Each data point is a distant celestial body, representing customer behaviors, preferences, purchase patterns, and market trends. Big data acts as the telescope's lens, gathering and focusing these data points to unlock their secrets. It enables e-commerce businesses to peer deeper into the universe of market dynamics. Like an astronomer gazing through the telescope, data analysts and scientists observe and interpret the information unveiled by big data. They scrutinize the stars (data points) to identify constellations (patterns) and celestial phenomena (trends), extracting meaningful insights that guide business decisions. Book a call, get advice from DATAFOREST, and move in the right direction.
Three Vs for Big Data in E-commerce
Big data refers to huge and complex data from various sources, including online platforms, social media, sensors, transactions, etc. It encompasses vast amounts of information that are difficult to process and analyze using traditional methods. The three Vs characterize big data in e-commerce: volume (a large amount of data), velocity (the high speed at which data is generated and processed), and variety (diversity of data types and sources).
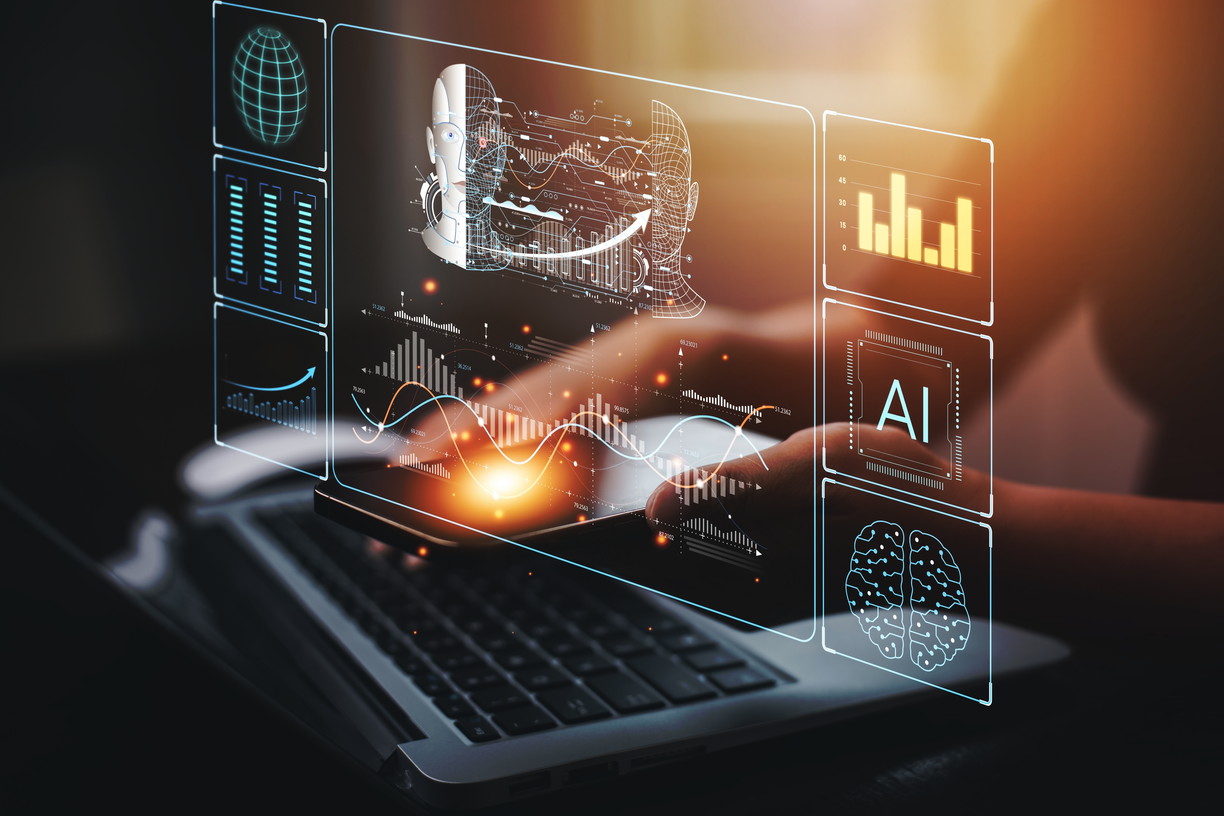
Big data in e-commerce gains a competitive advantage
In the e-commerce industry, big data is crucial in gaining valuable insights and making informed business decisions. Here are some key reasons for big data's importance.
