In the age of big data, manufacturing is getting a major makeover thanks to data science. This is more than a passing trend; it's a whole new industrial era. From refining demand predictions to making inventory management a breeze and foreseeing machine failures, data science gives manufacturers the toolkit they need to excel.
In this article, DATAFOREST company will explore the multifaceted impact of data science on the manufacturing industry. Keep reading!
Understanding Data in the Manufacturing World
Managing a manufacturing business is like walking a tightrope while juggling—complex and risky. Issues range from supply chain headaches to the tricky terrain of data privacy and security. Data science serves as a safety net and a guide, turning stumbling blocks into stepping stones via smart predictive analytics.
Explore the diverse range of data science success stories in e-commerce, retail, finance, cybersecurity, marketing, real estate, pharma, and insurance industries. When it comes to data science in manufacturing, we're talking about game-changing improvements, not just minor tweaks:
Types of Data Generated in the Manufacturing Process
Manufacturing is a complex landscape, generating a plethora of different data types. This varied data landscape is integral to integrating data science into manufacturing. Here's a deep dive into the types of data you're likely to encounter:
- Sensor Data: These are real-time readings collected from IoT sensors on manufacturing machinery. Sensor data is crucial for data science-driven predictive maintenance and real-time machine performance assessments.
- Machine Data: This includes metrics from the machines themselves, providing a snapshot of how efficiently your assets are operating. Data science can analyze this machine data to identify bottlenecks or inefficiencies in your manufacturing processes.
- Production Data: Data science finds a goldmine in production data, which captures the intricacies of quantities produced, downtime, and overall production rates. Data science can help streamline manufacturing operations and improve productivity by analyzing production data.
- Quality Data: Quality data emerges from quality-control tests and provides insights into the standard and reliability of your products. Data science methods can be applied to quality data for more rigorous, real-time quality checks, lowering defect rates and improving customer satisfaction.
- Inventory Data: This data relates to stock levels and is invaluable for optimizing inventory management. Through data science, predictive models can be developed to suggest optimal times for restocking, reducing carrying costs.
- Maintenance Data: Maintenance data logs details about equipment upkeep, repairs, and overall machinery health. Data science can use this information to devise more effective, predictive maintenance schedules, reducing downtime and costs.
- Process Data: Data science can dissect process data pertaining to manufacturing workflows and procedures. The analysis can uncover ways to make these processes more efficient or to identify steps that might be eliminated or automated.
- Supply Chain Data: Supply chain data gives insights into supplier reliability, logistics, and the flow of materials from origin to your factory. By applying data science techniques to this type of data, manufacturers can optimize routes, reduce transportation costs, and increase the reliability of material supply.
- Customer Feedback: Although not a traditional type of manufacturing data, customer feedback can offer unique perspectives on how products are received in the market. Data science can analyze this information for trends to help forecast demand and product enhancements.
Data science is the keystone for making sense of the multitude of data types generated in manufacturing. Whether predicting machinery failure from sensor data, enhancing product quality, or optimizing supply chain logistics, data science is indispensable in modern manufacturing. For the same purpose, you can book a call to us.

Sources of Data Collection and Generation in the Manufacturing Environment
Data in the manufacturing sector comes from a tapestry of sources.
- IoT Devices are your on-the-ground reporters, feeding back granular data from the factory floor.
- Quality Tests: Customized tests and inspections provide product-specific data, key to maintaining high standards.
- Supplier Interactions: These interactions produce supply chain data that can make or break your inventory management.
- Employee Inputs: Never underestimate the power of human observation. Workers often input crucial data that machines might miss.
- Market Trends: Keep an eye on the market pulse. It can offer invaluable insights for demand forecasting.
The wide variety of data sources is a gold mine for data scientists, enabling manufacturers to make comprehensive, informed decisions.
Possible Challenges in Managing and Harnessing Vast Amounts of Manufacturing Data
Given the sheer volume and diversity of information available, harnessing data for actionable insights is no small feat. Here are some significant challenges:
- Data Volume and Variety: Managing a tidal wave of data, often in multiple formats, is a Herculean task.
- Real-time Processing: For optimal performance, real-time data processing is critical. But it's easier said than done.
- Data Quality and Accuracy: Incorrect or outdated data can lead you astray. Achieving data quality is a constant challenge.
