Picture data science as a powerful lens through which we view the world and make important decisions. Historical data fuels it and serves as the glass through which we see insights. But this lens has a hidden flaw: it has been crafted from the glass of history, which, unfortunately, carries the fingerprints of bias. This bias in the glass introduces a distorted view of reality. It highlights certain aspects more than others, casting shadows on specific groups and amplifying the brightness of others. Data scientist’s quest is to calibrate the lens, polish it diligently, and minimize the distortions it introduces. If you need an individual approach to a solution, book a call.
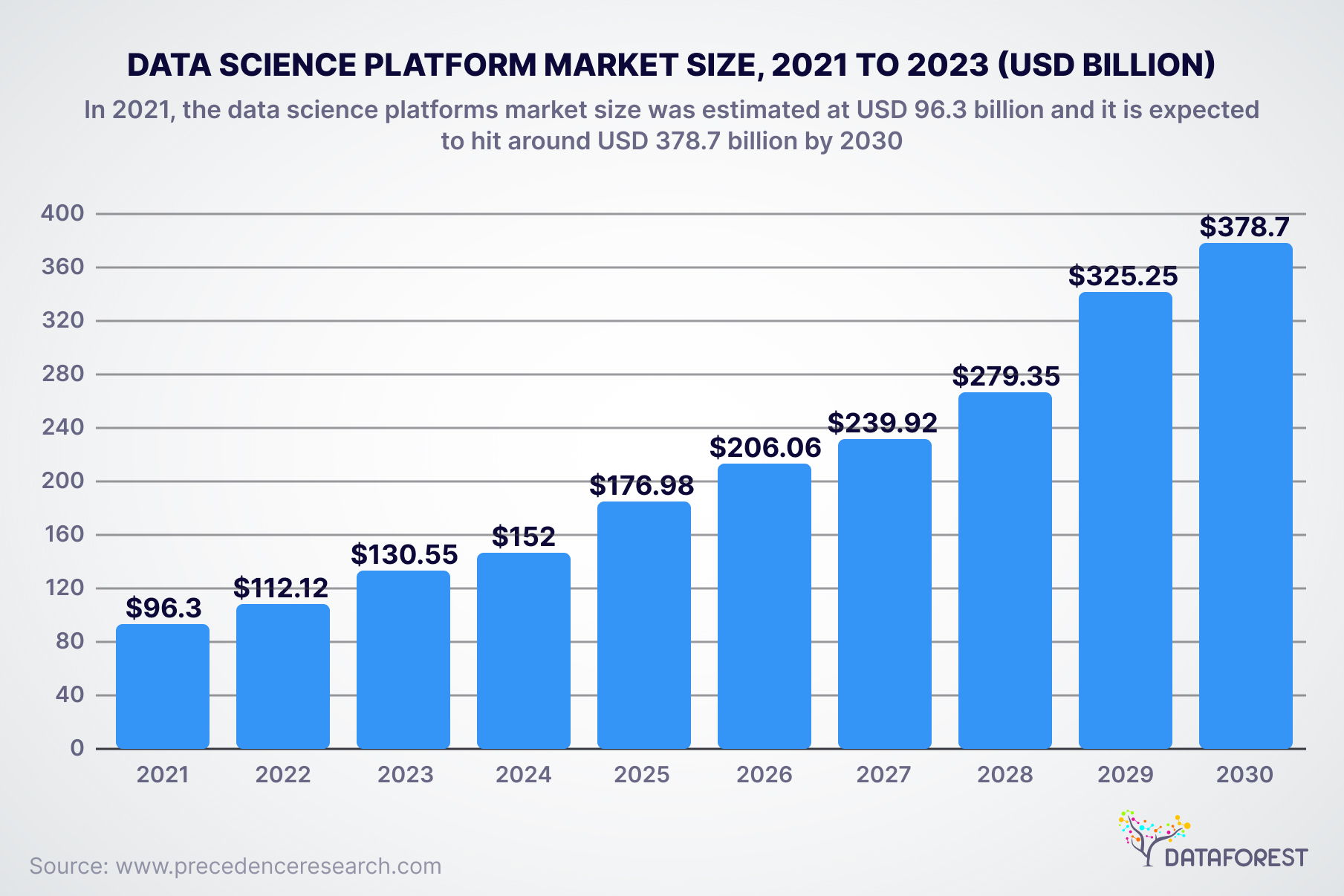
A Force for Equality in Data Science Trends
Data scientists strive to remove the fingerprints of bias from the glass, making it as neutral as possible. They face a paradoxical challenge: the more they clean the glass to reduce bias, the blurrier the view becomes. The lens loses some of its sharpness and ability to discern crucial details. The ultimate data science trends’ goal is to balance cleaning the glass and maintaining clarity. Schedule a call to complement reality with a profitable solution.