- Personalized customer shopping experience
- Demand forecasting and inventory management by big data in e-commerce
- Price optimization and competitive intelligence
- Fraud detection and risk management with big data in e-commerce
- Enhanced customer service
- Website optimization and user experience of big data in e-commerce
Personalized marketing with big data in e-commerce
Leveraging big data in e-commerce offers numerous benefits that contribute to improved customer insights and enhanced operational efficiency with key advantages.
- Enhanced customer insights
- Personalized marketing campaigns from big data in e-commerce
- Improved operational efficiency
- Real-time customer service by big data in e-commerce
- Competitive advantage
By harnessing the power of big data analytics, e-commerce companies drive growth and optimize business processes.
Retail e-commerce sales worldwide from 2014 to 2026
![Ecommerce Statistics 2022 [data and stats updated monthly]](https://cdn.prod.website-files.com/65d8ee5f025f02594c614c17/66e4364bfde491c5f6459665_65d8ee5f025f02594c61539d_1.jpeg)
Big data in e-commerce — Cosmic Terrain
Imagine big data in e-commerce like the cosmos. The data analysts are like astrophysicists, delving into the mysteries of the universe. They investigate the gravitational pulls of customer preferences, the cosmic collisions of market forces, and the cosmic background radiation of customer sentiment. Their expertise allows them to decipher the celestial language of big data and translate it into actionable insights.
Immense potential for businesses by big data in e-commerce
Big data refers to the enormous volume, velocity, and variety of data generated from various sources, including online platforms, customer interactions, transactions, social media, etc. Big data in e-commerce comprises customer information systems — browsing behavior, purchase history, demographics, and preferences — and data related to products, inventory, pricing, supply chain management, marketing campaigns, and customer feedback. Advanced analytics and machine learning techniques make e-commerce companies extract valuable information from big data, uncover patterns, and detect trends.
Primary characteristics of big data in e-commerce
- Volume refers to the sheer amount of data generated and collected. Big data involves enormous quantities that exceed traditional data methods' capacity. The importance of data continues to grow exponentially as more digital interactions occur.
- Velocity represents the speed at which data is generated, processed, and analyzed. This real-time or near real-time nature of data necessitates efficient processing and analysis techniques to derive timely insights from big data in e-commerce.
- Variety calls for diverse types of data within big data. It rings structured, unstructured, and semi-structured data. Big data includes a mix of all these data types, and effectively managing this variety requires advanced tools and techniques.
Big data in e-commerce: several challenges
E-commerce businesses face several challenges when handling and analyzing large volumes of data.
- Traditional database systems may struggle to handle the massive influx of data, leading to issues with storage capacity, data retrieval speed, and performance with big data in e-commerce,
- E-commerce must adopt technologies like distributed computing frameworks (e.g., Hadoop, Spark) or stream platforms to handle the velocity and variety of data.
- Integrating and consolidating data into a unified format can be challenging, especially when dealing with different data structures and formats according to big data in e-commerce.
- Compliance with data protection regulations, implementing robust security measures, and adopting encryption techniques are essential to safeguard customer data in the face of growing cybersecurity threats.
- Considering big data in e-commerce, businesses need to build a capable data team or collaborate with external partners to improve their expertise in handling and analyzing large volumes of data.
- Scaling up infrastructure, investing in analytical tools, and maintaining data storage and processing capabilities can be costly for e-commerce businesses.
Overcoming these challenges requires a holistic approach that involves implementing scalable infrastructure, adopting advanced analytics tools, and ensuring data quality and security.
Big data in E-commerce and Celestial Bodies
Applications of big data in e-commerce can be represented within the vast expanse of the cosmos, where various celestial bodies symbolize different use cases: personalized recommendation engines shine brightly, supply chain optimization orchestrates the movements of celestial bodies within the vast universe, and customer sentiment analysis is deciphering the cosmic background radiation.
Customer Insights of big data in E-commerce
Customer insights in big data in e-commerce refer to the deep understanding gained from analyzing customer data to uncover patterns, preferences, behaviors, and needs. Personalization is the process of tailoring experiences, content, and offers to individual customers based on their preferences, behavior, and needs.
Derived from big data
Customer insights derived from big data enable e-commerce businesses to:
- By analyzing browsing behavior, purchase history, and product interactions, businesses gain insights into what customers are interested in, their preferences, and the factors influencing their buying decisions within big data in e-commerce.
