An e-commerce platform grappled with a rising churn rate, affecting its profitability. Customer churn prediction analysis pinpointed the primary churn drivers as dissatisfaction with customer support and a cumbersome returns process. The e-shop overhauled its service framework, introducing a 24/7 chat support system and streamlining its returns process for enhanced user convenience. The company deployed a personalized email marketing strategy, offering exclusive discounts and recommendations based on past shopping behaviors to re-engage at-risk customers. Within six months, these initiatives led to a decline in churn rates and an increase in repeat customer transactions. The cost savings from reduced customer acquisition efforts, as a result of improved retention, were substantial, allowing more funds to be allocated to product development and market expansion. Industry studies suggest that a focused customer retention strategy reduces churn rates by 5% to 10%. If you need an individual approach to a solution, book a call.
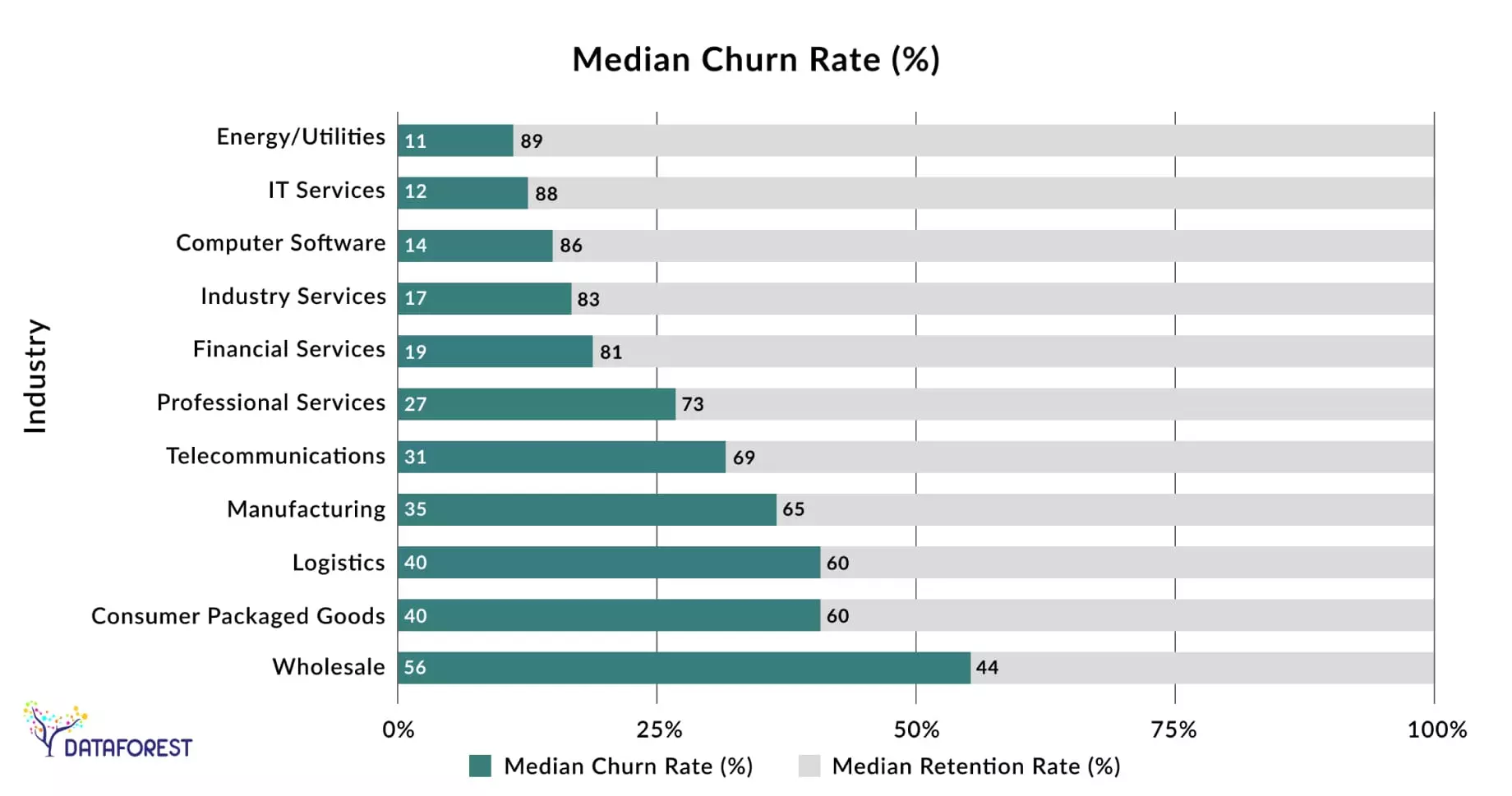
Median Customer Churn Rates by Industry 2022
Churn Prediction Solves Business Pain Points
Churn prediction equips businesses with the foresight to identify customers likely to leave, directly addressing the challenge of customer retention by enabling targeted intervention strategies. It streamlines resource allocation, ensuring efforts and investments are focused on retaining high-value customers at risk of churning rather than being spread thinly across the entire customer base. By foreseeing potential revenue loss due to customer attrition, churn prediction allows for proactive measures to maintain and potentially increase profitability. It enhances customer engagement by providing insights into customer preferences and dissatisfaction points, allowing personalized communication and service offerings. Churn prediction reveals product or service misalignments with customer expectations, guiding adjustments to effectively meet market demands. It supports strategic planning by offering predictive insights into customer base trends, aiding in long-term growth and adaptability decisions.
Churn Prediction Is an Early Warning System
Churn prediction identifies which customers are likely to stop using a company's products or services shortly. By figuring out who will likely churn, a business can try to keep these customers by addressing their concerns or offering them incentives to stay.
Churn prediction typically involves collecting data on customer behavior, such as how often they use the service, any complaints they've made, or changes in their usage patterns. Advanced AI analytics and churn prediction machine learning models then analyze this data to spot patterns or signs that a customer is becoming less engaged and might soon leave.