Data science is a critical enabler of progress
The significance of data science trends in driving innovation and decision-making cannot be overstated because of its importance in key aspects.
- Data-driven decision-making
- Identifying opportunities and trends
- Product and service innovation
- Personalization and customer experience
- Optimization and efficiency
- Risk management and fraud detection
- Healthcare and medicine
- Environmental sustainability
- Smart cities and urban planning
- Scientific discovery
Data science significance will grow as data becomes increasingly central to decision-making processes.
Dynamic and ever-evolving nature
New technologies, methodologies, and tools emerge rapidly, continually reshaping the landscape. Data scientists must stay abreast of the latest data science trends, adapt to changing data sources and formats, and continuously refine their skills to tackle diverse and complex challenges across industries. Flexibility, a willingness to learn, and an openness to innovation are crucial for thriving in this fast-paced and dynamic domain.
Upholding the principles of fairness
Data science trends continually evolve to address the bias vs. fairness mystery, seeking ways to create more equitable models. Some of the key trends and techniques include:
- Fairness-aware machine learning
- Algorithmic auditing and explainability
- Bias detection and mitigation
- Diverse and representative data collection
- Adversarial debiasing
- Human-in-the-loop approaches
- Ethics and data governance frameworks
Researchers are dedicated to refining these approaches and developing new techniques to tackle the bias vs. fairness anomaly.
Artificial Intelligence (AI) and Machine Learning (ML) Advancements
Artificial Intelligence (AI) advancements are like an ever-evolving decision lens, enhancing clarity through sophisticated algorithms and models. Machine Learning (ML) trends serve as the focus of this decision lens, continuously refining its capabilities with innovations in deep learning, reinforcement learning, and transfer learning, allowing AI to see and understand patterns previously unseen and make informed choices with greater precision.
Leading to smarter systems
As a data science trend, AI has become increasingly integrated into our daily lives, powering virtual assistants, recommendation systems, and personalized user experiences. Meanwhile, ML breakthroughs — such as deep learning and reinforcement learning — have enabled remarkable progress in natural language understanding, computer vision, and autonomous systems, propelling AI towards ever-expanding capabilities and applications.
AI and ML applications in various industries
- Healthcare. AI helps analyze medical images like X-rays and MRI scans for faster and more accurate diagnosis. ML models assist in predicting potential drug candidates and their effectiveness, speeding up the drug discovery process.
- Finance. AI algorithms analyze transaction data to identify fraudulent activities and prevent financial scams. ML-based credit scoring models assess creditworthiness, aiding loan approval processes as data science trends.
- Retail. AI-driven engines suggest personalized product recommendations to customers based on their preferences and behavior. ML models analyze sales data to predict future demand, optimizing inventory management and supply chain operations.
- Transportation. AI and ML technologies power self-driving cars to navigate safely and efficiently without human intervention. AI optimizes transportation routes for delivery services, reducing travel time and costs in the data science trends concept.