- Big data analytics identify distinct customer segments based on demographics, purchase patterns, or behavior. This segmentation allows businesses to tailor marketing strategies, product offerings, and customer experiences to specific groups.
- Analyzing historical data and employing predictive modeling techniques include predicting purchase likelihood, customer churn, cross- or upselling, and more. These predictions enable targeted marketing campaigns and personalized recommendations by big data in e-commerce.
Individual preferences with big data in e-commerce
With big data analytics, e-commerce businesses personalize customer experiences in various ways:
- Analyzing customer browsing behavior, purchase history, and similar customer profiles provides personalized product recommendations
- Big data analytics allows businesses to identify individual customer preferences, purchase patterns, and responsiveness to specific offers under big data in e-commerce.
- Personalization with big data extends to dynamic pricing, where businesses adjust prices based on factors such as demand, customer segmentation, and competitor pricing from big data in e-commerce.
- Big data insights help businesses create targeted content and marketing campaigns that resonate with specific customer segments.
Customer insights and personalization driven by big data in e-commerce are powerful tools for enhancing customer experiences and increasing customer loyalty.
Big data in e-commerce — supply chain optimization
Powering big data in e-commerce analytics gains valuable insights and makes data-driven decisions to streamline and enhance supply chain processes. Big data analytics forces e-commerce to optimize supply chain processes, improve operational efficiency, reduce costs, and provide customer satisfaction.
Real case study of using big data in e-commerce
Here's how big data contributes to supply chain optimization in e-commerce:
- Big data analytics enables accurate demand forecasting by analyzing historical sales data, customer behavior patterns, market trends, and external factors, such as seasonality or promotion, to big data in e-commerce.
- Businesses determine optimal stocking levels, reorder points, and replenishment strategies by analyzing real-time inventory data, customer demand patterns, and supplier performance metrics.
- Big data in e-commerce also supports efficient logistics and transportation management. Analyzing data on shipping routes, carrier performance, traffic patterns, and real-time tracking information optimizes delivery routes and selects the most cost-effective carriers.
Enhanced visibility with real-time data analysis
Real-time data analysis in supply chain processes offers several benefits.
- Clear visibility of big data in e-commerce enables businesses to make proactive decisions and respond swiftly to changes, minimizing stockouts, overstock situations, and supply chain disruptions.
- Real-time data analysis enables businesses to detect supply chain bottlenecks, delays, or disruptions as they occur.
- Analyzing real-time operational data allows for timely adjustments in procurement, production, and logistics, leading to reduced costs in the case of big data in e-commerce.
Making data-driven decisions in real-time helps optimize inventory, improve demand planning, enhance logistics efficiency, and ensure a responsive and agile supply chain that meets customer demands while minimizing costs.
