A radiologist is trying to diagnose early-stage neurological disorders. Traditional MRI scans are noisy, hiding tiny but critical details. Diffusion image generation models take these noisy images and clean them up, revealing details that might otherwise be missed. This means doctors catch diseases earlier and start treatment sooner. Computed tomography scans often get messed up by patient movement or metal implants. Diffusion image generation models fix these issues by learning to recognize and correct distortions, giving doctors a more precise picture to work with. Training AI models to diagnose rare conditions is challenging because there are insufficient data. Diffusion models generate high-quality synthetic images, boosting the amount of training data without needing tons of rare cases. Are you interested in the update? Book a call, and we'll tell you how to use diffusion image generation in a business.
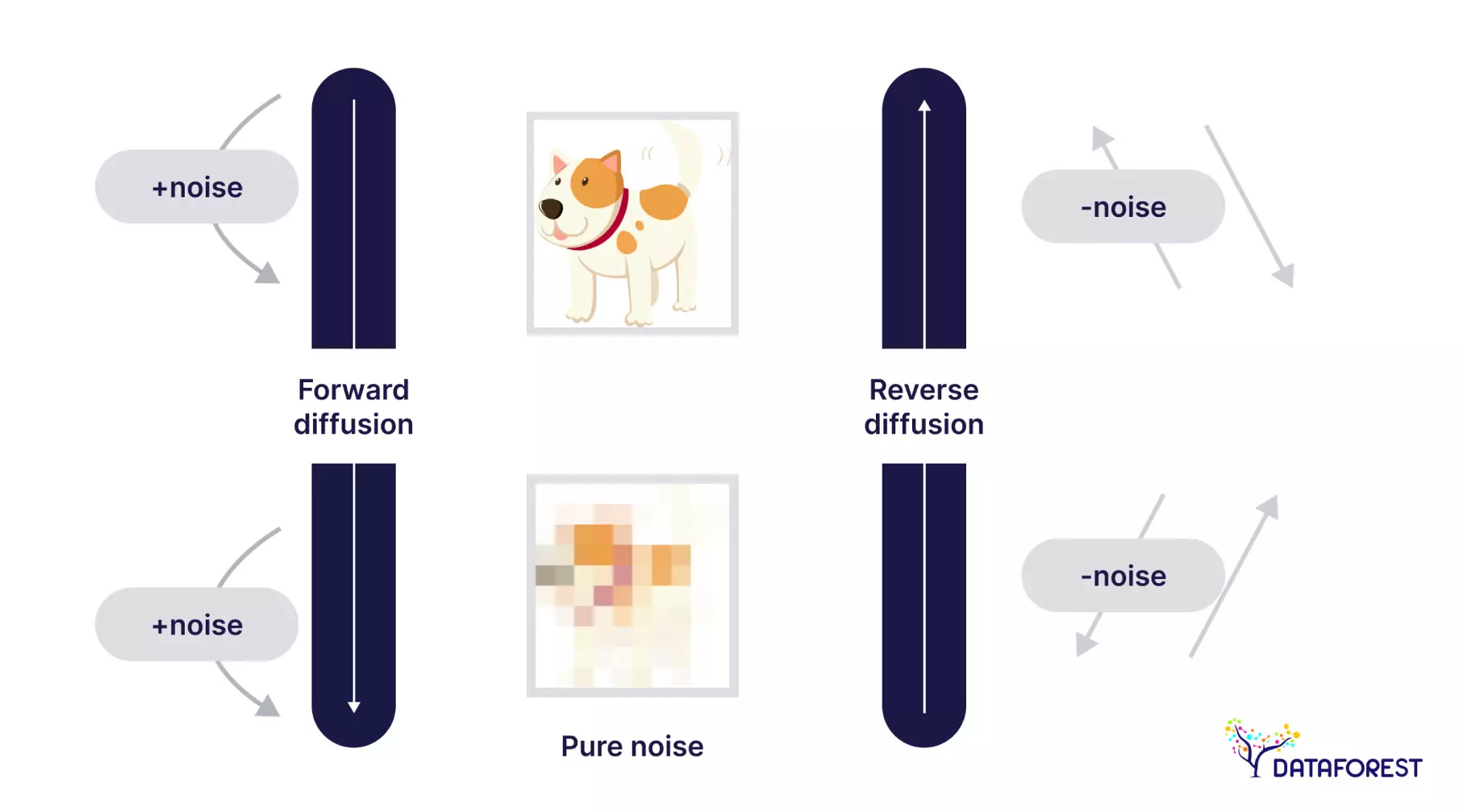
Diffusion Models for Image Generation: What They Are and How They Work
Diffusion image generation models are a type of machine learning algorithm used for generating high-quality images. They work by starting with a noisy image and iteratively refining it, removing noise step by step until a detailed image emerges. It’s a reverse process of adding noise to a picture until it becomes a clear photo.
