Imagine having a personal movie and TV show curator who always knows what you want to watch. That's exactly what Netflix achieved through the magic of data science. It isn't just about entertainment; it's about the art of turning data into customer satisfaction.
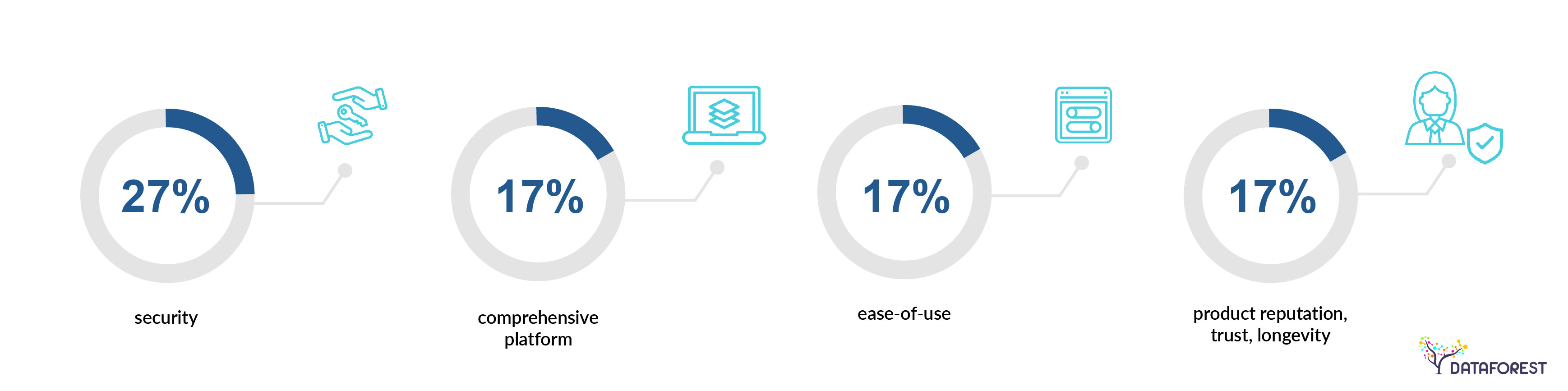
Factors companies consider when selecting analytics soft ware
Extracting Insights from Data for Modern Progress
Data science is an interdisciplinary field that utilizes scientific methods, algorithms, and systems to extract knowledge from structured and unstructured data. Its significance lies in its ability to empower businesses to make data-driven decisions. Data science is the key to harnessing the vast data available today to remain competitive in various industries. Select what you need and schedule a call.
The Fusion of Math, Computer Science, and Domain Expertise
Data science is drawing from a diverse range of fields to harness the power of data.
- Statistics forms the backbone of data science, providing the tools and techniques to analyze data, make predictions, and test hypotheses.
- Data science relies heavily on computer science for data handling, storage, and the development of algorithms. Programming languages Python and R are the best for data manipulation.
- The mathematical underpinnings of data science are essential, from linear algebra for machine learning to calculus for modeling complex relationships.
- Every field of data science requires a deep understanding of the domain it serves, be it healthcare, finance, marketing, or any other industry.
- Data engineering ensures that the data is collected, stored, and processed efficiently.
- Advanced machine learning enables predictive data science analytics, anomaly detection, and pattern recognition, allowing us to create smart algorithms that learn and adapt.
- The art of data visualization uses design principles and psychology to communicate complex data in a way that's understandable and engaging.
The ability to blend these various fields harmoniously is what makes advanced data science such a powerful force. If you think this is your case, then arrange a call.
The Four Pillars of Data Science
Data science calls for several key components, each crucial in extracting valuable insights.
Data Collection
It is the first step in the data science process. It's taking raw materials for a construction project. Data comes from various sources, including sensors, surveys, databases, social media, and more. The quality and quantity of data collected significantly impact the success of a data science project. Consider it as gathering the right ingredients for a recipe; you want fresh, high-quality produce to create a delicious dish.
Data Cleaning (Data Preprocessing)
Once you've collected the data, it often arrives messy. Data cleaning, or preprocessing, is like washing vegetables before cooking a meal. It requires removing errors, duplicates, outliers, and inconsistencies. Data cleaning ensures the data is accurate, complete, and ready for analysis. This step is necessary for the analysis to produce accurate or biased results.
Data Analysis
Data analysis is the heart of data science, where the real magic happens. This step involves using statistical techniques, machine learning algorithms, and domain knowledge to uncover patterns, relationships, and insights. It's solving a jigsaw puzzle where you're trying to piece together a meaningful picture from scattered fragments. Data analysis helps in making predictions, identifying trends, and making informed decisions.
Data Visualization
Data visualization is presenting the results of data analysis clearly. It's taking the delicious meal you've cooked and plating it beautifully. Visualizations, such as charts, graphs, and interactive dashboards, make complex data accessible to a broader audience. They help convey insights, tell a story, and enable stakeholders to make decisions.
Mastering Data Science
Data has become the lifeblood of modern problem-solving, making data scientists the contemporary heroes of the data-rich age.