- Data Security and Privacy: With significant data comes great responsibility. Ensuring data privacy security is non-negotiable.
- Analytics and Insights: Extracting actionable insights from raw data is the end goal, but it requires advanced analytics skills and software.
Despite these challenges, data science in the manufacturing industry offers a compelling solution. Advanced data science techniques, such as predictive analytics and machine learning, offer a lifeline. For example, predictive models can forecast when a machine is likely to fail, allowing for preemptive maintenance and minimizing downtime. Real-time analytics can also optimize supply chain performance and resource allocation, improving efficiency and cutting costs.
The data science in manufacturing isn't just an add-on; it's a transformative force that enables companies to unlock their full potential. If you want to always be on the cutting edge of technology, book a call.
Leveraging Data Science in Manufacturing: A Roadmap for Success
Data science is revolutionizing the manufacturing process by uncovering valuable insights from large volumes of data. From data integration to workflow automation, these tools can optimize your operations and boost efficiency.
Here are some key areas where data science is making significant contributions:
Predictive Maintenance: Say Goodbye to Unplanned Downtime
Traditional maintenance approaches wait for equipment failure, leading to unexpected disruptions and costs. Data science utilizes real-time data and advanced algorithms to predict equipment failures, allowing for proactive scheduling of maintenance tasks and reducing unplanned downtime.
Quality Control and Defect Detection: Ensuring Consistency and Excellence
Data science enhances quality control by analyzing sensor data in real time. Machine learning algorithms identify subtle irregularities or defects that may go unnoticed by humans, enabling manufacturers to address issues early, reduce waste, and deliver consistently high-quality products.
Supply Chain Optimization: Boosting Efficiency through Data-Driven Insights
Analyzing everything from demand patterns to supplier reliability, data science helps manufacturers optimize their supply chains. This leads to better inventory management, reduced lead times, and minimized costs, resulting in a more efficient and resilient supply chain.
Production Process Optimization: Maximizing Efficiency and Resource Utilization
Data science helps manufacturers optimize production processes by identifying bottlenecks and inefficiencies. By mapping out the production chain, manufacturers can make data-driven decisions to speed production, reduce costs, and allocate resources more effectively.
Energy Efficiency and Sustainability: Driving Positive Change
Data science has a crucial role in promoting sustainability by analyzing energy consumption patterns and identifying areas for improvement. This reduces the environmental impact and translates into significant cost savings for manufacturing operations.
In conclusion, data science is an indispensable tool for modern manufacturers seeking to improve efficiency, cut costs, and stay competitive in a rapidly evolving market. By harnessing the power of data, manufacturers can optimize maintenance, enhance quality control, streamline supply chains, improve production processes, and become more environmentally sustainable.
Gain a clear view of the reality of data science trends in 2023. Stay up to date with the latest advancements and developments in the field.

The Impact of AI and Machine Learning on Manufacturing: Driving Innovation and Efficiency
Based on World Economic Forum research, the manufacturing industry is experiencing a data-driven revolution by leveraging data and analytics to drive productivity, enhance customer experiences, and improve the societal and environmental impact of companies.
A study conducted by MIT and McKinsey & Company highlights a comprehensive survey of 100 companies to understand how leading adopters successfully utilize machine learning and data analytics in their manufacturing and operations. The study found that companies with the most significant benefits from digital technologies had strong governance, deployment strategies, partnerships, MI-trained employees, and data availability. These successful companies also invested up to 60% more in machine learning compared to their competitors. The integration of Artificial Intelligence (AI) and Machine Learning (ML) into the manufacturing sector is ushering in a transformative era of industry, characterized by increased efficiency, intelligent solutions, and enhanced adaptability.
Let's explore how these technologies are reshaping the manufacturing landscape.
AI and ML: Unveiling a New Era of Manufacturing Excellence Through Data Science
In its simplest form, AI (Artificial Intelligence) and ML (Machine Learning) allow machines to learn and make decisions based on data, eradicating the need for manual programming. In the realm of manufacturing, data science amplifies the impact of these technologies. By analyzing vast swaths of machinery, sensor, and production line data, data science powered by AI and ML provides actionable insights. The result? A smarter, more agile manufacturing approach finely tuned to meet the fluctuations of market demand.