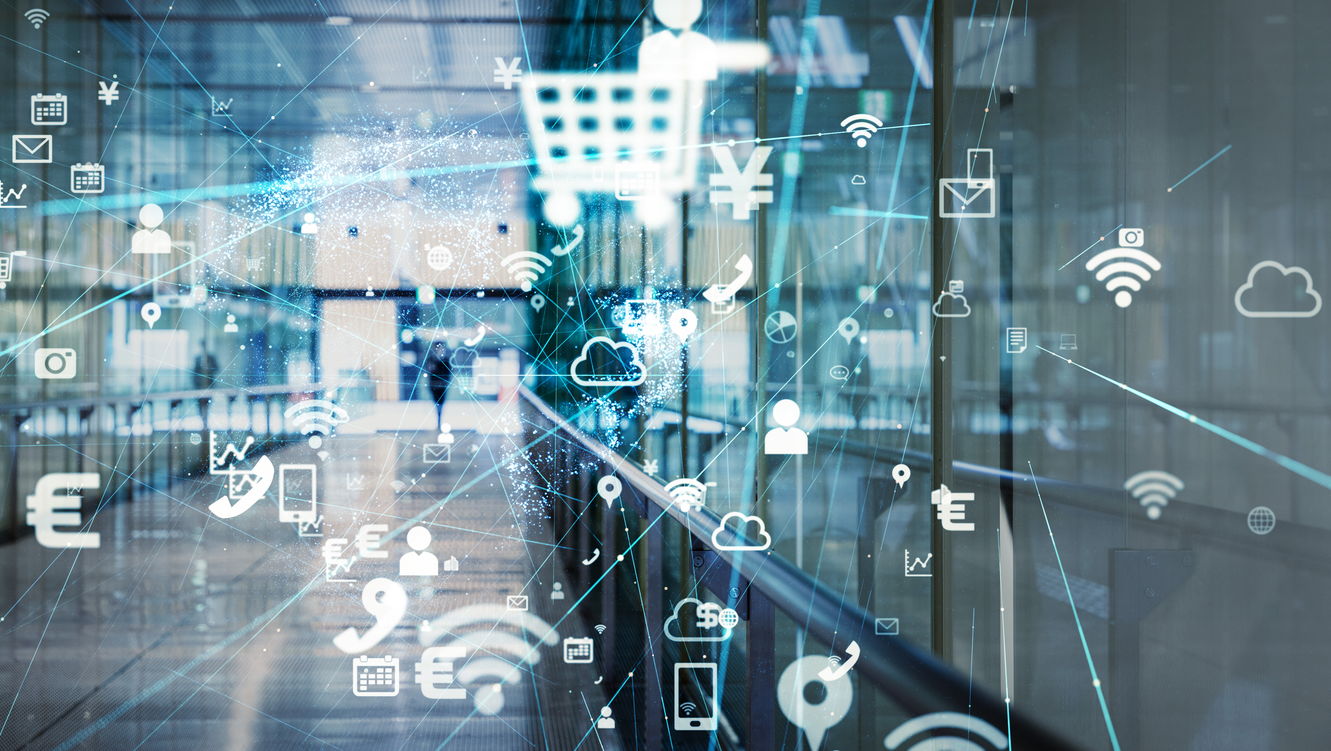
Fraud detection with big data in e-commerce
Big data analytics strengthen fraud detection, enhance security measures, protect customer data, and prevent financial losses. The ability to respond to security threats in real time ensures a secure and trustworthy e-commerce environment, fostering customer confidence.
Detect anomalies with big data in e-commerce help
Big data plays a crucial role in identifying and preventing fraudulent activities in e-commerce by providing the tools and insights needed to detect patterns and suspicious behaviors with data governance.
Big data in e-commerce: aggregation
Big data technologies enable aggregating and consolidating data from multiple sources, creating a comprehensive view of the e-commerce ecosystem. Bringing together diverse data sets analyzes various variables and identifies fraud indicators.
Big data in e-commerce: advanced analytics
Training algorithms on historical fraud data in data retail uncover patterns, anomalies, and risk factors that indicate potential fraud. Machine learning models continuously learn and adapt to new fraud patterns, improving accuracy.
Big data in e-commerce: real-time monitoring
Analyzing incoming data in real-time identifies suspicious transactions, unusual behavior patterns, or deviations from established norms. Real-time monitoring enables us to take immediate action: flagging transactions for manual review or implementing additional security measures.
Big data in e-commerce: behavioral analysis
Tracking historical data on customer interactions, browsing patterns, purchase history, and device information establishes normal behavior profiles for individual customers. Any deviations from these patterns are flagged as potentially fraudulent activities.
Big data in e-commerce: network analysis
Big data analytics allows businesses to analyze network traffic, IP addresses, and device information to detect fraudulent activities associated with botnets, multiple account creation, or identity theft.
Big data in e-commerce: collaborative fraud intelligence
Aggregating and anonymizing data analyze collective fraud patterns and trends, identify emerging threats, and collaborate with other organizations to prevent fraud more effectively. Collaborative efforts improve fraud detection accuracy and enable the industry to respond to new fraud techniques more efficiently.
Big data in e-commerce: adaptive fraud prevention
Businesses continuously analyze data to refine fraud detection models, update rules, and implement new security measures. This adaptability helps companies to stay ahead of fraudsters and minimize potential losses.
Use of machine learning algorithms under big data in e-commerce
Machine learning algorithms and anomaly detection techniques significantly mitigate potential threats and abnormalities in various systems and data.
We know how to handle Big Data; arrange a call and you will know too.
Big Data in E-commerce — Powerful Telescopes
In the vast universe of e-commerce, tools and technologies for big data are like powerful telescopes and space probes that allow businesses to explore, observe, and navigate the intricate cosmic landscape of data. These tools guide stars, helping e-commerce uncover hidden insights, navigate vast data galaxies, and unlock the mysteries of customer behavior and market dynamics.