Imagine a telecom company that offers internet services. It noticed that some customers were canceling their subscriptions, affecting the revenue. To tackle this issue, the company started collecting data on customer behavior, including how often customers contacted support, their satisfaction scores from surveys, payment history, and usage patterns. Using churn prediction models, they identified customers who showed signs of potential churn: decreased data usage, late payments, or negative survey feedback. With this information, the team targeted these at-risk customers with special offers—discounts on their next bill, upgrades to faster internet speeds for the same price, or personalized assistance to resolve any service issues they were experiencing. This proactive approach allowed us to retain customers who might have left for a competitor.
Impact of Churn Prediction on Large Companies
Churn prediction offers many benefits to large companies, from reducing customer attrition to increasing customer lifetime value (CLV) and improving overall customer satisfaction. These benefits impact the company's financial performance and market position.
Reducing Customer Attrition
By accurately identifying customers at risk of churning, companies can implement targeted intervention strategies to address their concerns, improve their experience, and ultimately retain them. For instance, a study by Bain & Company highlighted that increasing customer retention rates by just 5% can increase profits by 25% to 95%. In the context of a large company, utilizing churn prediction models to identify at-risk customers and offering personalized plans or services could prevent thousands of customers from leaving.
Increasing Customer Lifetime Value
Churn prediction enables companies to proactively engage with at-risk customers through personalized offers, loyalty programs, or improved service experiences, enhancing the customer's journey and increasing their CLV. According to a report by Frederick Reichheld of Bain & Company (the inventor of the Net Promoter Score), a 5% increase in customer retention correlates with at least a 25% increase in profit. A large e-commerce platform could use churn prediction insights to offer tailored discounts or exclusive early access to new products for customers showing reduced engagement, boosting repeat purchases and elevating CLV.
Improving Customer Satisfaction
Understanding the early signs of churn allows companies to address issues proactively. This aids in retaining customers and enhances brand reputation, which can attract new customers. A case study from a leading SaaS provider indicated that after implementing churn prediction strategies and focusing on high-risk accounts with customized engagement plans, customer satisfaction scores improved by over 10%, significantly reducing churn rates and increasing referral rates.