Real-Time Analytics and Anomaly Detection: Data Science as the Watchful Protector of Your Operations
When it comes to real-time analytics and anomaly detection, data science is the linchpin. AI algorithms that continuously sift through production data can quickly identify any anomalies or deviations. This swift identification allows for immediate corrective actions, whether that's mechanical fixes or quality adjustments. Thanks to data science, operational efficiency gets a significant boost, reducing unplanned downtime.
Robotics and Autonomous Systems: Data Science Ushers in Next-Level Factory Floor Efficiency
AI isn't just smart; it's trainable. This opens doors for robotics and autonomous systems that can perform complex tasks with high precision and little human oversight. Data science comes into play here by optimizing these AI-driven systems for maximum efficiency and adaptability, fundamentally transforming how manufacturing floors operate.
Demand Forecasting and Inventory Management: Data Science Makes Accurate Predictions a Reality
Inventory nightmares, be gone! Data science, aided by AI and ML, crunches historical sales data, market trends, and other variables to provide highly accurate demand forecasts. This invaluable data allows manufacturers to tweak production rates and fine-tune inventory, leading to cost-efficient, just-in-time supply chains.
Customization in the Age of AI: Data Science Enables Personalized Manufacturing
Consumers nowadays crave personalized experiences. In manufacturing, data science coupled with AI and ML can fulfill these demands in spades. Whether it's custom configurations or trend predictions, data science is at the heart of reshaping product design to meet individual customer preferences, thereby elevating customer satisfaction levels.
The Future of Manufacturing: Data Science Reveals Limitless Potential
As we gaze into the future, the potential for integrating data science, AI, and ML in manufacturing is boundless. From smarter predictive maintenance and adaptive supply chain management to continuous improvements powered by real-time data, data science is the catalyst.
In conclusion, the fusion of AI and ML with data science in manufacturing isn't just a fleeting trend but a game-changing paradigm shift. Early adopters of these data science technologies are set not just to survive but to excel in an era marked by relentless innovation and shifting market dynamics.
Navigating the Data Security Maze: Privacy and Protection in Modern Manufacturing
The Importance of Data Security in Manufacturing
In our data-centric world, manufacturing is no exception to the critical need for robust data security and privacy protocols. As manufacturing ecosystems grow increasingly complex and interconnected, the imperative to protect sensitive data cannot be overstated. Effective data governance is key to maintaining stakeholder trust and ensuring competitive advantage.
Solving the Data Privacy Puzzle
Manufacturers juggle an extensive array of data, from intricate production metrics to sensitive customer information. The challenge is to institute robust privacy measures that offer both security and functional utility. Properly designed data collection protocols, coupled with best-practice data handling techniques, set the stage for secure operations. Encryption methods and data anonymization are invaluable tools in this effort, safeguarding data while preserving its utility for analytical applications.
Safeguarding Intellectual Capital
Manufacturing sectors often hold a wealth of intellectual property—from proprietary design blueprints to unique production methodologies. Protecting these valuable assets from cyber threats and industrial espionage is paramount for both business sustainability and competitive positioning. Implementing stringent access controls, coupled with cutting-edge intrusion detection systems, can significantly mitigate these risks.