- Marketing and advertising. AI analyzes user data to deliver targeted advertisements that align with individual interests and preferences. ML models tailor content recommendations on social media and streaming platforms, enhancing user engagement.
AI and ML in automation, personalization, and predictive analytics
The impact of AI and ML advancements on automation, personalization, and predictive analytics in the context of bias and fairness conflict is promising and challenging.
Responsible AI practices will be crucial in balancing automation, personalization, and predictive analytics.
Upholding the Fairness by Data Science Trends
Ethical AI and Responsible Data Science are like precision-crafted decision lenses that provide clear insights and uphold the principles of fairness, transparency, and accountability. They ensure that the choices made through AI and data-driven processes consider the broader societal impact, fostering trust and benefiting all stakeholders.
Ethical considerations in data science trends
They are crucial in AI and data science because these technologies influence and impact people's lives in various ways. Ethical guidelines ensure that AI systems and data analyses are fair, unbiased, and respectful of individual rights and privacy. Companies avoid harmful consequences, promote transparency, and use AI and data science to benefit society by prioritizing ethics.
Implementation of ethical AI principles
Mitigation techniques for data science trends
Emphasis on responsible data practices requires ethical collection, storage, and data usage, ensuring transparency. Data privacy focuses on protecting individuals' personal information and implementing measures to safeguard sensitive data from unauthorized access. Bias mitigation techniques aim to identify and address biases in data and models to ensure fairness and equitable decision-making in AI and data science applications. Companies build trustworthy and ethically sound data-driven solutions by prioritizing these principles.
Big Data and Cloud Computing in Data Science Trends
Big Data analytics is like a vast and intricate decision lens that captures and processes enormous volumes of diverse data, revealing valuable insights and patterns that were previously hidden. Cloud computing acts as the flexible frame that supports this lens, providing scalable resources and enabling seamless data management and analysis on a global scale.
Three Vs of Big Data increasing with data science trends
- The volume of data refers to the sheer amount of information generated and collected. Data creation has explosive growth with the widespread adoption of digital technologies, the internet, social media, and connected devices.
- The variety of data pertains to the diverse types and formats generated. Data was primarily structured, residing in relational databases. With the rise of unstructured and semi-structured data, there is an increasingly vast array of formats.
- The velocity of data refers to the speed at which data is generated, collected, and processed. Real-time data streams from social media updates, sensor readings, and financial transactions require quick data processing and analysis.
Big data and cloud computing enable scalable analytics
Real-world examples showcasing the data science trends in the utilization of big data and cloud computing for scalable analytics include:
- Netflix uses big data and cloud computing to analyze user behavior, preferences, and viewing habits. This data provides personalized content recommendations to millions of users worldwide, enhancing user engagement and retention.
- Uber relies on big data and cloud computing to process vast amounts of real-time data from drivers and riders, optimizing ride-hailing services and dynamically adjusting prices based on demand and traffic conditions as data science trends.
- NASA uses big data and cloud computing to process and analyze data from space missions and satellite observations. Cloud-based platforms enable scalable analytics for understanding complex astronomical phenomena and climate patterns.
- Amazon Web Services (AWS) offers scalable analytics solutions like Amazon Redshift and Amazon EMR, allowing businesses to process and analyze massive datasets without the need for substantial on-premises infrastructure by using data science trends
Scalability and cost-effectiveness
The main benefits of cloud-based data storage, processing, and collaboration in data science trends are scalability and cost-effectiveness, as cloud platforms offer the flexibility to scale resources as needed and operate on a pay-as-you-go model, optimizing costs. Cloud-based collaboration tools enable seamless teamwork, real-time access to shared data, and efficient project collaboration among data science teams regardless of their locations.
Data Science Trends Connected to Devices and Sensors
Internet of Things (IoT) and Sensor Data Analytics are like a sophisticated lens capturing the hidden insights and patterns from a vast, connected network of devices and sensors. IoT connects various devices, generating massive volumes of data, while Sensor Data Analytics serves as the lens, skillfully processing and analyzing this data to reveal valuable information for informed decision-making.