Case Study: Global Retail Bank
It implemented churn risk prediction analytics to identify customers likely to close their accounts. By analyzing data points such as transaction frequency, account age, service usage patterns, and customer feedback, the bank targeted at-risk customers with personalized banking solutions, including tailored financial advice, flexible loan repayment options, and exclusive access to premium banking services. Within a year of implementing these strategies, the bank saw a 20% reduction in customer attrition rates and a 15% increase in CLV among the targeted customer segment. Furthermore, the bank reported a 30% improvement in customer satisfaction scores, attributing these gains to more effective strategies.
Implementing Churn Prediction Solutions
Implementing a churn prediction solution in a large business is a multifaceted process that involves several critical steps, from initial data collection to ongoing monitoring and refinement.
- Data Collection: The foundation of any churn prediction model is comprehensive and high-quality data. Businesses need to collect a wide range of data points. It includes structured data (usage frequency, purchase history) and unstructured data (customer reviews, support ticket texts).
- Data Preparation and Cleaning: Once data is collected, it must be cleaned and prepared for analysis. This step involves handling missing values, correcting errors, and standardizing formats. It's crucial for ensuring the accuracy and reliability of the customer churn prediction model.
- Feature Selection and Model Building: Techniques like logistic regression, decision trees, random forests, and neural networks can be used to build predictive models. A dedicated technology partner with expertise in AI can select the most appropriate algorithms, train the models on historical data, and validate their accuracy.
- Analysis and Insight Generation: The AI model analyzes the data to identify patterns and predictors of churn. The output is a set of insights regarding which customers are at risk of leaving and why. This phase requires data analysis tools and software managed by data scientists and AI churn prediction specialists.
- Action Planning and Intervention Strategies: Based on the insights generated, businesses need to develop targeted intervention strategies to retain at-risk customers. This involves personalized offers, improved customer support, product or service enhancements, or loyalty programs. A cross-functional team, including marketing, sales, and customer service, should collaborate to design and implement these strategies.
- Implementation and Monitoring: The intervention strategies are rolled out to the identified at-risk customers. It's essential to closely monitor the impact of these actions on customer behavior and churn rates, adjusting the strategies as necessary. This requires ongoing data collection and analysis, supported by an AI framework that adapts to new patterns and insights.
- Continuous Improvement: As the market, customer preferences, and business offerings evolve, the customer churn prediction and prevention model and its underlying strategies must be refined and updated. Continuous learning, facilitated by AI and machine learning, is vital to staying ahead of churn.
An external partner specializing in AI and data analytics (such as DATAFOREST) is crucial for successfully implementing a churn prediction solution. They bring the necessary expertise in data science, AI model development, and analytics to build, maintain, and refine the churn prediction model. They ensure the solution remains aligned with the latest technological advancements and objectives.
Churn prediction models and strategies require regular evaluation and adjustment to remain effective. Customer behavior, market conditions, and the competitive landscape constantly change, which can influence churn patterns. Ongoing monitoring, supported by a dedicated team, ensures the business can quickly adapt its strategies in response to new insights.
Churn Prediction Success Stories
These examples underscore the impact of churn prediction on a company's bottom line and overall customer satisfaction. By leveraging AI data analytics and machine learning, businesses can predict churn, understand its root causes, and take actionable steps to mitigate it.
- Based on engagement levels and other behavioral indicators, Netflix's sophisticated algorithms predict which customers might cancel their subscriptions. While specific churn reduction numbers are closely held, Netflix's data-driven approach has contributed to a consistently low churn rate compared to industry standards. Their focus on customer engagement and satisfaction, informed by churn prediction, supports customer base growth, with Netflix reporting over 200 million paid memberships worldwide.
- Bank of America has leveraged predictive analytics to identify customers likely to close their accounts or reduce their banking activity. Bank of America has improved customer retention rates through personalized banking solutions and targeted communication strategies. While specific figures are not publicly disclosed, their strategic focus on using data to enhance customer relationships has been instrumental in maintaining a strong and stable customer base.
- Amazon uses churn prediction algorithms to track customer satisfaction and predict potential churn by analyzing order history, return rates, reviews, and customer queries. Amazon's ability to anticipate and address customer needs before they lead to churn contributes to its industry-leading customer retention rates. The company boasts an estimated 90% retention rate for its Prime members, a critical factor in its sustained growth and market dominance. Schedule a call to complement reality with a profitable solution.