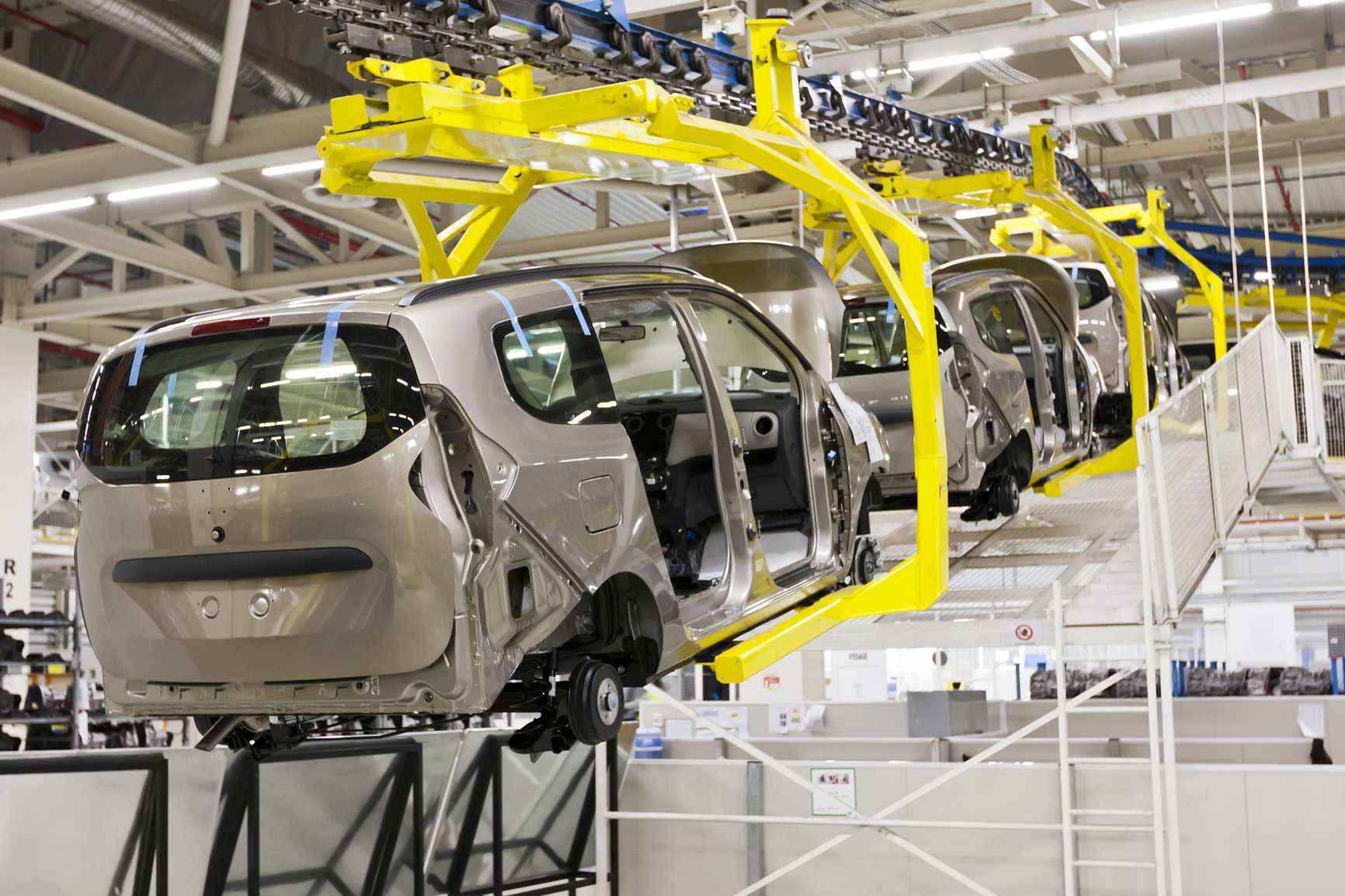
Regulatory Compliance: More than Just a Checkbox
In an era marked by stringent data governance regulations like GDPR and CCPA, compliance is not merely a legal necessity but a brand statement. Manufacturers must establish transparent, fully compliant data governance structures. This involves formulating clear policies covering data collection, storage, and sharing, and enforcing compliance through regular audits and ongoing adjustments to account for regulatory changes.
As manufacturing embraces the transformative capabilities of AI and ML, equally robust advances in data security and compliance must follow suit. The objective is to create an environment where innovation and data-driven insights are balanced with uncompromising data security and regulatory compliance. This holistic approach ensures not only business competitiveness but also the resilience and integrity of modern manufacturing ecosystems.
Navigating the Complexity: The Challenges and Opportunities of Data Science in Manufacturing
The application of data science in manufacturing industry is revolutionizing the way products are made, optimized, and delivered to customers, often leading to more competitive prices. While data science promises a transformative impact on manufacturing, this journey comes with its unique set of complexities and roadblocks. Recognizing these challenges is the first step toward realizing the game-changing potential that data science can offer.
Cultural Resistance and Financial Constraints
Change, especially of a technological kind, is often met with resistance from traditionalists who are more comfortable with manual processes. Coupled with the initial financial outlay for software and infrastructure upgrades, these barriers may deter especially smaller firms from adopting a data-centric model. To mitigate these hurdles, it's essential to align data science objectives with the organization's broader mission. Stakeholder buy-in and a well-articulated strategy can spell the difference between a project that stumbles and one that elevates the entire organization.