Forward Diffusion:
- Start with a clean image.
- Gradually add noise over several steps, making the image more and more chaotic.
- This process turns a clear photo into a grainy one.
Reverse Diffusion:
- Begin with a noisy image.
- Use a neural network trained to remove noise step by step.
- Each step makes the image less noisy and more detailed.
- Eventually, the image is refined back into a clear, high-quality picture.
The model is trained on a large dataset of images. Each image is paired with progressively noisier versions. The neural network learns how to predict the original image from its noisy versions. This involves minimizing the difference between the predicted clean image and the actual clean image.
Let’s say you have a photo that’s become pixelated. A diffusion image generation model will start with this noisy image and apply denoising steps. Each step gradually transforms the image back into its original. The model learned these steps by seeing many noisy and clean image pair examples.
Three Business Applications for Diffusion Image Generation
Business applications for diffusion image generation are the various ways companies utilize diffusion image generation models to create, enhance, and optimize visual content. These applications span several domains, providing realistic images that drive business value.
- Marketing and Advertising
In marketing and advertising, the quality of visual content makes or breaks a campaign. Diffusion image generation models produce stunning images that capture attention and convey messages more effectively.
Unique Visuals: Diffusion image generation models produce unique images tailored to specific marketing themes. For instance, a fashion brand can generate high-resolution images of new clothing lines, enhancing the appeal to potential customers.
Personalized Advertisements: These image-generation models create personalized ad visuals based on customer preferences. Imagine an online store generating custom ads showing products a user is most likely interested in.
Cost-Efficient Content: By automating the generation of visual content, companies save on costs associated with traditional photoshoots and graphic design. This is particularly useful for social media campaigns that require a constant stream of fresh visuals.
- Product Design and Development
Diffusion image generation models are invaluable in product design and development. They allow designers to create realistic prototypes and visualizations quickly and efficiently.
Prototyping: Designers generate detailed images of product prototypes, allowing for visual assessment and feedback before moving to physical production.
Virtual Showrooms: Companies create virtual showrooms with high-quality images. Customers interact with these images, viewing products from different angles and configurations.
Design Customization: Diffusion image generation models enable the creation of custom product designs on the fly. An automotive company could generate images of cars in different colors and with various customizations.
- Customer Engagement
Engaging customers with personalized and dynamic content is key to building loyalty and driving sales. Diffusion models create content that resonates with individual users.
Dynamic Personalization: Diffusion image generation models generate personalized content that adapts to the user's preferences and behavior in real time. For instance, an e-commerce site can display product images that match the user's style and past purchases.
Interactive Experiences: Diffusion image generation models create interactive and immersive experiences. For example, a fashion retailer could use these models to generate virtual try-on images, allowing users to see how clothes would look on them without physically trying them on.
Content Freshness: Keeping content fresh and relevant is crucial for customer engagement. Diffusion image generation models continually generate new images, keeping websites, apps, and social media feeds dynamic.
Case Studies of Image Generation with Diffusion Models
Diffusion image generation highlights real-world examples of businesses leveraging this technology to significantly improve their visual content creation, marketing, product design, and customer engagement.
Personalized Marketing Campaigns
Nike used diffusion image generation models to generate personalized ad visuals for their online and social media marketing campaigns. The models created dynamic images of sportswear and footwear tailored to individual preferences, showcasing products in various colors, styles, and settings that matched user profiles. Nike saw a 23% increase in user engagement with their ads, as customers were likelier to interact with content that resonated with their tastes. Personalized ads led to a 17% increase in conversion rates, as users were more inclined to purchase items that appeared tailored to preferences.
Virtual Showroom and Product Visualization
IKEA implemented diffusion image generation models to enhance their virtual showroom experience. These models generated realistic images of furniture and home decor items, allowing customers to visualize products in various settings and configurations. The virtual showroom featured interactive elements, enabling users to customize and view products in different room layouts. Customers reported a 28% improvement in their online shopping experience, citing the ability to see realistic product images as a key factor. IKEA experienced an 18% boost in online sales, attributed to the improved visualization and customization options the diffusion image generation models provided.
Design Customization and Prototype Visualization
Tesla utilized diffusion image generation models to streamline their electric vehicles' design and prototyping process. The models generated images of car prototypes, including customization options such as paint colors, interiors, and add-on features. This allowed the design team to refine designs based on stakeholder feedback and customer preferences. The time required for design iterations decreased by 37%, as the need for physical prototypes was reduced. Tesla's online configurator, powered by diffusion image generation models, saw a 32% increase in user interactions as customers enjoyed exploring their vehicle options.
Tips to Choose the Diffusion Model
The right diffusion image generation model best aligns with your business objectives, technical requirements, and operational constraints.