The growing network of interconnected devices
Internet of Things (IoT) is the concept of data science trends for connecting various physical objects to the internet, enabling them to collect and exchange data with each other and central systems. This interconnectedness allows for real-time monitoring, data analysis, and automated actions, transforming industries, cities, and everyday life with its potential for improved efficiency, convenience, and insights.
Impact of IoT in transforming various domains
Examples illustrating the use of IoT and sensor data analytics in various domains include:
- IoT sensors in roadways and traffic lights collect data on traffic flow, allowing for real-time adjustments to optimize traffic patterns and reduce congestion.
- As data science trends, IoT-enabled smart waste bins monitor fill levels, optimizing waste collection routes to reduce costs and environmental impact.
- IoT sensors track the location and condition of goods throughout the supply chain, enabling real-time monitoring and ensuring timely deliveries while minimizing losses.
- IoT devices in shipping containers and data warehouses monitor temperature-sensitive goods, ensuring optimal storage conditions and reducing spoilage in the data science trends concept.
- IoT sensors collect data on soil moisture, temperature, and crop health, enabling farmers to make data-driven irrigation, fertilization, and pest control decisions.
IoT's potential for real-time insights and predictive maintenance
In the IoT context, there is immense potential for real-time insights, predictive maintenance, and improved decision-making.
Real-time insights
Real-time data monitoring — temperature, humidity, traffic flow, or equipment status — enables immediate responses to changing conditions, allowing businesses to take proactive measures and respond swiftly to critical situations.
Predictive maintenance
Through continuous data analysis and machine learning algorithms, patterns and anomalies can be detected, predicting potential failures before they occur. This predictive capability allows the schedule of maintenance activities to minimize downtime and reduce maintenance costs proactively.
Improved decision-making
Data can be transformed into valuable insights that drive informed decision-making by applying advanced data analytics techniques. Whether it's optimizing supply chain operations, enhancing energy efficiency, or managing traffic flow in a city, IoT-driven data analytics empowers decision-makers with a comprehensive understanding of complex systems.
Deep Learning and Neural Networks in Data Science Trends
Deep learning is like a powerful and intricate lens that extracts complex patterns from vast and diverse datasets, similar to how the human brain processes information. Neural Networks, on the other hand, serve as the focal point of this lens, with multiple interconnected layers of artificial neurons working together to learn from data and make predictions, mimicking the interconnectedness and adaptability of the human brain's neural connections.
Continued advancements in deep learning techniques
- Within data science trends, deep learning researchers and practitioners continually explore and develop new neural network architectures (transformer models, attention mechanisms, and graph neural networks) to improve performance and efficiently address a variety of tasks.
- Transfer learning and pre-training on large-scale datasets have become a prevalent practice. Deep learning models achieve better performance with less data and time by using knowledge from pre-trained models and fine-tuning for specific tasks.
- Advancements in hardware — GPUs and specialized AI accelerators — have significantly accelerated deep learning training and inference, enabling the deployment of larger and more complex models that handle massive datasets and real-time applications as data science trends.
Real-world examples of deep learning applications
Data science trends in computer vision
- Deep learning models like Convolutional Neural Networks (CNNs) are used to identify objects, animals, or landmarks in photos for image classification tasks.
- Deep learning-based object detection algorithms enable systems to identify and locate multiple objects within images or videos commonly used in autonomous vehicles.
- Deep learning techniques power facial recognition systems, enabling applications like unlocking smartphones, verifying identity, and enhancing data security.
Natural Language Processing (NLP) as a part of data science trends
- Deep learning models like Transformer-based architectures enable accurate and efficient machine translation between different languages.
- Deep learning is employed for sentiment analysis tasks, determining a text's sentiment (positive, negative, neutral), and is helpful in social media monitoring.