The Gordian Knot of Data Silos and Integration
Data silos—repositories of isolated information within departments—complicate the data science landscape. These silos result in fragmented information that undermines efforts for a holistic approach to data analytics. The remedy is to implement a unified data architecture that fosters information-sharing across the organization. By standardizing data formats and adopting robust data governance frameworks, manufacturers can dismantle these silos, enabling more coherent and actionable insights.

Talent Gap: The Crucial Role of Data Scientists
Data science is not a plug-and-play solution; it demands specific skills for effective implementation and interpretation. A key challenge is the scarcity of highly skilled data scientists, a sought-after role across various industries. Here, investment in workforce development becomes non-negotiable. Upskilling existing staff or hiring and training new talents in data science will not only empower the organization but also make it more adaptable to rapid technological changes.
As our organization spearheads the integration of data science into manufacturing, DATAFOREST is a champion of a new paradigm of operational excellence. From predictive analytics that ensure seamless workflow to the nuance of personalized product designs, we are translating data into a strategic advantage. We acknowledge challenges like data silos and security but address them with tailored solutions that resonate with the unique needs of the manufacturing sector. As we continue to navigate the intricate landscape of data and production, our unwavering commitment is to steer manufacturing toward a future defined by efficiency, high-quality outputs, and sustainable practices. In embracing these challenges as opportunities for growth, we cement our role as architects of a manufacturing landscape that is both data-driven and deeply attuned to the evolving needs of the digital age.
DATAFOREST offers comprehensive data science services. Our team of experts specializes in predictive analytics, data insights, and business process automation. Find out how our data science solutions can help your business thrive.
If you have any questions or would like to discuss your project in detail, please feel free to contact us. We are here to help.
FAQ
How Does Data Science Transform Predictive Maintenance in Manufacturing?
In the manufacturing industry, predictive maintenance has been completely revamped by data science. Using real-time sensor data and machine learning algorithms, data science for manufacturing identifies patterns that point toward looming equipment failures. Maintenance teams can then take preemptive action to schedule repairs, drastically reducing downtime and saving money. This optimization of maintenance schedules, powered by production data science, makes the whole operation more efficient and cost-effective.
Can Data Science Improve Product Quality and Lower Defect Rates?
Absolutely! Data science in manufacturing plays a critical role in amplifying product quality and pinpointing defects. By leveraging machine learning to analyze data gathered during the manufacturing process, variations or inconsistencies that may indicate defects can be detected earlier. This early warning system improves the quality of the end product and significantly reduces waste. It's a win-win: better products and happier customers, thanks to data science in the manufacturing industry.
How Does Data Science Fuel Continuous Improvement in Manufacturing Processes?
Data science isn't just about solving existing problems; it's about continuous improvement. By diving deep into both historical and real-time data, production data science helps manufacturers pinpoint inefficiencies and bottlenecks in their operations. This treasure trove of insights offers avenues for fine-tuning workflows, cutting down cycle times, and boosting overall productivity. The iterative application of data science in manufacturing creates a cycle of constant refinement and growth.
Can Data Science Boost Sustainability in Manufacturing Operations?
Certainly! One of the lesser-known applications of data science in the manufacturing industry is its potential to significantly enhance sustainability. By analyzing energy use, waste generation, and supply chain logistics, manufacturers can unearth opportunities for resource optimization and waste reduction. From adjusting production schedules to reduce energy costs to streamlining transportation routes for lower emissions, data science for manufacturing is an ally in achieving sustainability goals—while often saving money and elevating brand reputation in the process.
How Can Smaller Manufacturers Leverage Data Science?
Don't think you need to be a manufacturing giant to benefit from data science. Small and medium-sized manufacturers can also harness the power of data science in manufacturing. Even with limited resources, these businesses can employ data analytics for actionable insights that can lead to better decision-making and sharpened competitiveness. Whether it's optimizing production processes or perfecting inventory management, the tools of data science in production are becoming increasingly accessible, especially through cloud-based platforms.
With data science becoming an integral part of the manufacturing landscape, it's not just a buzzword but a fundamental tool for efficiency, innovation, and sustainability. Whether you're a large enterprise or a small manufacturer, the message is clear: data science in manufacturing isn't an option; it's a necessity.