Scalability
When choosing a diffusion image generation model, consider how well it can scale with your business. If you expect to generate a large volume of images or need to support high traffic on your platform, opt for a model that can handle increased demand without compromising performance. Scalability ensures that your diffusion model will continue to deliver high-quality results as your business grows.
Integration
The suitable diffusion image generation model should seamlessly integrate with a workflow, whether part of a marketing platform, product design software, or customer engagement tool. To streamline the integration, look for models with well-documented APIs and support for popular programming languages.
Business Needs
Are you looking to enhance marketing visuals, create realistic product prototypes, or generate personalized customer content? Different diffusion image generation models excel in different areas, so choose one tailored to your primary use case. Understanding your goals will help narrow down the options to models that offer the best fit.
Cost and Resource Availability
Evaluate the cost of deploying and maintaining the diffusion image generation model. Consider the computational resources required, such as GPU availability and cloud infrastructure costs. Ensure you have the budget and technical resources to support the chosen model over the long term.
Performance and Quality
Assess the performance and output quality of the diffusion image generation models under consideration. Look for models that produce high-fidelity images with minimal artifacts and noise. Reviewing benchmarks and user reviews can provide insights into the performance capabilities of different models.
Popular Models and Tools
- Google's Imagen is one of the industry's leading diffusion image generation models. It is known for its ability to generate photorealistic images from text descriptions. It creates detailed, high-quality visuals ideal for marketing and advertising applications.
- DALL-E 2 by OpenAI is another popular model that generates images from textual descriptions. It offers impressive flexibility, allowing businesses to create various visuals, from product designs to marketing content. Its versatility makes it a strong choice for various SMB needs.
- NVIDIA's GauGAN is designed to create realistic landscape and environment images. It benefits industries like gaming and virtual reality, where detailed and immersive visuals are crucial.
- Stability Diffusion by Stability generates stable, high-quality image artifacts and ensures consistency. It's well-suited for applications requiring reliable and repeatable image generation, such as product visualization and design iteration.
Integrating Diffusion Models for Image Generation
Implementing and integrating diffusion image generation models transforms a business' visual content but comes with challenges. Follow this step-by-step guide and prepare for potential hurdles to ensure a successful integration.
Schedule a call to complement reality with a profitable diffusion image generation model.
Future Trends in Diffusion Image Generation
Future trends in diffusion image generation are the advancements and potential developments in this technology, which are expected to impact various industries significantly.
New advancements in model architectures, such as integrating more complex neural networks and enhanced algorithms, will lead to diffusion image generation models that are faster, more efficient, and capable of generating images. Combining diffusion models with other AI techniques, such as GANs and transformers, will result in hybrid image generation models that leverage the strengths of multiple approaches. This synergy will enhance the robustness and versatility of image generation, making it possible to create diverse types of visual content for various applications, from human faces to intricate landscapes. Future advancements will likely lead to real-time diffusion image generation.
Emerging AI-driven creativity tools will incorporate diffusion image generation models to assist designers and artists in generating content. These tools will offer suggestions, automate repetitive tasks, and enable users to explore new artistic possibilities.
Long-term Benefits:
- Companies that integrate diffusion image generation models into their workflows will benefit from enhanced visual content that more effectively captivates audiences.
- As diffusion image generation models become more advanced and widespread, visual content production costs will decrease.
- Businesses can offer personalized and dynamic visual content that enhances the customer experience.
- Diffusion image generation models will generate valuable data and insights into consumer preferences and behaviors.
- Diffusion image generation models will provide businesses with scalable and flexible solutions for image generation. As the technology evolves, companies will scale content to meet demands.