- Deep learning models can generate human-like text in chatbots, virtual assistants, and automated content creation.
Data science trends — recommendation systems
- Deep learning-based recommendation systems analyze user preferences to offer personalized content — movie recommendations on streaming platforms or product suggestions on e-commerce sites.
- Music streaming services use deep learning to recommend songs and curate personalized playlists based on users' listening history.
Tackling challenging problems
In data science trends, the impact of deep learning in complex pattern recognition, feature extraction, and high-dimensional data analysis is profound:
- Deep learning excels at recognizing complex patterns and relationships within data, especially in tasks like image and speech recognition. Deep learning models can automatically learn and represent intricate patterns by employing deep neural networks with multiple layers.
- Deep learning's ability to automatically learn hierarchical representations of data enables effective feature extraction. As the network learns from raw data, it identifies relevant features, reducing the need for manual feature engineering, which can be time-consuming and prone to human bias.
- Deep learning techniques handle high-dimensional data efficiently, making them well-suited for problems with large and complex datasets. In genomics, neuroscience, and financial modeling, deep learning's ability to handle this complexity allows for better understanding and insights from the data.
Due to data science trends, the advancement of deep learning techniques continues to revolutionize fields like computer vision, natural language processing, and data analytics.
Data Science Trends — Optimizing Machine Learning Models
Automated Machine Learning (AutoML) is like an intelligent lens that automates designing and building machine learning models. Like a lens that automatically adjusts focus to capture clear images, AutoML handles complex tasks like feature engineering, hyperparameter tuning, and model selection, simplifying and accelerating the machine learning workflow for data scientists and developers.
Automation and artificial intelligence techniques
AutoML, or Automated Machine Learning, as a part of data science trends, uses artificial intelligence techniques to automate various steps in the machine learning process. Its role in streamlining the machine learning process is to simplify tasks that would typically require substantial manual effort. AutoML automates data preprocessing, feature engineering, model selection, hyperparameter tuning, and model evaluation, enabling users to quickly create high-performing machine-learning models without extensive domain knowledge or manual experimentation.
Benefits of AutoML in democratizing data science
- AutoML platforms like Google AutoML and H2O.ai's AutoML have been used in Kaggle competitions. These platforms enable participants with varying machine learning expertise to build competitive models in the direction of data science trends.
- Business data analysts and domain experts use AutoML tools to analyze data and extract valuable insights without the need for extensive programming skills or data science expertise.
- Non-experts in finance use AutoML platforms to build predictive models for financial forecasting tasks, such as stock price or demand forecasting due to data science trends.
- AutoML has played a significant role in democratizing NLP applications. Non-experts build sentiment analysis models, text classifiers, and chatbots using AutoML tools like IBM Watson AutoAI or Microsoft Azure AutoML.
- In data science trends, AutoML is being adopted in e-commerce and retail industries to optimize product recommendations, demand forecasting, and customer segmentation. Business analysts and marketers leverage AutoML tools to make data-driven decisions without relying solely on data scientists.
AutoML reduces time and effort
Model development, feature engineering, and hyperparameter optimization are accelerated by implementing Automated Machine Learning techniques in data science trends. AutoML uses advanced algorithms to streamline and optimize the process of building machine learning models, identifying relevant features from raw data, and finding the best hyperparameters for optimal performance. This automation significantly reduces the manual effort and time required, making it more efficient for data scientists and non-experts to develop high-performing models quickly.
Better Understanding Data Science Trends
Data visualization and storytelling in data science are powerful lenses that transform complex datasets into compelling narratives. Data visualization presents data in graphical forms to make patterns and insights visible, while storytelling is the focal point, weaving a coherent narrative around the data to communicate meaningful and impactful insights to an audience.
Communicating insights
- Data visualization transforms complex datasets into visual representations, making it easier for data scientists and non-experts to grasp trends and relationships.