Popular tools and technologies
Leveraging these tools optimizes operations, enhances customer experiences, and drives growth in the competitive e-commerce landscape.
- Hadoop is an open-source framework that enables distributed processing of large datasets across clusters of computers. It consists of two main components: the Hadoop Distributed File System (HDFS) for distributed storage and the MapReduce programming model for distributed processing. Hadoop is well-suited for big data in e-commerce conception.
- Apache Spark is an open-source, fast, and general-purpose cluster computing system. It provides in-memory processing capabilities, significantly faster than traditional data processing tools like Hadoop MapReduce. Spark supports various programming languages and offers a rich set of libraries, including Spark SQL, Spark Streaming, and MLlib (Machine Learning library). It works perfectly with big data in e-commerce.
- NoSQL (Not Only SQL) databases are non-relational for handling large-scale, unstructured, and semi-structured data. They offer flexible data models, scalability, and high-performance capabilities, making them suitable for managing and querying big data in e-commerce. Popular NoSQL databases used in the e-commerce industry include MongoDB, Cassandra, and DynamoDB.
Other notable tools and technologies used in the e-commerce industry for big data analytics include Apache Kafka, Elasticsearch, Tableau, and Python/R.
Techniques Overview
In the e-commerce industry, dealing with large volumes of data requires efficient data storage, processing, and analysis techniques.
Case Studies of Big Data in E-commerce
Several real-world examples demonstrate successful implementations of big data in the e-commerce industry.
Notable examples of big data in e-commerce techniques
Amazon with big data in e-commerce
Amazon uses customer data to provide personalized product recommendations, optimize pricing strategies, and improve the customer experience. Its recommendation engine analyzes customer browsing and purchase history to suggest relevant products, leading to increased sales and customer satisfaction.
Alibaba with big data in e-commerce
One of the world's largest e-commerce companies, Alibaba, utilizes big data to enhance operations. It employs data analytics to analyze customer behavior, predict demand patterns, and optimize inventory management. In such a way, Alibaba streamlines its supply chain, reduces inventory costs, and delivers products more efficiently.
eBay with big data in e-commerce
eBay uses big data to improve its marketplace dynamics and customer experience. Analyzing data from various sources, including customer behavior, historical transaction data, and market trends, optimizes search results, pricing strategies, and seller performance metrics. It raises the overall user experience and facilitates successful transactions on the platform.
Specific cases
Stitch Fix with big data in e-commerce
This online personal styling service relies on big data analytics to curate personalized fashion recommendations for its customers. The company collects detailed information about customers' style preferences, body measurements, and feedback. By analyzing this data, Stitch Fix's algorithms generate customized clothing selections, leading to high customer satisfaction. They also increase sales.
Netflix with big data in e-commerce
Leading streaming service utilizes big data analytics to provide personalized content recommendations to its subscribers. The platform analyzes user behavior, viewing patterns, and ratings to suggest relevant movies and TV shows. This data-driven approach enhances user engagement, improves customer retention, and fuels Netflix's success in the highly competitive streaming industry.
Walmart with big data in e-commerce
It harnesses big data analytics to optimize inventory management and supply chain operations. By analyzing sales data, weather patterns, and other factors, Walmart forecasts demand, optimizes product stocking levels and minimizes out-of-stock situations. Customers can find the products they need, which leads to increased sales and customer satisfaction.
Using Big Data in Supply Chain Management Solution (2019)

Big Data in E-commerce: Guiding Star and Cosmic Challenge
In the e-commerce universe, big data is both a guiding star and a cosmic challenge. It overcomes black holes of data volume, meteor showers of data variety, and speed of light velocity. Big data also reaches constellations of Artificial Intelligence and Machine Learning, nebulas of Personalization, and dark matter of big data security.
Challenges with big data in e-commerce
Utilizing big data in e-commerce comes with its own set of challenges and limitations.
- Gathering and analyzing customer data must comply with privacy regulations, such as GDPR or CCPA, to ensure the ethical use of personal information.
- Processing large volumes of data is resource-intensive, requiring substantial investments in infrastructure, technologies, and skilled personnel for using big data in e-commerce.
- E-commerce data comes from diverse sources, making data quality a challenge. Inconsistent data leads to inaccurate insights and hinder effective decision-making.
