount, alternative credit scoring is a tool and a revolution in the credit assessment landscape. By harnessing a broad spectrum of data beyond traditional metrics, alternative credit scoring paves the way for broader financial opportunities, especially for those traditionally marginalized by the conventional credit system.
Alternative credit scoring shines as a beacon of innovation, significantly enhancing financial predictions' accuracy and inclusivity. This approach utilizes an eclectic mix of data points—from utility and rental payments to online behaviors and participation in the gig economy—providing a fuller, more nuanced perspective of an individual’s fiscal behavior. This very approach democratizes credit access, aligns with modern-day financial interactions, and meets the diverse needs of today's varied economic actors.
Moreover, the adaptability and flexibility intrinsic to alternative credit scoring models allow for a tailored assessment process sensitive to each applicant's unique situation and promote a more just and equitable credit system. This adaptability challenges the limitations and often constrictive nature of traditional credit systems and underscores the importance of a fair approach in financial assessments.
As we delve deeper into the complexities of data privacy and strive for greater transparency in model interpretability, we must champion and uphold these standards to foster trust and clarity in credit scoring processes, ensuring they are understandable and secure for users and regulators alike.
Embrace the future of financial assessment by engaging with DATAFOREST, where innovation meets inclusivity. Explore our success stories and stay informed with the latest industry insights on our blog. Let’s navigate this evolving landscape together, fostering a fairer, more inclusive financial world.
Ready to transform your financial assessment approach? Contact us to learn how DATAFOREST can tailor our advanced alternative credit scoring solutions to meet your needs. Together, we can redefine what’s possible in the financial industry.
FAQ
1. How can alternative credit scoring help businesses mitigate the risks associated with lending to underserved communities?
Alternative credit scoring is a transformative force in the financial industry, enabling businesses to extend credit to broader population segments. By incorporating data from non-traditional sources such as utility payments, rental histories, and social media activities, alternative credit scoring models provide a more holistic view of an applicant's financial behavior. This comprehensive approach allows lenders to assess the creditworthiness of individuals who might otherwise be overlooked by traditional credit scoring systems, thereby democratizing access to financial services and reducing the risks associated with lending to underserved communities.
2. Are there regulatory barriers that businesses need to navigate when implementing alternative credit scoring models?
Implementing alternative credit scoring models involves navigating a complex regulatory landscape. In regions like the European Union, stringent data protection regulations such as GDPR must be adhered to, while in the United States, the Fair Credit Reporting Act (FCRA) sets data accuracy and privacy standards. Moreover, businesses must ensure that their alternative credit scoring practices comply with fair lending laws, demonstrating that these innovations do not result in discriminatory outcomes. Navigating these regulatory frameworks is crucial for businesses to harness alternative credit-scoring benefits responsibly.
3. Can alternative credit scoring seamlessly integrate into financial institutions' existing credit assessment frameworks?
Alternative credit scoring can seamlessly integrate into existing credit assessment frameworks financial institutions use. This integration enhances traditional models by filling in gaps where data might be sparse or non-existent, especially for underbanked populations. Financial institutions can leverage conventional and alternative data credit scoring to create a more accurate and inclusive credit evaluation process. This synergy helps lenders make more informed decisions and extend credit more confidently across diverse applicant pools.
4. What role does explainability play in alternative credit scoring, significantly when decisions impact individuals' financial opportunities?
In alternative credit scoring, explainability is critical, particularly when decisions significantly impact an individual’s financial opportunities. Explainability ensures that the decisions made by credit scoring models are transparent, understandable, and justifiable. This transparency is vital for maintaining trust and fairness in financial decision-making processes. It ensures that all applicants understand how their credit scores are determined and can contest them if they feel the assessment is inaccurate.
5. How do alternative credit scoring models adapt to evolving consumer behaviors and market dynamics, ensuring their relevance and effectiveness over time?
Alternative credit scoring models are designed to adapt to changes in consumer behavior and market conditions over time. By utilizing advanced machine learning algorithms to analyze vast arrays of data continuously, these models can promptly adapt to shifts in the market or consumer behavior. Regular updates to the algorithms and collaboration with industry experts and regulators help ensure that alternative credit scoring remains relevant and practical, providing businesses with robust tools to manage credit risk dynamically and equitably.