- Well-designed data visualizations enhance clarity by intuitively presenting data. Data visualization aids in highlighting key points and insights with data science trends.
- Data visualization supports data storytelling, enabling data scientists and analysts to present insights as compelling narratives.
- Effective data visualization reveals outliers, anomalies, and hidden patterns that might otherwise go unnoticed in raw data as a part of data science trends.
- Data visualization is a common language that transcends disciplines and makes effective reporting between scientists, business stakeholders, and decision-makers.
- In data science trends, compelling data visualizations capture the attention of stakeholders and maintain their interest throughout the presentation.
- Data visualization facilitates data-driven decision-making by presenting evidence-based insights that support informed choices.
Data visualization and interactive dashboards
- Due to data science trends, the Johns Hopkins University COVID-19 dashboard became recognized during the pandemic for providing real-time data on confirmed cases, deaths, and recoveries worldwide.
- Tableau Public hosts a collection of interactive data visualizations and dashboards created by data enthusiasts and professionals.
- Gapminder presents a visualization of global development data. The Trendalyzer tool animates data over time, revealing fascinating insights about global data science trends.
- Google Analytics offers interactive dashboards for website analytics, allowing users to explore metrics related to website traffic, user behavior, and conversion rates.
- The World Bank provides interactive data visualizations covering various global economic and social indicators in the data science trends concept.
Presenting data within a narrative framework
The role of storytelling in data-driven narratives is to contextualize data insights, engage audiences, and clarify complex concepts. Storytellers make data relatable, evoking emotions that drive audience engagement and enabling stakeholders by weaving data into compelling narratives to make informed decisions based on data-driven insights.
Data Science Trends — Computers Interact with Human Language
Natural Language Processing (NLP) applications are a lens that allows computers to understand human language. Through NLP, computers process, analyze, and generate text, making it possible to develop applications that comprehend, respond to, and generate natural language, revolutionizing virtual assistants, chatbots, language translation, sentiment analysis, and text summarization in data science trends.
Improvements in language generation
- Introducing transformer-based models — BERT (Bidirectional Encoder Representations from Transformers) and GPT (Generative Pre-trained Transformer) — has improved NLP tasks by capturing contextual information and bidirectional context.
- Advancements in NLP have extended beyond textual data to include other modalities: images, audio, and video, and it's also in data science trends. Integrating multimodal learning techniques allows NLP models to understand and generate content using information from different sources.
- NLP techniques like zero-shot and few-shot learning have enabled models to perform tasks with minimal training data. NLP models adapt to new tasks with limited supervision, reducing the need for extensive labeled data.
- As NLP models become more powerful, the discussion of ethical considerations, fairness, and bias in language processing has become more prominent. Researchers are exploring ways to mitigate biases and ensure that NLP models are fair due to data science trends.
- Developing large-scale pre-trained language models, like GPT-3 and T5 (Text-to-Text Transfer Transformer), has opened new possibilities for language processing. These models perform various tasks by fine-tuning specific data.
- Advancements in NLP have driven significant progress in conversational AI, leading to more natural and context-aware chatbots and virtual assistants due to data science trends. They engage in meaningful conversations with users, making human-computer interactions more seamless.
- Research efforts in NLP have also focused on addressing challenges related to low-resource languages, aiming to build models that perform well with limited training data. These advancements aim to make NLP technologies more inclusive.
Communication between humans and machines in data science trends
Companies can harness the power of language processing to improve customer experiences, optimize operations, and expand their global reach through efficient language translation.
Sentiment analysis
- Companies use NLP-powered sentiment analysis to monitor social media platforms for customer feedback and brand perception. Businesses can gain valuable insights into customer sentiment and respond proactively to issues or trends.
- As a part of data science trends, e-commerce platforms use NLP to automatically analyze product reviews and classify sentiments as positive, negative, or neutral. It helps customers make informed purchasing decisions and enables businesses to identify areas for improvement.