Components of Data Science in Industries
- Retail data collection exemplifies how e-commerce giants like Amazon track user behavior, purchase history, and preferences to personalize recommendations. Data mining drives customer satisfaction and sales.
- In space exploration, data cleaning plays a critical role. The Mars Rover mission depended on accurate sensor data and meticulous data cleaning to ensure success. We don't even want to imagine the consequences of unclean data in such a scenario.
- In public health data, scientists used historical health data to predict disease outbreaks, such as the application of data analysis in tracking the spread of infectious diseases like COVID-19.
- A financial institution uses interactive dashboards and visualizations to gain real-time insights into market trends and investment opportunities. So, data visualization aids in informed financial decisions.
The Three Pillars of Data Science
- Data collection sets the stage by gathering raw data, data cleaning ensures data quality, and data analysis transforms this data into valuable insights.
- Data science is an iterative process, so three pillars are revisited as understanding deepens. Each iteration refines the process, resulting in more accurate insights.
- Data science serves as a bridge between past data and future insights, helping teams make data-driven solutions, innovate, and adapt in an ever-changing world.
The Data Science Behind Personalized Recommendations
These are just five of the many popular data science applications. Its versatility has led to its widespread adoption across industries and domains.
Healthcare and Medical Diagnosis
Data science revolutionizes healthcare by analyzing patient data for early disease detection, treatment optimization, drug discovery, and predictive healthcare outcomes.
E-commerce and Recommendation Systems
In online shopping, data science powers recommendation engines, improving customer engagement and sales.
Finance and Risk Management
The financial industry utilizes data science for risk assessment, fraud detection, algorithmic trading, and portfolio optimization, enabling more informed investment and financial decisions.
Marketing and Customer Insights
Data science helps businesses target marketing efforts effectively by analyzing customer behavior, optimizing ad campaigns, and measuring marketing ROI, resulting in improved customer acquisition and retention.
Transportation and Logistics
In transportation and logistics, data science optimizes routes, tracks shipments, and minimizes fuel consumption, leading to cost savings and efficient supply chain management.
The Moral Compass of the Digital Age
Ethical considerations in data science are paramount as the field deals with sensitive and potentially impactful information. Here are some key ethical considerations:
- Data scientists should ensure that personal data is collected and used with consent.
- Data scientists should be transparent about data use and the methods employed.
- Biased data can lead to unfair or discriminatory outcomes.
- If a model has adverse effects, there should be mechanisms to rectify them.
- Safeguards should be in place to prevent unauthorized access or data leaks.
- When collecting data, individuals should understand how their data will be used.
- Defining data ownership and rights is crucial, especially when data is shared.
- Ethical considerations intensify when working with sensitive data.
- Data science should respect human rights.
- Adherence to relevant laws, such as GDPR in Europe or HIPAA in healthcare, is crucial.
Ethical considerations in data science evolve with the field and may vary by context, but they are central to ensuring that data science is used responsibly and for the greater good.

Top benefits companies get from using analytics
Benefits of Data Science in Business
Data science benefits businesses (DATAFOREST, as the provider, gives them, too). Here are some advantages of data science:
- Informed Decision-Making
- Efficiency and Optimization
- Enhanced Customer Experience
- Competitive Advantage
- Risk Management
Data science as a service is available not only to large corporations but also to medium and small businesses. Please fill out the form, and we’ll tell you exactly how it will happen.
FAQ
What is data science, and how does it differ from traditional statistics?
It differs from traditional statistics in its broader scope, encompassing data collection, data cleaning, machine learning, and data visualization, and often focuses on analyzing vast and complex data science datasets to uncover hidden patterns and make predictions. In contrast, traditional statistics typically involves analyzing smaller, pre-defined datasets to test hypotheses and describe relationships within the data.
What is the difference between data science and data analytics?
Data science involves a broader spectrum of activities, including data collection, cleaning, analysis, and machine learning, and often focuses on extracting valuable insights and making predictions from data. Data analytics, on the other hand, primarily focuses on examining historical data to provide insights that inform decision-making and business strategy.
What is the difference between business intelligence and data science?
Business intelligence (BI) focuses on querying and reporting past data to support business decision-making, providing descriptive and diagnostic insights. Data science, on the other hand, encompasses a broader range of activities, including data collection, analysis, machine learning, and predictive modeling, to uncover valuable insights and make data-driven predictions, often delving into more complex and future-oriented analysis.
Can you give me a simple example of how data science is used daily?
In everyday life, data science is behind the personalized movie or music recommendations you receive from streaming platforms like Netflix or Spotify. They use data science algorithms to analyze your viewing or listening history, predict your preferences, and suggest content you'll likely enjoy, making your entertainment experience more tailored and engaging.
What is the difference between data science and business analytics?
Data science focuses on a broader and more technical range of tasks, including data collection, cleaning, machine learning, and predictive modeling, to extract valuable insights. Business analytics primarily deals with statistical analysis, reporting, and data visualization to support business decision-making, providing descriptive and diagnostic insights without delving deeply into machine learning or predictive modeling.