Churn Prediction Solution for Large Businesses
When selecting a churn prediction solution, big businesses should consider several key features to ensure the tool effectively meets their needs and seamlessly integrates into their existing workflows.
Ease of Use: The solution should have an intuitive user interface that allows users of varying technical skills to navigate and utilize its features effectively. This includes easy access to data insights, straightforward model training processes, and precise reporting tools.
Customization Options: Each business has unique needs and challenges. The churn prediction solution should offer robust customization options to tailor the predictive models according to specific business metrics, customer segments, and industry factors.
Integration Capabilities: It's crucial that the churn prediction solution can seamlessly integrate with existing CRM systems, data warehouses, and other business intelligence tools. This ensures data flows smoothly between systems, facilitating real-time analysis and actions.
Advanced Analytical and AI Capabilities: Look for solutions that leverage advanced analytics, machine learning, and artificial intelligence to analyze customer data and predict churn. Learning from new data and improving predictions over time is key to staying ahead of churn trends.
Scalability: The solution should be able to scale with your business, handling increasing volumes of data and customer interactions without a drop in performance. Scalability ensures that the tool remains effective as the business grows.
Data Security and Privacy Compliance: Given the sensitive nature of customer data, the solution must adhere to global data protection regulations (GDPR in Europe and CCPA in the USA) and ensure the highest data security and privacy standards.
Support and Training: Ensure that the provider offers comprehensive support and training services to help your team maximize the value of the solution. This includes technical support, user training, and resources for best practices in churn prediction.
Churn Prediction Challenges and Solutions
This matrix demonstrates how businesses can effectively address the challenges associated with churn prediction by adopting targeted solutions backed by examples from various industries that have successfully navigated these obstacles.
The Essential Role of Churn Prediction and Technology Partnerships
Churn prediction is a pivotal strategy for businesses seeking to secure their market position and enhance profitability. By identifying customers likely to disengage, companies proactively address their concerns, tailor personalized experiences, and significantly reduce attrition rates. Advanced analytics and machine learning, offered by technology providers, are crucial in parsing vast datasets to unveil patterns and risk factors associated with churn. These insights enable businesses to refine their product offerings, customer service, and engagement strategies, increasing customer satisfaction and loyalty. Furthermore, technology providers offer scalable solutions that grow with the business, ensuring that churn prediction efforts remain efficient and effective. Please fill out the form, and let's predict the future profitably.
FAQ
What kind of data is needed for effective churn prediction?
Effective churn prediction requires a comprehensive blend of customer interaction data, including usage patterns, transaction histories, service or product feedback, and customer support interactions. Additionally, demographic information and behavioral indicators, such as changes in usage frequency or payment irregularities, are crucial for identifying at-risk customers accurately.
How can businesses integrate churn prediction models into their operations and systems?
Businesses can integrate churn prediction models into their operations by embedding them into existing CRM and data analytics systems, ensuring seamless access to customer insights across all customer touchpoints. This integration facilitates real-time decision-making and targeted action plans for customer retention directly within the workflow of customer service, marketing, and sales teams.
Can churn prediction models be customized to suit the unique needs of a business?
Churn prediction models can be highly customized to suit the unique needs of a business by incorporating specific data points, customer behavior patterns, and industry-specific factors into the model's design. This customization allows for more accurate predictions and targeted retention strategies that align closely with a company's operational context and customer base.
How do changes in market dynamics or customer behavior affect churn prediction models?
Market dynamics or customer behavior changes can significantly impact churn prediction models, necessitating continuous updates and retraining with new data to maintain accuracy and relevance. Failure to adapt models to these changes may result in decreased predictive performance and less effective customer retention strategies.
What steps can businesses take to encourage customer loyalty and reduce the likelihood of churn?
Businesses can encourage customer loyalty and reduce churn by offering personalized experiences, rewards, and incentives tailored to customer preferences and behaviors, alongside maintaining high-quality customer service and support. Regularly soliciting and acting on customer feedback demonstrates a commitment to meeting customer needs and fosters a positive, long-term relationship.