Chatbots and virtual assistants
- Google's virtual assistant uses NLP to understand queries and provide relevant information and responses. The assistant engages in human-like conversations and helps with various tasks: setting reminders, answering questions, and making reservations about data science trends.
- Many companies deploy NLP-powered chatbots for customer support. These chatbots can understand and respond to customer inquiries in real time, providing efficient and personalized assistance and often resolving issues without human intervention.
Language Translation
- Google Translate automatically uses NLP techniques to translate text from one language to another. The system employs machine learning algorithms that learn from vast multilingual datasets to provide accurate, appropriate translations.
- NLP advancements are also in data science trends and have led to the development of neural machine translation models, significantly improving the quality of translated content. These models use deep learning to learn language patterns and provide more natural-sounding translations.
Personalized interactions with chatbots
Enhanced customer experiences through NLP involve personalized interactions with virtual assistants, delivering quick responses and tailored solutions to queries and concerns. NLP improves information retrieval by enabling advanced search engines and knowledge bases that process natural language queries, showing more contextually accurate and relevant search results due to trends in data science. Sentiment understanding via NLP empowers businesses to gauge customer feedback and opinions, enabling targeted improvements and proactive responses to enhance customer satisfaction and brand perception.
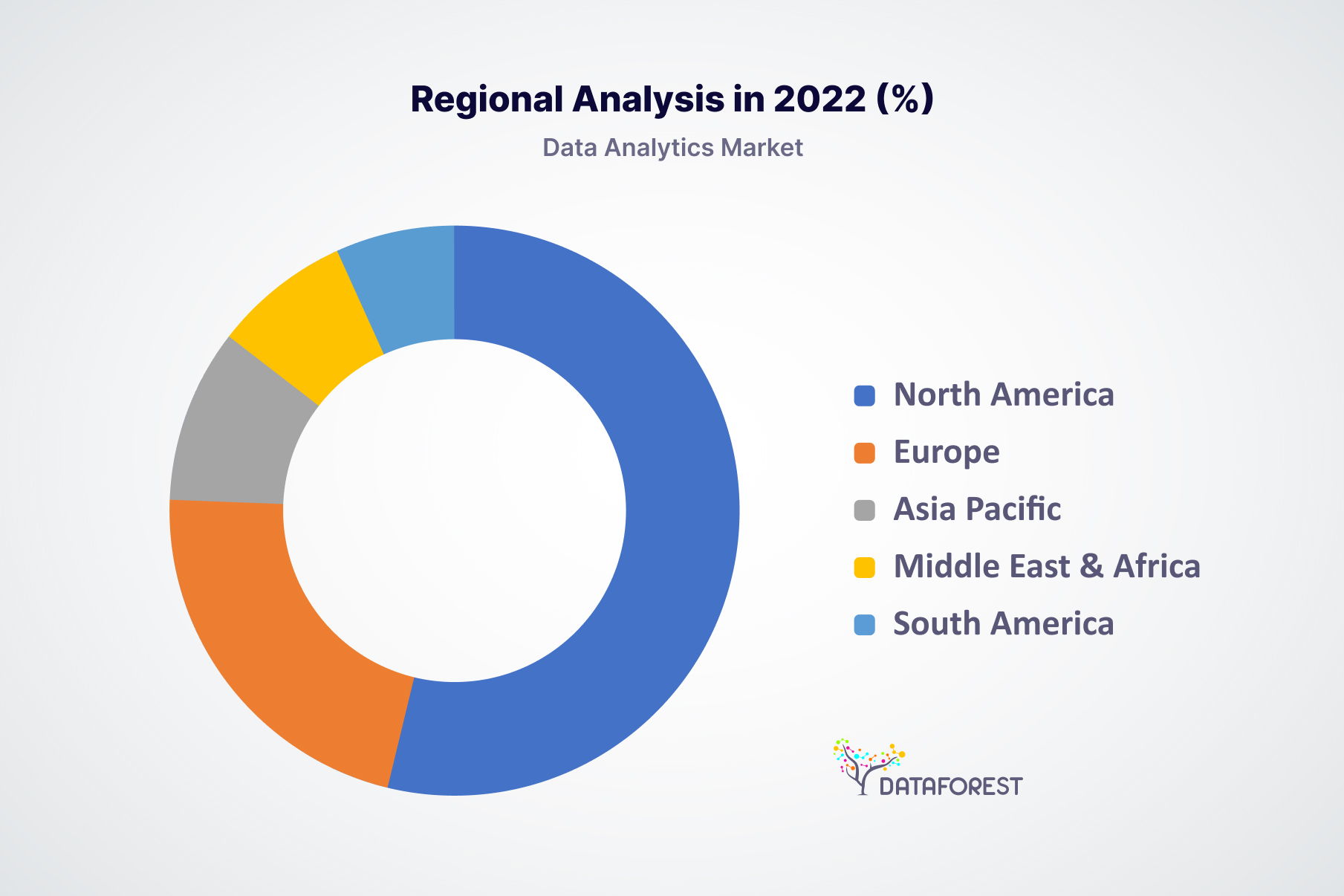

Data Science Trends and Proactive Measures
DATAFOREST has extensive experience in providing data engineering services. Therefore, it follows global industry trends and has a professional vision for its development. Fairness can beat bias in data science trends through proactive measures, including identifying and addressing data collection and preprocessing biases.
Data scientists implement fairness-aware algorithms that explicitly consider fairness during model training, using techniques like reweighted loss functions or adversarial debiasing. Data science promotes fairness and ethical considerations, creating AI systems that make unbiased decisions by prioritizing interpretability, diverse teams, and continuous monitoring.
You want to be in trend but need to know where to start. In this case, please fill out the form, and we will discuss it together in a consultation.
FAQ