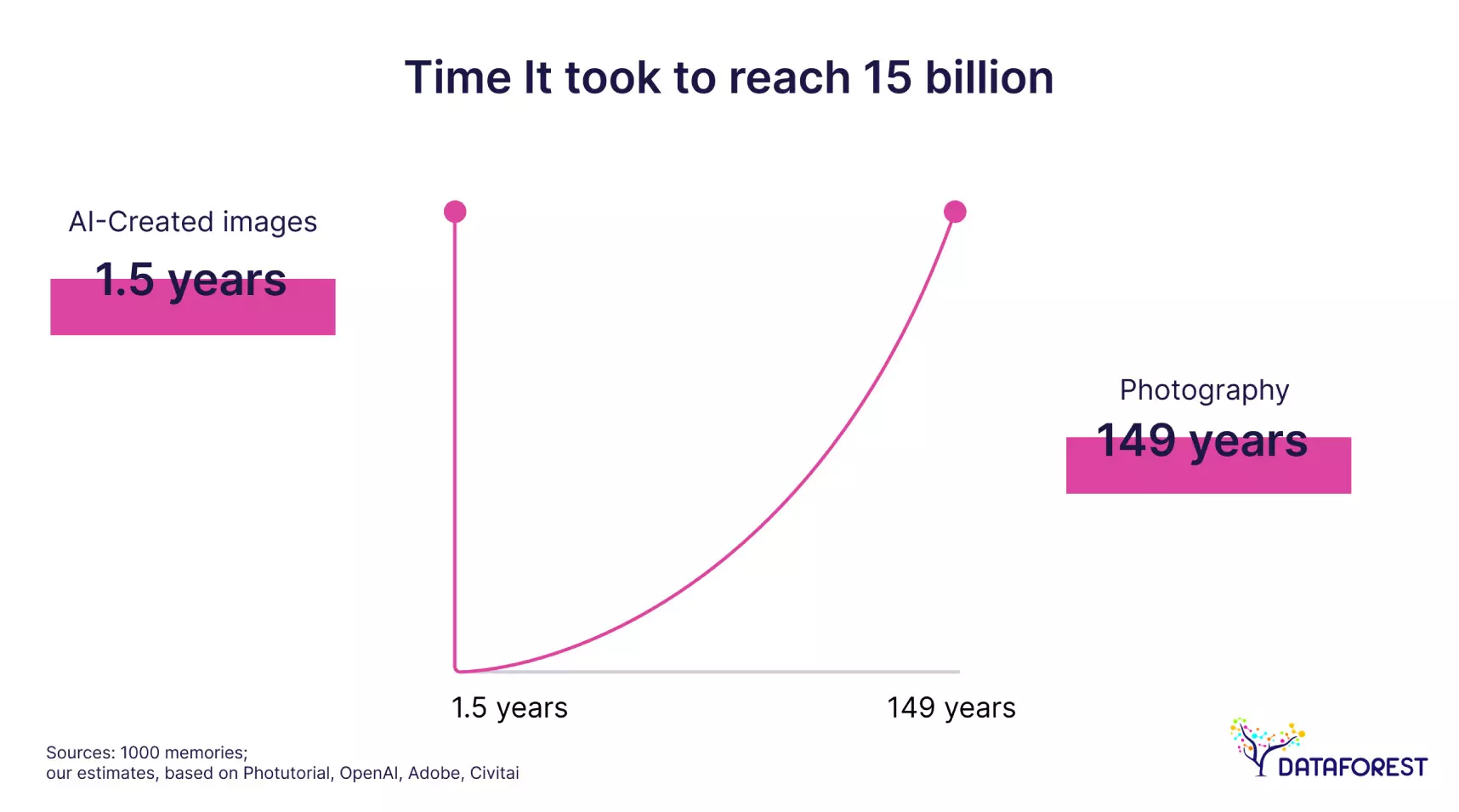
Diffusion Image Generation Models Key Question
AI consultants like DATAFOREST are asked one question more often than others regarding implementing diffusion image generation. How can we integrate diffusion image generation models into our existing business workflows to maximize efficiency and output quality? The process begins with identifying specific use cases and goals. Choosing a suitable model tailored to these needs is crucial. Integration involves setting up the necessary computational resources and utilizing well-documented APIs for smooth implementation. Training the model with relevant data and extensively testing it ensures high-quality outputs. Continuous monitoring and maintenance are essential to sustain performance. Overcoming challenges like resource constraints and technical issues is part of the strategy. Please fill out the form and make your business an art.
FAQ
How do diffusion image generation models generate images?
Diffusion image generation models generate images by starting with a noisy version and iteratively removing the noise through a reverse diffusion process, refining the image step by step. This involves training a neural network to predict and denoise the image at each step, producing a high-quality image.
Which is the best model for image generation?
The best model for image generation depends on the business's specific goals; for example, Google's Imagen excels at creating photorealistic images from text descriptions, making it ideal for marketing and advertising. OpenAI's DALL-E 2 offers flexibility and creativity for a wide range of visual content, while NVIDIA's GauGAN is particularly suited for realistic landscape and environment images, and Stability AI's Stable Diffusion focuses on producing consistent images.
What are the benefits of diffusion image generation models?
Diffusion image generation models produce detailed images by iteratively refining noisy inputs, making them ideal for various applications such as marketing, product design, and personalized content creation. They offer stable training, versatility, and the ability to generate realistic images, enhancing customer engagement and reducing content creation costs.
How many images do you need to train a diffusion image generation model?
Training a diffusion image generation model typically requires a large dataset, often tens of thousands to millions of images, to ensure the model learns to generate diverse outputs. The exact number depends on the complexity of the model and the quality of the images needed for the specific application.
How do we address the unstable diffusion image generation of an AI picture?
To address unstable diffusion image generation, ensure proper tuning of hyperparameters and utilize a well-structured training dataset to enhance model stability and output quality. Regularization techniques and advanced architectures mitigate instability and improve the consistency of generated images.