- As e-commerce businesses grow, scaling up data storage, processing, and analysis capabilities to handle this growing data volume is challenging.
- While big data in e-commerce provides valuable insights, interpreting and translating those insights into actionable strategies can be challenging.
- E-commerce businesses become targets for cyber threats. Protecting sensitive customer information and maintaining robust data security measures are critical challenges.
Overcoming these hurdles allows e-commerce to harness the full potential of big data.
Reshaping the industry
Emerging trends in big data for e-commerce are offering new opportunities for innovation.
- AI or ML big data technology analyzes vast amounts of data to identify patterns, trends, and customer behaviors.
- Predictive analytics uses historical data and machine learning algorithms to forecast future trends, customer behavior, and demand under big data in e-commerce.
- Real-time analytics allows businesses to act upon data in real time, enabling immediate responses to customer behavior and market changes.
- NLP techniques extract valuable insights about customer preferences and opinions by analyzing customer reviews, social media interactions, and other textual data.
- Big data enables advanced customer segmentation and personalized marketing strategies. Personalization based on big data insights boosts user engagement and satisfaction with big data in e-commerce.
- Integrating IoT devices in e-commerce generates vast amounts of data from connected devices, such as wearables and smart home devices.
- Augmented Reality (AR) and Virtual Reality (VR) technologies are transforming the e-commerce landscape by providing immersive and interactive experiences to customers.
These emerging trends in big data for e-commerce empower data-driven insights, increase customer experiences, and drive competitive advantage.

Big Data in E-commerce with Provider
DATAFOREST, like other data science providers, holds advanced analytics techniques to extract meaningful insights from large and diverse datasets, qualifying businesses to understand customer behavior, optimize pricing and inventory strategies, and improve operational efficiency. Data science providers also assist in building recommendation engines and personalized marketing campaigns based on data-driven insights, enhancing driving conversions. Additionally, they help in fraud detection and security by developing systems that analyze data patterns to identify and prevent fraudulent activities, safeguarding the integrity of e-commerce transactions.
Are you interested in data science as a service? Please fill out the form; we will use all our skills and experience for your benefit.
FAQ
What is the role of big data in improving customer personalization in e-commerce?
The role of big data in improving customer personalization in e-commerce is to analyze vast amounts of customer data to identify patterns, preferences, and behaviors, delivering tailored experiences, recommendations, and offers based on individual customer needs by big data in e-commerce.
How can big data analytics help e-commerce businesses optimize their pricing strategies?
Big data analytics can help e-commerce businesses optimize their pricing strategies by analyzing market trends, competitor pricing, customer behavior, and demand patterns to determine the optimal price points for products and services.
What are the major challenges in managing and analyzing big data in the e-commerce industry?
The major challenges in managing and analyzing big data in the e-commerce industry include data privacy concerns, resource requirements, data quality and integration, scalability, skill gap, and interpretation of data for actionable insights with big data in e-commerce.
How can big data assist in fraud detection and prevention in e-commerce?
Big data assists in fraud detection and prevention in e-commerce by analyzing large volumes of transactional and behavioral data to identify patterns, anomalies, and suspicious activities, enabling businesses to detect and mitigate fraudulent activities proactively.
What are some popular big data technologies used in e-commerce?
Some popular big data technologies used in e-commerce include Hadoop, Spark, NoSQL databases, and data visualization tools like Tableau and Power BI. They suit business owners’ well-being under big data in e-commerce.
How does big data contribute to effective inventory management in e-commerce?
Big data contributes to effective inventory management in e-commerce by analyzing historical sales data, customer demand patterns, and market trends to optimize stock levels, minimize stockouts and overstocks, and improve overall supply chain efficiency.
What types of data sources are typically utilized in big data analysis for e-commerce?
Typically, big data analysis for e-commerce utilizes various data sources such as transactional data, customer behavior data, website clickstream data, social media data, and external market data.
How can big data help e-commerce businesses identify and mitigate shopping cart abandonment?
Big data helps e-commerce businesses identify and mitigate shopping cart abandonment by analyzing customer behavior data, identifying patterns and bottlenecks in the checkout process, and implementing personalized retargeting strategies to encourage customers to complete their purchases.
How is business intelligence related to conversion rates?
Business intelligence leverages big data in e-commerce to analyze and optimize conversion rates by identifying patterns, trends, and customer behaviors influencing purchasing decisions.