How does ethical AI contribute to responsible data science practices?
Ethical AI contributes to responsible data science practices by ensuring that AI systems are developed, deployed, and used in ways that prioritize fairness, transparency, accountability, and societal well-being in order of current trends in data science.
What role do big data and cloud computing play in advancing data science capabilities?
Big data and cloud computing play a pivotal role in advancing data science capabilities by providing scalable and cost-effective infrastructure for storing, processing, and analyzing vast amounts of data, enabling data scientists to uncover valuable insights and develop sophisticated models at unprecedented scales within emerging trends in data science.
How are the Internet of Things (IoT) and sensor data analytics transforming industries through data science?
Internet of Things (IoT) and sensor data analytics are transforming industries through data science by enabling real-time data collection and analysis, fostering predictive maintenance, optimizing operations, and driving decision-making for enhanced efficiency and innovation.
How does data visualization enhance data storytelling and decision-making in data science?
Data visualization enhances data storytelling and decision-making in data science by presenting complex data visually compellingly, making patterns and insights more accessible and actionable, enabling data-driven narratives that resonate with stakeholders and inform effective decision-making processes.
How can companies leverage these data science trends to gain a competitive edge and drive innovation?
Companies can leverage data science trends to gain a competitive edge and drive innovation by harnessing advanced analytics, AI technologies, and responsible data practices to make informed, data-driven decisions, optimize processes, and develop innovative products and services that meet evolving customer needs.
What are the implications of these trends for data privacy and security?
The implications of these trends for data privacy and security include the need for robust data protection measures, ethical data handling practices, and stringent security protocols to safeguard sensitive information and mitigate potential risks of unauthorized access or misuse.
How are low-code platforms and business intelligence connected?
Low-code platforms (software development tools that allow users to build applications with minimal hand-coding) are revolutionizing business intelligence by enabling rapid application development and empowering non-technical users to create sophisticated data-driven solutions with minimal coding efforts.